Abstract
The use of meteorological autocorrelation variables and pollen concentrations from previous days, coupled with classification of meteorological data according to multivariate analysis techniques, is shown to improve the predictive power of multiple regression models for daily pollen forecasts. This paper presents an investigation of the meteorological and autocorrelation variables which influence pollen counts in Cartagena, from 1995 to 1999, as a basis for the development of predictive models. The analysis of total pollen concentrations, and especially Chenopodiaceae‐Amaranthaceae, was determined. Initially, forecasting models for total pollen counts were developed, using data from 1995 to 1998, and autocorrelation and meteorological variables. Secondly, predictive models were developed for different meteorological situations, which improved the results by decreasing the number of predictive parameters. Finally, data from 1999 were used to validate the predictive models.
Knowledge of airborne pollen concentration has proved to be of considerable importance for humans in fields such as agronomy, ecology, biology, allergy and occupation hygiene (Galán et al. Citation1995, Fornaciari et al. Citation1998). An accurate forecast of these concentrations would benefit pollinosis patients (Bringfelt et al. Citation1982, Antépara et al. Citation1995, Norris‐Hill Citation1995, Dahl & Strandhede Citation1996), as well as a prediction tool for different crops (Fornaciari et al. Citation1998).
Daily pollen counts vary as a function of multiple parameters, e.g., flowering patterns or meteorological conditions (Hyde & Adams Citation1960, Bringfelt et al. Citation1982, Andersen Citation1991, Alba et al. Citation2000, Emberlin et al. Citation2002). Therefore modelling these systems may be more complex than for other pollution data, where characteristics of emission source can be clearly established. Two approaches to model the movement of particulate matter in the atmosphere can be used: source and receptor orientated models (Di‐Giovanni et al. Citation1989, Norris‐Hill Citation1995).
Source orientated models use mathematical algorithms to analyse the dispersal of pollen far away from the source, or to define the delivery process. These models simplify the dispersal process, and require some knowledge of the pollen emission for each single source, or use a well‐defined area accounting for all the regions where it is possible to get pollen to the forecasting place. Emission and dispersion models have been developed to calculate mesoscale distribution of pollen types, where both vegetation maps and pollen sampling data are available (McCartney Citation1994, Kawashima & Takahashi Citation1995).
Receptor orientated models are designed to predict concentrations without knowledge of source conditions, which is often the case in aerobiological systems (Norris‐Hill Citation1995).
Receptor models for pollen concentrations have been used to develop forecasting models using previous time series (Moseholm et al. Citation1987, Emberlin et al. Citation1993), and to predict the severity or the start of the pollen season (Driessen et al. 1990, Andersen Citation1991, Ong et al. Citation1997, Fornaciari et al. Citation1998).
Recently considerable attention has been given to statistical models which use mathematical techniques to forecast the dispersion and distribution of airborne particles (Kolehmainen et al. Citation2001).
The aim of the research reported in this paper is to develop forecasting receptor orientated models using multiple lineal regression techniques for pollen concentration in Cartagena.
In a previous paper (Angosto et al. Citation2002); we have classified the different wind patterns in our city by means of a two‐step cluster analysis, as previously reported by other authors (Kalkstein et al. Citation1987). This classification has been used for developing forecasting models, together with other meteorological parameters, such as temperature, relative humidity, pressure, rain, and daily solar radiation. In order to evaluate long‐term trends, a method of time‐series analysis was also used.
MATERIAL AND METHODS
Total pollen concentrations were measured from 1995 to 1999 using a Hirst‐type volumetric sampler (Hirst sampler, Lanzoni VPSS‐2000), with an adjusted flow of 10 l/min. Particles with a size range between 2 and 200 μm in diameter were captured by means of a Melinex tape (cod. 200.700), impregnated with a silicon mixture, and with a driving speed of 2 mm per hour. The sample was processed as previously described (Moreno‐Grau et al. Citation1998), following the recommended standards of the Spanish Aerobiological Network (REA) (Domínguez et al. Citation1991).
Data collected in Cartagena () were recorded as average daily values, expressed as total pollen grains per cubic meter of air.
In order to avoid the annual variations in pollen concentrations which could mask the real patterns (Emberlin et al. Citation1993), the daily pollen content was standardized following Moseholm et al. (Citation1987), using the following equation:
np =standardized pollen count
pr =daily pollen count
py =total annual pollen concentration
Meteorological parameters selected for this study were daily minimum, average, and maximum values of temperature, relative humidity, air pressure, and wind speed, accumulated daily rainfall, and accumulative sunshine. Wind speed and wind direction were combined to create two categorical variables; wind range and wind course.
Wind course was obtained by grouping wind direction values into 12 classes, all with 30° of amplitude: North (N), North‐Northeast (NNE), East‐Northeast (ENE), East (E), East‐Southeast (ESE), South‐Southeast (SSE), South (S), South‐Southwest (SSW), West‐Southwest (WSW), West (W), West‐Northwest (WNW), and North‐Northwest (NNW).
Average wind speed values were grouped into four wind ranges: Range 1=0–4.9 km/h, Range 2=5–10.9 km/h, Range 3=11–15.9 km/h and Range 4≥16 km/h.
All statistical analyses were carried out using SPSS 10.0 software. First, Pearson's correlation coefficients and bilateral significance tests were used to explore the relationship between meteorological variables and standardized pollen concentrations. The values of these variables recorded during the previous days were also used in this correlation, which could influence in daily pollen counts.
Multiple regression models were developed using the Backward option in SPSS, which removes at each step any variable with a p‐value less than 0.10, 90% significance level (Vinacua Citation1997).
RESULTS AND DISCUSSION
A decrease in total pollen counts was observed from 1995 to 1999, except for 1998, which showed a slight increase relative to 1997 (). The same behavior is seen in Chenopodiaceae‐Amaranthaceae (). The highest total pollen counts were recorded during the month of May for 1995, 1996, and 1997 (), but in 1998 and 1999, the peak day occurred earlier (), probably due to extensive flowering of Cupressaceae.
Table I. Values for total pollen and Chenopodiaceae‐Amaranthaceae counted from 1995–1999, and the main meteorological parameters.
The highest peak for Chenopodiaceae‐Amaranthaceae was always recorded during the month of September (), coincident with the second flowering of this pollen type in our region, which is the most representative of that period (Moreno‐Grau et al. Citation1998).
The decrease observed for pollen counts from 1995 to 1999 can be attributed to the high drought suffered in this area, with a maximum accumulated rainfall of 229.3 mm registered during 1997 (), whilst average value for rainfall is 354.2 mm for the period 1975 to 1994. Other factors affecting the decrease could be changing crop species or substantial increase in the main source area.
The other values for meteorological parameters () are typical of an arid Mediterranean and subtropical climate (Moreno‐Grau et al. Citation1998).
Daily average total pollen concentrations from 1995 to 1999 are depicted with a dashed line (). Two distinct periods can be observed. The first, from January to August, is the main pollen period. It has saw‐toothed structure and includes taxa with pre‐spring and spring flowering. The second, from September to October, is coincident with the second flowering of Chenopodiaceae‐Amaranthaceae (represented by a continuous line).
Fig. 2. Daily average pollen count from 1995 to 1999. Total pollen (dashed line) ‐ Chenopodiaceae‐Amaranthaceae (continuous line).
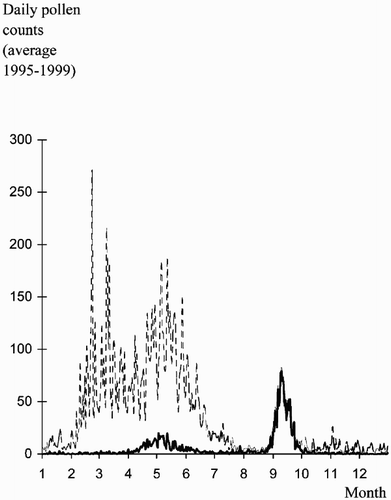
On the other hand, temperatures show an increasing tendency from the beginning of the year until summer time, and a decreasing trend from summer to December.
These trends led us to develop two multiple regression models for pollen forecasting, one for total pollen concentrations, from January to August, and a second one for the highest peak of Chenopodiaceae‐Amaranthaceae (September and October).
As a first step, and following recommendations of different authors (Emberlin et al. Citation1993, Díaz de la Guardia et al. 1998, Stark et al. Citation1997, Galán et al. Citation1995, Citation2000), the relationships between different independent variables and the dependent variable for each model were explored.
Pearson's correlation coefficients, comparing standardized daily average total pollen counts for the first 30 weeks and second flowering of Chenopodiaceae‐Amaranthaceae with different meteorological parameters and variables for the previous days, provides information on which variables correlate with pollen production (). A negative correlation coefficient was found for mean atmospheric pressure (MAP), with a 95% significance level, implying that an increase atmospheric in MAP leads to a decrease in airborne pollen counts. Glassheim et al. (Citation1995) have reported a positive and significant correlation between MAP and pollen concentrations. In our study, the settlement of air masses would reduce the flotation ability for the biggest pollen grains, thus having a stronger effect during the second flowering of Chenopodiaceae‐Amaranthaceae than for total pollen.
Table II. Pearson's correlation coefficients between daily average pollen count meteorological parameters during 1995–1999.
For total pollen, relative humidity (RH) and its minimum daily value (RHMIN) show significant negative correlation. This behavior has already been reported by other authors (Herrero & Fraile Citation1997), and is explained by variation in shape and size of pollen grains according to their hydration level (Blackmore & Barnes Citation1986). As humidity increases, pollen grains increase their ability for wet deposition.
Wind speed (WS) showed a positive and 99% significant correlation with both total and Chenopodiaceae‐Amaranthaceae pollen, which indicates an increase in pollen concentrations with the increase of wind speed. This fact could be conditioned to the distance between the pollen sampler and plant masses: with a shorter distance, wind could act as a dilution factor.
Accumulative sunshine (AS) is positively correlated with total pollen counts, because of its influence with formation and delivery of pollen grains (Akers et al. Citation1979). Similar results have been reported by Bringfelt et al. (Citation1982) and Glassheim et al. (Citation1995). However, the second peak of Chenopodiaceae‐Amaranthaceae displayed a low negative Pearson's correlation coefficient with this meteorological parameter, as shown by a gradual decay of solar radiation during the month of September and an increase of this pollen type. Similar results have been reported by Muñoz et al. (Citation2000) for mean temperature and relative humidity.
For both pollen peaks, there is a 99% significant correlation with standardized pollen counts in the previous three days. This fact is highly influenced by pollen delivery pattern.
The next step was to develop multiple lineal regression models. The first regression models were developed using those meteorological parameters with a significant correlation with pollen counts from 1995 to 1998.
The two best regression models for total pollen and the best for each cluster, (first 30 weeks of the year), are from 1995 to 1998 (). Variables were included in the model following a step forward method. Models 1 and 2 for total pollen yield a determination coefficient of 0.54, when the following variables are included: pollen count of the previous day, minimum relative humidity, pollen count of three days before, wind speed and direction, and the variations of maximum and minimum temperature for four and six days before. Although the value of unexplained variance is still high, determination coefficients are higher than those previously obtained where for single variables and for models and data reported by other authors (Bringfelt et al. Citation1982, Galán et al. Citation1995).
Table III. Results of multiple regression analysis for the two best models for total standardized pollen (first 30 weeks of each year) (1995–1998) and for each cluster.
Wind direction appears to be an important parameter for the amount and type of pollen arriving at the pollen sampler (Herrero & Fraile Citation1997, Moreno‐Grau 1998). For that reason, and in order to achieve a better result for our models, a two‐step cluster multivariate analysis has been used for a daily classification of wind fluxes, previously reported in Angosto et al. (Citation2002). The average and 95% confidence interval data for standardized total pollen concentration includes the period 1995 to 1999 ().
Fig. 3. Average values and 95% confidence interval data for total standardized pollen concentrations for each cluster, from 1995 to 1999.
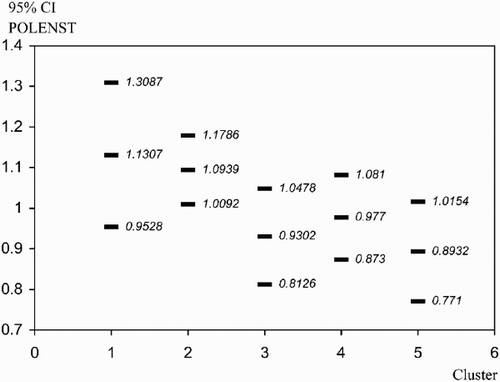
Statistically significant differences were observed between different clusters, which imply that each wind cluster picks up different pollen source areas. The highest pollen counts were obtained for cluster 1, noted for a predominance of NNW and N wind direction, and including high pressures with intermediate wind speed (Angosto et al. Citation2002). This fact could be explained due to the situation of the main vegetation masses in respect to the pollen sampler.
The masses of air coming from the sea are represented by clusters 3 and 4. As reported in a previous paper (Angosto et al. Citation2002), cluster 3 groups low pressure situations with a maximum wind speed in the city and a predominant SSW wind direction. On the other hand, cluster 4 includes high pressure situations, with a maximum of temperature and N and NNE predominant wind directions. Both clusters showed a relatively low pollen count.
The lowest pollen count was achieved for cluster 5, representing the minimum wind speeds without a predominant wind direction.
For these reasons, we consider that cluster variable stands for the influence of wind fluxes, and therefore can be applied to the prediction of pollen concentrations. It is easier to handle this variable for statistical studies and modelling procedures than wind direction variable alone.
The next step was to develop forecasting models for each wind situation (for each cluster). Standardized total pollen concentration was obtained for these models by dividing the original data according to the cluster group corresponding to each day. The best model was determined for each cluster situation (). Except for cluster 4 (C4), all models show stronger determination coefficient than those passed on the full data set. The highest determination coefficient was for cluster 1 (C1), 0.685. Except for cluster 5 (C5), all the cluster models also used a much lower number of predictive variables.
The results of the two best multiple regression models for the second peak of Chenopodiaceae‐Amaranthaceae yielded a similar determination coefficient (), but the first one uses only two variables, standardized pollen count of two and three previous days, and the second model the same two variables plus the maximum pressure value for this day.
Table IV. Results of multiple regression analysis for the two best models obtained for second peak of Chenopodiaceae/Amaranthaceae (1995–1998) and for each cluster.
When the data for this second peak of Chenopodiaceae‐Amaranthaceae were subdivided according to the cluster (see ), stronger r2 values were obtained for C2 and C3, 0.839 and 0.682, and for clusters 1, 4, and 5. The predictive equations explain a similar variance to that explained for the whole sample.
Our next step was validation of the best models with data from 1999. The validation was performed for the best model of total pollen count and second peak of Chenopodiaceae‐Amaranthaceae (model 1) ( & ). Models representing total pollen count by clusters () were also validated. Models by clusters for the second peak of Chenopodiaceae‐Amaranthaceae were not validated because sample size was too small for cluster subsets.
The dispersion plot of predictions and observed data of the best model for total standardized pollen (), with an adjusted determination coefficient of 0.541 is concordant between forecast and observed pollen counts, as shown by the Pearson's correlation coefficient of 0.97 (99% significant−R2 =0.94).
Fig. 4. Validation of the best model for total standardized pollen concentration using data from 1999.
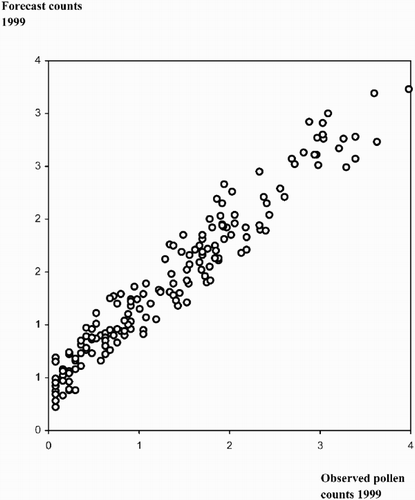
For the calculated and critical values (α=0.05) of variance (F) and mean values (t) (), the critical value is always higher than calculated value. Thus, we cannot reject the null hypothesis of equal means and variances.
Table V. Calculated and critical values (α=0.05) for variance (F) and mean values (t) for both models.
Using data from 1999 a dispersion plot of the validation of the best model corresponding to the second peak of Chenopodiaceae‐Amaranthaceae was constituted (). Although the number of samples is not very high, because of a short flowering period, they seem to fit the model, with a correlation coefficient between predicted and observed data of 0.83 (99% statistically significant). As for the previous model for total pollen concentrations, we cannot reject the null hypothesis for equal means and variances (see ).
The results of the best model validation for total pollen count for each cluster shows that the highest and 99% statistically significant coefficients were achieved for clusters 1, 2, 3, and 5, meanwhile cluster 4 obtained a lower Pearson's correlation coefficient and only 95% significance (). This fact could be explained by the lower number of days which were gathered by that cluster.
Table VI. Determination and correlation coefficients between predicted and calculated data for 1999, for each cluster situation. Calculated and critic values (α=0.05) for variance (F) and mean values (t).
Statistic parameters F and t for test of variance and mean differences showed that null hypothesis could not be rejected for clusters 1, 2, 3, and 5, meanwhile for cluster 4 null hypothesis for variance differences has to be rejected.
CONCLUSIONS
The use of meteorological autocorrelation variables and pollen counts in previous days clearly improved the results of pollen predictive models, both for total and Chenopodiaceae‐Amaranthaceae counts.
Prior classification of meteorological data (a two‐step cluster analysis) and the development of a separate model for each meteorological situation, also improved the forecasting power of the models by decreasing the number of predictive variables.
Models 1 and 2 developed for the forecasting of total pollen concentration could be used in other cities of the Mediterranean area, with similar meteorological characteristics. Meanwhile, models obtained for each cluster could only be used in those cities of the Mediterranean area with wind patterns similar to those identified for the city of Cartagena.
ACKNOWLEDGEMENTS
Authors acknowledge the Environmental Service Department of the City Town of Cartagena for their support with this study.
References
REFERENCES
- Akers TG Edmonds RL Kramer CL Lighthart B McManus ML Schichting HE Solomon AM, Jr Spendlove JC 1979 Modelling of aerobiological systems. – In: Aerobiology: The ecological systems approach (ed. R. L. Edmonds), pp. 11–84 – Dowden, Hutchington & Ross Inc. Philadelphia PA
- Alba , F , Díaz De La Guardia , C and Comtois , P . 2000 . The effect of meteorological parameters on diurnal of airborne olive pollen concentration. . – Grana , 39 : 200 – 208 .
- Andersen , TB . 1991 . A model to predict the beginning of the pollen season. . – Grana , 30 : 269 – 275 .
- Angosto JM Elvira‐Rendueles B Bayo J Moreno J Vergara N Moreno‐Clavel J Moreno‐Grau S 2002 Wind classification through cluster analysis for the development to predictive statistical models on atmospheric pollution. – Air Pollut. 10 635 644 (Comput. Mechan. Publ., Southampton)
- Antépara , I , Fernández , JC , Gamboa , P , Jauregui , I and Miguel , F . 1995 . Pollen allergy in the Bilbao area (European Atlantic seaboard climate): pollination forecasting methods. . – Clin. Exp. Allergy , 25 : 133 – 140 .
- Blackmore S Barnes SH 1986 Harmomegathic mechanisms in pollen grains. – In: Pollen and spores: Form and function (ed. S. Blackmore & I. K. Ferguson), pp. 137–149 – Acad. Press London
- Bringfelt , B , Engström , I and Nilsson , S . 1982 . An evaluation of some models to predict airborne pollen concentration from meteorological conditions in Stockholm, Sweden. . – Grana , 21 : 59 – 64 .
- Dahl , A and Strandhede , S . 1996 . Predicting the intensity of the birch pollen season. . – Aerobiologia , 12 : 97 – 106 .
- Diáz de la Guardia , C , Alba , F , Girón , F and Sabariego , S . 1998 . An aerobiological study of Urticaceae pollen in the city of Granada (S. Spain): correlation with meteorological parameters. . – Grana , 37 : 298 – 304 .
- Di‐Giovanni , F , Beckett , PM and Flenfley , JR . 1989 . Modelling of dispersion and deposition of tree pollen within a forest canopy. . – Grana , 28 : 129 – 139 .
- Domínguez , E , Galán , C , Villamandos , F and Infante , F . 1991 . Handling and evaluation of the data from the aerobiological sampling. . – Monogr. REA/EAN , 1 : 1 – 18 .
- Driesen , MNBM , Van Herpen , RMA and Smithuis , LOMJ . 1990 . Prediction of the start of the grass pollen season for the southern part of the Netherlands. . – Grana , 29 : 76 – 87 .
- Emberlin , J , Detandt , M , Gehrig , R , Jäger , S , Nolard , N and Rantio‐Lehtimäki , A . 2002 . Responses in the start of Betula (birch) pollen seasons to recent changes in spring temperatures across Europe. . – Int. J. Biometeor. , 46 : 159 – 170 .
- Emberlin , J , Savage , J and Jones , S . 1993 . Annual variations in grass pollen seasons in London 1961–1990: trends and forecast models. . – Clin. Exp. Allergy , 23 : 911 – 918 .
- Fornaciari , M , Pieroni , L , Ciuchi , P and Romano , B . 1998 . A regression model for the start of the pollen season in Olea europaea. . – Grana , 37 : 110 – 113 .
- Galán , C , Alcázar , P , Cariñanos , P , García , H and Domínguez‐Vilches , E . 2000 . Meteorological factors affecting daily Urticaceae pollen counts in southwest Spain. . – Int. J. Biometeor. , 43 : 191 – 195 .
- Galán , C , Emberlin , J , Domínguez , E , Bryant , RH and Villamandos , F . 1995 . A comparative analysis of daily variations in the Gramineae pollen counts at Córdoba, Spain and London, UK. . – Grana , 34 : 189 – 198 .
- Glassheim , JW , Ledoux , RA and Vaughan , TR . 1995 . Analysis of meteorologic variables and seasonal aeroallergen pollen counts in Denver, Colorado. . – Ann. Allergy, Asthma Immunol. , 75 : 149 – 156 .
- Herrero , B and Fraile , C . 1997 . Annual variation of airborne pollen in the City of Palencia, Spain, 1990–1992. . – Grana , 36 : 358 – 365 .
- Hyde , HA and Adams , KF . 1960 . Airborne allergens at Cardiff, 1942–1959. . – Acta Allergol. , 15 : 159 – 169 .
- Kalkstein , LS , Tan , G and Skindlov , JA . 1987 . An evaluation of three clustering procedures for use in synoptic climatological classification. . – Am. Meteor. Soc. , 26 : 717 – 730 .
- Kawashima , S and Takahashi , Y . 1995 . Modelling and simulation of mesoscale dispersion processes for airborne cedar pollen. . – Grana , 34 : 142 – 150 .
- Kolehmainen , M , Martikainen , H and Ruuskanen , J . 2001 . Neural networks and periodic components uses in air quality forecasting. . – Atmosph. Environm. , 35 : 815 – 825 .
- McCartney HA 1994 Physical factors in the dispersal of aerobiological particles. – In: Aerobiology. 5th Int. Conf., Bangalore 1994. Proc. (ed. S. N. Agashe) pp. 439–450 – Science Publs Enfield NH
- Moreno‐Grau , S , Bayo , J , Elvira‐Rendueles , B , Angosto , JM , Moreno , JM and Moreno‐Clavel , J . 1998 . Statistical evaluation of three years pollen sampling in Cartagena, Spain. . – Grana , 37 : 41 – 47 .
- Moseholm , L , Weeke , ER and Petersen , BN . 1987 . Forecast of pollen concentrations of Poaceae (grasses) in the air by time series analysis. . – Pollen Spores , 29 : 305 – 322 .
- Muñoz , AF , Silva , I , Tormo , R , Moreno , A and Tavira , J . 2000 . Dispersal of Amaranthaceae and Chenopodiaceae pollen in the atmosphere of Extremadura (SW Spain). . – Grana , 39 : 56 – 62 .
- Norris‐Hill , J . 1995 . The modelling of daily Poaceae pollen concentrations. . – Grana , 34 : 182 – 188 .
- Ong , EK , Taylor , PE and Knox , RB . 1997 . Forecasting the onset of the grass pollen season in Melbourne (Australia). . – Aerobiologia , 13 : 43 – 48 .
- Stark , PC , Ryan , LM , McDonald , JL and Burge , HA . 1997 . Using meteorologic data to predict daily ragweed pollen levels. . – Aerobiología , 13 : 177 – 184 .
- Vinacua B 1997 Análisis estadístico con SPSS para Windows. Estadística básica. – McGraw‐Hill Madrid