Abstract
Specific airborne fungal spore types trigger respiratory allergy symptoms in sensitive individuals. Aiming to reduce the risk for allergic patients, we constructed predictive models for the fungal spore circulation in Thessaloniki, Greece. Monthly and daily autoregressive forecasting models were developed (Dynamic Regression) for the airborne spore concentrations of Alternaria and Cladosporium, the most abundant fungal taxa in the area. The forecast horizons were respectively 12 months and one week. The accuracy of each predictive model was tested by means of six statistical criteria. Special attention was paid to the lag effect of all factors, both meteorological and fungal spore records. Aerobiological sampling was conducted over 1996–2002, using a Burkard trap. Records of 18 meteorological parameters were used for the same period. Residual analyses tested the adequacy of the models. Monthly forecasting models were highly significant, with adjusted R2 = 0.68 for Alternaria, and 0.81 for Cladosporium. The respective values of adjusted R2 for the daily models were 0.62 and 0.70. Cladosporium spore counts were consistently influenced by solar radiation, whereas Alternaria was influenced by air temperature (mean and minimum). For monthly forecasts, records of the preceding month, and the 12 month spore record was significant for Alternaria, whereas for Cladosporium, the lags were 12 and 24 months. With the daily models, the respective required periods were mainly the preceding one to two weeks and the last two years for both fungal taxa, in addition to a one‐year lag in the case of Cladosporium. Daily models could be further improved by co‐estimating interdiurnal variability and fungal spore sources.
Forecasting of aeroallergens (pollen grains and fungal spores) has been a popular scientific goal for many researchers. However, the majority of these efforts have more frequently aimed at the prediction of airborne pollen count; advanced forecasting models of airborne fungal spore circulation are comparatively few (Katial et al. Citation1997), and most of them usually display low predictability (Angulo‐Romero et al. Citation1999; Mitakakis et al. Citation2001; Troutt & Levetin, Citation2001; Stennett & Beggs, Citation2004).
Fungal spores of specific taxa are very often implicated in respiratory allergy symptoms (Solomon, Citation1978; Hasnain et al. Citation1994; D'Amato et al. Citation1997; Halonen et al. Citation1997; Corsico et al. Citation1998; Resano et al. Citation1998; Mari et al. Citation2003; Gioulekas et al. Citation2004). Mostly fungal spores – and not pollen grains – have been reported to be responsible for hospital admissions related to asthma attacks or even acute respiratory failure (O'Hollaren et al. Citation1991; Kauffman et al. Citation1995; Dales et al. Citation2000; Bush & Prochnau, Citation2004); hence, these kinds of respiratory diseases are usually well documented for children and young adults (O'Hollaren et al. Citation1991; Targonski et al. Citation1995; Dales et al. Citation2000; Downs et al. Citation2001; Verini et al. Citation2001; Bush & Prochnau, Citation2004). There is an urgent need for developing advanced forecasting models in order to reduce the risk for allergic patients.
The dispersal and transport of airborne fungal spores — and therefore the predictive power of created statistical models — are influenced by several meteorological factors, such as wind, rainfall and air temperature components, atmospheric pressure, solar radiation, and relative humidity (Solomon, Citation1978; Hjelmroos, Citation1993; Katial et al. Citation1997; Kurkela, Citation1997; Angulo‐Romero et al. Citation1999; Corden & Millington, Citation2001; Mitakakis et al. Citation2001; Munuera Giner et al. Citation2001; Troutt & Levetin, Citation2001; Stennett & Beggs, Citation2004). Nevertheless, the exact relationship of spore counts and meteorological factors is not thoroughly understood. Moreover, the indirect effect and time‐delayed interrelationship has rarely been investigated and forecasts have been created either for rather short time‐horizons or with limited accuracy (Mitakakis et al. Citation2001; Stennett & Beggs, Citation2004).
The aim of this study was to examine the relationship between the atmospheric fungal spore content and the prevailing meteorological parameters in the area of Thessaloniki, the second‐largest city in Greece. No predictive modelling of the aeroallergen circulation season has been developed in Greece before. Our ultimate goal was to create forecasting models of high predictability which might be applicable to other regions as well.
Material and methods
Statistical method
Autoregressive models were processed, using the statistical technique of Dynamic Regression (Stergiou et al. Citation1997) and the program of Forecast Pro (Business Forecast Systems, USA, Business Forecast Systems, 1992–Citation1995).
Equation of Dynamic Regression:
Where:
Yt – Dependent variable | |||||
Xt – Independent variable | |||||
c – constant | |||||
aκ – Autoregressive term of the dependent variable | |||||
Yt‐κ – Dependent variable with a lag (t‐κ) | |||||
bμ – Lagged term of the independent variable | |||||
Xt‐μ – Independent variable with a lag (t‐μ) | |||||
θλ – Cochrane‐Orcutt autoregressive error term | |||||
et‐λ – Auto‐regression error. |
This statistical method refers to a multivariate regression technique, which performs time series analysis with the power to investigate serial correlations both among dependent and independent variables. Thus, it refers not only to the direct ‘dose‐response effect’, but also to the construction of time‐delayed relations (lags) among various variables (Chatfield, Citation1989). It is well known that environmental data collected over consecutive time periods are often serially correlated, with data closely associated having higher correlations between them than data farther apart (Katial et al. Citation1997; Mitakakis et al. Citation2001; Munuera Giner et al. Citation2001; Stennett & Beggs, Citation2004). Similarly to dependent and independent variables, regression errors could additionally be correlated serially. Misleading results (high R2 but low fitting/forecasting power) might be obtained if this kind of relationship is neglected (Chatfield, Citation1989). For this reason, the Cochrane‐Orcutt autoregressive error term was inserted in our models (Business Forecast Systems, 1992–Citation1995). Moreover, via Dynamic Regression we decomposed our fungal spore time series in order to describe the behaviour of airborne spore levels over time (existing trends, seasonality, irregularities).
The accuracy of the created predictive models of airborne spore circulation was tested with six different criteria (Business Forecast Systems, 1992–Citation1995; Stergiou et al. Citation1997), as follows:
Regression coefficient R2: the fraction of the sample variance explained by the model.
Ljung‐Box test (Q statistic): checks for autocorrelation in the first several lags of the residual errors. If the Ljung‐Box test is significant for a correlation model then the model needs improvement. The test is significant if its probability is >0.99.
Forecast error: standard error of the within‐sample forecasts, computed by running the forecast model through the historic data. It is used as an estimate of the one‐step forecast error.
Mean Absolute Percent Error (MAPE): within sample the MAPE is a measure of goodness‐of‐fit. Out‐of‐sample it is a measure of actual forecasting accuracy. The MAPE is an important evaluation statistic in the time series analysis.
Mean Absolute Deviation (MAD): the average of the absolute values of the errors. This is a measure of goodness‐of‐fit and an important statistic in time series analysis.
Root Mean Square Error (RMSE): the square root of the average of the squared errors. It comprises an important measure of goodness‐of‐fit.
All data were processed: 1) as summed monthly values, and 2) as daily values, over the period 1996–2002. Variables were not transformed (e.g. logarithmically) a priori; we processed the raw values of all variables, so as not to obscure a probable non‐linearity of occurring models, and because data transformations sometimes underestimate the regression residuals. Residual analyses of the created forecasting models were conducted in order to check the models' performance (Chatfield, Citation1989; Zar, Citation1999). Similarly, we tested the autocorrelation function of the models' residual errors, by means of correlograms and Q statistic probability. These tests in fact refer to the serial interdependence of regression errors and comprise white‐noise estimates (Chatfield, Citation1989; Business Forecast Systems, 1992–Citation1995). Finally, hold‐out samples for both monthly and daily models were taken – with a forecast horizon of one year and one week, respectively – and were checked against the actual values of each time series, so as to verify the accuracy of the constructed predictive models (Chatfield, Citation1989; Stergiou et al. Citation1997).
Fungal spore counts
Daily and monthly summed counts of airborne fungal spores were used from 1996–2002 data. The records were performed using a 7‐day recording Burkard volumetric trap (Burkard Manufacturing, Rickmansworth, Hertfordshire, England), a worldwide‐accepted instrument for aerobiological sampling. The methodology followed was exactly the one described by most scientists (e.g. Solomon, Citation1978; British Aerobiology Federation, Citation1995; Gioulekas et al. Citation2004). In brief, each weekly tape was cut into seven pieces – each corresponding to a day of the weekly sampling. Afterwards these were stained (with a solution of saffron, gelatine, glycerol, and phenol) and mounted on microscope slides with cover slips and stored in special cases. Daily records of airborne fungal spores were obtained by counting all spores in three longitudinal traverses per microscope slide at x 400. After the spore counting in each sampling area, a specific — for the microscope used — correction factor was applied. Thus, the final counts of fungal spores were expressed as daily numbers of spores per cubic metre of air. The taxa of Cladosporium spp. and Alternaria spp. were selected, as these comprise the most abundant in the air of Thessaloniki (Gioulekas et al. Citation2004). Also, the fungal spores of Alternaria (O'Hollaren et al. Citation1991; Kauffman et al. Citation1995; Targonski et al. Citation1995; D'Amato et al. Citation1997; Halonen et al. Citation1997; Corsico et al. Citation1998; Resano et al. Citation1998; Downs et al. Citation2001; Verini et al. Citation2001; Mari et al. Citation2003; Bush & Prochnau, Citation2004) and Cladosporium (Hasnain et al. Citation1994; D'Amato et al. Citation1997; Resano et al. Citation1998) are considered as the most commonly implicated to respiratory allergy symptoms worldwide, including the area of Thessaloniki (Gioulekas et al. Citation2004).
Meteorological data
Meteorological data were also used in daily and monthly summed values, conducted over the same period (1996–2002, Université de Thessaloniki 1997–Citation2003). The meteorological station was located in the centre of the city, less than one kilometre from the Burkard trap. These variables were: mean, maximum and minimum air temperature (in °C), relative humidity (%), solar radiation (mWh/cm2), atmospheric pressure (mm Hg), and presence or absence of dew (0, 1). All the above meteorological parameters are considered as the most important for fungal spore shedding, dispersion, and transport (Solomon, Citation1978; Hjelmroos, Citation1993; Katial et al. Citation1997; Kurkela et al. Citation1997; Angulo‐Romero et al. Citation1999; Corden & Millington, Citation2001; Mitakakis et al. Citation2001; Munuera Giner et al. Citation2001; Troutt & Levetin, Citation2001; Stennett & Beggs, Citation2004). Taking into consideration the special contribution of the parameters of rain and wind to spore circulation (Solomon, Citation1978; Hjelmroos, Citation1993; Katial et al. Citation1997; Kurkela, Citation1997; Mitakakis et al. Citation2001; Troutt & Levetin, Citation2001), we additionally inserted variables referring to their interaction effect. Particularly, we processed the individual parameters of rainfall height and rainfall duration, and the mean daily rainfall effect (expressed as mm of rainfall per day). Moreover, we used the interaction effects of wind direction (in octants: north, north‐east, east, south‐east, south, south‐west, west, north‐west), speed (in km/h), and persistence (in hours). Thus, we used the product of the wind speed and the sum of hours a wind is blowing from a specific direction in each calendar day; that is, eight wind variables were created, each corresponding to the interaction effect of wind speed‐persistence for each different wind direction.
Results
Significant variations in fungal spore atmospheric levels over time were found for both taxa. Alternaria and Cladosporium presented increased airborne spore counts in 1999 and 1997 respectively (Fig. ).
Figure 1 Time series of the daily fungal spore counts(per m3 of air) of the two studied taxa in the area of Thessaloniki during 1996–2002: (A) Alternaria, (B) Cladosporium.
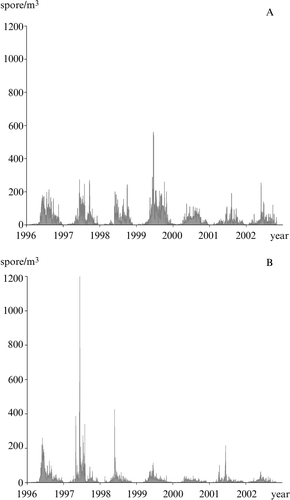
Monthly forecasting models
The time series of Alternaria spore counts was highly seasonal and slightly trended, whereas that of Cladosporium was seasonal but stationary. Alternaria seems to have a direct relationship with minimum air temperature, compared with Cladosporium which was affected by solar radiation (Table ). In the first case, past fungal spore counts displayed lag‐effects of one and 12 months, whereas in the second case, these lags were 12 and 24 months. The forecasting criteria (Table ) and the fitting of the created models (Fig. ) were both quite good. A great proportion of the variance was explained by each model (adjusted R2 = 0.68 for Alternaria and 0.81 for Cladosporium), and the residual analysis proved the adequacy of the models (Fig. ), since no statistically significant differences were found between the fitted and the actual fungal spore counts. Similarly, accuracy of all created models was verified by the no‐significant Ljung‐Box probability (higher than 0.99) for serial autocorrelation errors in the regressions. Forecasts of 12 months ahead further proved the simultaneous out‐of‐sample predictive power of the constructed models, as these gave values of adjusted R2 = 0.74 for Alternaria and 0.83 for Cladosporium, along with low values of MAPE for both of them. Considering interrelationships with meteorological variables, when we forecasted a year ahead – in contrast to the in‐sample data modelling – fungal spore counts of Alternaria correlated significantly with the interaction effect of rainfall (and not with air temperature). Similarly, those of Cladosporium were negatively related – apart from solar radiation – to the interaction effect of south‐west winds.
Figure 2 Actual and fitted summed monthly fungal spore counts(per m3 of air) of the two studied taxa in the area of Thessaloniki over 1996–2002. Continuous darker line indicates the fitting period, whereas the dashed line indicates the actual fungal spore counts: (A) Alternaria, (B) Cladosporium.
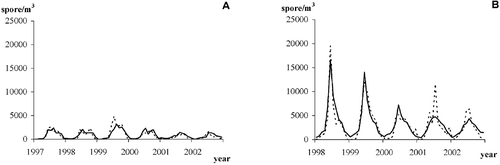
Figure 3 Residual analysis plots of fitted spore counts(Y‐axis) over time (X‐axis). A, B. Residuals for monthly forecasting models. C, D. residuals for daily forecasting models (A & C. Alternaria; B & D. Cladosporium).
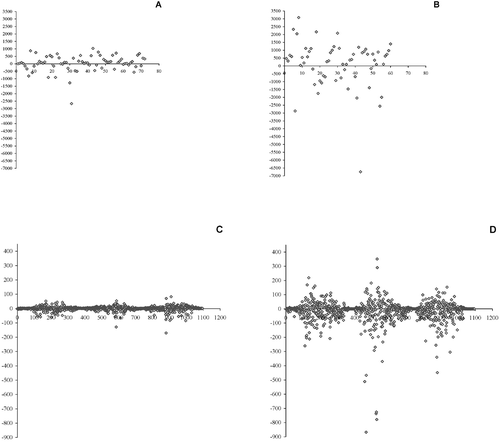
Table I. Dynamic Regression models referring to the summed monthly spore counts of the two fungal taxa (dependent variables), the monthly average values of 12 meteorological factors (independent variables), and the Cochrane‐Orcutt autoregressive term over the period 1996–2002.
Daily forecasting models
The time series of fungal spore counts for both taxa were seasonal and trended, yet presenting a simultaneous high irregular pattern (especially in the case of Cladosporium). Alternaria had a direct relationship with mean air temperature, compared with Cladosporium which was affected again by solar radiation (Table ). In the first case, past fungal spore counts displayed significant lag‐effects mainly of one and four days, in addition to one week and two years. In the case of Cladosporium, these lags were mainly of one and two years, and two weeks. The forecasting criteria (Table ) were quite low, and both of the created models were quite good (Fig. ), even though the model for Cladosporium could be further improved (Ljung‐Box probability higher than 0.99; Table ). A great proportion of the variance was explained by each model (adjusted R2 = 0.62 for Alternaria and 0.70 for Cladosporium). Nevertheless, the residual analyses (Fig. ) and the identification of autocorrelation function revealed significant differences between the fitted and the actual fungal spore counts. It seems that there is a strong serial interdependence among autocorrelation errors, and although many Cochrane‐Orcutt autoregressive errors were included in each daily predictive model (Table ), there is still a slowly decaying (proportionate to the increase of lags) serial interdependence of these errors. Forecasts of seven days ahead were checked against the actual spore records, and revealed that even though the models gave values of adjusted R2 = 0.62 for Alternaria and 0.70 for Cladosporium, they constantly overestimated the fungal spore levels for both taxa.
Figure 4 Actual and fitted daily fungal spore counts(per m3 of air) of the two studied taxa in the area of Thessaloniki over 1996–2002. Continuous darker line indicates the fitting period, whereas the dashed line indicates the actual fungal spore counts: (A) Alternaria, (B) Cladosporium.
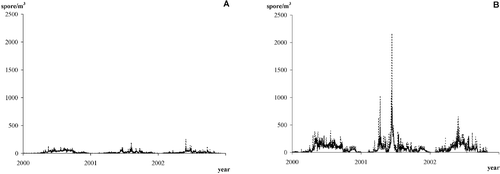
Table II. Dynamic Regression models referring to the daily spore counts of the two studied fungal taxa, the daily values of 12 meteorological factors, and the Cochrane‐Orcutt autoregressive term over the period 1996–2002.
Discussion
Airborne spores of the fungi Alternaria and Cladosporium have been documented as highly allergenic agents in Thessaloniki, Greece (Gioulekas et al. Citation2004), similarly to other locations worldwide (Hasnain et al. Citation1994; Targonski et al. Citation1995; D'Amato et al. Citation1997; Halonen et al. Citation1997; Corsico et al. Citation1998; Resano et al. Citation1998; Verini et al. Citation2001; Mari et al. Citation2003). Many researchers relate the occurrence of respiratory allergy symptoms with the presence of these spores in the ambient air (Kauffman et al. Citation1995; Downs et al. Citation2001). In fact it has been frequently reported that severe asthma episodes, even acute respiratory failure incidents, have been provoked after prolonged exposure to the above fungal spores types (O'Hollaren et al. Citation1991; Kauffman et al. Citation1995; Dales et al. Citation2000; Bush & Prochnau, Citation2004), particularly in sensitised children and young adults (O'Hollaren et al. Citation1991; Targonski et al. Citation1995; Dales et al. Citation2000; Downs et al. Citation2001; Verini et al. Citation2001; Bush & Prochnau, Citation2004).
Therefore, there is an increasing interest in the development of statistical models, of high predictive power, for atmospheric levels of allergenic fungal spores that will allow allergic individuals to take preventative action. Nevertheless, the existing predictive models are sometimes poor forecasters (Hjelmroos, Citation1993; Angulo‐Romero et al. Citation1999; Mitakakis et al. Citation2001; Troutt & Levetin, Citation2001; Stennett & Beggs, Citation2004).
For the above reasons, it was the first time that airborne fungal spore levels were forecasted in Greece. The statistical method used (autoregressive models) is considered as an advanced statistical technique (Chatfield, Citation1989); similar methods have very rarely been processed for this type of time series (Katial et al. Citation1997). We evaluated fungal spore counts as continuous observations, which, hence, are usually serially correlated between them. We, moreover, considered the covariances of 18 of the most significant meteorological factors (Solomon, Citation1978; Hjelmroos, Citation1993; Katial et al. Citation1997; Kurkela et al. Citation1997; Angulo‐Romero et al. Citation1999; Corden & Millington, Citation2001; Mitakakis et al. Citation2001; Munuera Giner et al. Citation2001; Troutt & Levetin, Citation2001; Stennett & Beggs, Citation2004). The constructed predictive models displayed high forecasting ability, both in a short‐ (daily) and a long‐term (monthly) perspective. This is believed to be due to the assessment of the direct relationships between variables, and also the indirect (time‐delayed) effect (Katial et al. Citation1997; Mitakakis et al. Citation2001; Munuera Giner et al. Citation2001; Stennett & Beggs, Citation2004), along with the co‐estimation of seasonality and cyclic/periodic components (Chatfield, Citation1989; Business Forecast Systems, 1992–Citation1995).
Our results, referring to the relationship of airborne fungal spore levels and prevailing meteorological factors, are similar to those obtained by other researchers. It seems that circulation of Alternaria spores is mainly influenced by air temperature, whereas Cladosporium is influenced by solar radiation (Solomon, Citation1978; Hjelmroos, Citation1993; Angulo‐Romero et al. Citation1999; Corden & Millington, Citation2001; Munuera Giner et al. Citation2001; Troutt & Levetin, Citation2001; Stennett & Beggs, Citation2004). However, differences have been reported very frequently from this modelling pattern. A large proportion of the above variability is due to the proximity and abundance of the source of fungal spore and the geobotanical characteristics of each studied region. Many authors indicate that airborne fungal spore levels are dependent on crop production and proximity of grassland areas (Solomon, Citation1978; Mitakakis et al. Citation2001; Corden et al. Citation2003; Pepeljnjak & Segvic, Citation2003).
Finally, the interrelationship of fungal spore records and meteorological factors is also connected to the forecast horizon of the model. Some predictive models may be quite accurate when assessing values from inside the time series (in‐sample/fitting accuracy), yet when these estimations extend to a certain horizon (out‐of‐sample/forecasting accuracy), variable results might be obtained (Business Forecast Systems, 1992–Citation1995). In the case of Thessaloniki, long‐term (12‐month) predictions differed from the in‐sample monthly models, in terms of the meteorological factors included in each model. Alternaria was related positively to the interaction effect of rainfall and Cladosporium negatively to the interaction effect of south‐west winds. In the first occasion, we believe this differentiation is due to the annual cyclic phenomena of Alternaria spores' liberation and dispersion, whereas in Cladosporium the cause is the location of spore sources (extensive crop production areas are found to the south‐west and grasses as lower vegetation at a neighbouring forest to the north‐east). Similar incidents have been documented by other researchers as well (Kurkela, Citation1997; Corden & Millington, Citation2001; Mitakakis et al. Citation2001; Munuera Giner et al. Citation2001, Troutt & Levetin, Citation2001; Corden et al. Citation2003; Pepeljnjak & Segvic, Citation2003).
The obtained forecasting models, in general, are believed to perform well, however significant variations have been found in a short‐term basis (daily modelling). Residual analysis proved significant serial interdependence among autoregressive errors for different lags (Fig. ). Although the Cochrane‐Orcutt autoregressive error term was included in both daily models for various lags (Table ), the above serial interdependence was still observed. It seems as if the daily models could not respond well to the irregularities observed in the daily pattern of each time series (Fig. ), which are caused by the intermittent meteorological variations and the high seasonality of daily time series (Fig. ). The use of hourly data (Hjelmroos, Citation1993; Kurkela, Citation1997; Troutt & Levetin, Citation2001) – both for meteorological and fungal spore variables – would help us understand their exact relationship thoroughly and improve the assessment of interdiurnal variability, which was not taken into account in these forecasts.
Conclusions
The autoregressive predictive models used proved to be of high forecasting ability for the circulation of fungal spores in Thessaloniki, Greece, explaining the greatest of the variance of the time series and with relatively low forecast errors. Alternaria spores were strongly related to air temperature, as Cladosporium was to solar radiation; both taxa were mainly correlated with past records with a time delay of one to two years and up to the two most recent weeks. The interaction effects of rainfall and wind components were also considered necessary for long‐term (annual) predictions of airborne fungal spore concentrations. It is believed that our forecasting models could be further improved, if we enhance our knowledge base on fungal spore production, emission, dispersion, and transport – by means of detection of local and regional sources of fungal spores – and focus more profoundly on the interdiurnal and seasonal variations in spore‐meteorological variable time series.
Acknowledgements
The authors wish to thank Prof. C. Balafoutis (Department of Meteorology‐Climatology, School of Geology, Aristotle University of Thessaloniki, Greece), for kindly providing us with the meteorological data.
Special acknowledgement is also given to Prof. D. Vokou and Dr. J. M. Halley (Department of Ecology, School of Biology, Aristotle University of Thessaloniki, Greece), as well as to Assoc. Prof. K. Stergiou (Department of Zoology, School of Biology, Aristotle University of Thessaloniki, Greece), for the scientific support and the useful comments on our work.
References
- Angulo‐Romero , J. , Mediavilla‐Molina , A. and Domínguez‐Vilches , E. 1999 . Conidia of Alternaria in the atmosphere of the city of Cordoba, Spain in relation to meteorological parameters. . Int. J. Biometeor. , 43 : 45 – 49 .
- British Aerobiology Federation . 1995 . Airborne pollens and spores. A guide to trapping and counting, , 1 , Rotherham, , UK : Natl Pollen & Hayfever Bureau .
- Bush , R. K. and Prochnau , J. J. 2004 . Alternaria‐induced asthma. . J. Allergy & Clin. Immunol. , 113 : 227 – 234 .
- Business Forecast Systems . 1992–1995 . Forecast Pro for Windows: User's manual guide. Extend. Ed. Vers. 2.10 , Belmont, MA : Business Forecast Systems, Inc .
- Chatfield , C. 1989 . The analysis of time series. An introduction (4th ed) , London/Glasgow/New York/Tokyo/Melbourne/Madras : Chapman & Hall .
- Corden , J. M. and Millington , J. J. 2001 . The long‐term trends and seasonal variation of the aeroallergen Alternaria in Derby, UK. . Aerobiologia , 17 : 127 – 136 .
- Corden , J. M. , Millington , W. M. and Mullins , J. 2003 . Long term trends and regional variation in the aeroallergen Alternaria in Cardiff and Derby UK‐ are differences in climate and cereal production having an effect? . Aerobiologia , 19 : 191 – 199 .
- Corsico , R. , Cinti , B. , Feliziani , V. , Gallesio , M. T. , Liccardi , G. Loreti , A. 1998 . Prevalence of sensitization to Alternaria in allergic patients in Italy. . Ann. Allergy Asthma Immunol. , 80 : 71 – 76 .
- D'Amato , G. , Chatzigeorgiou , G. , Corsico , R. , Gioulekas , D. , Jäger , L. and Jäger , S. 1997 . Evaluation of the prevalence of skin prick test positivity to Alternaria and Cladosporium in patients with suspected respiratory allergy ‐ A European multicenter study promoted by the Subcommittee on Aerobiology and Environmental Aspects of Inhalant Allergens of the European Academy of Allergology and Clinical Immunology. . Allergy , 52 : 711 – 716 .
- Dales , R. E. , Cakmak , S. , Burnett , R. T. , Judek , S. , Coates , F. and Brook , J. R. 2000 . Influence of ambient fungal spores on emergency visits for asthma to a regional children's hospital. . Am. J. Respir. Critic. Care Med. , 162 : 2087 – 2090 .
- Downs , S. H. , Mitakakis , T. Z. , Marks , G. B. , Car , N. G. , Belousova , E. G. and Leuppi , J. D. 2001 . Clinical importance of Alternaria exposure in children. . Am. J. Respir. Critic. Care Med. , 164 : 455 – 459 .
- Gioulekas , D. , Damialis , A. , Papakosta , D. , Spieksma , F. T. M. , Giouleka , P. and Patakas , D. 2004 . Allergenic fungi spore records (15 years) and sensitization in patients with respiratory allergy in Thessaloniki‐Greece. . J. Investig. Allergol. Clin. Immunol. , 14 : 225 – 231 .
- Halonen , M. , Stern , D. A. , Wright , A. L. , Taussig , L. M. and Martinez , F. D. 1997 . Alternaria as a major allergen for asthma in children raised in a desert environment. . Am. J. Respir. Critic. Care Med. , 155 : 1356 – 1361 .
- Hasnain , S. M. , Al‐Frayh , A. S. , Harfi , H. A. , Gad‐el‐Rab , M. O. , Al‐Moberik , K. and Al‐Sedairy , S. T. 1994 . Cladosporium as an airborne allergen in Saudi Arabia. . Ann. Saudi Med. , 14 : 142 – 146 .
- Hjelmroos , M. 1993 . Relationship between airborne fungal spore presence and weather variables. Cladosporium and Alternaria . Grana , 32 : 40 – 47 .
- Katial , R. K. , Zhang , Y. M. , Jones , R. H. and Dyer , P. D. 1997 . Atmospheric mold spore counts in relation to meteorological parameters. . Int. J. Biometeorol. , 41 : 17 – 22 .
- Kauffman , H. F. , Tomee , J. F. C. , Van Der Werf , T. S. , De Monchy , J. G. R. and Koeter , G. K. 1995 . Review of fungus‐induced asthmatic reactions. . Am. J. Respir. Critic. Care Med. , 151 : 2109 – 2116 .
- Kurkela , T. 1997 . The number of Cladosporium conidia in the air in different weather conditions. . Grana , 36 : 54 – 61 .
- Mari , A. , Schneider , P. , Wally , V. , Breitenbach , M. and Simon‐Nobbe , B. 2003 . Sensitization to fungi: epidemiology, comparative skin tests, and ige reactivity of fungal extracts. . Clin. Exp. Allergy , 33 : 1429 – 1438 .
- Mitakakis , T. Z. , Clift , A. and McGee , P. A. 2001 . The effect of local cropping activities and weather on the airborne concentration of allergenic Alternaria spores in rural Australia. . Grana , 40 : 230 – 239 .
- Munuera Giner , M. , Carrion Garcia , J. S. and Navarro Camacho , C. 2001 . Airborne Alternaria spores in SE Spain (1993–98). Occurrence patterns relationship with weather variables and prediction models. . Grana , 40 : 111 – 118 .
- O'Hollaren , M. , Yunginger , J. W. , Offord , D. J. , Somers , M. J. , O'Connell , E. J. and Ballard , D. J. 1991 . Exposure to aeroallergens as a possible precipitating factor in respiratory arrest in young patients with asthma. . N. Engl. J. Med. , 324 : 359 – 363 .
- Pepeljnjak , S. and Segvic , M. 2003 . Occurrence of fungi in air and on plants in vegetation of different climatic regions in Croatia. . Aerobiologia , 19 : 11 – 19 .
- Resano , A. , Sanz , M. L. and Oehling , A. 1998 . Sensitization to Alternaria and Cladosporium in asthmatic patients and its in vitro diagnostic confirmation. . J. Investig. Allergol. Clin. Immunol. , 8 : 353 – 358 .
- Solomon , W. R. 1978 . “ Aerobiology and inhalant allergens. I. Pollen and fungi. ” . In Allergy principles and practice , Edited by: Middleton , E , Reed , C. E and Ellis , E. F . Vol. 2 , Saint Louis, MO : CV Mosby Co .
- Stennett , P. J. and Beggs , P. J. 2004 . Alternaria spores in the atmosphere of Sydney, Australia, and relationships with meteorological factors. . Int. J. Biometeor. , 49 : 98 – 105 .
- Stergiou , K. I. , Christou , E. D. and Petrakis , G. 1997 . Modelling and forecasting monthly fishering catches: comparison of regression, univariate and multivariate time series methods. . Fish. Res. , 29 : 55 – 95 .
- Targonski , P. V. , Persky , V. W. and Ramakrishnan , V. 1995 . Effect of environmental moulds on risk of death from asthma during the pollen season. . J. Allergy & Clin. Immunol. , 95 : 955 – 961 .
- Troutt , C. and Levetin , E. 2001 . Correlation of spring spore concentrations and meteorological conditions in Tulsa, Oklahoma. . Int. J. Biometeor. , 45 : 64 – 74 .
- Université de Thessaloniki . 1997–2003 . Observations météorologiques de Thessaloniki 1996–2002 , Thessaloniki : Thessaloniki. Univ . Univ, Ann. Inst. Météor. Climatol., 65–71
- Verini , M. , Rossi , N. , Verrotti , A. , Pelaccia , G. , Nicodemo , A. and Chiarelli , F. 2001 . Sensitization to environmental antigens in asthmatic children from a central Italian area. . Sci. Total Environ. , 270 : 63 – 69 .
- Zar , J. H. 1999 . Biostatistical analysis, , 4 , Upper Saddle River, NJ : Prentice Hall .