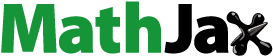
ABSTRACT
A large number of diversity measures have been proposed in the literature, almost all of which are designed for sets with elements that differ in multiple aspects. However, these types of measures are not appropriate for sets with elements that differ in a single aspect only, such as duration, value or probability. In this essay I present a new measure of diversity, designed specifically for single aspect diversity. The measure captures the intuitive idea that single aspect diversity is affected by the maximal dissimilarity between the elements, the number of different elements, and the even spacing of the elements’ aspect values, when represented as points in one dimension. I show that the measure satisfies six plausible conditions for a single aspect diversity measure, which concern scale independence, minimal diversity, maximal dissimilarity, restricted function growth, strict monotonicity, and even spacing. The measure is also characterised.
Diversity is an important concept in many scientific areas, such as statistics, biology and economics. Considering its importance, it is hardly surprising that there are a large number of diversity measures in use. It is surprising, however, that none of these measures seem to work for the diversity of sets with elements which differ in just one quantitative aspect. While extant measures work for the diversity of people with respect to age and income, for example, they do not work for the diversity of people with respect to age or income only. This is a disadvantage.
In this essay I will present a new real valued measure of diversity, designed for sets with elements that differ in a single quantitative aspect. The measure can, for example, be used for the diversity of populations with respect to well-being, or for the diversity of choice sets with respect to price. Since single aspect differences can be represented by one-dimensional distances, this type of diversity can be called ‘one-dimensional diversity’.
The diversity of a set plausibly depends on the dissimilarities between its elements. The more dissimilar the elements are overall, the more diverse the set. For one-dimensional diversity, this intuitively involves a larger maximal dissimilarity and a larger number of dissimilar elements. It also intuitively involves aspect values being closer to evenly spaced. However, no previously proposed measure captures all of these intuitions. If we simply aggregate all dissimilarities, as some have proposed, we get the result that the set of aspect values (20, 21, 60) is as diverse as the set (20, 100), which intuitively underestimates the importance of a larger maximal dissimilarity. But if we instead consider only average dissimilarity, as others have proposed, we get the result that the set (20, 100) is more diverse than the set (20, 21, 100), which intuitively underestimates the importance of additional elements. Also, both these measures have the result that the set (20, 21, 100) is as diverse as the set (20, 60, 100), which ignores the intuition that an even spacing of values matters as well.
All these intuitions can be captured, however, if we measure single aspect diversity by aggregating the absolute differences between adjacent aspect values by a strictly concave root function. This is the diversity measure that I shall propose here.
The measure rewards a larger maximal dissimilarity, more elements, and an even spacing of single aspect values. More precisely, it satisfies the following plausible conditions for a single aspect diversity measure:
A ratio scale independence condition, stating that ratio relations between degrees of diversity should be preserved when the underlying dissimilarity measure is multiplied by a positive number.
A minimal diversity condition, stating that the empty set and singleton sets should be classified as offering no diversity.
A diameter condition, stating that a set with a larger maximal dissimilarity should be ranked above any set with a smaller maximal dissimilarity, in comparisons between sets that have the same number of elements and whose elements’ single aspect values are evenly spaced.
A restricted growth condition stating that the addition of an element y to a set A should not increase diversity by more than the diversity assigned to the set (x, z), where d(x, z) is the largest dissimilarity between the elements of A ∪ (y).
A strict monotonicity condition, stating that the addition of an element that is dissimilar to all the other elements of a set, should result in more diversity.
An even spacing condition, stating that a set of elements with single aspect values that are evenly spaced should be ranked above any set of elements with single aspect values that are not evenly spaced, in comparisons between sets with the same number of elements and the same maximal dissimilarity.
The essay will be structured as follows: in Section 1 I motivate the need for a measure of one-dimensional diversity; in Section 2 I discuss the concept of diversity and how the conception used in this essay differs from other conceptions; in Section 3 I make some necessary assumptions; in Section 4 I propose conditions that a measure of diversity should satisfy; in Section 5 I present some previously proposed measures and show how none of them satisfies all of the proposed conditions; in Section 6 I present my own measure, which satisfies the conditions, in Section 7 I explain how the measure is unique, and in Section 8 I make some concluding remarks. An appendix with a uniqueness proof follows.
1. Motivation
Diversity is discussed in a wide variety of scientific areas, including applied mathematics, statistics, information science, computer science, linguistics, chemistry, biology, earth science, geography, anthropology, psychology, sociology, and economics. In philosophy, diversity is discussed in (for example) epistemology, philosophy of science, ethics, political philosophy, and decision theory.Footnote1 These discussions have in part concerned the concept of diversity and its measurement, and as a result, a large number of diversity measures have been proposed. One may thus wonder why we need another one. The straightforward answer is that there are no measures for one-dimensional diversity, based on the assumption that the diversity of a set is a function of single aspect ratio scale dissimilarities between its elements, and which also accommodate the intuitions that the largest dissimilarity, the number of dissimilar elements, and the even spacing of the elements’ aspect values determine the diversity of a set. In fact, there are no one-dimensional diversity measures at all, designed specifically for single aspect ratio scale dissimilarities – at least not if we distinguish between diversity and dispersion, which I will argue below that we should. (Ratio scale dissimilarities are dissimilarities measurable on a ratio scale, allowing representation of ratio relations between dissimilarities, as well as representation of equality, order and interval relations. Ratio scales are characterised by having non-arbitrary zero points and arbitrary units.)
The lack of one-dimensional diversity measures would not be a problem if there were no use for one-dimensional measures in any of these areas. But this is hardly the case. Single aspect properties are of interest in all sciences, and whenever the objects of some set differ in only one aspect, it could be useful to measure the diversity of the set with regard to that aspect. Among one-dimensional properties of scientific interest we have: data size, probability, confirmation, time, length, speed, mass, luminosity, pitch, pressure, temperature, humidity, acidity, sweetness, enjoyment, willingness, credence, price, inflation, income, equality, age, and birth rate. Additional properties of philosophical interest include properties such as cogency, goodness, and well-being. A one-dimensional diversity measure could also be used to compare sets of elements which include different numbers of some kind of element, such as the diversity of a set of countries which include different numbers of people.
The lack of one-dimensional diversity measures, dependent on ratio scale dissimilarities, would also not be a problem if measures designed for other purposes could be used instead. However, there are at least two reasons why they cannot. First, most diversity measures cannot be adapted to register ratio scale dissimilarities at all, whether one-dimensional or multi-dimensional. Second, even measures that are designed for multi-dimensional ratio scale dissimilarities are not suitable for one-dimensional ratio scale dissimilarities, since there is a specific feature desirable for multi-dimensional measures which is not desirable for one-dimensional measures. Let us look at these two problems in turn.
The first problem with finding a measure suitable for one-dimensional diversity is that most measures are designed for models that do not register dissimilarity relations at all. There are many measures that only register number of elements, attributes, or classes, for example, and these cannot be used to measure diversity regarded as a function of single aspect dissimilarities.Footnote2 A count of elements does not register the dissimilarities between the elements at all (other than them being distinct elements); a count of attributes does not register that elements can differ within a single aspect; and a count of classes does not register that elements can differ within a single class. These types of measures are thus disqualified from the start. Trying to redesign these types of measures for single aspect dissimilarities is not going to work either, because even if we could treat specific values of some single aspect as elements, attributes, or classes, we could not capture the dissimilarity relations between them in this way.
The second problem is that even measures that are designed for diversity regarded as a function of dissimilarity relations are not suitable for one-dimensional ratio scale dissimilarity relations. Some measures will not work well because they are designed only for ordinal relations (relevant information is thus lost if they are applied).Footnote3 Other measures will not work well because they are designed for multiple aspect dissimilarities.Footnote4 This last point is perhaps surprising because one might think that multiple aspect measures should be capable of handling single aspect diversity as well. There is a reason, however, to think that they are not. Relative to multiple aspect diversity, it is reasonable to think that elements that are equally dissimilar to one another are more diverse than elements that are not – everything else being equal (number of elements and largest dissimilarity).Footnote5 For example: if the elements are represented as points in Euclidean space and their dissimilarities are represented as distances, a set appears more diverse if the elements are represented as positioned in the corners of a simplex (everything else being equal). This way the elements are as dissimilar to one another as possible, relative to some fixed number of elements and a fixed largest dissimilarity.Footnote6 As an extension of this ideal, it is also reasonable to think that sets with elements that are closer to being equally dissimilar to one another are more diverse than sets with elements that are further away from being equally dissimilar, everything else being equal. This equidistance ideal, however, is hardly reasonable for single aspect diversity, not just because only two elements could be equally dissimilar to all other elements with respect to a single aspect, but also because the ideal conflicts with the intuition that objects are more diverse when their aspect values are closer to being evenly spaced in one-dimensional space, everything else being equal. This conflict might seem surprising, but an example can illustrate the point: Let us assume that closeness to equidistance can be measured by a sum of differences between the dissimilarities between the elements of a set, where a smaller sum represents larger closeness and 0 represent equidistance. Let us next compare the sets of aspect values: A = (0, 53, 106, 159, 212, 265) and B = (0, 70, 105, 140, 210, 280) with the same total sum of dissimilarities. Here, the values in A are distributed at even intervals on a real line, but the values in B are closer to being equidistant, relative to the dissimilarities between all pairs of values. (The sum of differences between the distances between the values in B is smaller than the sum of differences between the distances between the values in A.) A single aspect diversity measure should rank A over B in diversity, but a multiple aspect diversity measure would rather rank B over A, in keeping with the ideal of equidistance. For this reason, a multiple aspect diversity measure will not be adequate for single aspect diversity. A specific one-dimensional diversity measure based on ratio scale dissimilarities is thus needed.
2. Diversity
The term ‘diversity’ does not express a single, precise concept; rather, it is used to express a variety of different diversity conceptions. Among these, there is a specific conception of one-dimensional diversity which will be the focus of this paper. Let us see how this conception differs from some of its alternatives.
In general, diversity is a quantitative property of sets (which could be populations or assortments or something else). While the elements of a set can be more or less dissimilar to one another, only sets can be more or less diverse. However, a set, A, is more diverse than another set, B, if and only if the elements of A are more dissimilar to one another than what the elements of B are. Diversity is thus the same as collective dissimilarity.Footnote7
According to the single aspect diversity conception which will be the focus here, the diversity of a set is dependent on dissimilarity relations between elements and is affected in particular by the largest dissimilarity, the number of dissimilar elements, and the even spacing of the elements’ aspect values, when represented as points in one-dimensional space. Since this is not the only conception of diversity in use, let us contrast it with others that occur in the literature. (Conceptions will be individuated rather coarsely here because if we were to regard each measure as the expression of a distinct conception, the presentation would be much too long.)
All conceptions of diversity seem somehow to involve the idea that the diversity of a set is dependent on dissimilarities. However, the relevant dissimilarities are not always considered to be dissimilarities between the elements of the set, and the dependence on dissimilarities is not always considered to be a direct one. There seems to be at least three views where dissimilarities only play an indirect role. Let us consider these views first.
One indirect view is that the diversity of a set is the same thing as the number of elements of the set.Footnote8 Since the individual elements of a set are separated by being dissimilar, dissimilarities play an indirect role at least for individuating elements. To identify diversity with number of elements is a rather blunt view, however. It does not capture that the diversity of a set could be affected by the elements being dissimilar in different aspects. Neither does it capture that the diversity of a set could be affected by degrees of dissimilarities between its elements.
Another indirect view is that the diversity of a set is the same thing as the number of attributes that the elements of the set exemplify.Footnote9 Since attributes are separated by being dissimilar, dissimilarities play an indirect role also in this case, although for individuating attributes. This view is rather blunt as well, since it does not capture that a set of elements that differ in just one aspect could be diverse. Thus, this view is at most appropriate for multiple aspect diversity.
A third indirect view is that the diversity of a set is dependent (somehow) on the classes that the elements of the set could be sorted into – either the number of classes (richness), the number of individuals per class (abundance), the dissimilarities between classes, how evenly the elements can be sorted into classes (with maximal evenness being that each class has an equal number of members), or some combination of these four features.Footnote10 Since classes are separated by being dissimilar, dissimilarities play an indirect role even for these cases. However, these views cannot capture all aspects of diversity either, since they cannot capture that a set of elements belonging to just one class can be diverse.
Other views on diversity consider the diversity of a set to be more directly dependent on dissimilarities. Let us consider such views next.
One direct view is that the diversity of a set is a function of dissimilarities, but not just a function of the dissimilarities between the elements of the set, but a function of the dissimilarities between the elements of the set and elements outside the set: either all the elements of the universal set or some element representing the mean of the universal set (which may or may not belong to the set).Footnote11 This conception is not very plausible, however, since it represents diversity as an extrinsic property of sets, rather than as an intrinsic one. An implausible implication of this view is that it is not possible to rank sets in terms of diversity, without knowledge of the elements of the universal set. Another implausible implication is that a set of two elements which are very similar could be ranked as more diverse than a set of two elements which are very dissimilar, due to their different relations to the universal set.
A closely related view is that the diversity of a set is a function of the dissimilarities between the elements of a set and some element representing the mean of the set (which also may or may not belong to the set).Footnote12 This view equates diversity with dispersion, which can be measured by variance, standard deviation, or mean deviation.Footnote13 One problem with these types of measures is that the addition of a mean element to a set would not increase the diversity of the set, which it intuitively should.
Another direct view is that the diversity of a set is just the totality of the dissimilarities between the elements of a set.Footnote14 This view is not sensitive to the distribution of the totality of dissimilarities among individual dissimilarities, however. It is not sensitive to whether the dissimilarities are equal or whether the aspect values of the elements are evenly spaced.
Other direct views regard the diversity of a set as some aggregate of some selected dissimilarities between the elements of a set.Footnote15 Many different proposals have been made here, but none of them is sensitive to all three properties mentioned above (magnitude, number and even spacing). We will look at some of these proposals later.
The direct view that is most similar to the one that I will consider is that the diversity of a set is a function of the dissimilarities between the elements in such a way that it is affected by the magnitude of dissimilarities, the number of dissimilar elements and the equal size of the dissimilarities.Footnote16 However, as we have just seen, this view seems appropriate only for multiple aspect diversity as it cannot accommodate the view that even spacing between one-dimensional values matters for diversity.
3. Assumptions
Before we can consider proposals for measures of one-dimensional diversity, some assumptions regarding diversity, dissimilarity and single quantitative aspects need to be made. The purpose of a diversity measure is to assign numbers to sets so that quantitative relations between degrees of diversity are accurately represented by numerical relations. Since the diversity of a set is intrinsically dependent on the dissimilarities between the elements of a set, a diversity measure should be a function of a dissimilarity measure. And since dissimilarity, in turn, is intrinsically dependent on some aspect of the elements, a dissimilarity measure should, in turn, be a function of a measure of some quantitative aspect. Thus, both diversity and dissimilarity measures are derived measures of a measure of some quantitative aspect.Footnote17
Let us first assume that there is a finite universal set of objects, X. Next, let us assume that there is a measure q of some single aspect Q. The measure q assigns real numbers to all objects in a way that represents the degree to which they are Q and also represents the quantitative relations between degrees of Q by numerical relations. I will assume that the measure q is ratio scale, which means that the measure represents ratio relations between degrees of Q, as well as difference relations, ordinal relations and equality relations. A ratio scale measure of a quantitative property can more precisely be described as follows: First, let X be the set of relevant objects and let φ1, … φn be n-place relations that hold between the elements xi in X, and which compare them in degrees of Q; S = 〈X, φ1, … φn〉 is then a relational system. Next, let R be a set of real positive numbers and let ρ1, … ρn be n-place relations that hold between the numbers in R; T = 〈R, ρ1, … ρn〉 is then another relational system. The property Q is measureable by real positive numbers when the system S is represented by the system T through a function f: X → R such that for each sequence of elements xi ∈ X and each relation φi, it is the case that φi(x1 … xn) if and only if ρi(f(x1) … f(xn)).Footnote18 And Q is measureable on a ratio scale when S is also represented by T through any function g, such that g = αf, where α > 0.Footnote19 The function f and each function g are measures of Q.
Next, let us assume that the dissimilarity d between two objects, x and y, with respect to Q, can be measured by the absolute difference between q(x) and q(y), thus:
Dissimilarity as absolute difference: d(x, y) = |q(x) – q(y)|.Footnote20
Non-negativity: d(x, y) ≥ 0.
Identity of Indiscernibles: d(x, y) = 0 if and only if x = y.
Symmetry: d(x, y) = d(y, x).
Triangle Equality: d(x, y) = d(x, z) + d(z, y).
The Identity of Indiscernibles implies that objects that have exactly the same degree of Q are identical (this is a natural view to take since the objects are identical with respect to Q). It also implies that sets cannot be expanded by adding elements that are exactly alike elements that are already included in the set.
The one-dimensional Euclidean space 〈X, d〉 can be associated with a vector dX, where the distances are indexed with positive integers and then ordered. To construct this vector, the elements in X are first indexed from 1 to n, as x1, x2 … xn−1, xn in such a way that q(x1) ≥ q(x2) ≥ … q(xn−1) ≥ q(xn). Then the distances are indexed so that d1 = d(x1, x2), d2 = d(x2, x3) etc. For each subspace 〈A, d〉 of 〈X, d〉 there is a vector dASP which contains the distances d1 to dn−1, which are the shortest path distances connecting all the elements of A. This vector is particularly important since all distances between the elements of a set A can be derived from the shortest path distances, using the Triangle Equality. To give an example: for the set of lengths (10 m, 20 m, 25 m) the associated shortest path vector is [10 m, 5 m], which can be used to derive the vector of all distances [10 m, 5 m, 15 m].
The maximal dissimilarity (or distance) between two elements in a set A will be called the diameter of A, and will be denoted by Ø(A) (it is also known as the range of A). The number of elements in A will be denoted by n or #A.
With these assumptions and notations in place, we can continue to consider conditions for a measure of diversity.
4. Conditions for a one-dimensional diversity measure
There are an infinite number of ways in which a measure could be a function of dissimilarities. The idea that a diversity measure should be a function of a dissimilarity measure is thus insufficient for identifying a diversity measure and we must rely on further ideas about diversity. Here I will use the ideas that the diversity of a set is affected by the magnitude of the dissimilarities between the elements, the number of dissimilar elements, and the even spacing of the elements’ aspect values when represented as points on the real line. These three ideas will be used to formulate necessary conditions for a measure of diversity. First, however, I will consider a more general condition.
4.1 Ratio scale independence
The first consideration is important for any derived measure: a derived measure should be independent of scale. That is: ratio relations between different degrees of whatever quantity that is measured should be preserved even if an underlying ratio measure on which the derived measure depends changes scale. In the case of diversity, ratio relations between degrees of diversity should be preserved even if the underlying dissimilarity (and aspect) measure changes scale. It should not matter for the assessment of the diversity of a set of incomes whether incomes are measured in dollars or cents, for example. A diversity measure should thus satisfy the following condition:
The Ratio Scale Independence Condition: For a diversity measure D that is a function of a dissimilarity function d, it holds that for all numbers K > 0, there is a number L = L(K) > 0, where L is an increasing function of K such that if d is multiplied by K, then D is multiplied by L.Footnote22
4.2 Minimal diversity
A second condition concerns the minimal limit of diversity. Since diversity is a function of dissimilarity, a set whose members are not dissimilar could not be diverse. This is the case both for the empty set and for singleton sets: the empty set because it does not contain any elements that can be dissimilar at all and singleton sets because they contain only one element, which cannot be dissimilar to itself. A measure of diversity should thus satisfy the following condition:
The Minimal Diversity Condition: For a diversity measure D and any set A, if #A ≤ 1, then A is not diverse and thus D(A) = 0, or D(A) is undefined.Footnote24
4.3 Magnitude
The smallest sets that can be diverse are thus sets of two elements. Between these two elements, there is only one dissimilarity relation (assuming that d(x, y) and d(y, x) are regarded as the same relation). If diversity is a function of dissimilarities, the diversity of this kind of set should plausibly depend on the magnitude of this one dissimilarity relation. A higher degree of dissimilarity should imply a higher degree of diversity. Thus the following condition should hold:
The Limited Diameter Condition: For a diversity measure D and any sets A and B and any elements x, y, w, z, such that A = (x, y) and B = (w, z), if d(x, y) > d(w, z), then A is strictly more diverse than B and thus D(A) > D(B).Footnote25
For sets of two elements, diversity is a function of the magnitude of a dissimilarity relation. But, obviously, also for larger sets, diversity is a function of dissimilarity relations, and for them too, the magnitude of the dissimilarity relations should matter. Here, there is a difference between multiple aspect diversity and single aspect diversity, however.
For multiple aspect diversity, it seems important that each dissimilarity relation be as large as possible in order for the elements of a set to be as dissimilar to one another as possible. The reason for this is that for multiple aspect cases, represented in several dimensions, each dissimilarity relation can be of the same maximal degree. For example, for a set of three elements, three dissimilarity relations could have the same maximal degree. (If the elements were represented as points and the dissimilarity relations were represented as straight lines, such a set would be represented as a maximised triangle in two dimensions.) For single aspect cases, however, it cannot be important that each dissimilarity relation be as large as possible in order for the elements of a set to be as dissimilar to one another as possible. Firstly, all dissimilarity relations cannot be of the same maximal degree, if a set has cardinality larger than two. Secondly, even if it is favourable that one dissimilarity relation is maximised (the diameter), it is not favourable that the other relations are. This can be shown with some examples. The set A = (30, 70) seems more diverse than the set B = (30, 50) due to the larger diameter of A. However, the set C = (30, 69, 70) seems less diverse than the set D = (30, 50, 70), even though C has a larger second largest dissimilarity. An even spacing of values is intuitively more important here than the size of the second largest dissimilarity.
The diameter thus plays a special role for single aspect diversity since it is the only dissimilarity relation that should be maximised. We can use the diameter to formulate a condition for a single aspect diversity measure. Obviously, it will not do to formulate a condition that says that any set with a larger diameter is more diverse than any set with a smaller one. Such a condition would be reasonable only if the diameter would always be more important than either the number of elements or an even spacing of elements, and this does not seem to be the case. The set A = (25, 70) is intuitively less diverse than the set B = (25, 40, 55, 69), and the set C = (25, 26, 70) is intuitively less diverse than the set D = (25, 47, 69), for example. A larger diameter cannot compensate for fewer elements or an uneven spacing of values in these cases. The ideal of a larger diameter must thus be combined with the ideal of a larger number of elements and an even spacing of values. Nevertheless, if we keep numbers and spacing fixed, and only vary the diameter of sets, the set with the larger diameter should be more diverse. Thus, the following condition should hold:
The Diameter Condition: For a diversity measure D and any sets A and B, such that #A = #B and all elements of A and all elements of B have values that are evenly spaced in one dimension, if Ø(A) > Ø(B) then A is strictly more diverse than B and thus D(A) > D(B).Footnote26
4.4 Cardinality
I have proposed that diversity is dependent on the number of dissimilar elements. Not everyone agrees with this, however. There is a tradition of separating diversity from number, claiming that diversity is independent of number.Footnote27 This idea can be captured for example by measuring diversity by average dissimilarity. Intuitively, however, this seems wrong. The average dissimilarity is larger in the set (20, 100) than in the set (20, 50, 100). Yet, the second set seems more diverse since it has an additional value exemplified.
There is thus reason to think that diversity is affected by number. But the question is what number: number of elements or number of dissimilarities?
Since diversity is dependent on dissimilarities, it might seem that diversity should be affected by the number of dissimilarities. One proposal is that the diversity of a set is proportional to the number of dissimilarities. This view is problematic, however. When the number of elements is n, the number of dissimilarities is n2/2. Of these, all but n dissimilarities (those between elements and themselves) are non-zero dissimilarities that might contribute to the diversity of a set. For each added element, the number of elements grows with 1, but the number of non-zero dissimilarities grows linearly with the size of the set. If a set has 100 elements, the addition of a single element results in 100 additional dissimilarities. If all of these contributed proportionally to the diversity of a set, diversity would grow excessively with each added element.Footnote28 This excessive growth has several implausible implications. One implausible implication is that for large sets, the more numerous set is more likely to be more diverse – regardless of its diameter or its spacing of values. For example, the set A = (20, 21, 59, 60) might counterintuitively be considered more diverse than the set B = (20, 40, 60), although the elements of B seem more collectively dissimilar. Another implausible implication is that in the choice of adding an element to either a small set or a large set in order to maximise diversity, one should add the element to the larger set – even in those cases where there is already a very similar element in the larger set. For example, in the choice of adding an object with a value of 21 to either of the two sets C = (40, 60) and D = (20, 40, 60) in order to maximise diversity, the recommendation would be to add the object to the second set. Both these implications are implausible. A larger set is not generally more diverse. And the contribution of an element to a set should not depend on the size of the set in this way.
Due to the implausible implications of excessive growth, the diversity of a set cannot be proportional to the number of dissimilarities (or anything else that leads to excessive growth). It should at most be proportional to the number of elements. This restriction will prevent the implication that the contribution of an additional element to a set increases with the size of the set. We can capture this idea by formulating a condition that restricts the growth of a diversity measure. One way to do this is to propose that the addition of an element to a set should never add more to the diversity of the set than whatever value the diversity measure gives to the set that contains the two elements that are most dissimilar in the larger set – the diameter of the larger set, in other words. More formally this could be expressed as follows:
The Restricted Growth Condition: For a diversity measure D and any set A and any element y ∉ A, it holds that D((A ∪ (y))) ≤ D(A) + D((x, z)), where x, z ∈ A ∪ (y) and x and z are chosen so that the dissimilarity d(x, z) = Ø(A ∪ (y)).Footnote29
One proposal is that if diameter and even spacing are kept fixed, and only numbers are varied, the set with the larger number of elements is more diverse. However, such an idea would be controversial. According to this idea, the set A = (20, 40, 60, 80) is more diverse than the set B = (20, 50, 80). But this does not seem obvious. Perhaps the aspect value of 50 in B compensates for the absence of the aspect values of 40 and 60 in A in terms of diversity. After all: 50 is more dissimilar to both 20 and 80 than either of 40 and 60 is dissimilar to 20 and 80. Nevertheless, cardinality seems to matter at least if we compare sets with their own subsets. If we start with the set A = (20, 60) and then add a new aspect value to A, for example, y = 50, we would judge A ∪ (y) as more diverse than A because it contains an additional (and qualitatively different) element. Thus, satisfying the following condition should be required of any diversity measure:
The Strict Monotonicity Condition: For a diversity measure D and any elements x, y and a set A such that #A ≥ 1, it holds that: If an element y is dissimilar to all elements x ∈ A and y ∉A then A ∪ (y) is strictly more diverse than A and thus D(A ∪ (y)) > D(A).Footnote30
There are some potential objections to the Strict Monotonicity Condition, however. One possible objection is that A ∪ (y) would not be more diverse than A if the element y is very similar to an element in A. For example: if A = (20, 60) and y = 20.1, then A ∪ (y) might not be more diverse than A.
This reasoning seems to presuppose that it is types of elements that affect diversity, rather than individual elements. Two very similar elements would not affect diversity separately, since they belong to the same type. Such an idea is problematic, however. For example, let us define some types of Q so that each aspect value would belong to one and only one type. We could, for example, define the types of Q as half-open intervals of q, as follows: [0, 0.2], [0.2, 0.4], [0.4, 0.6] … etc. In this case we would get the result that y = 59.9 increases the diversity of A = (20, 60) whereas y = 20.1 does not, since the values 59.9 and 60 are of different types whereas the values 20 and 20.1 are not. This is not really a plausible solution since both y:s differ from an element of A by the same difference of 0.1. However, if we instead defined types so that all aspect values within (for example) 0.1 of each other always would count as belonging to the same type, we would get an infinite number of types and any set (apart from the empty set) would contain elements of an infinite number of types. The set A would, for example, exemplify elements of the types [19.9, 20.1], [19.99, 20.11], [19.999, 20.111] and so on. This is not a plausible solution either.
Perhaps there is a way to formulate this idea without implausible consequences, but there is really no need to. It is much easier to stick with the Strict Monotonicity Condition. If an element is added to A which is very similar to an element already in A, we should not say that diversity does not increase, but rather that it increases just slightly.
Another possible objection to the Strict Monotonicity Condition is the following: A ∪ (y) cannot be more diverse than A because A ∪ (y) must be less diverse than A ∪ (y). We can come to this conclusion by assuming, first that diversity is the opposite of unity and second, that unity is an increasing function of similarities (just as diversity is an increasing function of dissimilarities). If this is the case we can say that A ∪ (y) must be more unitary than A since all the similarities that hold between the elements in A also hold between the elements in A ∪ (y), but there are some similarities that hold between the elements in A ∪ (y) that do not hold between the elements in A. We can also say that since diversity and unity are opposites, A ∪ (y) must be less diverse than A.
This reasoning presupposes a certain relationship between unity and diversity which is problematic, however. If unity depends on similarities and diversity depends on dissimilarities, and if each element is both similar and dissimilar to every other element, then unity and diversity would both increase when a set increases. Thus, they cannot be opposites in the sense that if one increases the other decreases. So this objection will not work either.
4.5 Even spacing
The last property that I have proposed as affecting the diversity of a set is the even spacing of the elements’ aspect values in one dimension.Footnote31 When the elements of a set have aspect values that are evenly spaced, they seem to be more collectively dissimilar. The set A = (20, 40, 60) seems more diverse than the set B = (20, 21, 60), for example.
The importance of even spacing is unique for single aspect diversity. For multiple aspect diversity, it is rather equal dissimilarities that matter. This is because in multiple aspect cases all elements in a set could have the same dissimilarity to all other elements, which they cannot have in single aspect cases, if the set has more than two elements. (For n elements, there must be at least n – 1 dimensions in order for all the elements to have the same distance to all the other elements.)
For the property of even spacing, we might again propose an everything-else-being-equal type of condition, where spacing is varied and the other two factors are held fixed. Such a condition would claim that if two sets A and B have the same number of elements and the same diameter, then A is strictly more diverse than B, if the elements of A are closer to being evenly spaced than are the elements of B. The problem with such a condition would be that it is not clear exactly what ‘closer to being evenly spaced’ means.
A more precise understanding of ‘closer to being evenly spaced’ requires a measure of even spacing, and considering the many possibilities for such a measure, any particular choice would be controversial. So rather than provide an interpretation of ‘closer to being evenly spaced’, it is better to adopt a different condition. I propose that we adopt a condition that only concerns comparisons of elements that are completely evenly spaced with elements that are not. In order to formulate such a condition, we must first define the expression ‘completely evenly spaced’. To do this, we first index the elements of each set with positive integers from 1 to n according to the degree of q that they exemplify, so that the elements x1 to xn are ordered such that q(x1) < q(x2) < … < q(xn-1) < q(xn). We then have:
A set A with n elements xi ∈ A, indexed and ordered from 1 to n, has elements that are completely evenly spaced with respect to q, if and only if with respect to their dissimilarities in q, d(x1, x2) = d(x2, x3) … = d(x n-2, xn-1) = d(xn-1, xn).
The Limited Even Spacing Condition: For a diversity measure D, and for all sets A, B such that #A = #B and Ø(A) = Ø(B), if there is a bijection from the elements x ∈ A to the elements y ∈ B such that for each pair of elements (xi, yi) it is that case that q(xi) = q(yi), except for one case (xj, yj) which is such that q(xj) ≠ q(yj) and where the consecutive elements xj-1, xj, xj+1 are completely evenly spaced, whereas the consecutive elements yj-1, yj, yj+1 are not completely evenly spaced, then A is strictly more diverse than B, and thus D(A) > D(B).
The Even Spacing Condition: For a diversity measure D, and for all sets A, B such that #A = #B and Ø(A) = Ø(B), if the elements x ∈ A have values that are completely evenly spaced and the elements y ∈ B have not, then A is strictly more diverse than B, and thus D(A) > D(B).
4.6 Summary of conditions
Three properties were proposed to affect the one-dimensional diversity of a set: the largest dissimilarity between the elements, the number of dissimilar elements and the even spacing of the elements’ aspect values. The importance of these three properties for diversity was captured in several conditions for a measure of diversity: for diameter, a Diameter Condition was accepted (implying a Limited Diameter Condition); for number, a Strict Monotonicity Condition; and for even spacing, an Even Spacing Condition (implied by a Limited Even Spacing Condition, which will be used later). The Diameter Condition requires that a set with a larger diameter is ranked above a set with a smaller diameter, for comparisons between sets with the same number of elements and the same even spacing; the Strict Monotonicity Condition requires that a set is ranked above its proper subsets; and the Even Spacing Condition requires that a set with evenly spaced elements is ranked above a set without evenly spaced elements, for comparisons between sets with the same diameter and the same number of elements. In addition, a Ratio Scale Independence, a Minimal Diversity and a Restricted Growth Condition were accepted. The Scale Independence Condition requires that the measure should preserve ratio relations between degrees of diversity when the underlying dissimilarity measure changes scale; the Minimal Diversity Condition requires that the empty set and singleton sets are classified as offering no diversity and the Restricted Growth Condition requires that the diversity measure does not present diversity as growing excessively with the addition of an element to a set.
All six conditions are independent of one another, mostly because they do not concern the same situations. The Ratio Scale Independence Condition is the only condition that concerns how a diversity measure should respond to a change of scale of the underlying dissimilarity measure. The Diameter Condition is the only condition that concerns comparisons between sets where both cardinality and even spacing are the same but diameter differs. The Even Spacing Condition is the only condition that concerns comparisons between sets where both cardinality and diameter are the same but the distribution of exemplified aspect values differs. The other three conditions concern comparisons between sets of different cardinality. However, the Minimal Diversity Condition concerns only sets of one or fewer members. It does not imply the Strict Monotonicity Condition since that condition applies to sets with a larger cardinality than 1. Neither is it implied by the Strict Monotonicity Condition since that condition does not concern the empty set. The Minimal Diversity Condition does not imply the Restricted Growth Condition since that condition applies to sets of all sizes. Neither is it implied by the Restricted Growth Condition since that condition does not exclude that the empty set or singleton sets could have a diversity value above 0. Moreover, the Restricted Growth Condition does not imply the Strict Monotonicity Condition since it is compatible with additional elements not adding additional value. Neither does the Strict Monotonicity Condition imply the Restricted Growth Condition since it does not exclude the possibility that D((x, y)) could be 0. All of these conditions are fulfilled by the proposed measure, which we will consider in the sixth section. First, however, we shall look at some previously proposed measures which do not satisfy all of the conditions. These measures are not designed specifically for one-dimensional ratio scale dissimilarity relations, but they are still relevant to consider as they are designed for diversity regarded as a function of dissimilarities.
5. Previous measures
The presentation of previously proposed measures will be brief, as I will only present a list of measures and a table that shows how they fare in relation to the proposed conditions. All the proposals are designed for diversity as a function of dissimilarities (sometimes represented by metric space distances). The measures in question are:
The Total Dissimilarity Measure, which measures diversity by the total sum of all dissimilarities between all elements of a set.Footnote32
The Average Dissimilarity Measure, which measures diversity by the average dissimilarity between all elements of a set.Footnote33
The Range Measure, which measures diversity by the diameter of a set.Footnote34
The Lexical Measure, which measures diversity (ordinally) by comparing sets with respect to their largest dissimilarity, their second largest dissimilarity and so on.Footnote35
The Average Aggregation Measure, which measures diversity by aggregating the average dissimilarity from each element to all the other elements of the set (disregarding the dissimilarity between an element and itself).Footnote36
The Maximal Aggregation Measure, which measures diversity by aggregating the maximal dissimilarity from each element to the other elements of the set.Footnote37
The Minimal Aggregation Measure, which measures diversity by aggregating the minimal dissimilarity from each element to the other elements of the set (disregarding the dissimilarity between an element and itself).Footnote38
The Elimination Measure, which measures diversity by aggregating dissimilarities selected by an iterative procedure where elements are eliminated one by one.Footnote39
The Minimal Path Measure, which measures diversity by aggregating those dissimilarities which connect all elements of a set by a minimal path.Footnote40
These measures are described and characterised in more detail in the literature where they occur. Here we shall only look at how they fare with regard to the proposed conditions. This is captured in the following table:
Diversity measures and conditions
The measures fare the worst with respect to the Even Spacing Condition, since this condition is satisfied only by the Minimal Aggregation Measure. This can be shown with some examples. Let us first consider the set A = (20, 30, 40) which has values that are evenly spaced. This set should be ranked above B = (20, 21, 40) which does not have values that are evenly spaced. However, the Total Dissimilarity Measure ranks A as equally diverse as B and so does the Average Dissimilarity Measure, the Range Measure, the Average Aggregation Measure and the Minimal Path Measure. The Lexical Measure and the Maximal Aggregation Measure even rank B over A. Although the Elimination Measure ranks A over B, as the condition requires, it ranks (10, 17.5, 25, 40) above (10, 20, 30, 40), and so it does not fulfil the condition either.
6. The shortest path root measure
We have finally come to the measure proposed in this essay.
To construct this measure we first index and order all the n elements x in the set A that we are to assess, in accordance with their degree of Q, measured by q. (Note again that elements that have the same degree of Q are considered to be identical elements.) The elements are indexed from 1 to n, as x1 to xn, in such a way that q(x1) < q(x2) … q(xn-1) < q(xn). The dissimilarity d between two elements, xi and xj with respect to Q is then measured by their absolute difference in q. Last we define the diversity measure as follows:
D(A, d) = , where D is a measure of diversity, A is a set, d is a measure of dissimilarity, as defined above, n is the number of elements in A, xi are elements in A, indexed and ordered from 1 to n, as described above, and 0 < r < 1 and α > 0.
D(A, d) =
The Shortest Path Root Measure satisfies all of the six conditions. The measure satisfies the Scale Independence Condition, since when all the dissimilarities between the elements of A are multiplied by K, D(A) is multiplied by Kr . The measure satisfies the Minimal Diversity Condition, since it does not assign any value at all to the empty set and singleton sets. The measure satisfies the Diameter Condition, since it is a strictly increasing function of dissimilarity values. The measure satisfies the Restricted Growth Condition, since D(A) cannot grow by more than D((x, y)) when y is added to A (in fact, unless A is a singleton set, it always grows by less). The measure satisfies the Even Spacing Condition, since it is an additively separable strictly concave function of dissimilarities between adjacent aspect values.Footnote41 Lastly, the measure satisfies the Strict Monotonicity Condition, for two reasons: (i) when an element y is added that expands the diameter of a set A, the measure satisfies the condition because it is a strictly increasing function of dissimilarity values; (ii) when an element y is added that does not expand the diameter of A (because it is added in between two other elements), the measure satisfies the condition because it is an additively separable strictly concave function. It thus has the property of strict subadditivity, a property which is defined as follows:
A function f is strictly subadditive if and only if f(d1 + d2) < f(d1) + f(d2) holds for all di > 0.
7. The uniqueness of the shortest path root measure
The Shortest Path Root Measure satisfies the six conditions, but one may wonder how unique the measure is in this regard. As it turns out, it is possible to show that the Shortest Path Root Measure is unique among continuous analytic functions in satisfying all of the conditions in combination with a Symmetry Condition. More precisely, it is possible to show that the Shortest Path Root Measure is the only continuous analytic function that jointly satisfies the Ratio Scale Independence Condition, the Limited Diameter Condition, and the Limited Even Spacing Condition, together with a Symmetry Condition. A proof of this can found in the Appendix.
The restriction to continuous analytical functions is standard for characterisation proofs of various measures. Symmetry assumptions are standard too, but worth discussing. Since the Shortest Path Root Measure is a function of the shortest path distances, the symmetry condition that the measure satisfies concerns only these distances. We can thus formulate a relevant symmetry condition as follows:
The Symmetry Condition: For a diversity measure D, and for all sets A, B such that #A = #B if there is a bijection from the elements x ∈ A to the elements y ∈ B so that for each pair of dissimilarities between adjacent elements (d(xi, xi+1), d(yj, yj+1)), it is that case that d(xi, xi+1) = d(yj, yj+1), then A is equally diverse as B, and thus D(A) = D(B).Footnote42
Against the view that a one-dimensional diversity measure should be symmetric, one could propose the following counterexample: Consider the following two sets of objects: A = (1, 2, 11, 12) and B = (1, 2, 3, 12). The vectors of shortest path distances are dASP = [1, 9, 1] and dBSP = [1, 1, 9]. According to the Symmetry Condition, the two sets are equally diverse. However, A is intuitively more diverse than B. This shows that the sizes of the dissimilarities are not the only things that matter, but something else matters as well. One proposal is that what additionally matters is the shortest path dissimilarities between the shortest path dissimilarities, and the shortest path dissimilarities between the shortest path dissimilarities between the shortest path dissimilarities a.s.o. (The vector of shortest path dissimilarities between the shortest path dissimilarities is [8, 8] for A, and [0, 8] for B.)
If we wish to accommodate the above intuition, we cannot use a symmetric measure on vectors of shortest path distances but must use an asymmetric measure. A potential method would be to use a lexical measure which first compares aggregate dissimilarities, modified by a root function, and if equal, compares aggregate dissimilarities between dissimilarities, modified by a root function and, if equal, continues this process until there are no values left to aggregate. I will not discuss the virtues or problems of such a complex measure here, however, but will just note that one could argue both for and against symmetrical one-dimensional diversity measures.
8. Conclusion
In this essay I have introduced a new kind of diversity measure, designed for sets with elements that differ only in a single aspect, which I called the Shortest Path Root Measure. The measure is responsive to the magnitude of the diameter, the number of dissimilar elements and the even spacing of the elements’ aspect values. More precisely, it satisfies six necessary conditions for a single aspect diversity measure: Ratio Scale Independence, Minimal Diversity, Diameter, Restricted Growth, Strict Monotonicity, and Even Spacing. By satisfying these conditions the measure improves on previous proposals, which do not satisfy all of them. In addition, it can be shown that the Shortest Path Root Measure is the only one, within the class of continuous analytic functions, which jointly satisfies four conditions: the Ratio Scale Independence Condition, listed above, a Limited Diameter Condition (implied by the Diameter Condition), a Limited Even Spacing Condition (implying the Even Spacing Condition, assuming transitivity) and a Symmetry Condition. If these conditions are necessary for a one-dimensional diversity measure, then the Shortest Path Root Measure is necessary for measuring one-dimensional diversity as well.Footnote44
Disclosure statement
No potential conflict of interest was reported by the author(s).
Notes
1 Philosophical overviews can be found in for example Page Citation2007 (epistemology), Gravel Citation2009 (philosophy of science), Audi Citation2007 (ethics), Crowder Citation2007 (political philosophy), and Bossert, Pattanaik, and Xu Citation2003 (decision theory).
2 For number of elements diversity measures, see Van Hees (Citation2004) and Gravel (Citation2009); for number of attributes diversity measures, see Walker et al (Citation1999), Nehring and Puppe (Citation2002), and Shi and Eberhart (Citation2008); and for number of classes diversity measures, see Connell and Orias (Citation1964) and Lloyd and Ghelardi (Citation1964).
3 For ordinal diversity measures, see Nehring and Puppe (Citation2002), Bervoets and Gravel (Citation2007), and Pattanaik and Xu (Citation2008).
4 For multiple aspect diversity measures, see Rao (Citation1982), Wen, Wang, and Cheng (Citation1998), Barker and Martin (Citation2000), Ursem (Citation2002), Bossert, Pattanaik, and Xu (Citation2003), Van Hees (Citation2004), Lacevic, Konjicija, and Avdagic (Citation2007), Lacevic and Arnaldi (Citation2010), and Enflo (Citation2012).
5 Cf. Dowden (Citation2011, 6).
6 See Enflo (Citation2012).
7 Compare Junge who defines homogeneity as similarity among more than two objects (Citation1978, 114).
8 For diversity as cardinality, see Van Hees (Citation2004) and Gravel (Citation2009).
9 For diversity as number of attributes, see Walker, Kinzig, and Langridge (Citation1999) and Nehring and Puppe (Citation2002).
10 For diversity as number of classes, see Connell and Orias (Citation1964) and Lloyd and Ghelardi (Citation1964); for diversity as dissimilarities between classes, see Vane-Wright, Humphries, and Williams (Citation1991), Eiswerth and Haney (Citation1992), Faith (Citation1992), Weitzman (Citation1992), Walker, Kinzig, and Langridge (Citation1999), and Izsák and Papp (Citation2000); for diversity as number and evenness, see Shannon and Weaver (Citation1949), Simpson (Citation1949), McIntosh (Citation1967), Junge (Citation1994), and Justus (Citation2011); for diversity as number and dissimilarities, see Helmus et al. (Citation2007), Chao, Chiu, and Jost (Citation2010) and Dowden (Citation2011); for diversity as abundance and dissimilarities, see Barker (Citation2002); for diversity as evenness and dissimilarities, see Barker (Citation2002), Ricotta (Citation2004); for diversity as number, evenness and dissimilarities, see Warwick and Clarke (Citation1995), Ganeshaiah, Chandrashekara, and Kumar (Citation1997), Shimatani (Citation2001), Mason et al. (Citation2003), Ricotta and Avena (Citation2003), Weikard, Punt, and Wesseler (Citation2006), Helmus et al. (Citation2007), Stirling (Citation2007), and Allen, Kon, and Bar-Yam (Citation2009).
11 For diversity as dissimilarities to elements in the universal set, see Gravel (Citation2009) and Eiswerth and Haney (Citation1992). Cf. Gustafsson (Citation2010). For diversity as dissimilarities to a mean element of the universal set, see Ursem (Citation2002).
12 For diversity as dissimilarities to the mean of a set, see Crocker (Citation1980), Morrison and De Jong (Citation2002), and Shi and Eberhart (Citation2008).
13 For variance, see Fisher (Citation1919); for standard deviation, see Pearson (Citation1894); and for mean deviation, see Eddington (Citation1914).
14 For diversity as totality of dissimilarities, see Barker and Martin (Citation2000).
15 For diversity as selected dissimilarities, see Rao (Citation1982), Wen, Wang, and Cheng (Citation1998), Ursem (Citation2002), Bossert, Pattanaik, and Xu (Citation2003), Van Hees (Citation2004), and Bervoets and Gravel (Citation2007).
16 For diversity as number, equality and dissimilarities, see Enflo (Citation2012). Cf. Dowden (Citation2011).
17 See Suppes and Zinnes (Citation1963, 17–19).
18 See Suppes and Zinnes (Citation1963, 5, 7, 11).
19 See Stevens (Citation1946, 678).
20 See Russell (Citation1903, 179).
21 An early example of dissimilarity represented by distance can be found in Russell (Citation1903, 171). A later example can be found in Shepard (Citation1958, 510). For the original presentation of metric spaces, see Fréchet (Citation1910). Discussion of these and other dissimilarity measures can be found in Enflo (Citation2020).
22 Similar scale independence conditions have previously been proposed by Solow and Polasky (Citation1994, 97–98), Hubálek (Citation2000, 255), Mason et al. (Citation2003, 573), and Dowden (Citation2011, 4).
23 Shannon’s diversity (and entropy) index (Citation1949) and Rényi’s diversity (and entropy) index (Citation1961) are examples of logarithmic measures.
24 See Dowden (Citation2011, 3).
25 See Bossert, Pattanaik, and Xu (Citation2003, 418), Bervoets and Gravel (Citation2007, 264), and Gravel (Citation2009, 39).
26 This diameter condition is implied by Weitzman’s Monotonicity in Distances condition (Weitzman Citation1992, 392) and Nehring and Puppe’s Monotonicity in Dissimilarity condition (Citation2002, 1182).
27 For the view that diversity is independent of number, see for example Patil and Taillie (Citation1982, 548).
28 Cf. Page (Citation2010, 59).
29 This restricted growth condition is rather similar to a condition proposed by Weitzman (but for species). See Weitzman (Citation1992, 392).
30 Similar monotonicity conditions have been proposed previously by Solow, Polasky, and Broadus (Citation1993, 62), Bossert, Pattanaik, and Xu (Citation2003, 413), Van Hees (Citation2004, 258), Pattanaik and Xu (Citation2008, 264), Gravel (Citation2009, 20), and Dowden (Citation2011, 3). Monotonicity conditions for species have been proposed by Weitzman (Citation1992, 376), Daly, Baetens, and De Baets (Citation2018, 8).
31 Cf. Nehring and Puppe (Citation2003, 177).
32 See Barker and Martin (Citation2000, 1005), Wineberg and Oppacher (Citation2003, 1494), and Hashemi and Endriss (Citation2014, 424).
33 See Rao (Citation1982, 3) and Wen, Wang, and Cheng (Citation1998, 773).
34 See Gravel (Citation2009, 43). Cf. Shi and Eberhart (Citation2008, 1064).
35 See Bervoets and Gravel (Citation2007, 264–265).
36 See Van Hees (Citation2004, 263).
37 See Van Hees (Citation2004, 261).
38 See Weitzman (Citation1992, 367) and Van Hees (Citation2004:, 260).
39 See Weitzman (Citation1992, 375).
40 See Faith (1996, 1286), Nehring and Puppe (Citation2002, 1158), and Lacevic and Arnaldi (Citation2010, 5). Cf. Petchey and Gaston (Citation2002, 403).
41 According to both Kempton (Citation1979) and Rao (Citation1982), concavity is a necessary feature of a diversity measure. Concave measures have also been used for measuring other kinds of properties in areas were even spacing, evenness or equality of some aspect values are considered important. There are, for example, concave egalitarian social welfare measures (in Weirich Citation1983), concave risk averse expected utility measures (in Pratt Citation1964) and concave information measures (in Hartley Citation1928).
42 Symmetry conditions have been proposed for multiple aspect dissimilarity diversity by, for example, Laxton (Citation1978, 53), Jost (Citation2008, 927), Page (Citation2010, 67), Leinster and Cobbold (Citation2012, 9), Hashemi and Endriss (Citation2014, 426), and Daly et al. (Citation2018, 126).
43 That diversity measures should be symmetrical is taken for granted by, for example, Laxton (Citation1978, 53), Kreutz-Delgado and Rao (Citation1999, 1082), Jost (Citation2008, 927), Leinster and Cobbold (Citation2012, 9), and Hashemi and Endriss (Citation2014, 426).
44 I am grateful to Per Enflo, Victor Moberger, and several referees for their help in improving this paper.
45 See Glaeser (Citation1963, 205–206).
References
- Allen, Benjamin, Mark Kon, and Yaneer Bar-Yam. 2009. “A New Phylogenetic Diversity Measure Generalizing the Shannon Index and its Application to Phyllostomid Bats.” The American Naturalist 174 (2): 236–243.
- Audi, Robert. 2007. Moral Value and Human Diversity. New York: Oxford University Press.
- Barker, Allen L., and Worthy N. Martin. 2000. “Dynamics of a Distance-Based Population Diversity Measure.” Proceedings of the IEEE Congress of Evolutionary Computation: 1002–1009.
- Barker, Gary M. 2002. “Phylogenetic Diversity: A Quantitative Framework for Measurement of Priority and Achievement in Biodiversity Conservation.” Biological Journal of the Linnean Society 76 (2): 165–194.
- Bervoets, Sebastian, and Nicolas Gravel. 2007. “Appraising Diversity with an Ordinal Notion of Similarity: An Axiomatic Approach.” Mathematical Social Sciences 53 (3): 259–273.
- Bossert, Walter, Prasanta K. Pattanaik, and Yongsheng Xu. 2003. “Similarity of Options and the Measurement of Diversity.” Journal of Theoretical Politics 15 (4): 405–422.
- Chao, Anne, Chun-Huo Chiu, and Lou Jost. 2010. “Phylogenetic Diversity Measures Based on Hill Numbers.” Philosophical Transactions of the Royal Society B: Biological Sciences 365 (1558): 3599–3609.
- Connell, Joseph H., and Eduardo Orias. 1964. “The Ecological Regulation of Species Diversity.” American Naturalist 98 (903): 399–414.
- Crocker, Lawrence. 1980. Positive Liberty: An Essay in Normative Political Philosophy. Hague: Martinus Nijhoff Publishers.
- Crowder, George. 2007. Theories of Multiculturalism: An Introduction. Cambridge: Polity.
- Daly, Aisling J., Jan M. Baetens, and Bernard De Baets. 2018. “Ecological Diversity: Measuring the Unmeasurable.” Mathematics 6 (7): 119–147.
- Dowden, Chris. 2011. “A New Axiomatic Approach to Diversity.” Computing Research Repository.
- Eddington, Arthur. 1914. Stellar Movements and the Structure of the Universe. London: Macmillan.
- Eiswerth, Mark E., and Christopher J. Haney. 1992. “Allocating Conservation Expenditures: Accounting for Inter-Species Genetic Distinctiveness.” Ecological Economics 5 (3): 235–249.
- Enflo, Karin. 2012. “Measures of Freedom of Choice.” Diss., Uppsala University, Uppsala.
- Enflo, Karin. 2020. Measures of Similarity.” Theoria 86 (1): 73–99.
- Faith, Daniel P. 1992. “Conservation Evaluation and Phylogenetic Diversity.” Biological Conservation 61 (1): 1–10.
- Fisher, Ronald A. 1919. “The Correlation Between Relatives on the Supposition of Mendelian Inheritance.” Earth and Environmental Science Transactions of the Royal Society of Edinburgh 52 (2): 399–433.
- Fréchet, Maurice. 1910. “Les Dimensions D'un Ensemble Abstrait.” Mathematische Annalen 68 (2): 145–168.
- Ganeshaiah, K. N., K. Chandrashekara, and A. R. V. Kumar. 1997. “Avalanche Index: A New Measure of Biodiversity Based on Biological Heterogeneity of the Communities.” Current Science 73 (2): 128–133.
- Glaeser, Georges. 1963. “Fonctions Composées Différentiables.” Annals of Mathematics 77 (1): 193–209.
- Gravel, Nicolas. 2009. “What is Diversity?” In Economics, Rational Choice and Normative Philosophy, edited by Thomas A. Boylan, and Ruvin Gekker, 15–55. London: Routledge.
- Gustafsson, Johan E. 2010, “Freedom of Choice and Expected Compromise.” Social Choice and Welfare 35 (1): 65–79.
- Hartley, Ralph V. L. 1928. “Transmission of Information.” Bell System Technical Journal 7 (3): 535–563.
- Hashemi, Vahid, and Ulle Endriss. 2014. “Measuring Diversity of Preferences in a Group.” ECAI'14: Proceedings of the Twenty-first European Conference on Artificial Intelligence: 423–428.
- Helmus, Matthew R., Thomas J. Bland, Christopher K. Williams, and Anthony R. Ives. 2007. “Phylogenetic Measures of Biodiversity.” The American Naturalist 169 (3): E68–E83.
- Hubálek, Zdenek. 2000. “Measures of Species Diversity in Ecology: An Evaluation.” Folia Zoologica 49 (4): 241–269.
- Izsák, János, and László Papp. 2000. “A Link Between Ecological Diversity Indices and Measures of Biodiversity.” Ecological Modelling 130 (1–3): 151–156.
- Jost, Lou. 2008. “Mismeasuring Biological Diversity: Response to Hoffmann and Hoffmann.” Ecological Economics 68 (4): 925–928.
- Junge, Kenneth. 1978. “A General Parametric Model of Similarity and Subjective Difference.” Scandinavian Journal of Psychology 19 (1): 111–119.
- Junge, Kenneth. 1994. “Diversity of Ideas About Diversity Measurement.” Scandinavian Journal of Psychology 35 (1): 16–26.
- Justus, James, et al. 2011. “A Case Study in Concept Determination: Ecological Diversity.” In Philosophy of Ecology, edited by Bryson Brown, 147–168. Amsterdam: North-Holland.
- Kempton, Rob A. 1979. “The Structure of Species Abundance and Measurement of Diversity.” Biometrics 35 (1): 307–321.
- Kreutz-Delgado, Kenneth, and Bhaskar D. Rao. 1999. “Sparse Basis Selection, ICA, and Majorization: Towards a Unified Perspective.” 1999 IEEE International Conference on Acoustics, Speech, and Signal Processing Proceedings 2: 1081–1084.
- Lacevic, Bakir, Samim Konjicija, and Zikrija Avdagic. 2007. “Population Diversity Measure Based on Singular Values of the Distance Matrix.” Proceedings of the 2007 IEEE Congress on Evolutionary Computation: 1863–1869.
- Lacevic, Bakir, and Edoardo Arnaldi. 2010. “On Population Diversity Measures in Euclidean Space.” Proceedings of the 2010 IEEE Congress on Evolutionary Computation: 1–8.
- Laxton, R. R. 1978. “The Measure of Diversity’.” Journal of Theoretical Biology 70 (1): 51–67.
- Leinster, Tom, and Christina A. Cobbold. 2012. “Measuring Diversity: The Importance of Species Similarity.” Ecology 93 (3): 477–489.
- Lloyd, Monte, and Raymond J. Ghelardi. 1964. “A Table for Calculating the ‘Equitability’ Component of Species Diversity.” Journal of Animal Ecology 33 (2): 217–225.
- Mason, Norman W. H., Kit MacGillivray, John B. Steel, and J. Bastow Wilson. 2003. “An Index of Functional Diversity.” Journal of Vegetation Science 14 (4): 571–578.
- McIntosh, Robert P. 1967. “An Index of Diversity and the Relation of Certain Concepts to Diversity.” Ecology 48 (3): 392–404.
- Morrison, Ronald W., and Kenneth A. De Jong. 2002. “Measurement of Population Diversity .” Selected Papers from the 5th European Conference on Artificial Evolution 2001: 31–41.
- Nehring, Klaus, and Clemens Puppe. 2002. “A Theory of Diversity.” Econometrica 70 (3): 1155–1198.
- Nehring, Klaus, and Clemens Puppe. 2003. “Diversity and Dissimilarity in Lines and Hierarchies.” Mathematical Social Sciences 45 (2): 167–183.
- Page, Scott E. 2007. The Difference: How the Power of Diversity Creates Better Groups, Firms, Schools, and Societies. Princeton: Princeton University Press.
- Page, Scott E. 2010. Diversity and Complexity. Princeton: Princeton University Press.
- Patil, Ganapati, and Charles Taillie. 1982. “Diversity as a Concept and Its Measurement.” Journal of the American Statistical Association 77 (379): 548–561.
- Pattanaik, Prasanta K., Yongsheng Xu, et al. 2008. “Ordinal Distance, Dominance and the Measurement of Diversity.” In Rational Choice and Social Welfare: Theory and Applications, edited by Prasanta K. Pattanaik, 259–269. Berlin and Heidelberg: Springer Verlag.
- Pearson, Karl. 1894. “Contributions to the Mathematical Theory of Evolution.” Philosophical Transactions of the Royal Society of London A 185: 71–110.
- Petchey, Owen Leonard, and Kevin J. Gaston. 2002. “Functional Diversity (FD), Species Richness, and Community Composition.” Ecology Letters 5 (3): 402–411.
- Pratt, John W. 1964. “Risk Aversion in the Small and in the Large.” Econometrica 32 (1): 122–136.
- Rao, C. Radhakrishna. 1982. “Diversity: Its Measurement, Decomposition, Apportionment and Analysis.” Sankhyā: The Indian Journal of Statistics, Series A 44 (1): 1–22.
- Rényi, Alfred. 1961. “On Measures of Entropy and Information.” Proceedings of the 4th Berkeley Symposium on Mathematics, Statistics and Probability 1960: 547–561.
- Ricotta, Carlo. 2004. “A Parametric Diversity Measure Combining the Relative Abundances and Taxonomic Distinctiveness of Species.” Diversity and Distribution 10 (2): 143–146.
- Ricotta, Carlo, and G. C. Avena. 2003. “An Information-Theoretical Measure of Taxonomic Diversity.” Acta Biotheoretica 51 (1): 35–41.
- Russell, Bertrand. 1903. Principles of Mathematics. Cambridge: Cambridge University Press.
- Shannon, Claude E., and Warren Weaver. 1949. Mathematical Theory of Communications. Urbana: University of Illinois Press.
- Shepard, Roger. 1958. “Stimulus and Response Generalization: Tests of a Model Relating Generalization to Distance in Psychological Space.” Journal of Experimental Psychology 55 (6): 509–523.
- Shi, Yuhui, and Russell C. Eberhart. 2008. “Population Diversity of Particle Swarms.” Proceedings of the 2008 IEEE Congress on Evolutionary Computation: 1063–1067.
- Shimatani, Kenichiro. 2001. “On the Measurement of Species Diversity Incorporating Species Differences.” Oikos 93 (1): 135–147.
- Simpson, Edward H. 1949. “Measurement of Diversity.” Nature 163 (4148): 688.
- Solow, Andrew, Stephen Polasky, and James Broadus. 1993. “On the Measurement of Biological Diversity.” Journal of Environmental Economics and Management 24 (1): 60–68.
- Solow, Andrew, and Stephen Polasky. 1994. “Measuring Biological Diversity.” Environmental and Ecological Statistics 1 (2): 95–107.
- Stevens, Stanley Smith. 1946. “On the Theory of Scales of Measurement.” Science 103 (2684): 677–680.
- Stirling, Andy. 2007. “A General Framework for Analysing Diversity in Science, Technology and Science.” Journal of the Royal Society Interface 4 (15): 707–719.
- Suppes, Patrick, Joseph L. Zinnes, et al. 1963. “Basic Measurement Theory.” In Handbook of Mathematical Psychology, edited by R. Duncan Luce, 1–76. Hoboken, NJ: John Wiley and Sons.
- Ursem, Rasmus K. 2002. “Diversity-Guided Evolutionary Algorithms.” Proceedings of the International Conference on Parallel Problem Solving from Nature VII: 462–474.
- Van Hees, Martin. 2004. “Freedom of Choice and Diversity of Options: Some Difficulties.” Social Choice and Welfare 22 (1): 253–266.
- Vane-Wright, Richard I., Christopher J. Humphries, and Paul H. Williams. 1991. “What to Protect? – Systematics and the Agony of Choice.” Biological Conservation 55 (3): 235–254.
- Walker, Brian, Ann Kinzig, and Jenny Langridge. 1999. “Plant Attribute Diversity, Resilience, and Ecosystem Function: The Nature and Significance of Dominant and Minor Species.” Ecosystems 2 (2): 95–113.
- Warwick, Richard, and K. Robert Clarke. 1995. “New ‘Biodiversity’ Measures Reveal a Decrease in Taxonomic Distinctness with Increasing Stress.” Marine Ecology Progress Series 129 (1–3): 301–305.
- Weikard, Hans-Peter, Maarten Punt, and Justus Wesseler. 2006. “Diversity Measurement Combining Relative Abundances and Taxonomic Distinctiveness of Species.” Diversity and Distribution 12 (2): 215–217.
- Weirich, Paul. 1983. “Utility Tempered with Equality.” Noûs 17 (2): 423–439.
- Weitzman, Martin L. 1992. “On Diversity.” Quarterly Journal of Economics 107 (2): 363–405.
- Wen, Jinyu, Shaorong Wang, and Shjie Cheng. 1998. “Measurement Based Power System Load Modeling Using a Population Genetic Algorithm.” POWERCON'98. 1998 International Conference on Power System Technology. Proceedings 1: 771–775.
- Wineberg, Mark, and Franz Oppacher. 2003. “The Underlying Similarity of Diversity Measures Used in Evolutionary Computation.” Genetic and Evolutionary Computation Conference: 1493–1504.
Appendix
The uniqueness of the shortest path root measure
Uniqueness theorem: If a diversity measure D is a continuous analytic function of a vector of positive real numbers, representing the dissimilarities between the elements of a set with respect to the elements’ values of some single aspect q, and if D satisfies the four conditions of Limited Diameter, Limited Even Spacing, Symmetry and Ratio Scale Independence, then D is a Shortest Path Root Measure.
* * *
The conditions and the measure:
The Limited Diameter Condition: For a diversity measure D and any sets A and B and any elements x, y, w, z, such that A = (x, y) and B = (w, z), if d(x, y) > d(w, z), then A is strictly more diverse than B and thus D(A) > D(B).
The Limited Even Spacing Condition: For a diversity measure D, and for all sets A, B such that #A = #B and Ø(A) = Ø(B), if there is a bijection from the elements x ∈ A to the elements y ∈ B such that for each pair of elements (xi, yi) it is that case that q(xi) = q(yi), except for one case (xj, yj) which is such that q(xj) ≠ q(yj) and where the consecutive elements xj-1, xj, xj+1 are completely evenly spaced so that d(xj-1, xj) = d(xj, xj+1), whereas the consecutive elements yj-1, yj, yj+1 are not completely evenly spaced so that d(yj-1, yj) ≠ d(yj, yj+1), then A is strictly more diverse than B, and thus D(A) > D(B).
The Symmetry Condition: For a diversity measure D, and for all sets A, B such that #A = #B if there is a bijection from the elements x ∈ A to the elements y ∈ B so that for each pair of dissimilarities between adjacent elements (d(xi, xi+1), d(yj, yj+1)), it is that case that d(xi, xi+1) = d(yj, yj+1), then A is equally diverse as B, and thus D(A) = D(B).
The Ratio Scale Independence Condition: For a diversity measure D that is a function of a dissimilarity function d, it holds that for all numbers K > 0, there is a number L = L(K) > 0, where L is an increasing function of K such that if d is multiplied by K, then D is multiplied by L.
D(A, d) = α, where D is a measure of diversity, A is a set, d is a measure of dissimilarity d(xi, xj) = |q(xi) – q(xj)|, n is the number of elements in A, and xi are elements in A, indexed and ordered from 1 to n, as x1 to xn, in such a way that q(x1) < q(x2) … q(xn-1) < q(xn) and where 0 < r < 1 and α > 0.
* * *
Assumptions
We assume that the one-dimensional diversity of a set is a function of the single aspect dissimilarities between the elements of a set, which in turn is a function of the elements’ values of some single aspect q. Consequently, the one-dimensional diversity of a set is ultimately a function of the element’s values of q. We also assume that there is a real valued measure q of some single aspect Q and that we can measure the dissimilarity d between two objects, x and y, with respect to Q, by the absolute difference between q(x) and q(y).
We further assume that there is a finite universal set of objects, X. We index all the elements of X from 1 to n, as x1, x2 … xn−1, xn so that q(x1) < q(x2) < … q(xn−1) < q(xn). The same principle is used to index the elements of subsets of X. All the shortest path dissimilarities between the elements of X are indexed so that d1 = d(x1, x2), d2 = d(x2, x3) etc.
Proof
First, we make the assumption that a one-dimensional diversity measure D is a continuous analytic function of a vector of positive real numbers, representing the dissimilarities between the elements of a set with respect to the elements’ values of some single aspect q.
We will see that under very general assumptions on the diversity measure D, the shortest path dissimilarities d(xi, xi+1) = | q(xi) – q(xi+1) |, 1 ≤ i ≤ n – 1, play a special role when measuring the one-dimensional diversity of a set. We will prove the following theorem:
Theorem: If the diversity D of a set A is given by a strictly concave, continuous function D of a vector of shortest path dissimilarities between the elements of A with respect to q, and thus of a vector of values of the elements of A with respect to q, and if q(x1) = a and q(xn) = b are fixed, then D is maximized when d(xi, xi+1) = d(xj, xj+1) = (b – a)/(n – 1), for all i and j, 1 ≤ i ≤ n – 1, 1 ≤ j ≤ n – 1.
From (I) we get the Theorem as follows: Assume that for a set B* not all the d(xi, xi+1):s are the same. Then there must be one i, for which d(xi, xi+1) ≠ d(xi+1, xi+2) for B*. Then, by forming a set A* for which q(xi+1) = 1/2(q(xi) + q(xi+2)), we get from (I) that D(A*) > D(B*). Thus D(B*) is not maximal and thus the Theorem is proved.
That D satisfies the Limited Even Spacing Condition trivially implies that D is a strictly concave, continuous function of a vector of shortest path dissimilarities between the elements of A with respect to q.
Next, let the shortest path distances between the elements of A be written as a vector dASP = [d1, d2, … , dm] with m = the number of shortest path distances, m = n – 1. We get D(A, d) = D(f(d1), f(d2), … , f(dm)). The Symmetry Condition gives that D is a symmetric function. Since D is an analytic and symmetric function of f(d1), f(d2), … , f(dm), the expansion of a differentiable symmetric function via elementary symmetric functions gives:
D(A, d) = D(dASP) = +
+
+ higher order terms in the f(di) f(dj)’s. … (I)Footnote45
Now consider A = Aj with d1 = d2 = … = dj = d and dj+1 = dj+2 = … = dm = 0, 1 ≤ j ≤ m (this is hypothetical since we have assumed that we cannot have 0 distances without identity). Putting this into (I) gives:
D(Aj) = gj(d) = jC(n1) f(d) +{C(n2)J + C(n3) j}(f(d))2 + higher order terms with J = j(j – 1) = the number of (di)(dj)’s different from 0. … . (II)
= αdr = C(n1) f(d) +{C(n2)
+ C(n3) }(f(d))2 + higher order terms … (III)
We thus see that the acceptance of the four conditions necessitates a Shortest Path Root Measure.
Q.E.D.