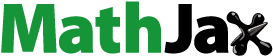
Abstract
We describe the design and evaluation of an innovative course for beginning undergraduate mathematics students. The course is delivered almost entirely online, making extensive use of computer-aided assessment to provide students with practice problems. We outline various ideas from education research that informed the design of the course, and describe how these are put into practice. We present quantitative evaluation of the impact on students' subsequent performance (N = 1401), as well as qualitative analysis of interviews with a sample of 14 students who took the course. We find evidence that the course has helped to reduce an attainment gap among incoming students, and suggest that the design ideas could be applied more widely to other courses.
1. Introduction
The transition to university mathematics is known to be difficult; in part because of ‘the new demands of “advanced mathematical thinking” at university’, but also because of ‘the social and “cultural” aspects of transition’ (Williams, Citation2015, p. 26). Prior research has found that mathematics and engineering students are among the least positive about their experience of transition to university (Pampaka et al., Citation2012), and that over the course of the first year of university mathematics, students' beliefs about the discipline become less consistent with the consensus expressed by a group of mathematicians (Code et al., Citation2016). Students' performance on programme is also strongly related to their mathematics entry qualifications (Chongchitnan, Citation2019; Rach & Ufer, Citation2020).
To address these issues, the University of Edinburgh recently introduced a new course, ‘Fundamentals of Algebra and Calculus’ (FAC). The course is offered in the first semester of Year 1, and is delivered almost entirely online so that it can be offered to a potentially large group of students with efficiency in terms of staffing, estates and timetabling. It runs in parallel with a linear algebra course that has in-person classes, so students' overall experience is of blended learning. Students are advised to take the course based on their entry qualifications and their performance in a diagnostic test (Kinnear, Citation2018) taken before the start of semester. The course makes extensive use of computer-aided assessment, and its design draws on a variety of ideas from cognitive science and mathematics education research.
The aim of this paper is to describe the design and implementation of the course, and to present the results of our evaluation of it. In particular, we address the following questions:
How can ideas from cognitive science and education research be implemented in an online mathematics course, making use of computer-aided assessment?
What effect does the course have on students' performance in mathematics?
How do students respond to the approaches used to teach the course?
To address these questions, we first present a series of ideas drawn from the literature and describe how the course puts each idea into practice (Section 2). Following this, we draw on data from three cohorts of students (N = 1401) to compare the subsequent performance of those who took the course with those who did not (Section 3). Finally, we report on the results of interviews conducted with a sample of 14 students who took the course (Section 3).
2. Course design
In this section, we will discuss research findings that relate to various aspects of course design, and illustrate how these have informed the design of our course, FAC.
First, some words on how FAC fits into the curriculum. At the University of Edinburgh, undergraduate students normally take 60 credits of courses per semester, with courses typically offered in units of 10 or 20 credits (and 10 credits notionally requiring 100 hours of work). On mathematics programmes, there are three compulsory 20 credit courses in year 1, with the remaining 60 credits to be chosen from other disciplinesFootnote1. The compulsory mathematics courses are Introduction to Linear Algebra (ILA) taught in semester 1, and Calculus and its Applications (CAP) and Proofs and Problem Solving (PPS) taught in semester 2. FAC is offered as an option in semester 1, so mathematics students would study it alongside ILA and one other option.
2.1. Syllabus
The FAC course is aimed partly at better preparing students for the semester 2 calculus course, CAP. The intention was to allow CAP to be better focussed, by assuming a more consistent background of calculus concepts among the class. The topics in FAC are thus drawn from those that are considered to be fundamental concepts and skills for an understanding of first-year calculus (Sofronas et al., Citation2011).
2.1.1. Putting it into practice
The topics in FAC are based on the content of typical high school syllabuses (in particular, SQA Advanced Higher Mathematics and GCE A-Level Further Mathematics), with a focus on calculus methods and supporting algebraic work. The differentiation content includes chain, product and quotient rules, as well as implicit, parametric and logarithmic differentiation. The integration content includes a basic appreciation of the definition in terms of Riemann sums, but mainly focuses on techniques such as substitution, integration by parts and integrating rational functions using partial fractions.
Since the semester consists of 11 weeks, we have created 10 units which are studied in the first 10 weeks, with a final assessment taking place in week 11. Each of the ten weekly units is dedicated to one specific topic falling (broadly) under one of the categories of ‘Algebra’ or ‘Calculus’, as shown in Table .
Table 1. Weekly schedule of topics in the course, alternating between algebra and calculus.
2.2. Distributed practice
University courses typically involve multiple opportunities for students to be exposed to information or experiences pertaining to gaining new knowledge or skills, be they no more than a number of practice questions at the end of each chapter of a textbook. The distribution in time of such opportunities on any one particular topic has an effect on how well the associated knowledge and skills are retained. Specifically, a consistent finding in cognitive science is that spaced practice is better than massed practice (Cepeda et al., Citation2008). One study with a precalculus course for engineering students compared the effect of distributing questions on a topic across multiple quizzes rather than having them all in the quiz associated to the topic (Hopkins et al., Citation2016). Spacing out the questions in this way required students to engage in more effortful retrieval of the learned solution procedure from memory, and the study found that it resulted in students performing better on the exam and in a subsequent course.
2.2.1. Putting it into practice
To introduce gaps between study of the same or related topics, we interleave the algebra and calculus weeks, and break up as far as possible the weeks on differentiation and integration within that interleaving. The weekly schedule for the course is shown in Table .
The material for each weekly unit is released at the beginning of the appropriate week of the semester, so students following the course as materials become available will follow this pattern of study.
Furthermore, in a similar way to the precalculus course described by Hopkins et al. (Citation2016), assessments later in the course draw on skills from previous units. This often arises in a natural way; for instance, finding areas between curves using integration naturally builds in recall practice of the procedure for finding intersections of curves.
2.3. Exposition interspersed with questions
There is a growing body of evidence that active learning approaches are preferable to traditional lecturing (Freeman et al., Citation2014). Findings from cognitive science perhaps offer an explanation for the effectiveness of active learning approaches. For instance, the testing effect is the well-established finding that ‘retrieval of information from memory produces better retention than restudying the same information for an equivalent amount of time’ (Roediger & Butler, Citation2011, 20). This gives support to the idea of designing courses so that students are routinely retrieving from memory, as in active learning, and one way to do this is by mixing questions into the exposition.
The testing effect has been seen in numerous laboratory studies (Roediger & Karpicke, Citation2006), and also in classroom settings. This has given rise to the notion of ‘test-enhanced learning’, where tests are used as a tool to help students to learn during a course (Brame & Biel, Citation2015).
The question then arises of where in the exposition to include questions. Questions could be reserved for the end of a substantial piece of content, or interspersed throughout. Evidence suggests that neither approach alone has an advantage over the other, but that being tested twice – i.e. combining both approaches – has a definite benefit (Uner & Roediger, Citation2018).
2.3.1. Putting it into practice
The course is designed around the fundamental principle of ‘putting the textbook in the quiz’. The learning materials comprise a set of coherently organized digital exercises and expositions (CitationSangwin & Kinnear, Citationin press). Short blocks of text, worked examples, interactive diagrams, and short, focused video clips are embedded within a sequence of exercises and questions. Putting all the textbook content into a quiz allows us to mix exposition and questions, promoting active learning.
Students work through the quizzes, and success on the course is judged by their success on the quizzes. There are no textbooks, lecture notes, or regular timetabled teaching events. A student's grade on the course is determined by combining the results of the 10 weekly Unit Tests (together worth 80% of the grade) with a final 2-hour test covering topics from the whole course (worth the remaining 20%). We give further details on grading in the next section.
Each of the 10 weekly units has a consistent structure, with
a ‘Getting Started’ section, which motivates the week's topic and reviews pre-requisite content (e.g. differentiation facts when starting integration),
four sections of content, each of which is designed to take around 2 hours to complete (roughly equivalent to one lecture plus associated practice),
a 90-minute ‘Practice Quiz’ with a mix of questions on the week; this can be taken an unlimited number of times, with full feedback provided on each attempt, and
a 90-minute ‘Unit Test’ which is similar in style to the Practice Quiz, but only allows a single attempt.
All of these are implemented as quizzes in the Moodle virtual learning environment, making extensive use of the STACK question type (Sangwin, Citation2013). To harness the benefits of multiple testing, sections of content often include a final set of mixed practice questions on the entire section, in addition to the questions interspersed with the exposition. An example of this can be seen in the excerpt from the course shown in Figure , which also illustrates the other features of the course materials. A full weekly unit is available for demonstration purposes at https://stack-demo.maths.ed.ac.uk/demo (August 2021).
Figure 1. An excerpt from section 4.2 of FAC, on computing antiderivatives. This shows (a) the use of the Moodle quiz structure to create pages and sections, and the use of a ‘mixed practice’ section at the end of the quiz (b) the use of expository text, (c) an embedded video of a worked example with narration, (d) related STACK questions integrated with the text, in this case showing the validation and feedback provided to students, (e) fully worked solutions are provided once a student has attempted a question; where questions are randomized, these worked solutions reflect the version shown to the student. At the end of the worked solution, there is a ‘Try another question like this one’ button, that replaces the question with a different random variant for further practice.
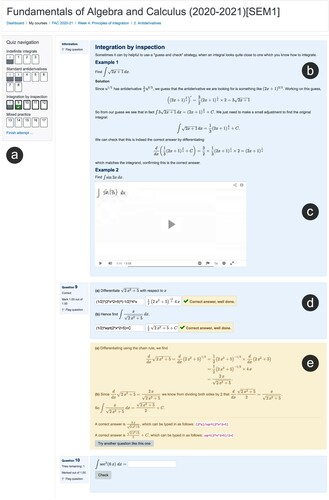
2.4. Specifications grading
Specifications grading is an approach to grading in which individual assessments are simply graded as pass/fail, and, with some opportunity for resubmission, the final grade for the course is determined by the student's performance across these multiple assessments(Nilson, Citation2014). When applied to human-marked assessment, the markers' time is not spent deciding on a numerical value (e.g. a mark out of 10) for a submission, but on the (typically easier) task of determining whether the submission meets the standards required. Feedback on failing submissions is targeted to helping students understand why the work was not satisfactory, enabling them to attempt to improve and resubmit. The system has been used in a pure mathematics context (Williams, Citation2018).
2.4.1. Putting it into practice
Each weekly Unit Test was graded as Mastery (80%+), Distinction (95%+), or otherwise as a Fail. The final grade across the 10 weekly tests (accounting for 80% of the course grade) was then determined by the number of weekly units passed at which level, as shown in Table . In particular, to pass the course overall, Mastery level in at least 7 weekly units was required. Thus high expectations were set: even just a passing mark demanded a numerical outcome of at least 80% in 7 out of 10 weekly assessments. The same threshold was imposed on each weekly Practice Quiz: the Unit Test was not unlocked for attempts until at least 80% was gained on the Practice Quiz.
Table 2. Table used to decide grades based on the results of the 10 unit tests completed during the semester.
2.5. Learning community
Previous research has noted that it is particularly important for students in transition from school to university to have opportunities to meet and work with other students (Williams, Citation2015). We were also mindful of concerns that online mathematics courses ‘could provoke a return to a backward pedagogy, with learners’ participation reduced to reading and individual work on exercises' (Engelbrecht & Harding, Citation2005, 256). Thus, we were keen to augment the course with some opportunities for students to interact with their peers.
2.5.1. Putting it into practice
At an initial meeting in week 1Footnote2, we form students into ‘autonomous learning groups’ of 4–6 at random to work on a collaborative task related to the week 1 content. We set up each group in the course's virtual learning environment so that students have a way of contacting each other after the meeting. In subsequent weeks, we encourage students to meet with their group to complete (non-assessed) tasks for each weekly unit. The logistics of these meetings are left entirely to the students.
2.6. Task design
Research findings also inspired the design and implementation of individual tasks within the course. For instance, we make use of faded worked examples (Renkl et al., Citation2002), example generation prompts (Goldenberg & Mason, Citation2008) and embed opportunities for students to engage in retrieval practice relating to earlier topics (Hopkins et al., Citation2016). Details of all of these aspects have been published previously (Kinnear, Citation2019).
3. Quantitative evaluation
Here we address the research question what effect does the course have on students' performance in mathematics?
We note that this is not an experimental intervention: students were not randomly allocated to take FAC, but instead were advised to take it based on their entrance qualifications. Thus, we cannot be definitive in attributing any effects to the course itself. Nevertheless, this sort of quasi-experimental evaluation is routine and worth doing (Gopalan et al., Citation2020).
3.1. Method
We will use two different approaches, described in the following sections. Before proceeding, we first outline the Bayesian approach used to analyze the data. We use this approach since it can produce estimates for parameters of interest, such as the mean score of a group on a given test, along with easily interpretable indications of the uncertainty about those estimates:
‘In a Bayesian framework, the uncertainty in parameter values is represented as a probability distribution over the space of parameter values. Parameter values that are more consistent with the data have higher probability than parameter values that are less consistent with the data’. (Kruschke, Citation2018, p271)
The Bayesian approach takes a prior distribution of probabilities and updates this in light of the data to produce a posterior distribution of probabilities: the results therefore depend on the choice of priors. In the analyses we report here, we used uniform priors, but the results are not substantially changed when different priors are used. We summarize these distributions using the median and 95% highest density interval (HDI). The HDI is the range of values around the median value which contain 95% of the mass of the posterior probability distribution, and has the property that no value outside the HDI is more credible than a value inside the HDI (Kruschke, Citation2018). So, for instance, if one group's gain is estimated as +2.5 with 95% HDI [1.9, 3.2], this would be viewed as evidence of a positive impact on this group since the HDI takes only positive values.
3.1.1. Pre- and Post-test
Our first approach is to compare students' scores on a Pre- and Post-test. This is a commonly used approach (e.g. Carlson et al., Citation2010; Maciejewski, Citation2015). The test we use is our institution's own Mathematics Diagnostic Test (MDT). This test has a more procedural focus than instruments such as the Precalculus Concept Assessment (Carlson et al., Citation2010), but nevertheless has been found to correlate well with subsequent performance in first-year courses (Kinnear, Citation2018). A sample of the MDT can be found at https://doi.org/10.5281/zenodo.4772435.
For the Pre-test, we use MDT results from the start of the academic year. All students taking first-year mathematics courses are invited to complete the MDT at the start of the academic year. Students have access to the test online once they are enrolled on a mathematics course, which can be up to a month before the start of semester. Completing the test is not mandatory but students are encouraged to complete it before meeting their Personal Tutor to discuss their course choices.
For the Post-test, we use MDT results gathered from the first weekly online assessment in the semester 2 calculus course, Calculus and its Applications (CAP).
3.1.2. Course results
Our second approach is to analyze students' final results on two other mathematics courses, both of which are compulsory for year 1 students in mathematics and computer science:
Introduction to Linear Algebra (ILA), in semester 1. In 2018/19 it was a co-requisite for students enrolled on FAC. In subsequent years this requirement was relaxed, so not all FAC students were taking ILAFootnote3.
Calculus and its Applications (CAP), in semester 2. One of the aims of FAC is to better prepare students to take this course.
For each course, 80% of the final result is based on an exam, with the remaining 20% coming from various coursework tasks such as written homework and online quizzes. Due to the impact of Covid-19, the results for CAP in 2019/20 were decided on the basis of the 20% coursework component alone, and the results for ILA in 2020/21 were based on weekly coursework (worth 60% of the grade) and a final online test completed remotely (worth 40% of the grade).
We gathered data from three cohorts of students, in academic years 2018/19, 2019/20 and 2020/21; Table shows the number of students enrolled in the courses each year, and the proportions where MDT Pre-test data is available. Histograms of all the available test results are shown in the Appendix (Figure ).
Table 3. Summary of the available results data. This includes all students who took one of FAC, ILA or CAP each academic year. The ‘N’ column shows the number of students for whom results are available, and the ‘Took Pre-test’ gives the number (and proportion of N) where corresponding Pre-test data is also available.
3.2. Results
For the Diagnostic Test data, Figure shows a raincloud plot (Allen et al., Citation2019) of the scores for both the Pre- and Post-test, broken down by whether or not students took FAC. This shows each individual's scores as well as summaries of the overall distribution for each group. We have combined the three cohorts as the same pattern is observed in each year, as confirmed by Bayesian model comparisonFootnote4. This shows a difference between the FAC and non-FAC students on the Pre-test, but not on the Post-test.
Figure 2. Raincloud plot of Pre- and Post-test scores for all students, grouped by whether or not students took FAC. Points show individual student scores and these are summarized by boxplots and density functions.
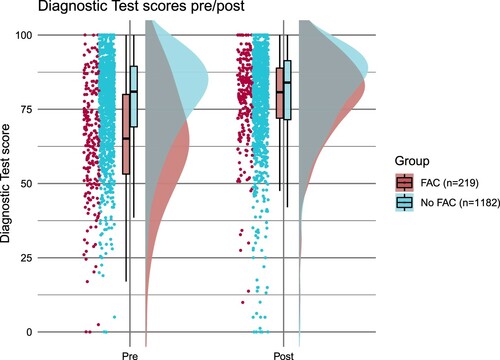
The pattern – that FAC students improve while non-FAC students remain static – was confirmed with a Bayesian ANOVA test (Goodrich et al., Citation2020), which gives estimates for the mean scores for the different groups, and differences between them. As shown in Table , the FAC students had a mean gain of 13.7 percentage points (95% HDI ) while the non-FAC students had a much more modest gain of 1.8 percentage points (95% HDI
). The difference between the mean Post-test results for the two groups was
(95% HDI
), confirming that both groups had a similar level of performance on the Post-test.
Table 4. Results of a Bayesian ANOVA estimating the mean Pre- and Post-test scores for FAC and non-FAC students, along with estimates for the gain in scores between Pre- and Post-test for each group.
An alternative way of viewing these results is given in the leftmost panel of Figure . This shows a linear regression where Post-test scores are predicted on the basis of Pre-test scores, with separate regression lines for FAC and non-FAC students. The fact that the FAC students' regression line is ‘lifted up’ relative to the non-FAC regression line parallels the results of the ANOVA test. This regression approach can also be extended to compare the FAC and non-FAC students' performance on other courses.
Figure 3. Plots of students' results on (a) the Post-test, (b) ILA, (c) CAP, against their scores in the Pre-test, along with regression lines (from a Bayesian linear regression) fitted separately for the FAC and non-FAC groups.
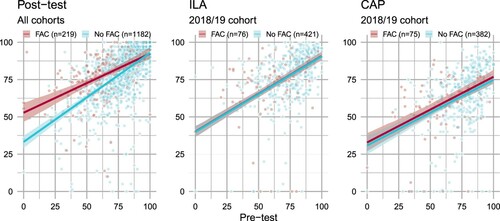
For ILA, suitable data were available from all three cohorts (the histogram in the Appendix confirms that there is a reasonably consistent grade distribution each year). For the Bayesian linear regression, a model comparison approach showed that the best model included a term for the cohortFootnote5, so we do not combine the cohorts for analysis. The middle panel of Figure shows the results for the 2018/19 cohort, where it is apparent that the regression lines for FAC and non-FAC groups are very similar. Inspecting the contrast between FAC and non-FAC groups confirms this, with the difference between the groups estimated at 0.2 (95% HDI ). Since the HDI spans 0, we cannot conclude there is a difference between the groups.
For CAP, final results are currently only available for the 2018/19 and 2019/20 cohorts. As with ILA, model comparison for the Bayesian linear regression showed that the best model included a term for the cohort. The results for the 2019/20 cohort are nonstandard since, as noted earlier, the exam was cancelled due to Covid-19 (and the histogram in the Appendix shows an unusual grade distribution for that class). Therefore, we focus on the 2018/19 cohort, whose results are shown in the rightmost panel of Figure . The regression line for the FAC group appears slightly higher than the non-FAC group – but with the difference between them estimated at 2.0 (95% HDI ), we cannot conclude there is a difference between the groups.
3.3. Discussion
We found that students who took FAC made large gains from the Pre- to Post-test (13.7 percentage points, 95% HDI ), effectively closing an attainment gap that had been present at the start of the academic year. In terms of performance on other year 1 mathematics courses, we did not find any difference overall between the students who took FAC and those who did not.
There are two ways to view the results from ILA and CAP. On the one hand, the fact that the FAC regression lines shown in Figure are not ‘lifted up’ in the same way as for the Post-test could be viewed as a failure to have an impact on students' performance beyond the relatively narrow measure of the Diagnostic Test. On the other hand, since enrolment on FAC was not randomized but was instead based on students (and their academic advisors) identifying a need for further practice with high school mathematics, we cannot rule out the possibility that these students' performance on ILA and CAP would have been lower without FAC. That is, taking FAC may still have led to gains in the students' performance, to bring them into line with their peers. Moreover, we note that the explicit aim of FAC was to bring students to the same level, and these results are in line with that.
There are two further possible limitations to note. First, the Diagnostic Test measure used for the Pre/Post test could be regarded as too easy, as it is essentially based on material that is pre-requisite to the content of FAC. However, previous work has found that scores on this test correlate well with Y1 performance (Kinnear, Citation2018), and it is widely accepted that prior knowledge from school is important for university success (Rach & Ufer, Citation2020).
Second, the Covid-19 pandemic affected two of our three cohorts. The impact on the Pre/Post-test comparison is minimal: the first two cohorts completed these tests before the pandemic, so only the 2020/21 cohort was affected. In fact, the pattern of results for the 2020/21 cohort is remarkably consistent with previous years, as can be seen in the histograms in the Appendix (Figure ). Moreover, in the linear regression, cohort was identified as not being an important predictor. For the ILA and CAP comparisons, we have focused on the 2018/19 cohort which was entirely unaffected by Covid-19, though we note that the results were similar across all cohortsFootnote6.
Overall we have some evidence of the course having a positive impact on students' subsequent performance. In the next section, we explore the impact further by investigating students' experiences of the course.
4. Qualitative evaluation
Here we address the research question how do students respond to the approaches used to teach the course? In line with previous research which has sought to understand students' experiences and attitudes (e.g. Alcock et al., Citation2015; Martínez-Sierra & García González, Citation2014; McGuffey et al., Citation2019), we planned to carry out a mix of focus group discussions and individual interviews with students, during the 2019/20 academic year. This was part of a larger project to evaluate the impact of the course, with a particular focus on its part in ‘widening participation’ (Croxford et al., Citation2014) efforts at our university.
4.1. Method
4.1.1. Participants
Within the 2018/19 and 2019/20 cohorts of FAC students, we identified students who were also taking ILA as a compulsory part of their degree, and for whom the university held contextual data (which in practice meant they were from the UK). Participants were then recruited by an e-mail inviting them to take part in the research. All participants were offered a £10 Amazon voucher as an incentive.
One focus group took part during the first week of the semester with 3 students. We had difficulty recruiting students for further focus groups, so the majority of the data collection took place through individual interviews with the researcher (author 2).
Twelve students took part in one-on-one semi-structured interviews (one of these had also taken part in the focus group at the start of the semester). Of these 8 were male, 4 were female; 5 were current students (taking the course in academic year 2019/20) and 7 had completed FAC in the previous year; 3 were registered with the School of Mathematics and 9 with the School of Informatics. Seven had been identified as fitting into a widening participation category in our university's admissions process.
All participants were given full information about the project including how their data would be handled and rights to withdraw their consent at any time. Ethical approval was granted through the School of Mathematics. During the interviews students were asked about their study approach, their experiences of studying online, their interactions with others during the course and the design of the course including the feedback and assessment regime.
Interviews took place either on Skype or on the phone and were audio recorded. They typically lasted 30–40 minutes. The interviews were then transcribed by a professional transcriber.
4.1.2. Data analysis
Thematic analysis was used to identify common themes related to the ways in which students approached and experienced learning mathematics with FAC. For the main data analysis we took an inductive approach to the analysis, in which codes and subsequent theory are derived from the data itself without any preconceived ideas influencing the analysis.
For a second analysis, we used a deductive approach, with two categories – persistence and confidence – from the MAPS questionnaire (Code et al., Citation2016) used as codes for our data analysis.
Analysis followed the six steps described by Braun and Clarke (Citation2006). This involved familiarization with the data and initial coding, where the transcripts were checked against the audio recordings and read through a number of times in order for the researcher to become familiar with the interviews. Relevant passages were highlighted and notes were made about the key ideas being expressed. Formal coding was then carried out in Excel. As the analysis progressed data extracts and codes were discussed by the team of researchers and themes were developed. Throughout this stage the researchers returned to the data to check that the themes were representative of the interviews, so that themes were developed in an iterative process. Finally, a thematic map was created to show the interconnections between some of the themes (see Figure ).
4.2. Results
The design of both learning environments and learning tasks have an impact on the way in which students approach their learning. Taking an inductive approach to data analysis we found that some aspects of the course design were linked to students taking a strategic/goal-oriented approach (Entwistle & McCune, Citation2004) that led them to ‘gaming the system’, but these aspects of the course were also related to a high degree of persistence and to students having high aspirations for their learning.
A complex interplay was found between students' perceptions of the workload on the course, how new the material was for the students, some design aspects such as the 80% pass mark needed on the practice test to unlock the unit test, and the system attributes, such as the automated marking which necessarily gave marks only for the final answer, with no credit being given for working (as students are used to with human-based marking).
4.2.1. Perceived workload
Workload was of concern to all students with some students feeling that the course took up more time than they were expecting. Student 2 for example said ‘Well, it took up a lot of my time… even if I knew how to do the maths, it was still taking me a long time’ and student 3 commented that the time demand was ‘a lot more than I thought it should have been, especially if you were working through it properly’.
Student 2 also felt that as this was a ‘catch-up’ course rather than a University-level course it should have had a lower work load:
it was a bit frustrating that this was a course aimed at people who were struggling with maths or hadn't got that good grades in maths and then was taking so much time that I ended up spending more time on it than my other courses. (Student 2)
However this was not a universal experience: student 5 felt that this perception of a high workload was not to do with the volume of work itself but rather that the course was all carried out in the same mode (i.e. individually on a computer), compared to other courses which had a variety of different types of activities:
So I don't think it was ever the course per se but it was just the fact that all of the workload is focused in one area. It can feel overwhelming as opposed to some of the courses which are lectures, tutorials, it's all spread among different things. (Student 5)
When workload did impact on the way that students studied, this was often something that they viewed as being detrimental to their learning and resulted in them working in a way that was not their preferred approach to learning. Student 3 for example, when discussing missing out some of the core content, acknowledged that ‘the only disadvantage would be I wouldn't take it, like, in as much. Wouldn't have gained knowledge’ and that she ended up ‘doing it to pass the test, rather than doing it to learn the course’.
Similarly Student 7 commented that his approach would have been different if the workload had been reduced:
And so I think maybe if there were less questions, if I had more time to get less material in me, I think I'd probably stick with my initial way. That's the way I usually engage with maths, right, I try and get it all right, make sure I can do it all, and then sit the test. (Student 7)
4.2.2. Strategic approach and gaming the system
For some students the perception of high workload resulted in them taking a strategic approach to the course, as student 1 commented, ‘it's almost like the goal just becomes passing it, rather than doing well in it’.
One way that this strategic approach manifested was that some students felt that they did not have time to go through the course in the way it was designed to be experienced. Student 7 for example, commented that it was ‘unsustainable’ and as a consequence he ‘adopted a less rigorous and more efficient way of tackling it’.
As hinted at by the comment above, students took alternative approaches to the course to get the desired outcome of passing the unit test. We have termed this ‘gaming the system’. For example some students did not work through the full course materials available each week, but instead opted for directly attempting the practice test.
I: So are you saying you went straight for the test, the practice test?
S: Yeah well, I did comb through the material first. But I skimmed through it. (Student 9)
The automated feedback given by STACK for the incorrect answers was then used by students to fill in gaps in their knowledge.
So near the end when I was trying to sort of pass the practice test to get onto the final one, you could check a lot of your answers before you submitted it. (Student 3)
There were a number of reasons given for this approach: a perception of high workload, as described above, meant that students felt that they didn't ‘have time to go through all the individual parts’ (Student 6) (i.e. the STACK questions) while students who had studied similar topics previously felt that they had already covered the content and that going over it again was unnecessary.
But a lot of the time, I will just go through the practice tests to see what I got wrong and then learn from that answer, in a way. I know recently I have kind of gone for the practice tests because it's a subject I, I've done pretty thoroughly so I think I'll be okay with it and just because of the time restraints [sic]. (Student 6)
Another ‘gaming the system’ approach which students used particularly to save time was to answer the STACK question using an arbitrary value in order to get the feedback that is automatically provided by the system.
I found myself, like I would get to the point where like if I didn't know how to do a question, I would just type in random numbers so that it would show me how to do the question. (Focus Group Student 2)
As above, this seemed to be precipitated by a perceived lack of time, but also indicates a lack of willingness to put in the effort to think about difficult questions.
A lot of the time it'll pose a question and they haven't really indicated how to solve it, and that's quite frustrating… if I know how to do it, I'll do it, and I won't really think too hard about problem solving because of the volume of work, so… if I don't know how to approach the question, I don't really have enough time to ponder very hard about it, so I'll just put usually zero into the answer box. (Student 7)
Yet despite this Student 7 also displayed a realization that this persistence is what is expected of him ‘that's how maths works… if you don't understand then try and do it’.
4.2.3. High pass mark
The high pass mark of 80%, needed both to pass the practice test in order to unlock the unit test, and to pass the unit test itself was something about which students had strong opinions. Two themes were apparent in the data – the frustration that they felt at having to achieve this grade (related to the lack of marks for partial working), and the positive impact that the high grade had on their approach to the work.
Frustration. Many students felt a degree of frustration with aspects of the marking system. One aspect of this was the high pass mark of 80% needed to pass each test, which is much higher than the 40% typical of their other university courses.
I mean, honestly, it was a little bit frustrating because I feel like sometimes you'd be doing it, like, a couple of times and then it's almost like the goal just becomes passing it, rather than doing well in it. (Student 1)
Another aspect on which many students commented was that answers were marked by the system as either right or wrong, with no marks given for partially correct working.
But you could have two, or even one question wrong, from a tiny bit of working error, and that would mean failed the practice. So that was frustrating. (Student 3)
Students felt particularly strongly about this if they had had experience of failing the test by only a few percentage points. As Student 4 points out, a small slip can lead to the answer being incorrect and therefore result in the loss of marks for the entire question:
I remember failing two of them by, I think I got 79 and 75 and I remember being gutted… but given the fact that if you could miss out an X or an X squared and you're doing five percent on a question and you miss it out and you lose the five percent just for doing one small thing, sort of where you'd get maybe half the marks in a normal paper if you'd done something silly like that there, whilst on this one here, it was a zero. (Student 4)
Higher Standard of Work. While there was frustration at the grading system there was also an understanding of why the course was designed this way. Student 1 for example points out that FAC is a preparatory course for university-level maths modules and therefore it is reasonable to expect students to be fluent in the content.
The grading system, the 80% pass mark is obviously a lot higher than… university exams, or the majority of them anyway. And I kind of get why that is, because the content isn't quite university level, or, and it's a little bit easier, and they kind of want to push you to ace that work so that you can go on to do the university stuff. (Student 1)
Similarly Student 8 felt that much of the content was revising things that had been covered in school and therefore ‘it made sense that they want you to be like really good at it’.
Students also commented that the higher than normal pass mark changed the way in which they approached the course, encouraging them to ‘work a bit harder for them’, ‘make sure to do every question like really carefully’, to ‘kind of dig deep’ and to ‘really think about what I was putting in’.
Some students commented that this approach resulted in a greater depth of knowledge
[It] meant that I did understand things a lot more. It forced me to spend more time to actually understand things. (Student 12)
4.2.4. Working with others
Students were split between those who preferred to work alone ‘So me personally, I work by myself’ and those who commented on the lack of opportunities for interaction as part of the course:
there wasn't much opportunity to meet other people on the course really, (Student 2)
there was no contact time, so it just felt like it was me and the computer, (Student 3)
sometimes I was sitting there for four weeks thinking I'm sitting on a laptop doing this here, there's not much contact with anything. (Student 4)
A few students felt that the nature of the course didn't lend itself to working with other people in a formal way:
It's fundamentally something which, you know, meeting up with people and talking about it like that can't really help. (Student 9)
Furthermore, Student 1 pointed out that the STACK questions had randomly generated numbers, so the questions looked different for each student (though the principle of solving the question didn't change).
…whereas with FAC, all the questions are different for everyone. They're the same question, but the numbers are all changed, so that made it kind of difficult to work with other people on it, because nobody had the same question really (Student 1)
and some found studying in formal groups to be difficult:
like mandatory group study can be kind of daunting to them cause it's like, ‘oh I'd rather just study on my own’. For me anyway, that's how I prefer to approach it most of the time. (Student 5)
Although students were encouraged to form autonomous learning groups, in most cases interviewees said that these ceased meeting after the first few weeks. When students did work together it tended to be through informal interactions with people that they had met on the course ‘I've got two friends… who are on the course. I speak to them a lot’, during one of the face-to-face introductory lectures, or with friends from other courses who had done Advanced Higher Mathematics or equivalent ‘I am friends with physicists, so they were all pretty good at maths at the time’, or in some cases friends from school who were also now at the University.
When students did interact with each other, they often did so using technology, rather than meeting face-to-face. For example Student 3 commented that ‘That was something that happened a lot, everyone would text each other to say, oh, have you managed to do this and solve this question’.
Other students sent images of their working to friends who would add feedback and send them back:
Like sometimes I'd like take a picture of a question, send it to my friend and ask her how she would do it. And then she'd send me back a picture of her working and the answer she got. (Student 12)
4.2.5. Advantages of online study
While some students missed interaction with others they were also positive about some of the benefits that were brought about by the course being online. For example, students appreciated the time saving that came from not needing to travel to campus for lectures and tutorials:
I felt like with FAC it was easier to fit in those hours because I didn't have to, kind of, factor in me getting to uni, me getting back from uni and the time that took, if I was gonna do work, I could just sit down and do the work, you know, rather than having to travel and things like that and that being a factor. (Student 1)
Students also liked the flexibility that they had over both the time and place of study. Students could access the course wherever they liked: ‘Well, you can do it when you're in bed!’, ‘I find myself going to like a coffee shop or something, doing it there’, and also whenever they liked. This meant that it could be fitted in around other commitments such as lectures.
the fact that it was very independent was helpful because it means I could do it in times that suited me around the other lectures which was nice. (Student 5)
It's really… useful for me especially because I never know where I'll be cause I tend to be going back and forth from a lot of places. (Student 6)
Students also commented on the way that this flexibility gave them the ability to take control of their learning, enabling them as Student 4 pointed out to study when they could be most productive:
I felt that I learnt better as well because in lectures you're sitting there concentrating for an hour, whilst in this course here, I could do 15 minutes work, grab a coffee, go outside, do another half an hour, do something else. Concentrating for an hour long and having someone speak at you the whole time doesn't help me very much most of the time. (Student 4)
Students also noted there was a particular benefit for those with chronic (and acute) illnesses.
I've been like ill a lot recently as well so it's made it a lot easier for me to be online because it means that I don't have to like be able to actually physically make it to uni. (Student 10)
Student 1 also commented that while lecture captures can help students with illnesses keep up with the material, a course that is fully online like FAC gives everyone an equal opportunity of engagement.
So I really liked the online thing, and … especially for students with chronic illness. … I know lectures are recorded, but there is quite a pressure to actually attend, and there's always all this research about how students that actually attend lectures do better than those that don't attend, so the fact that it was all online and that everyone was doing it online was really nice for me because it was very flexible for my lifestyle, and things like that. (Student 1)
4.2.6. Persistence
Some aspects of the design of the course seemed to encourage students to keep trying to solve the questions even when they got stuck – i.e. to develop persistence.
At a general level the weekly tests kept students working on the course and made sure they ‘kept on top of it’.
The 80% pass mark, discussed in more detail above, also resulted in students putting more effort into the tests, for example by checking their working thoroughly.
The lack of opportunities for interaction with other students was commented on by a number of participants. One disadvantage of this was that ‘there was no fast-track option of having it explained to you’, however while this may seem to be a negative aspect it also, as Student 11 commented ‘does sometimes encourage you to go and try and work it out yourself’. Student 7 noted that this approach to maths in particular is likely to be beneficial for understanding:
But in maths, it feels like I'm more, like, I'm untangling a knot and then kind of getting at something, and so someone telling me the answer, it's hard to kind of draw anything from that in the maths sense. (Student 7)
Student 12 agreed with this positive impact of having no one to ask for help and described how this experience in FAC encouraged him to become more persistent during the course.
perseverance definitely changed because I was thinking that beforehand if I couldn't solve a question I would maybe try it a few times and then normally I'd just ask for help. But then FAC, I couldn't really go for help, I just had to stick it through and just keep bashing out question after question after question until I understood it. And then I did finally click. So then I actually saw that if I actually did put the work in, it would eventually click. (Student 12)
Other students demonstrated persistence and also their deep learning approach by denying themselves access to resources that would make it easier for them to solve the questions, such as their own notes, or information online.
I: So did you try and do it without looking up things first?
S: Of course, yes, just to try and get it, and then if I was really struggling or I couldn't do it, I would look it up. I think… it's not trying to pass exams, it's trying to learn the knowledge for future things. (Student 3)
4.2.7. Confidence
There is evidence that FAC improved students' confidence in their maths ability:
It just helped me to like feel a bit more confident with the whole thing in general cause I wasn't quite sure about my maths ability. (Student 8)
I think it's especially good for students that have any sort of kind of doubt about their ability. So if anyone's a bit worried about how difficult the work's going to be, or if everyone's going to be better than them, or if they're not quite, their understanding isn't quite as good as they'd like it to be, and things like that, it's essentially the perfect course (Student 1)
and helped them with other university-level maths courses:
Well I'm already seeing results with it to be honest because I was reading the ILA textbook for like the reading quiz… actually understanding the language through using like saying parallel vectors and stuff like that because I've already spent ages like actually studying it and doing examples on it. As soon as it mentions all the stuff I can immediately visualize or like understand what it's saying. So it makes actually reading the language or reading like actual textbook definitions much easier (Focus Group Student 3)
4.3. Discussion
The interview data indicates that students tended to find the course beneficial, but also highlights aspects of the design that caused some difficulties.
The demands of the specifications grading scheme and perceived high workload were a source of frustration for some students, but also a prompt to improve study habits. This frustration led to some evidence of ‘gaming the system’, whereby students exploited the online course delivery and automated assessment to work through the materials only highly selectively, and access worked solutions to questions without first attempting them. On the other hand, the reasons for the high standards imposed seemed to be understood, and these high standards acted as a driver to encourage students to work with persistence and care.
Students appreciated the flexibility of studying online, allowing them to study at times and places of their choice. This was mentioned of being of particular benefit to students with personal situations (e.g. illness) that would otherwise limit their ability to engage with their studies. The online delivery was, however, seen to promote feelings of isolation. While some students reported working successfully with other students both face-to-face and using technology, the limited uptake for the autonomous learning groups shows that a challenge remains in convincing students of the value of peer interactions and setting up a structure which encourages this.
These interviews provided rich data about students' experience of the course, which has helped to shape future iterations of the course; we return to this in the next section. In future work we will be analyzing responses to the MAPS survey (Code et al., Citation2016) across two cohorts of students, to give a broader view on the possible effect of FAC on students' attitudes.
5. Conclusion
We have described the design of an online course in introductory mathematics, that puts into practice various ideas from education research. Our evaluation of the course has found evidence of a positive impact on students – students who took the course achieved a mean gain of 13.7 percentage points (95% HDI ) on a test of high-school mathematics between the start of Semester 1 and the first week of Semester 2. In the sample of students who took part in interviews, most found the course beneficial and would recommend it to others.
The design that we described in Section 2 was devised for the relatively procedural focus in recapping high school mathematics, but it remains to be seen if this could extend to more mainstream university mathematics courses. There is already some indication that aspects of the design could be used more widely – for instance, the use of specifications grading in an ‘introduction to proofs’ course (Williams, Citation2018) – but further work is needed to support extensive use of computer-aided assessment for more advanced topics (Kinnear et al., Citation2020). For instance, the existing capabilities of computer-aided assessment mean that skills such as curve sketching or writing proofs can only be assessed indirectly (e.g. Bickerton & Sangwin, Citation2021).
Our interviews with students also highlighted some concerns that could be addressed in the design, and we highlight three aspects in particular. First, students perceived the course to have a high workload – but this may be largely a matter of perception that could be mitigated by managing expectations (Kember, Citation2004). In optional feedback surveys at the end of each weekly unit, students reported spending a mean of 10.7 hours per week working on the course (CitationSangwin & Kinnear, Citationin press), which if anything is slightly less than would be expected for a 20-credit course. Still, some students reported working substantially longer than this, and proactive monitoring of students who may be struggling is an important reason why online courses such as FAC need proper ongoing oversight from academic staff (CitationSangwin & Kinnear, Citationin press). In the most recent iteration of the course, student feedback did not highlight workload as a particular concern. This was perhaps due to stronger messaging upfront about the expected workload: for instance, we added an orientation quiz to the start of the course, which explicitly asks students to enter their plan for a weekly schedule of work on the course.
Second, students reported frustration at losing marks over small mistakes, particularly when they felt traditional paper-based marking would have likely given them partial marks. We have been able to address this for several of the STACK questions by implementing checks for ‘errors carried forward’ and awarding partial marks for these; this is an ongoing process where we are able to refine the course materials each year. We also added another opportunity to retake unit tests at the end of semester (so students have up to 3 attempts to achieve a Mastery result) to relieve some of the stress about high stakes.
Third, the theme of ‘gaming the system’ emerged in the interviews, where students would focus their efforts squarely on passing the unit tests. The Moodle system collects logs of all user actions, and some exploratory analysis of these does confirm that some students skip straight to the end-of-unit assessments each week. As noted by some students in the interviews, this could be because they felt they were already sufficiently familiar with a particular topic, which could be an example of appropriate self-regulation (Entwistle & McCune, Citation2004). In future work, it would be possible to analyze student study patterns in more detail (cf. Chen et al., Citation2018). This could include exploring patterns of usage that may indicate lapses in academic integrity (Seaton, Citation2019), though the interviews and our informal contact with students do not give us serious concerns about this. More importantly, it would be interesting to identify if there are certain student engagement patterns that are associated with future success, as these may inform advice to students about effective study strategies.
In conclusion, we have demonstrated an approach to delivering an online mathematics course that integrates a range of ideas from education research. Our model has already helped to inform the design of a new online course at our own institution. With the aftermath of Covid-19 likely to see increased use of online teaching, we hope the model will be useful more widely.
Acknowledgements
The authors are grateful to Mine Çetinkaya-Rundel and two anonymous reviewers who provided helpful comments on earlier versions of the paper.
Disclosure statement
No potential conflict of interest was reported by the authors.
Additional information
Funding
Notes
1 See http://www.drps.ed.ac.uk/20-21/dpt/utmathb.htm for full details
2 This was offered at multiple times to avoid timetable clashes. Meetings took place in a ‘teaching studio’ (a large room with several tables set up for group work), except in 2020/21 when they ran over Zoom.
3 Students from a variety of other programmes such as architecture and economics enrolled on FAC as an outside option, without also enrolling on ILA
4 The linear regression with the strongest support was , i.e. with no interaction term and with no reference to the cohort.
5 The best model was , reflecting the fact that the mean course results varied from year to year (see histogram in the Appendix).
6 Note that at the time of writing, results for CAP in 2020/21 were not yet available
References
- Alcock, L., Brown, G., & Dunning, C. (2015). Independent study workbooks for proofs in group theory. International Journal of Research in Undergraduate Mathematics Education, 1(1), 3–26. https://doi.org/https://doi.org/10.1007/s40753-015-0009-7
- Allen, M., Poggiali, D., Whitaker, K., Marshall, T. R., & Kievit, R. A. (2019). Raincloud plots: A multi-platform tool for robust data visualization [version 1; peer review: 2 approved]. Wellcome Open Research, 4(63). https://doi.org/10.12688/wellcomeopenres.15191.1
- Bickerton, R. T., & Sangwin, C. J. (2021). Practical online assessment of mathematical proof. International Journal of Mathematical Education in Science and Technology. https://doi.org/https://doi.org/10.1080/0020739X.2021.1896813
- Brame, C. J., & Biel, R. (2015). Test-enhanced learning: The potential for testing to promote greater learning in undergraduate science courses. CBE Life Sciences Education, 14(2), 14:es4. https://doi.org/https://doi.org/10.1187/cbe.14-11-0208
- Braun, V., & Clarke, V. (2006). Using thematic analysis in psychology. Qualitative Research in Psychology, 3(2), 77–101. https://doi.org/https://doi.org/10.1191/1478088706qp063oa
- Carlson, M., Oehrtman, M., & Engelke, N. (2010). The precalculus concept assessment: A tool for assessing students' reasoning abilities and understandings. Cognition and Instruction, 28(2), 113–145. https://doi.org/https://doi.org/10.1080/07370001003676587
- Cepeda, N. J., Vul, E., Rohrer, D., Wixted, J. T., & Pashler, H. (2008). Spacing effects in learning a temporal ridgeline of optimal retention. Psychological Science, 19(11), 1095–1102. https://doi.org/https://doi.org/10.1111/j.1467-9280.2008.02209.x
- Chen, X., Breslow, L., & DeBoer, J. (2018). Analyzing productive learning behaviors for students using immediate corrective feedback in a blended learning environment. Computers and Education, 117(2), 59–74. https://doi.org/https://doi.org/10.1016/j.compedu.2017.09.013
- Chongchitnan, S. (2019). Electronic preparatory test for mathematics undergraduates: Implementation, results and correlations. MSOR Connections, 17(3), 5–13. https://doi.org/https://doi.org/10.21100/msor.v17i3
- Code, W., Merchant, S., Maciejewski, W., Thomas, M., & Lo, J. (2016). The mathematics attitudes and perceptions survey: An instrument to assess expert-like views and dispositions among undergraduate mathematics students. International Journal of Mathematical Education in Science and Technology, 47(6), 917–937. https://doi.org/https://doi.org/10.1080/0020739X.2015.1133854
- Croxford, L., Docherty, G., Gaukroger, R., & Hood, K. (2014). Widening participation at the university of Edinburgh: Contextual admissions, retention, and degree outcomes. Scottish Affairs, 23(2), 192–216. https://doi.org/https://doi.org/10.3366/scot.2014.0017
- Engelbrecht, J., & Harding, A. (2005). Teaching undergraduate mathematics on the internet. Educational Studies in Mathematics, 58(2), 253–276. https://doi.org/https://doi.org/10.1007/s10649-005-6457-2
- Entwistle, N., & McCune, V. (2004). The conceptual bases of study strategy inventories. Educational Psychology Review, 16(4), 325–345. https://doi.org/https://doi.org/10.1007/s10648-004-0003-0
- Freeman, S., Eddy, S. L., McDonough, M., Smith, M. K., Okoroafor, N., Jordt, H., & Wenderoth, M. P. (2014). Active learning increases student performance in science, engineering, and mathematics. Proceedings of the National Academy of Sciences of the United States of America, 111(23), 8410–8415. https://doi.org/https://doi.org/10.1073/pnas.1319030111
- Goldenberg, P., & Mason, J. (2008). Shedding light on and with example spaces. Educational Studies in Mathematics, 69(2), 183–194. https://doi.org/https://doi.org/10.1007/s10649-008-9143-3
- Goodrich, B., Gabry, J., Ali, I., & Brilleman, S. (2020). Rstanarm: Bayesian applied regression modeling via Stan. [R package version 2.21.1]. https://mc-stan.org/rstanarm.
- Gopalan, M., Rosinger, K., & Ahn, J. B. (2020). Use of quasi-experimental research designs in education research: Growth, promise, and challenges. Review of Research in Education, 44(1), 218–243. https://doi.org/https://doi.org/10.3102/0091732X20903302
- Hopkins, R. F., Lyle, K. B., Hieb, J. L., & Ralston, P. A. (2016). Spaced retrieval practice increases college students' short- and long-term retention of mathematics knowledge. Educational Psychology Review, 28(4), 853–873. https://doi.org/https://doi.org/10.1007/s10648-015-9349-8
- Kember, D. (2004). Interpreting student workload and the factors which shape students' perceptions of their workload. Studies in Higher Education, 29(2), 165–184. https://doi.org/https://doi.org/10.1080/0307507042000190778
- Kinnear, G. (2018). Improving an online diagnostic test via item analysis. Proceedings of the Fifth ERME Topic Conference on Mathematics Education in the Digital Age (pp. 315–316). University of Copenhagen. https://www.math.ku.dk/english/research/conferences/2018/meda/proceedings/MEDA_2018_Proceedings.pdf.
- Kinnear, G. (2019). Delivering an online course using STACK. Contributions to the 1st international STACK conference 2018. Friedrich-Alexander-Universität Erlangen-Nürnberg. https://doi.org/https://doi.org/10.5281/zenodo.2565969.
- Kinnear, G., Jones, I., & Sangwin, C. (2020). Towards a shared research agenda for computer-aided assessment of university mathematics. In A. Donevska-Todorova, E. Faggiano, J. Trgalova, Z. Lavicza, R. Weinhandl, A. Clark-Wilson, & H.-G. Weigand (Eds.), Mathematics education in the digital age (MEDA) proceedings. Johannes Kepler University. https://hal.archives-ouvertes.fr/hal-02932218.
- Kruschke, J. K. (2018). Rejecting or accepting parameter values in Bayesian estimation. Advances in Methods and Practices in Psychological Science, 1(2), 270–280. https://doi.org/https://doi.org/10.1177/2515245918771304
- Maciejewski, W. (2015). Flipping the calculus classroom: An evaluative study. Teaching Mathematics and its Applications, 19(4), hrv019. https://doi.org/https://doi.org/10.1093/teamat/hrv019
- Martínez-Sierra, G., & García González, M. D. S. (2014). High school students' emotional experiences in mathematics classes. Research in Mathematics Education, 16(3), 234–250. https://doi.org/https://doi.org/10.1080/14794802.2014.895676
- McGuffey, W., Quea, R., Weber, K., Wasserman, N., Fukawa-Connelly, T., & Mejia Ramos, J. P. (2019). Pre- and in-service teachers' perceived value of an experimental real analysis course for teachers. International Journal of Mathematical Education in Science and Technology, 50(8), 1166–1190. https://doi.org/https://doi.org/10.1080/0020739X.2019.1587021
- Nilson, L. B. (2014). Specifications grading: Restoring rigor, motivating students, and saving faculty time. Stylus Publishing.
- Pampaka, M., Williams, J., & Hutcheson, G. (2012). Measuring students' transition into university and its association with learning outcomes. British Educational Research Journal, 38(6), 1041–1071. https://doi.org/https://doi.org/10.1080/01411926.2011.613453
- Rach, S., & Ufer, S. (2020). Which prior mathematical knowledge is necessary for study success in the university study entrance phase? Results on a new model of knowledge levels based on a reanalysis of data from existing studies. International Journal of Research in Undergraduate Mathematics Education, 6(3), 375–403. https://doi.org/https://doi.org/10.1007/s40753-020-00112-x
- Renkl, A., Atkinson, R. K., Maier, U. H., & Staley, R. (2002). From example study to problem solving: Smooth transitions help learning. The Journal of Experimental Education, 70(4), 293–315. https://doi.org/https://doi.org/10.1080/00220970209599510
- Roediger, H. L., & Butler, A. C. (2011). The critical role of retrieval practice in long-term retention. Trends in Cognitive Sciences, 15(1), 20–27. https://doi.org/https://doi.org/10.1016/j.tics.2010.09.003
- Roediger, H. L., & Karpicke, J. D. (2006). The power of testing memory: Basic research and implications for educational practice. Perspectives on Psychological Science, 1(3), 181–210. https://doi.org/https://doi.org/10.1111/j.1745-6916.2006.00012.x
- Sangwin, C. J. (2013). Computer aided assessment of mathematics. Oxford University Press. https://doi.org/https://doi.org/10.1093/acprof:oso/9780199660353.003.0003
- Sangwin, C. J., & Kinnear, G. (in press). Coherently organised digital exercises and expositions. PRIMUS.
- Seaton, K. A. (2019). Laying groundwork for an understanding of academic integrity in mathematics tasks. International Journal of Mathematical Education in Science and Technology, 50(7), 1063–1072. https://doi.org/https://doi.org/10.1080/0020739X.2019.1640399
- Sofronas, K. S., DeFranco, T. C., Vinsonhaler, C., Gorgievski, N., Schroeder, L., & Hamelin, C. (2011). What does it mean for a student to understand the first-year calculus? Perspectives of 24 experts. Journal of Mathematical Behavior, 30(2), 131–148. https://doi.org/https://doi.org/10.1016/j.jmathb.2011.02.001
- Uner, O., & Roediger, H. L. (2018). The effect of question placement on learning from textbook chapters. Journal of Applied Research in Memory and Cognition, 7(1), 116–122. https://doi.org/https://doi.org/10.1016/j.jarmac.2017.09.002
- Williams, J. (2015). Mathematics education and the transition to higher education: Transmaths demands better learning-teaching dialogue. In M. Grove, T. Croft, J. Kyle & D. Lawson (Eds.), Transitions in undergraduate mathematics education (pp. 25–37). The Higher Education Academy, University of Birmingham.
- Williams, K. (2018). Specifications-based grading in an introduction to proofs course. Primus, 28(2), 128–142. https://doi.org/https://doi.org/10.1080/10511970.2017.1344337