Abstract
Production planning of final assembly systems is a challenging task, as the often fluctuating order volumes require flexible solutions. Besides, the calculated plans need to be robust against the process-level disturbances and stochastic nature of some parameters like manual processing times or machine availability. In the paper, a simulation-based optimisation method is proposed that utilises lower level shop floor data to calculate robust production plans for final assembly lines of a flexible, multi-stage production system. In order to minimise the idle times when executing the plans, the capacity control that specifies the proper operator–task assignments is also determined. The analysed multi-stage system is operated with a pull strategy, which means that the production at the final assembly lines generates demands for the preceding stages providing the assembled components. In order to guarantee the feasibility of the plans calculated for the final assembly lines, a decomposition approach is proposed to optimise the production plan of preceding stages. By this way, the robust production can be ensured resulting in reduced losses and overall production costs even though the system is exposed to changes and disturbances.
1. Introduction
Flexible systems are often applied by companies to remain competitive on the market characterised by often changing customer needs regarding both volume and variety. Flexible manufacturing and assembly systems exist for decades, however, they are still one of the most fundamental solutions to efficiently react on internal and external changes and disturbances (ElMaraghy et al. , Citation2013). Assembly lines are often the last stages of the process chain to finish the products, however, it is of crucial importance to manage properly the preceding stages of process chain as well, in order to keep the due dates and the customer-expected service level.
In the paper, a novel planning and control methodology is introduced for multi-stage production systems. As a demonstrator of the method, a real system is selected which consists of a flexible machinery to produce the components that are used in the final assembly lines to assemble the end products delivered to the customers. Between these two main stages (i.e., the final assembly and the preceding steps), an inventory is applied to balance the production, and maintain its continuousness even though different lot sizes and control methods are applied. The main objective of the method is to calculate robust production plans for the multi-stage production system in consideration, in order to ensure the smoothness of the production in the face of disturbances and maintain the target level of the performance indicators, even in a volatile environment.
1.1. Robust production planning
In general, the production planning layer of the well-known supply chain planning matrix is responsible for transforming customer orders into production orders by solving lot-sizing problems that match the order stream with available capacities, resulting in a production plan (Meyr, Wagner, and Rohde , Citation2015). Production plans relying on deterministic parameters often fail to cope with the dynamic effects of the execution environment and the considerable uncertainty of the underlying planning information. In order to prevent the losses caused by the optimistic planning with idealistic parameters, robust techniques are required, which provide feasible production plans. Robustness in production planning involves refined approaches that aim at handling predictable or unpredictable changes and disturbances. They respond to the occurrence of random events (reactive approaches) or protect the performance of the plan by anticipating to a certain degree the occurrence of uncertain events (proactive approaches) (Tolio, Urgo, and Váncza Citation2011; Herroelen and Leus Citation2004). In our terminology, robust planning is the logical layer of robust production. A production plan is called robust if it results in an acceptable level of the selected performance indicators even if unpredictable disruptions occur during the execution of the plan. Efficient ways of taking uncertainties into account, and to achieve more robust solutions are either applying stochastic models (Sahinidis Citation2004; Naeem et al. Citation2013) (e.g., by estimating the underlying stochastic processes), or using adaptive and cooperative approaches which allows prompt responses to changes and disturbances (Monostori et al. Citation2010).
As deterministic models usually fail to provide executable plans due to the existence of uncertain and stochastic parameters (e.g. machine breakdowns, reject/scrap rates or manual processing times), simulation-based optimisation (also referred as simulation optimisation) methods are often applied to calculate robust plans. In general, they consist of a mathematical optimisation model, in which the objective function or constraint(s) are represented by functions that are approximated using simulation results (Azadivar Citation1999). The reason for applying simulation in these cases is the computational complexity or the lack of analytical form of the objective function and/or constraints. In production planning, simulation-based optimisation is mostly applied by iteratively adjusting parameter values according to the results of simulation experiments, until the target values of the performance indicators are reached (Byrne and Hossain Citation2005; Laroque et al. Citation2012; Melouk et al. Citation2013; Irdem et al. Citation2010; Gansterer, Almeder, and Hartl Citation2014).
Several key performance indicators (KPI) are applied to measure the robustness of a production plan (Naeem et al. Citation2013; Aytug et al. Citation2005), however, total backlog (or the related service level) and lateness are used in most of the cases (Stevenson, Hendry, and Kingsman Citation2005). Lödding (Citation2012) defines backlog as the difference of the planned and actual outputs of the production, whereas lateness is a time–dimension metric measuring the difference between the actual and planned completion of production orders. Lateness is an execution related KPI, which is basically caused by the disturbances if the plan is not robust enough, accordingly, it characterises robustness more efficiently as it strongly relies on the execution of the plan. The robustness of a plan often works against other efficiency criteria, hence, it means a trade-off is required if the objective is to increase robustness. The cost of robustness can have different forms, a simple example might be the cost of additional capacities that need to be allocated for the same amount of work (Zanjani, Masoumeh, and Ait-Kadi Citation2010). In the paper, cost of robustness is measured with the difference of the total production costs that incur when executing a robust and non-robust plan.
1.2. Production planning in multi-stage systems
Considering deterministic, multi-stage production planning models, several efficient approaches exist to solve even complex problem instances. The analysed problem is usually formalised as a multi-level (often referred as multi-stage) capacitated lot-sizing problem (MLCLSP), aimed at minimising the overall production costs involving setup and inventory costs. In most of the cases, so-called echelon-stocks are introduced in the model, representing the stock of components that are produced in different stages of the process chain (Pochet and Wolsey Citation2006). In general, MLCLSP is formulated as a single optimisation problem that determines the optimal amount of components to be produced in different time periods. Due to the highly complex nature of the problem, existing approaches are either seeking to implement efficient heuristics, or to decompose the problem and solve the resultant single-level subproblems sequentially.
As for the heuristics-based approaches, Sahling et al. (Citation2009) propose a new algorithm to solve the MLCLSP as a big bucket problem, allowing to produce any number of products within a period, however, partial sequencing of the orders is solved by determining the release of the first and last orders in each period. Helber and Sahling (Citation2010) apply the same fix-and-optimise heuristics as it provides a flexible and most efficient known solution for the MLCLSP. The approach can manage general product structures and consider the lead times of products, nonetheless deviations and uncertainties of the parameters cannot be treated. Similarly, the aggregate production planning problem of a two-stage system is solved by Ramezanian, Rahmani, and Barzinpour (Citation2012), applying genetic algorithm and Tabu search. In contrast to heuristics-based approaches, decomposition-based solutions apply echelon-stock variables, simplifying the original multi-level problem to a series of single-item lot-sizing subproblems (Pochet and Wolsey Citation2006). It requires to run multiple planning models to solve the multi-level problem, however, these single-stage models take significantly less computational efforts to be solved.
1.3. Towards robust, multi-level planning in practice
Although both multi-stage and robust planning have extensive literature, only a few solutions exist to solve the combined problem of them. Aghezzaf, Sitompul, and Van den Broecke (Citation2011) propose an inventory decomposition-based approach to solve the robust, multi-level planning problem. Alem and Morabito (Citation2012) apply robust optimisation to solve a multi-stage planning problem from a furniture industry, whereas Schemeleva et al. (Citation2012) propose a memetic algorithm to solve a similar problem. Kozłowski et al. (Citation2014) introduce a predictive approach for multi-stage systems with stochastic parameters, however, this solution is more suitable for long-term planning with large order quantities. Zanjani, Masoumeh, and Ait-Kadi (Citation2010) apply an inventory-based decomposition for production planning in a manufacturing environment with random yield. The approach results in robust solutions, yet it considers aggregate and constant capacities, which is not suitable in case of assembly processes with stochastic processing times and flexible capacities.
The efficiency of the above approaches are proven, however, from practical point of view, most lot-sizing approaches are not suitable for everyday use due to the ‘hard-wired’ procedural heuristics that follow highly specific problem logics (Helber and Sahling Citation2010). Production planning of multi-stage systems is a major step of material requirement planning systems, most of which ignore capacity constraints and disregard setup, production and inventory costs when deciding about lot sizes (Berretta, França, and Armentano Citation2005). These characteristics often leads to capacity infeasible plans in industrial practice. Almeder et al. (Citation2015) tackle this issue by solving the MLCLSP in two steps: batching and lot-streaming. Albeit enterprise resource planning (ERP) systems are significantly improved in the integration of material and capacity planning (Hvolby and Steger-Jensen Citation2010), they are still unable to perform satisfactory in a dynamic, uncertain environment (Tenhiälä and Helkiö Citation2015). To tackle these challenges more efficiently, advanced planning and scheduling systems (APS) combine production planning and scheduling and utilise ERP data to adjust the plans to the actual status of the production system (Fleischmann, Meyr, and Wagner Citation2005). Most APS apply what-if analysis to determine the quality of the plan before releasing it to the shop floor, and this analysis is often performed by simulation considering the latest shop floor data (Krenczyk and Jagodzinski. Citation2015; Ko, Kim, and Yoo Citation2013). These approaches enable to evaluate the production schedules in a proactive way, and adjust them to the actual status of the physical system. Even though these methods offer efficient solutions to calculate feasible production plans, they do not consider the underlying production information that can be gathered from the shop floor, but only use higher level planning data such as cycle times or expected lead times.
In the paper, a novel planning method is introduced that can be used in the planning and scheduling layer of an APS, and the essence of the method is given in the combination of shop floor data from the manufacturing execution (MES) and the ERP systems, facilitating to calculate robust production plans. The method combines data analysis techniques and discrete-event simulation (DES) in the mathematical model of production planning and scheduling. Similar to Kibira et al. (Citation2015), the proposed method is aimed at utilising sensor-level data in production planning in a proactive way, however, the objective in our methodology is to decrease the overall production costs while being robust against the disturbances that might worsen the performance of the plan. In contrast to most, iterative simulation-based optimisation techniques, our method relies on linear regression models, thus requires less computation efforts. Although linear models may seem overly simplistic, they represent properly practical problems and can outperform more sophisticated models in running time or robustness of the solution. Compared to the previous robust optimisation and iterative simulation-based techniques, our method results in less lateness for lower costs (cost of robustness), while keeping the simplicity and thus short running time of the planning algorithms, enabling to apply it in real industrial environment.
2. Problem statement
As introduced earlier, the analysed production system is composed of more stages: the final products are assembled on flexible flow lines that are designed for producing different product variants in batches, while the main components are machined in a preceding machinery segment. Multi-stage production systems require special production planning approaches to balance and coordinate the production along the entire process chain. In the analysed case, precise production planning is important to minimise the changeovers required to setup the line from one product variant to another, besides, capacity control is responsible for allocating the proper amount of human workforce to the process, to keep the customer due dates without lateness. The above characteristics results in a special version of the MLCLSP, in which a complementary problem of the human capacity control also need to be solved, meanwhile, the solution of this subproblem is utilised when planning the production.
The primary focus of the paper is the production planning of the assembly lines, seeking cost-optimal plans that determine the lot sizes, release dates as well as the capacity requirements. In order to handle the changes and disturbances in a robust way, the proposed planning method is combined with a lower level capacity control, specifying the work hours and when and to which workstations human resources are allocated (Lödding Citation2012). While the objective of planning is to decrease costs by eliminating the unnecessary changeovers and decreasing the stock levels, the capacity control is responsible for balancing the workload of the operators and eliminating the idle times.
The overall objective is to calculate near-optimal, robust plans for the final assembly stage that pulls the production of the previous stages. As the customer service level of the company is mostly influenced by the final products, the resulted plans need to be robust against the assembly-related changes and disturbances (e.g. machine breakdowns or process time deviations) that have a negative impact on the service level. In order to maintain this performance indicator on a desired level, a decomposition approach is proposed that splits the multi-stage production planning problem into two main subproblems: the combined production planning and capacity control of the final assembly lines and the production planning of the previous stages. In order to meet the quantity and due date requirements of the customers, the problem of the assembly lines is solved first, as the pull strategy directly generates demands and thus constraints in the production planning problem of the pre-inventory stages. In this way, the integrity of the production plan along the entire process chain can be guaranteed.
2.1. Characteristics of the considered production environment
In order to define the planning problem precisely, the characteristics of the production system are introduced as they follow. The first stage of the system is a machinery, where different modules are manufactured, providing the main components of the final products assembled in the last stage. Between the assembly lines and the machinery, an in-process inventory is found that splits the process chain into two main parts: the pre-inventory processes and the final assembly (Figure ).
2.1.1. Pre-inventory processes
In the machinery, modules are manufactured on flexible resources, and a single machine is enough to complete all machining processes of a given module. Although the machines are automated, the material handling and setup processes require human labour that is provided by assigning the operators to machines with different control modes. These control modes determine the machine–operator assignments, and they are adjusted according to the production volumes.
The machined parts are transferred to shared resources, where the process times are workload-independent but product-specific, therefore this stage is characterised with the lead time of a single product from the machinery to the in-process inventory. Holding this inventory is necessary to balance the different economic lot sizes in the machinery and assembly segments, as in general, bigger lots are preferred in the machinery due to the significantly longer setups than that of the assembly lines.
2.1.2. Final assembly lines
The final assembly line is the last stage of the process chain where final products are assembled from the previously machined base modules and additional components coming from external suppliers. The products are assembled on flexible flow lines, which means that they are capable of producing a set of different product types in separate batches. Similar to the machinery, setups take place when changing from one product type to another; however, these setups are significantly shorter than those of the machinery. The lines have a general structure, consisting of manually operated workstations, an automated test machine and a manual rework station. Each product has to pass a functional test, and products failing the test are transferred to the rework station for correction, then they are retested. The ratio of total retested parts and the total assembled volumes is the reject rate that depends on the product type, and means a challenging stochastic factor when balancing the workload and planning the production. In general, there are fewer operators than assembly workstations, therefore, it is a crucial point to find the right balance between human and machine capacities to assemble the target volumes and keep the workload of operators on a desired level.
2.2. Specification of the combined planning and control problem
Having the structure of the process chain characterised, it is possible to formalise the multi-stage production planning and capacity control problem. The notation applied in the latter sections is summarised in Table .
Table 1. The applied notation.
2.2.1. Component supply planning
The pre-inventory processes can be considered as the suppliers of the main components (modules), which are required by the assembly stage to finalise the products. The whole system is operated with a pull strategy, which means that the end product customer orders are pulling the production of the preceding stages. In the planning problem, we consider a discrete time horizon consisting of a set of micro-time periods , and each period
has the same length
. Compared to the planning model of the assembly lines, the resolution of the pre-inventory planning model is higher, as
. The higher resolution enables to simplify the lead times
to be given in micro-periods, without losing the accuracy of the plans. The volume demands determined by the final assembly is available on the whole planning horizon
. The main questions are the production lot sizes
, which specify the volume of module m machined in time
on machine j. Besides, the corresponding control modes
has to be determined that give the assignment of operator o and machine j in time
. The goal is to minimise the overall production costs that consists of the operator and inventory costs. In the problem of the component supply planning, not only the machinery segment, but also shared resources are considered.
2.2.2. Final assembly planning and control
As the final assembly lines have a common general structure, the emerging production planning and capacity control problem is similar to the one that was specified for the pre-inventory processes (Section 2.2.1). In this case, the customer orders directly influence the production plan, as they refer to the end products. The order volumes of the different product variants are available on a certain horizon that is split up into a set of production shifts .
In case of the final assembly, the planner has to decide about the production lot sizes of different product variants , and the corresponding shift plan which specify the headcount of the operators
in each shift t. Each order
is characterised by its volume
and due date
. Make-to-stock option is available in each shift, therefore in case of capacity shortage, orders can be fulfilled from stocks, however, holding inventory is associated with extra costs
. Order fulfilment after the due date is possible (backlogging) but it is penalised with extra costs
. The objective of the planning is to provide an optimal, executable plan that results in minimal the production costs and increased utilisation of the capacities (machines and human operators).
The capacity control of the final assembly specifies the proper assignment of the operators to the assembly tasks, in order to balance their workload and decrease the idle times caused by the product variant-dependent bottleneck and the reject rates. In this subproblem, the objective is to determine the assignment policies for each product variant and each possible operator headcounts (that can be applied to assemble a given product variant). It means that the number of operators can be changed from shift to shift, according to the production rates. However, more production lots are released in one shift requiring different operator–task assignments, while the headcount of operators cannot be changed. In the industrial practice, this problem is solved by defining standard work instructions based on the norm times, however, this approach often tends to be inefficient as the norm times are considered to be deterministic, whereas they have certain deviation in the real life.
3. Production planning methodology with decomposition
In order to solve the complex multi-stage planning and control problem described above, a decomposition approach is proposed. By this way, the complexity of the multi-stage lot-sizing problem can be reduced to feasible single-stage subproblems, while the coherence of the final solution is ensured by linking the models with common constraints. As the customer orders need to be managed in the production planning model of the final assembly lines which pull the production of the preceding stages, the whole problem can be decomposed at the inventory, as it is responsible for balancing the process differences between the assembly and machining. Consequently, the resulted subproblems can be described with two planning models: the production planning (and capacity control) models of the assembly lines and pre-inventory system.
First, the planning problem of the assembly lines needs to be solved, as the resulted plan generates demands for the preceding stages. In case the process chain is virtually cut at the inventory, the schedule of assembly lines gives the volume of base modules that needed to be available in the inventory to assemble the product in time. This inventory level can be applied as a constraint in the production planning model of the pre-inventory stages.
Having the lot sizes determined by the above described way, the corresponding human workforce requirements also need to be specified. In the machinery, it gives the operator–machine assignments, while in the assembly segment it means the in-process capacity control, more specifically the headcount of the operators and the operator–task assignments. In the machinery, the operators need to perform the material handling only, which means the change of the product in the fixtures. This is done in parallel with the machining of other parts, therefore, a single operator is usually assigned to more machines at the same time. In the assembly segment, operators perform the processes themselves, thus, it is a crucial point to assign them the proper workload in order to avoid overload and thus lateness of the plan. Moreover, underestimation of the workload results in idle times and extra costs, which is also avoidable when calculating the capacity control. Therefore, in the machinery, shift planning is done together with the lot-sizing applying the same model, whereas in the assembly the capacity control is decoupled from the production planning model and only the necessary headcount is calculated together with the production plan. The workflow of the planning procedure is depicted by Figure .
4. Robust production planning method for the final assembly lines
4.1. Description of the applied simulation models
The robust planning method of the final assembly lines relies on simulation models, which are used for multiple purposes in the approach: to determine the capacity control of the lines as well as to provide realistic data to build reliable capacity prediction models upon. The simulation model of the lines is built using a common data representation, utilising the generic structure of the lines. Static elements of the simulation model are only the structure of the lines (workstations, layout), and the routing of the products. The essence of the simulation model is a data interface, ensuring that each relevant production parameter is updated before the experiments according to the actual MES data. In this way, processing and testing times, reject rates and machine availability are given as stochastic values, and the parameters of the distribution functions are adjusted by a function that process the latest MES log data. Accordingly, the direct link between the simulation model and the physical system can be always maintained, resulting in reliable results without any direct user interaction (Monostori et al. Citation2010).
4.2. Capacity control of the assembly lines
The capacity control of the assembly lines specifies the assignment of the operators with the assembly tasks. In this case, the general scheme of the assembly lines is applied, in which three main tasks are distinguished: assembly, rework and final assembly. The capacity control takes the operator headcount as an input, and specifies the operator–task assignments, considering that more tasks can be assigned to a single operator. The assignments are many–many type ones, which means that an operator might perform more task and a given task can be assigned to more operators. In order to determine the proper capacity controls for each product and possible headcount, the DES model of the assembly lines are applied. Even though state-of-the-art assembly systems are usually equipped with advanced sensor network, the real workload of the operators is hard to monitor. The DES model of the assembly line can provide reliable results about the workloads, and several various control policies can be evaluated. The main advantage of using simulation for such purposes is its capability to represent the stochastic nature of important processes. In order to select the proper capacity control, several random generated, but possible operator control scenarios are analysed. The main output of the simulation analysis is the utilisation of the operators and the performance of the line. In this way, the proper controls can be selected for each product type and operator headcount.
4.3. Prediction of the capacity requirements with regression models
In flexible, manually operated assembly systems, the prediction of capacity requirements is often complicated, due to the variety of product types and the deviation of processing times. Though, stochastic or robust optimisation models can be applied to cope with non-deterministic parameters, they require high computation efforts and special solver algorithms. Additionally, diverse reject rates of the product variants and the varying rate of rework also increase the complexity of planning models.
In order to tackle these problems, a production planning model is proposed calculating simultaneously the near-optimal production plan and the headcount of human operators, even besides the aforementioned factors. The essence of the method is the introduction of capacity requirements as a general function of the products assembled in the same shift. These functions can be approximated by regression methods, and then integrated directly in the production planning model. In order to approximate the real capacity requirement of a given production lot mix assigned to the same shift, a multivariate linear regression model is proposed. The efficiency of applying regression models for capacity planning in an uncertain environment was shown by the authors in preceding publications (Gyulai et al. Citation2014; Gyulai, Kádár, and Monostori Citation2014). The input variables of the regression are the volumes
assembled in the same period t, and the output is the total manual time that is required to assemble the products. As stated in Section 4.1, the training data-set of the regression models is provided by the simulation model of the assembly line applying MES log data to represent the actual value and distribution of the parameters. The simulation is executed to analyse various possible scenarios, projecting the expected future behaviour of the system from any certain point of time (query time of the log). The applied regression function is defined as follows:
(1)
By this way, the actual capacity requirements (including rework rates, machine downtimes, operator movements and capacity control-related effects) of the batches assembled in the same shift can be estimated. The function can be integrated in the production planning model, which is described in the coming section.
4.4. Simulation-based robust production planning model
The production planning problem is formulated as a mixed-integer programming model including the capacity requirement function , as well as other constraints like the order due dates and inventory holding costs. The decision variables of the model specify the number of allocated operators
for each shift, the number of setups
, the assembled volumes
and the release of the orders
. The model minimises an objective function that is the sum of deviation (early delivery and holding), setup and personnel costs (Equation2
(2) ).
(2)
(3)
(4)
(5)
(6)
(7)
(8)
The constraints include the fulfilment of all customer orders (Equation3(3) ), the calculation of setups (Equation4
(4) ) and volumes (Equation5
(5) ), the capacity restrictions (Equation6
(6) ,Equation7
(7) ) as well as integrity constraints (Equation8
(8) ). Equation (Equation9
(9) ) defines the extra cost of late delivery and inventory holding:
(9)
The resulting production plan gives the required headcount of operators over the horizon and the assignment of customer orders to production shifts. As stated in Section 2.2.1, setup times are significantly shorter than in the machinery and also sequencing within the time period is neglected, resulting in a big bucket lot-sizing model.
5. Pre-inventory production planning
The production plan of the assembly lines specifies the lot sizes and release times, which directly generates demands for modules that are needed to be available in the inventory to execute the plan by assembling the products. This volume is then set as a constraint in the lot-sizing model of the machinery, however, the planning objectives of this lot-sizing is slightly different from the ones of assembly lines. The module inventory is needed to be kept on a minimum level, and the applied human workforce also has to be minimised. The production planning model of the pre-inventory segments is formulated as it follows (mixed-integer programming model).(10)
(11)
(12)
(13)
(14)
(15)
(16)
(17)
(18)
(19)
(20)
(21)
(22)
(23)
The objective is to minimise the total inventory and human labour costs over the planning horizon (Equation10(10) ), while providing enough modules (Equation11
(11) ) for the assembly processes. The balance equation is responsible for linking the consecutive micro-periods through the volume of products in the inventory: the inventory level
in period
equals to the sum of product volume that was available in the inventory in
, the products that arrive in the inventory from the machinery (through the shared resources) minus the products that are used in the assembly segment (Equation12
(12) ). In the machinery, module-dependent setup times are required to switch the machine from one type to another. These setup times are significantly longer than those of the assembly lines, therefore, the setups need to be represented in the model, as they decrease the available capacity. In order to consider the setups, indicator variables
and
are introduced in the model (Equation13
(13) ). In constraint (Equation13
(13) ),
parameter links the integer
and binary
variables: it is an arbitrarily chosen big number and its lower bound is the maximum volume of products that can be produced in period
on a single machine:
. The capacity constraint of the machines specifies that the sum of machine processing times and setups times cannot exceed the length of a micro-period (Equation18
(18) ). The capacity of the human workforce limits the number of products that can be machined (Equation19
(19) )–(Equation22
(22) ). In order to assign the operators to the machines and the machined products, an additional indicator variable
is introduced. Similar to the parameter
,
is an arbitrarily chosen big number linking the binary
and integer
variables; its lower bound is the maximum volume of products that can be produced in period
on a single machine with a single assigned operator:
. Constraint (Equation23
(23) ) represents the assumption that only one module type can be produced on each machine per time period. Additionally, integrity constraints are set on the necessary decision variables (Equation24
(24) ). In contrast to the case of assembly lines, the production planning model of the pre-inventory processes is a single stage, small bucket lot-sizing model specifying the sequence of production lots and the corresponding operator control as well.
6. Numerical results
In order to demonstrate the feasibility and robustness of the proposed production planning method, a real case study from the automotive industry was selected: the company in the case study is a manufacturer of braking systems for commercial vehicles. In the target production system, the pre-inventory processes are responsible for producing the main product modules. First, the steel casts are machined, then deburring and surface treatment processes take place in the shared resources segment. In the machinery, flexible machines are equipped with fixtures that hold several products from the same type, however, setups are required when changing from a certain module type to another, and the setup times are sequence-independent. After the machinery and surface treatment, modules can be either taken directly to the assembly lines, or kept in the inventory. Regarding the assembly segment, several lines are available for assembling the final products, however, they can be planned independently from each other as there are neither material flow nor shared resources among the lines. In the subsequent sections, the steps of the planning workflow are introduced by numerical results.
6.1. Production planning and capacity control of the final assembly lines
6.1.1. Selection of the proper capacity control policies
According to the specified workflow, the first step towards the robust production is to determine the proper capacity control policy for the assembly lines. As stated in Section 2.2.2, the capacity control defines the assignment of the operators to the different tasks based on the assembled product types and the headcount. In order to solve this task, the simulation model of the lines was applied (implemented in Siemens Tecnomatix Plant Simulation), analysing several possible control scenarios. The applied measures were the throughput of the analysed line, and a control policy is considered to be better than another if its throughput is higher. In each simulation run only one product type was analysed by running the experiment on a fixed time horizon. The result of the analysis was a matrix, containing the operator–task assignments with the highest throughput and the least idle times for each p and
.
6.1.2. Prediction of the capacity requirements
The next step of the method is the prediction of the real capacity requirements, as the norm time-based calculations often fail to give reliable results due to the stochastic nature of some parameters (e.g. manual processing times), and the random events like machine breakdowns, or products that fail the functional test. In order to tackle this, multivariate linear regression models were defined for each assembly line, to calculate the overall human workforce required to assemble the given product volumes. The regression models of each assembly line were defined according to (Equation1(1) ), the regression coefficients and model parameters were computed using the R Studio environment and the general linear regression function of R statistical computing language (R Development Core Team , Citation2008), which took less than 1 second to fit the models.
The regression models are built over a data-set, provided by simulation runs as described in section 4.1. As the simulation model applies the latest MES data, it represents precisely the actual physical processes, and can provide an arbitrarily large amount of data (in very short time) by simulating the behaviour of the system in various scenarios. In the test case, the simulation provided production data of 4072 shifts, that was split up into training (1357 shifts) and test (2794 shifts) sets. The input variables were the product types p assembled on a given line and the headcount of the operators . According to the results, the multivariate linear model provides precise prediction for the real capacity requirements, as the coefficient of determination
in each of the cases, and for all p values,
, which indicates that the selected input variables are statistically significant ones (Figure ).
6.1.3. Production and shift planning
The above-described regression model can be applied directly in the production planning model that was implemented and solved in FICO®Xpress, the optimisation algorithms were run until an optimality gap of at most 6% was achievedFootnote1. In case of the assembly lines, robustness of the plan is requested, thus the method was compared to other existing robust planning methods within a benchmark study.
The basis of the benchmark is the deterministic norm time-based planning (NTP), which is applied in most ERP systems. The main difference between NTP and the proposed, simulation- and regression-based robust planning method (RPN) is the calculation of the capacity requirements: while in the RPN, the regression model (Equation1(1) ) is applied in constraint (Equation6
(6) ), the NTP applies norm cycle times to calculate the required human workforce. In NTP, constraint (Equation6
(6) ) has the following form:
(24)
Besides the proposed RPN method, the commonly applied iterative form of simulation-based optimisation (as introduced in section 1.1) was also analysed on the test case, refining iteratively the capacity requirements after each simulation run. Furthermore, the planning task was also formulated as a mixed-integer robust optimisation (RO) problem with uncertainty sets. This modelling technique was introduced by Ben-Tal, El Ghaoui, and Nemirovski (Citation2009), and currently applied in various fields where robust solutions for a problem with uncertain parameters is requested (Bertsimas, Brown, and Caramanis , Citation2011); gabrel2014recent. In RO models, uncertainty of the parameters is given by uncertainty sets that can have different forms (e.g. ellipsoidal). In the benchmark, a robust counterpart called RCT of NTP is applied where the cycle times are represented as uncertain parameters with lower and upper bounds. The last analysed method called RCO is also a robust optimisation model, in which the proposed RPN method is reformulated by adding some uncertainty to the coefficients, as the model fitting in regression always have a certain error. Thus, this method (RCO) can be seen as an extended version of RPN.
In the test cases, a fixed-horizon planning problem for a selected final assembly line was investigated, and solved by all the above-described methods (NTP, RPN, ITR, RCO, RCT). The input parameters of production planning in the benchmark were customer orders concerning nine product types assembled on the selected line. In order to provide a comprehensive study, the methods were analysed by different planning scenarios that included average and also complex problem instances. As for the length of the planning horizon, four different cases were tested: . In each case, problem instances were generated with different amount of orders: normal, high and extreme order scenarios were analysed, in which order due dates were uniformly distributed along the planning horizon. In each category of order scenarios, 10 different instances were generated, and each was solved by all planning methods. Thus, the benchmark included 120 problem instances in total, resulting in 600 solutions given by the 5 different methods.
Table 2. Benchmark of robust production planning methods.
The results of the benchmark are summarised in Table , where each row includes the average results of the 10 problems instances in an order scenario. The main results are the total lateness and the objective function value that gives the total cost of production. The values are given in average percentage: when solving a problem instance with the five different methods, 100% corresponds to the method with most lateness and the highest cost (in case of both lateness and cost the lower values are the better). Besides lateness and cost, the running time of the algorithms is also displayed in seconds. The bracketed superscript values indicate the number of problem instances (out of 10) that a given method could not solve within a time limit of 1800 seconds.
Figure 4. Total lateness (left) and cost (right) results of the benchmark with the five different planning methods.
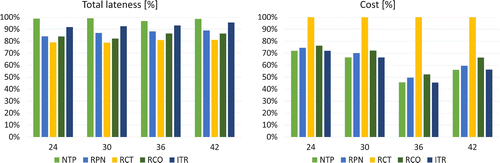
The results show that from robustness point of view, the proposed method (RPN) and its robust counterpart (RCO) always outperform NTP method and the iterative simulation-based planning, only the RCT method could result in lower lateness levels, however, it could not solve most of the instances with high or extreme number of orders. Moreover, it resulted in very high objective function values (cost), in contrast to RPN, which resulted in only slightly higher costs than NTP, thus the cost of robustness in this case is much lower, while it could solve all problem instances (Figure ). As for the running time of the methods, robust methods require high CPU times, while simulation-based RPN and ITR have comparable running times (the CPU time of RPN includes the CPU time of fitting the regression model).
6.2. Production and capacity planning of the pre-inventory processes
The production planning model of the pre-inventory processes (Section 5) is responsible for calculating the lot sizes and the corresponding shift plans with the operator–machine assignments
, to ensure that the modules required by the final assembly lines will be available in the inventory. In order to analyse different production scenarios, the DES model of the pre-inventory segments was applied, simulating the machining, deburring and surface treatment processes. The characteristics of the test system are as they follow. In the machinery,
flexible machines are available, and
different base module types are produced. The resolution of the plans is 2 hours, therefore,
and
. The parameters of the modules are summarised in Table . The demands
for the modules were randomly generated by uniform distribution with the following bounds:
. The production planning model (introduced in Section 5) was implemented in FICO®Xpress, and solved by its default branch and bound solver, with the stopping criterion that the optimality gap should be at most 6%. The average running time of the solver algorithm was 180 seconds.
Table 3. Module parameters in the test case.
In order to analyse the performance of the planning method if some of the parameters are stochastic, a sample production plan was executed in a simulation environment. In case the calculated plans cannot be executed properly, final assembly of products will be in late. In the analysis, different production scenarios were compared, in which the lead times , the manual processing times
and machine availability A were modified to possess a certain deviation instead of being deterministic. Machine availability is the time percentage, during which a machine can be used for production. The main measures were the total backlogs realised at the inventory when the demands of final assembly lines were not satisfied. In this case, the total (
) and percental (
) amount of backlogs were observed:
(25)
(26)
where is the total volume produced of module m in period
resulted by the simulation analysis.
In the test scenarios, the effect of the uncertain lead and manual operation times were analysed by representing them as stochastic variables with normal distributions, specified by the mean and standard deviation parameters
. In each scenarios, the standard deviation of the time parameters were set to 10% of the mean value:
. The latter input parameters of the test scenarios and the corresponding simulation results are summarised in Table .
Table 4. Simulation analysis: execution of the production plans (pre-inventory processes).
According to the test results, the proposed planning method is able to produce the expected outcome, more specifically to plan the production of the pre-inventory processes in a way to provide enough modules for the final assembly processes without backlogs. By this way, the smoothness of production can be maintained, and the execution of the production plans calculated for the final assembly lines is independent of the pre-inventory processes in most of the test scenarios. In some of the scenarios, backlogs occur during the execution, which means that the final assembly of the product cannot be started in the planned time. Although it would cause problems in a real production situation, backlogs only occur in the scenarios with extreme parameters (e.g. processing times increased by 60%), and the amount of realised backlogs in that cases are also relatively low.
6.3. Discussion of the results
As in general, it is a managerial decision whether it is worth for a company paying extra amounts to increase the robustness of the plans, however, it is proven by the benchmark results that the cost of robustness is not really significant if the RPN method is applied. More specifically, the robustness of the planning can be increased by 11% in average, for additional costs of 3%, which is advantageous compared to the other analysed robust planning methods. Besides, the CPU time of running the solver (together with the data analysis) in normal and high-order numbers is only increased by 10 seconds in average, while robust plan can be obtained by RPN within maximum 800 seconds even in the hardest problem instances with extreme orders. It is also important that robustness cannot be simply increased by increasing the norm cycle times (Figure ), but properly combining production lots and defining their release date. While in RPN one does not have to care about adjusting the simulation and planning parameters as they are imported from MES, it is important to precisely set the uncertainty sets in robust optimisation models, which is not possible in several cases. As for the robustness, it can be concluded that robustness is increased significantly (11% in average), without much extra efforts, which is a considerable advantage of the RPN method.
The main benefit of the proposed workflow is its easy integration in the existing planning workflow without significant modifications in the models. For companies applying MES and ERP systems, accessing and loading the data in the analysis model by queries can be done easily, without any special requirements. The major prerequisite of applying the proposed robust planning workflow is the simulation model of the assembly lines, which however can be quickly built if a common structure can be applied. In the planning model, modifications and additional modules are not necessary, as only the capacity requirements need to be changed, the other parts of the model remain the same, in contrast to robust optimisation tools that require special solver engines usually not available at companies. From implementation point of view, the tool itself is flexible and does not require hard-wired heuristics, besides, the simulation model itself can be also used for multiple purposes: e.g. defining the capacity control modes or projecting the future behaviour of the system in various conditions. Thanks to the MES connection, the models always utilise up-to-date production data, while able to consider the stochastic nature of processes and parameters, which is not possible in the current norm time-based planning.
7. Summary and conclusions
In the paper, a novel planning method was introduced for process chains that are operated with pull strategy. The planning problem was decomposed at the in-process inventory of the modules, therefore, the planning procedure has two main phases: first, the production plans of the final assembly lines are calculated, and then the production planning of the pre-inventory processes is done, considering already the calculated plans of the assembly lines. In case of the final assembly planning, a simulation-based optimisation method was applied to manage the stochastic variables and random events in the mathematical model, without increasing its complexity and running time. Therefore, regression models were applied to predict the actual capacity requirements of different production scenarios instead of calculating the plans according to the idealistic cycle times. Besides the production planning, the simulation models of the assembly lines are applied to determine the proper control policies of the lines, which resulted in reduced idle times and balanced operator workloads. The performance of the calculated plans was analysed by executing them in a simulation environment, representing the possible random events and stochastic parameters. According to the test results, the proposed planning method provides robust production plans, and performs well in a real production environment.
Besides the production planning of the final assembly lines, important part of the proposed methodology is the planning of the material supply. In the pre-inventory planning model, the objective was to minimise the inventory costs of the modules, while providing the parts that are required by the final assembly lines. The operator–machine assignment problem was also solved to decrease the human capacity requirements of the production. According to the results, the proposed method is able to plan the production in a way that the continuousness of the production along the whole process chain can be ensured, and customer expected service-level can be maintained.
As for the future work, three potential directions are identified by the authors that worth further research work. First option is the analysis of different, common-applied manufacturing systems like machine flow shops where manufacturing lead time is one of the most important planning parameters. Pfeiffer et al. (Citation2016) highlighted that data analysis techniques on shop floor data can be applied to accurately predict the lead times that enables to calculate robust production and capacity plans. In that case, additional prediction parameters need to be considered like work-in-progress of buffer levels. Besides the manufacturing environment, second direction is the application of different function approximation techniques that can be combined with mathematical optimisation tools. In case of complex relations among the parameters, piecewise linear regression models might be suitable to predict the target parameters while keeping the linearity of the optimisation model. Third possible future direction is linking the method with the latest IT solutions applied in cyber-physical production systems (CPS). In CPSs, detailed data about products, processes and the system is available to gather information that can be further utilised in planning and control applications.
Additional information
Funding
Notes
No potential conflict of interest was reported by the authors.
1 All the computational experiments presented in the paper were performed on a laptop with 8GB RAM, and Intel®Core i5 CPU of 2.6 GHz and under Windows 8.1 64 bit operating system.
References
- Aghezzaf, El-Houssaine, Carles Sitompul, and Frank Van den Broecke. 2011. “A Robust Hierarchical Production Planning for a Capacitated Two-stage Production System.” Computers & Industrial Engineering 60 (2): 361–372.
- Alem, Douglas Jose, and Reinaldo Morabito. 2012. “Production Planning in Furniture Settings via Robust Optimization.” Computers & Operations Research 39 (2): 139–150.
- Almeder, Christian, Diego Klabjan, Renate Traxler, and Bernardo Almada-Lobo. 2015. “Lead Time Considerations for the Multi-level Capacitated Lot-sizing Problem.” European Journal of Operational Research 241 (3): 727–738.
- Aytug, Haldun, Mark A. Lawley, Kenneth McKay, Shantha Mohan, and Reha Uzsoy. 2005. “Executing Production Schedules in the Face of Uncertainties: A Review and Some Future Directions.” European Journal of Operational Research 161 (1): 86–110.
- Azadivar, Farhad. 1999. “Simulation Optimization Methodologies.” In Proceedings of the 31st Conference on Winter Simulation: Simulation–a Bridge to the Future-Volume 1, 93–100. Phoenix, AZ: ACM.
- Ben-Tal, Aharon, Laurent El Ghaoui, and Arkadi Nemirovski. 2009. Robust Optimization. Princeton, NJ: Princeton University Press.
- Berretta, Regina, Paulo M. França, and Vinícius A. Armentano. 2005. “Metaheuristic Approaches for the Multilevel Resource-constrained Lot-sizing Problem with Setup and Lead Times.” Asia-Pacific Journal of Operational Research 22 (02): 261–286.
- Bertsimas, Dimitris, David B. Brown, and Constantine Caramanis. 2011. “Theory and Applications of Robust Optimization.” SIAM Review 53 (3): 464–501.
- Byrne, Mike, and Murad Hossain. 2005. “Production Planning: An Improved Hybrid Approach.” International Journal of Production Economics 93: 225–229.
- ElMaraghy, H., G. Schuh, W. ElMaraghy, F. Piller, P. Schönsleben, M. Tseng, and A. Bernard. 2013. “Product Variety Management.” CIRP Annals-Manufacturing Technology 62 (2): 629–652.
- Fleischmann, Bernhard, Herbert Meyr, and Michael Wagner. 2005. “Advanced Planning.” In Supply Chain Management and Advanced Planning, 81–106. Berlin, Heidelberg: Springer-Verlag.
- Gabrel, Virginie, Cécile Murat, and Aurélie Thiele. 2014. “Recent Advances in Robust Optimization: An Overview.” European Journal of Operational Research 235 (3): 471–483.
- Gansterer, Margaretha, Christian Almeder, and Richard F. Hartl. 2014. “Simulation-based Optimization Methods for Setting Production Planning Parameters.” International Journal of Production Economics 151: 206–213.
- Gyulai, Dávid, Botond Kádár, András Kovács, and László Monostori. 2014. “Capacity Management for Assembly Systems with Dedicated and Reconfigurable Resources.” CIRP Annals-Manufacturing Technology 63 (1): 457–460.
- Gyulai, Dávid, Botond Kádár, and László Monostori. 2014. “Capacity Planning and Resource Allocation in Assembly Systems Consisting of Dedicated and Reconfigurable Lines.” Procedia CIRP 25: 185–191.
- Helber, Stefan, and Florian Sahling. 2010. “A Fix-and-optimize Approach for the Multi-level Capacitated Lot Sizing Problem.” International Journal of Production Economics 123 (2): 247–256.
- Herroelen, Willy, and Roel Leus. 2004. “Robust and Reactive Project Scheduling: A Review and Classification of Procedures.” International Journal of Production Research 42 (8): 1599–1620.
- Hvolby, Hans-Henrik, and Kenn Steger-Jensen. 2010. “Technical and Industrial Issues of Advanced Planning and Scheduling (APS) Systems.” Computers in Industry 61 (9): 845–851.
- Irdem, D. Fatih, N. Baris Kacar, and Reha Uzsoy. 2010. “An Exploratory Analysis of Two Iterative Linear Programming - Simulation Approaches for Production Planning.” IEEE Transactions on Semiconductor Manufacturing 23 (3): 442–455.
- Kibira, Deogratias, Qais Hatim, Soundar Kumara, and Guodong Shao. 2015. “Integrating Data Analytics and Simulation Methods to Support Manufacturing Decision Making.” In Proceedings of the 2015 Winter Simulation Conference, 2100–2111. Huntington Beach, CA: IEEE Press.
- Ko, Keyhoon, Byung H. Kim, and SeockK Yoo. 2013. “Simulation Based Planning & Scheduling System: MozArt.” In Proceedings of the 2013 Winter Simulation Conference: Simulation: Making Decisions in a Complex World, 4103–4104. Washington, DC: IEEE Press.
- Kozłowski, Edward, Walter Terkaj, Arkadiusz Gola, Mikuláš Hajduk, and Antoni \’{S}wić. 2014. “A Predictive Model of Multi-stage Production Planning for Fixed Time Orders.” Management and Production Engineering Review 5 (3): 23–32.
- Krenczyk, Damian, and Mieczyslaw Jagodzinski. 2015. “ERP, APS and Simulation Systems Integration to Support Production Planning and Scheduling.” In 10th International Conference on Soft Computing Models in Industrial and Environmental Applications, 451–461. Springer.
- Laroque, Christoph, Robin Delius, Jan-Hendrik Fischer, and Dennis Horstkaemper. 2012. “Increase of Robustness in Production Plans Using a Hybrid Optimization and Simulation Approach.” International Journal on Advances in Systems and Measurements5 (3 and 4):100–112.
- Lödding, Hermann. 2012. Handbook of Manufacturing Control: Fundamentals, Description, Configuration. Berlin, Heidelberg: Springer Science & Business Media.
- Melouk, Sharif H., Nickolas K. Freeman, David Miller, and Michelle Dunning. 2013. “Simulation Optimization-based Decision Support Tool for Steel Manufacturing.” International Journal of Production Economics 141 (1): 269–276.
- Meyr, Herbert, Michael Wagner, and Jens Rohde. 2015. “Structure of Advanced Planning Systems.” In Supply Chain Management and Advanced Planning, 99–106. Berlin, Heidelberg: Springer.
- Monostori, László, Balázs Csáji, Botond Kádár, András Pfeiffer, Elizabeth Ilie-Zudor, Zsolt Kemény, and Marcell Szathmári. 2010. “Towards Adaptive and Digital Manufacturing.” Annual Reviews in Control 34 (1): 118–128.
- Naeem, Mohd Arshad, Dean J. Dias, Rupak Tibrewal, Pei-Chann Chang, and Manoj Kumar Tiwari. 2013. “Production Planning Optimization for Manufacturing and Remanufacturing System in Stochastic Environment.” Journal of Intelligent Manufacturing 24 (4): 717–728.
- Pfeiffer, András, Dávid Gyulai, Botond Kádár, and László Monostori. 2016. “Manufacturing Lead Time Estimation with the Combination of Simulation and Statistical Learning Methods.” Procedia CIRP 41: 75–80.
- Pochet, Yves, and Laurence A Wolsey. 2006. Production Planning by Mixed Integer Programming. New York: Springer-Verlag.
- R Development Core Team. 2008. R: A Language and Environment for Statistical Computing. Vienna, Austria: R Foundation for Statistical Computing. ISBN 3-900051-07-0. http://www.R-project.org.
- Ramezanian, Reza, Donya Rahmani, and Farnaz Barzinpour. 2012. “An Aggregate Production Planning Model for Two Phase Production Systems: Solving with Genetic Algorithm and Tabu Search.” Expert Systems with Applications 39 (1): 1256–1263.
- Sahinidis, Nikolaos V. 2004. “Optimization under uncertainty: state-of-the-art and opportunities.” Computers & Chemical Engineering 28 (6): 971–983.
- Sahling, Florian, Lisbeth Buschkühl, Horst Tempelmeier, and Stefan Helber. 2009. “Solving a Multi-level Capacitated Lot Sizing Problem with Multi-period Setup Carry-over via a Fix-and-optimize Heuristic.” Computers & Operations Research 36 (9): 2546–2553.
- Schemeleva, Kseniya, Xavier Delorme, Alexandre Dolgui, and Frédéric Grimaud. 2012. “Multi-product Sequencing and Lot-sizing under Uncertainties: A Memetic Algorithm.” Engineering Applications of Artificial Intelligence 25 (8): 1598–1610.
- Stevenson, Mark, Linda C. Hendry, and B. G. Kingsman. 2005. “A Review of Production Planning and Control: The Applicability of Key Concepts to the Make-to-order Industry.” International Journal of Production Research 43 (5): 869–898.
- Tenhiälä, Antti, and Pekka Helkiö. 2015. “Performance Effects of Using an ERP System for Manufacturing Planning and Control under Dynamic Market Requirements.” Journal of Operations Management 36: 147–164.
- Tolio, Tullio, Marcello Urgo, and József Váncza. 2011. “Robust Production Control Against Propagation of Disruptions.” CIRP Annals-Manufacturing Technology 60 (1): 489–492.
- Zanjani, Kazemi, Mustapha Nourelfath Masoumeh, and Daoud Ait-Kadi. 2010. “A Multi-stage Stochastic Programming Approach for Production Planning with Uncertainty in the Quality of Raw Materials and Demand.” International Journal of Production Research 48 (16): 4701–4723.