Abstract
Lean is and will still be one of the most popular management philosophies in the Industry 4.0 context and simulation is one of its key technologies. Many authors discuss about the benefits of combining Lean and simulation to better support decision makers in system design and improvement. However, there is a lack of reviews in the domain. Therefore, this paper presents a four-stage comprehensive review and analysis of existing literature on their combination. The aim is to identify the state of the art, existing methods and frameworks for combining Lean and simulation, while also identifying key research perspectives and challenges. The main trends identified are the increased interest in the combination of Lean and simulation in the Industry 4.0 context and in their combination with optimisation, Six Sigma, as well as sustainability. The number of articles in these areas is likely to continue to grow. On the other hand, we highlight six gaps found in the literature regarding the combination of Lean and simulation, which may induce new research opportunities. Existing technical, organisational, as well as people and culture related challenges on the combination of Lean and simulation are also discussed.
Introduction
In recent decades organisations have sought to introduce more variety and quality in products and services while simultaneously reducing costs, increasing profits, increasing the efficiency of production processes for shorter product life-cycles, and investing in the employees’ working conditions and customer satisfaction. Huge transformation has occurred in these areas over the last century, and even greater changes are expected in the context of Industry 4.0. The goal pursued in this industrial revolution is to provide companies with the needed flexibility to face those changes (Gaddi, Garbellini, and Garibaldo Citation2017; Hirsch-Kreinsen Citation2016).
Lean is probably one of the most popular current management philosophies and it will still be key in the Industry 4.0 context (Buer, Strandhagen, and Chan Citation2018; Goienetxea Uriarte, Ng, and Urenda Moris Citation2018). The review by Ciano et al. (Citation2019) also confirms the increasing interest in the link between Lean and Industry 4.0. Lean aims to eliminate waste from companies’ processes to make them more efficient and increase their competitiveness, while at the same time adding value to their customers (Liker Citation1996). It emerged in Japan in the post-war environment of the nineteen-fifties when Taiichi Ohno defined specific methods and tools to make Toyota Motor Company more competitive. These methods and tools were integrated in a system that became known initially as the Toyota Production System (Liker Citation2004) and later as ‘Lean’ (Womack, Jones, and Roos Citation1990). Although Lean was first designed and applied in manufacturing, it has been extended to other domains such as healthcare and construction (Bhamu and Singh Sangwan Citation2014). There are many methods, concepts and philosophies that have been employed together with Lean in the literature. Some of these are Theory of Constraints, Six Sigma (Lean sigma or Lean-Six Sigma), agility (Leagile) or sustainability concepts (Lean and green) (Bhamu and Singh Sangwan Citation2014; Hines, Rich, and Holweg Citation2004).
However, the literature barely addresses the impact of the introduction of different Industry 4. 0 technologies in already established management philosophies such as Lean, and how Lean will influence in the implementation of these technologies (Buer, Strandhagen, and Chan Citation2018). The term virtual factory, sometimes used interchangeably with digital factory or digital twin, is a key notion within Industry 4.0 (Mrugalska and Wyrwicka Citation2017). It can be defined as an integrated simulation model which provides an advanced decision support capability (Jain et al. Citation2016). Simulation is, therefore, a relevant technology in the Industry 4.0 context. Simulation techniques, were mostly developed in the fifties as a support tool for analysing, designing and improving companies’ processes (Goldsman, Nance, and Wilson Citation2010; Robinson Citation2005). They have evolved considerably in recent years and can now even support strategic, tactical and operational decision-making processes (Negahban and Smith Citation2014). According to Banks et al. (Citation2014), simulation is ‘the imitation of the operation of a real-world process or system over time’.
Some authors have discussed the needs or issues to be addressed in the future by simulation, including a more inclusive link between Lean and simulation where simulation needs to become a natural activity within Lean system design and improvement projects (Diamond et al. Citation2002). As defended by Fowler and Rose (Citation2004), one of the biggest challenges faced to increase the use of simulation is its acceptance by industry. They state that simulation should be presented as complementary to Lean tools in order to support system design and improvement. Modelling and simulation have also been envisioned as key technologies to transform companies ‘into lean, dynamic, responsive entities that thrive on challenge and change’ (IMTR Citation2000). At the same time, many other authors have identified simulation as the perfect complement to Lean methods and tools (Abdulmalek and Rajgopal Citation2007; Ferrin, Miller, and Muthler Citation2005; Goienetxea Uriarte et al. Citation2016; Jia Citation2010; Robinson et al. Citation2012) and to overcome some of their limitations, such as: (1) not taking into consideration the variability of the system (Standridge and Marvel Citation2006); (2) lack of providing a systemic view on how the changes in one component affect others (Marvel and Standridge Citation2009; Standridge and Marvel Citation2006); (3) lack of dynamic behaviour and incapability of Lean tools to evaluate non-existing processes before implementation (Goienetxea Uriarte et al. Citation2016); and consequently, (4) an inability to analyse complex systems. However, the literature shows that there is a lack of comprehensive frameworks on how to combine IT tools and Lean (Pinho and Mendes Citation2017), and specifically simulation and Lean.
Assuming that the applicability of and interest in Lean and simulation will continue to grow in the Industry 4.0 context (Goienetxea Uriarte, Ng, and Urenda Moris Citation2018; Ruiz Zúñiga, Urenda Moris, and Syberfeldt Citation2017; Wagner, Herrmann, and Thiede Citation2017), we set out to review the existing work on combining them. The main contributions of this paper are:
To our knowledge, this is the first comprehensive review conducted in the field. The existing reviews are analysed and their gaps are discussed.
The review provides an overview of the evolution of Lean and simulation in the literature, including an analysis of the domains for this application, as well as the most employed methods and tools.
A comparative review of the existing frameworks, methods and methodologies for combining Lean and discrete event simulation, as well as their characteristics are provided.
The future research perspectives including the existing trends and limitations found in the conducted review, as well as the existing challenges are identified and described.
The article serves as reference material for professionals, researchers and academics working with Lean, simulation, and/or decision-making support techniques. The domain of the review is wide enough to be attractive to those working in the industrial, healthcare and construction sectors, or with education in Lean and/or simulation. The different methods, methodologies, and frameworks reviewed here may serve as examples for those who want to integrate simulation as a tool in their Lean working standards, or those working with simulation who want to determine how Lean principles and tools could support their simulation processes. It also offers examples where simulation has been used to teach Lean concepts.
This article is structured as follows. Section 2 introduces the methodology used to conduct the various stages of the review, and Section 3 presents the evolution of the combination of Lean and simulation in the literature. Section 4 analyses existing reviews on Lean and simulation. Section 5 elaborates on the existing methods, methodologies or frameworks for combining Lean and DES, and Section 6 describes the future research perspectives and existing challenges. Finally, Section 7 reveals the conclusions and future work.
Literature review methodology
The aim of this paper is to offer a review of the combination of Lean and simulation. To conduct this study, a systematic literature review approach was used as defined by Tranfield, Denyer, and Smart (Citation2003), where a plan to conduct the review was defined, the review was conducted, and the results and conclusions are reported in this paper.
The review of academic publications presented in this paper involves four stages as shown in Figure . These are:
Analysis of the evolution of the combination of Lean and simulation in the literature.
Analysis of the review papers on the combination of Lean and simulation.
Analysis of the literature on frameworks, methodology or methods of combining Lean and simulation.
Analysis of the future research perspectives and existing challenges.
The review is based on literature recorded in the Scopus citation database (www.scopus.com). Scopus is the largest abstract and citation database, indexing many serial and non-serial publications and millions of conference papers, including the majority of the most important journals in the field of operations management research as listed in Theoharakis et al. (Citation2007), Olson (Citation2005) and Barman, Hanna, and LaForge (Citation2001). It is also one of the most common sources to conduct bibliometric analyses and the most complete one in the engineering field (Mongeon and Paul-Hus Citation2016).
In the first stage, the keywords ‘lean’ and ‘simulation’ were entered in the search tool of Scopus to obtain a first list of works combining these two terms in the title, abstract or keywords. The search included papers between 1990 and the 4 September 2018 (when the search was conducted). The year 1990 was used as the starting date because that was the year in which the term ‘Lean’ was made popular in the book by Womack, Jones, and Roos (Citation1990). The results were then filtered by subject area and keywords. Text mining software called VOSViewer, developed by Van Eck and Waltman (Citation2010), was also applied to detect non-relevant repeating terms and exclude them from the list. A continuous combination of text mining and Scopus filtering tools narrowed down the number of hits until text mining no longer showed any irrelevant clusters of terms. The exclusion criteria are presented in Table . A general analysis of the filtered documents was then conducted in stage 1 to obtain the evolution on the combination of Lean and simulation in the literature.
Table 1. Summary of the exclusion criteria per stage of the review.
The analysis in stages 2 and 3 was based on these filtered documents, which were further filtered based on the specific terms to be analysed in each stage. These are shown in Figure . Stage 2 analysed papers including the terms ‘review*’ or ‘survey*’ within the fields of title, abstract and keywords. Similarly, the terms ‘framework’ or ‘method*’ were included in Stage 3. The term ‘model’, which may also be employed to refer to framework or method, was not included in the search because it produced too many articles referring only to the models developed via simulation. As discrete event simulation (DES) is the most popular simulation method in the literature (Jahangirian et al. Citation2010; Negahban and Smith Citation2014), the search in this stage included the word ‘discrete*’ to be able to select only those papers related to DES. Further details are provided in section 4.
The abstracts of the identified documents were first reviewed to determine their relevance, and thereafter the full text of each selected document was also read to ensure that it was indeed relevant for inclusion. The exclusion criteria for stages 2 and 3 are also detailed in Table . Relevant information from each of the selected documents was extracted and detailed at each stage. When a document referred to another publication, the references were followed up and incorporated in the review where relevant. The relevant documents were classified according to different criteria at each stage and important knowledge was extracted. Finally, a general analysis of the gaps, trends, future directions and challenges was performed in stage 4, based on the previous stages.
Note that in the remainder of this article, the documents identified by Scopus are generally referred to as papers and articles, even though some of them are chapters from books.
Stage 1: evolution of the combination of Lean and simulation in the literature
A generic search on ‘lean’ and ‘simulation’ identified 5,386 publications. Figure (a) illustrates the evolution of this combination of terms over time (results from 2018 and 2019 are not included in the chart to avoid showing partial statistics).
Figure 2. Number of publications per year combining ‘lean’ and ‘simulation’ (a) and ‘lean’, ‘simulation’ and ‘optimisation’ (b).
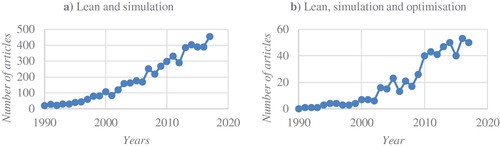
The combination of simulation and optimisation, the so-called simulation-based optimisation (SBO) techniques or ‘intelligent simulation’ (Jahangirian et al. Citation2010), although still not a mature technique, is of growing interest in the field of operational research to provide optimal or near-optimal solutions to decision makers. Optimisation, as defined by Deb (Citation2001) is ‘a procedure of finding and comparing feasible solutions until no better solution can be found’. Therefore, Figure (b) shows the number of papers combining the keywords ‘lean’, ‘simulation’ and ‘optimisation’.
In both Figures (a) and (b), the number of articles quintupled between 2000 and 2017, while the number of articles published per year between 2003 and 2009 also more than doubled. This implies that the combination of Lean, simulation and optimisation is becoming increasingly popular.
As the number of published papers combining ‘lean’, ‘simulation’ and ‘optimisation’ is relatively limited, we analyse only the papers related to Lean and simulation in this stage 1, as well as in stages 2 and 3.
After the exclusion of non-relevant terms and subject areas, 1194 papers remained. A text mining analysis was then performed, and Figure shows the results. The size of each circle is proportional to the number of times that term appeared in the papers analysed (with a bigger circle representing a higher number of papers). The lines between the terms represent a connexion between them.
Figure 3. Text mining results and clustering of the most repeated terms in the selected papers that combine ‘lean’ and ‘simulation’.
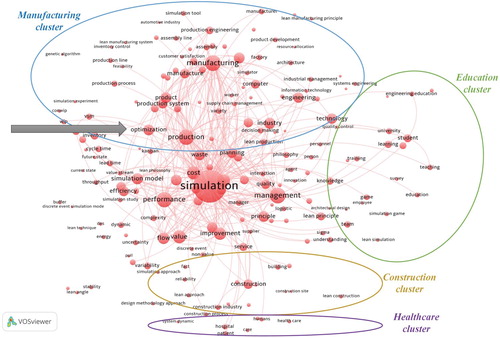
‘Simulation’ is the word that appears most often in the search. It is linked to other keywords such as ‘management’, ‘performance’, ‘efficiency’, and ‘improvement’, all related to the use of simulation to support decision-making in system design and improvement. The term ‘lean’ was identified in different combinations of words, including ‘lean principle’, ‘lean philosophy’, ‘lean production’, and ‘lean manufacturing system’. This is not surprising, as there is no standard way to express the concept of Lean, its principles or tools in the literature (Mirdad and Eseonu Citation2015). The performance indicators/results used most often in the papers are ‘lead time’, ‘throughput’, ‘cycle time’, ‘buffer’, ‘waste’, ‘customer satisfaction’, ‘planning’, ‘quality’ and ‘cost’. The Lean tools that most often appear within the shown terms are, according to Figure , those related to ‘value stream mapping’ (VSM) (also current and future state), ‘Kanban’, ‘CONWIP’ and ‘inventory control’, as also reported by other authors (Gurumurthy and Kodali Citation2011; Tokola, Niemi, and Väistö Citation2016). A more specific search was then conducted based on the toolbox of Bicheno and Holweg (Citation2009) to select the most characteristic Lean methods and tools used in combination with simulation. Just in Time (JIT), which is one of the pillars of the Lean house (Bicheno and Holweg Citation2009), appears many times in the results, mainly in combination with Kanban. This combination is usually tested with simulation before implementation in a real system. It is not strange that the most cited method is VSM, as many authors point out that simulation (mainly DES) is the perfect tool to complement or substitute VSM (Lian and Van Landeghem Citation2007). The second pillar, Jidoka, and its related methods or tools such as Poka-Yoke and Andon, were not often found, probably because it is difficult to simulate these concepts. They are applied in a later stage, usually in the implementation phase of system design or improvement projects. CONWIP was cited many times along with Kanban as a method to be tested with simulation before implementation (see e.g. Koulouriotis, Xanthopoulos, and Tourassis Citation2010). While many of the articles stated that they had employed Lean methods and tools, many do not mention any specific Lean method or tool employed in combination with simulation in the searched fields. Therefore, rather than showing an accurate number of papers for each method or tool, Figure shows the tendency to use them in combination with simulation.
Four different clusters of papers were identified in Figure according to the area of application: manufacturing, education, healthcare, and construction. The majority of the selected papers were within those application areas, although individual papers on industries such as transport, fishing, mining, and aeronautics were also identified. The majority of papers within the manufacturing domain addressed production system design and improvement projects, but there were also cases analysing the supply chain. The education cluster includes papers related to the use of simulation to teach Lean concepts. Simulation in these cases may refer to simulation models, such as DES, or to the use of simulation games, which are not necessarily computer-based. The construction cluster gathers papers related to the ‘lean construction’ concept and simulation is used mainly to test future Lean scenarios. The healthcare cluster contains papers addressing mainly hospital flow improvements and resource allocation studies. Almost half of the papers were from the industrial domain, followed by the education domain, as shown in Figure .
There are three main simulation paradigms: DES, system dynamics (SD) and agent-based modelling (ABM) (Borshchev Citation2014). DES (the most common) and SD were identified among the most repeated terms. The term ‘optimisation’, pointed to by an arrow in Figure , appears in some papers. Even the term ‘genetic algorithms’, which relates to optimisation, appeared in the text mining results.
An analysis by year of the selected papers is shown in Figures and . Figure shows the evolution of the total number of selected papers published with the combination of ‘lean’ and ‘simulation’, subdivided into the most common methods including DES, SD, ABM, as well as mathematical models, Monte Carlo, and education games/simulation. There were also a few articles that combined more than one method (e.g. multi-method and hybrid modelling). In those cases, the same paper was classified under two or more of the methods shown in Figures and .
Figure shows that more than sixty percent of the total number of papers were published between 2010 and 2017. Although individual papers were published in the early nineties, it seems that the initial years of the 2000s were an inflection point in the flourishing of this area. Unsurprisingly, DES is still the most reported method, followed by educational games and simulations, as shown in Figure . Both of them show a positive trend, which means that interest in these areas is growing within the research community. SD is also slightly rising. ABM and Monte Carlo are the least reported methods in the literature, with a range of zero to three papers per year and no clear growing trend. Mathematical models were mostly reported in the mid-2000s, with a decreasing trend in the last decade, as was also noted by Brailsford et al. (Citation2009). This pattern can also be seen in Figure , with the unusual exception that there are more publications between 2000 and 2009 than in the last decade.
Figure shows the top ten countries publishing in this field. The USA leads this list, followed by Germany and China. It is not surprising that North America and Europe dominate as they historically have an industrial background and have more advanced technological infrastructure than other areas of the world. But Brazil, India, and China, three of the so-called BRIC countries (O’Neil Citation2001), also appear in the top ten. The globalisation of American and European companies to these countries has probably promoted interest in the areas of Lean and simulation, and it is believed this trend will continue to grow. Japan, the country where Lean first emerged, is also on the list.
Stage 2: review papers on the combination of Lean and simulation
There are many reviews of Lean (Bhamu and Singh Sangwan Citation2014; Jasti and Kodali Citation2015), how it has been implemented (Yadav et al. Citation2017), and Lean frameworks (Anand and Kodali Citation2010a; Chay et al. Citation2015). There are also many reviews on the use of simulation in different domains such as manufacturing (Jahangirian et al. Citation2010; Negahban and Smith Citation2014; Smith Citation2003) and healthcare (Brailsford et al. Citation2009; Mielczarek and Uziałko-Mydlikowska Citation2012; Van Lent, Vanberkel, and Van Harten Citation2012). Although SBO is a more recent combination of techniques, there are reviews on SBO methods and applications (April et al. Citation2003; Figueira and Almada-Lobo Citation2014; Tekin and Sabuncuoglu Citation2004; Wang and Shi Citation2013). However, this article does not aim to review Lean and simulation on their own, but we set out to identify previous review papers that combine the terms.
After excluding the non-relevant papers, five reviews were deemed to meet the criteria for inclusion, as detailed in Table .
Table 2. Review articles on the combination of Lean and simulation.
Mohamad et al. (Citation2016) identify ten articles in which simulation was employed to enhance the performance of Lean tools. They identify the most important factors for successfully implementing Lean tools as waste identification, the application of Lean tools in the manufacturing process, and the evaluation of Lean tools via simulation. Additionally, they propose a method called Lean Tools Simulation (LeTS-2015) in which simulation is employed to evaluate the impact of three Lean tools (Kanban, cellular manufacturing and Single Minute Exchange of Die) before implementation and to support better decision-making.
Tokola, Niemi, and Väistö (Citation2016) identify 24 papers in which simulation is used to evaluate the implementation of different Lean tools. They also note the most reported methods and tools (e.g. VSM, Kanban, changes in the layout, pull systems, WIP control) and those that could be studied via simulation but have rarely been studied that way in the literature (e.g. Poka-Yoke, U-shaped lines, and kitting). The review also showed that reduction of work in process (WIP), lead time, labour and floor space are the most common simulation results after applying different Lean methods and tools.
More specifically, Gurumurthy and Kodali (Citation2011) review the use of simulation with VSM, while Chu and Shih (Citation1992) review simulation with JIT including Kanban. Rane et al. (Citation2015) review the existing literature on techniques to improve assembly lines. Claiming that Lean is mostly employed for that purpose, they analyse additional techniques that can be applied to achieve Lean configurations, including simulation and SBO. Their review focuses on case studies on the use of these techniques in manufacturing applications, mostly in assembly lines and more specifically in vehicle assembly lines.
Of the five articles selected above, the one by Tokola, Niemi, and Väistö (Citation2016) is the most comprehensive, taking into account the reported number of references. However, the selection of papers was done by searching only the titles of articles for the keywords ‘manufacturing’, ‘lean’ and ‘simulation’, and the scope of their review is therefore very limited. The rest of the selected papers are either Lean tool specific (focused only on VSM or JIT) or application specific (assembly line).
Our review thus shows that there is still a gap in the literature for a complete review of this combination, including a more general view of Lean which is not tool or application specific. All the selected papers, except the one by Chu and Shih (Citation1992), were published in the last decade; this is in line with the statements in stage 1. Surprisingly, no healthcare, construction or education review paper discussing Lean and simulation in combination was found. This opens an opportunity for further research.
Stage 3: review of frameworks, methodology or methods of combining Lean and simulation
There are many publications explaining how to conduct a simulation study. Probably the most popular are the books by Banks et al. (Citation2014) and Law (Citation2007). Authors like Womack, Jones, and Roos (Citation1990) and Liker (Citation2004) provide information on how to implement Lean, although there is no single, easy path to do so. There are also articles presenting frameworks for implementing Lean, such as those by Anand and Kodali (Citation2010b) and Hines, Rich, and Holweg (Citation2004), or the disciplined approach to implementing Lean described by Hobbs (Citation2004). However, there is a lack of frameworks for the integration of Industry 4.0 techniques, such as simulation, and Lean (Buer, Strandhagen, and Chan Citation2018; Goienetxea Uriarte, Ng, and Urenda Moris Citation2018). Similarly, Pinho and Mendes (Citation2017) identify a lack of integrated comprehensive frameworks in their review on the role of information technologies in Lean contexts. Therefore, stage 3 of this study focuses particularly on the analysis of the existing frameworks, methods or methodologies for combining Lean and simulation.
The search in this stage included the word ‘discrete*’ to be able to select only those papers related to DES. DES is the most reported simulation method in the literature (Jahangirian et al. Citation2010; Negahban and Smith Citation2014), and as concluded in Stage 1, it is also the mostly combined method with Lean. DES models are discrete, stochastic and dynamic, three characteristics that are usually present in real-world problems, which is probably why DES is so popular (Jahangirian et al. Citation2010; Negahban and Smith Citation2014). DES is also considered as one of the key technologies to create virtual factories in the Industry 4.0 context (Jain et al. Citation2016). That is why this stage of the review focuses on DES.
In total 152 papers were retrieved for analysis. Many of these were focused on the combination of VSM with simulation. This is not surprising, because as previously identified in Stage 1, the combination of the Lean tool VSM with simulation is the most popular one in the literature. Many articles focus on the benefits of applying VSM and DES in combination in order to create a dynamic process map. Some examples are the papers by Abdulmalek and Rajgopal (Citation2007); Bal, Ceylan, and Taçoğlu (Citation2017); Atieh et al. (Citation2016); de Jong and Beelaerts van Blokland (Citation2016); and Da Silva and Ferreira (Citation2014). Others integrated simulation and VSM, with a simulation model being generated from a VSM map (Jia and Perera Citation2009; Lian and Van Landeghem Citation2007), or even created VSM from simulation models (Goienetxea Uriarte et al. Citation2019; Solding and Gullander Citation2009). Still, other studies included the combination of VSM and simulation with the study of Kanban (Sabaghi, Rostamzadeh, and Mascle Citation2015), or CONWIP (Yang et al. Citation2015). There were also combinations with additional techniques such as the use of constraint programming (Samant, Mittal, and Prakash Citation2018), or 3D-post simulation visualisation to support decision-making (Moghadam, Barkokebas, and Al-Hussein Citation2014). However, as concluded by Jarkko et al. (Citation2013) after their review of the combination of VSM and DES, the existing literature merely presents specific case studies without delving into the combination of the tools. As described in Table , such papers were excluded from the review, as the goal of this article is not to present a review of the combination of VSM and simulation. Only papers presenting frameworks or methodologies for combining Lean and simulation more comprehensively have been included, excluding those merely focused on a specific tool and related to a specific case study. In addition, some papers have been included that combine VSM and simulation with an original approach, not just introducing simulation after a VSM has been developed. Finally, 39 articles were included for comparison out of the initial 152.
A comparative analysis of the existing frameworks, methods or methodologies for combining Lean and simulation is shown in Table . The articles are grouped by domain (first column) and ordered by date (second column). The remaining columns classify the article according to the criteria described below. The parameters included for comparison are similar to those employed by Anand and Kodali (Citation2010b) when analysing different Lean frameworks, with some additions. The criteria used were selected because they are important when implementing new working standards in organisations. A brief description of the parameters and criteria is provided below:
Domain: The domain represents the specific domain in which the authors performed the study. These domains are related to the clusters identified in the first stage of this study (industry, construction, healthcare, and education). Although many articles pointed out the applicability of their frameworks or methods in other domains, most of them were not implemented in those domains, and therefore only the primary application domain of the article has been identified.
Author and year: The author or authors who wrote the article and the publication year.
Main purpose: According to Robinson et al. (Citation2012), Lean and simulation can be combined for three different purposes: (1) education, using simulation to teach Lean concepts; (2) evaluation, using simulation to evaluate current and future scenarios; and (3) facilitation, using simulation to facilitate discussions during Lean events (e.g. kaizen events) by offering quick evaluation of different improvement scenarios.
Comprehensiveness: Lean and simulation may be combined in different ways. Some combinations are just method/tool oriented, while others reference Lean principles. Comprehensiveness is, therefore, a measure of the completeness of the framework, method or methodology for combining Lean and simulation. It takes into account various ways of combining Lean and simulation, as shown in Figure , including:
c1) Lean method and tool analysis as an input to simulation.
c2) Simulation to evaluate the impact of Lean methods and tools before implementation.
c3) Lean method and tool implementation after simulation.
c4) Simulation to facilitate discussions during Lean events (e.g. kaizen events).
c5) Simulation to teach Lean concepts.
c6) Inclusion of Lean principles in the simulation process.
Figure 9. Different ways of combining Lean and simulation in the literature and their link to the purpose of the combination.
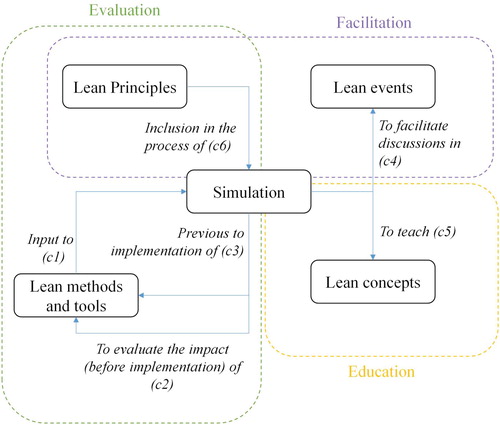
Table 3. Papers about frameworks, methods or methodologies which combine Lean and DES.
The level of comprehensiveness is defined as low, medium or high depending on the extent to which the above combinations are identified in the article. Articles are classified as having low comprehensiveness if only one or two ways of combining Lean and simulation are mentioned, medium comprehensiveness if three options are mentioned, and high comprehensiveness if more than three are mentioned.
Clarity in structure: This parameter indicates whether the framework, method or methodology has a clear structure (e.g. steps to take, which methods or tools to employ first) to facilitate its implementation in an organisation. It is rated as low, medium or high. ‘Low’ means no clear structure, while ‘high’ means a clear set of activities and indications on how to combine Lean and simulation.
Role definition: This parameter measures whether the article identifies the roles of the stakeholders in the combination of Lean and simulation. It will be rated as yes or no, depending on whether the roles are identified or not.
Remarks: Specific remarks on each article are included.
The charts in Figure offer a frequency analysis for each of the criteria described above.
Figure 10. Frequency analysis of the selected articles by different criteria: (a) domain, (b) purpose, (c) comprehensiveness level, (d) comprehensiveness – ways of combination, (e) clarity of structure and (f) role definition.
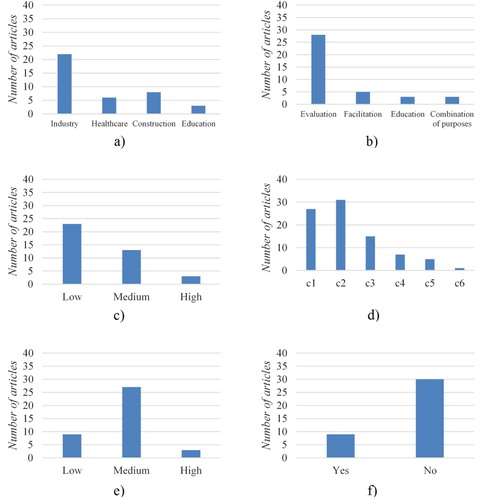
Analysis by domain
The articles were classified into domains on the basis of the application area presented in the article, although many identified the possibility of applying their approaches in other domains. They all had a specific application area, except for the book by El-Haik and Al-Aomar (Citation2006) which focused mainly on the industrial sector but also presented an example from healthcare.
The majority of the selected papers are from the industrial domain, followed by construction and healthcare, as shown in Figure (a). There are very few education articles presenting frameworks or methods where Lean and DES are combined. This result may seem to contradict Figure , which shows the search results from stage 1 and identified many articles in the education domain mentioning simulation and Lean (it was the second largest application domain after industry). The main reason for the difference is probably that DES was introduced as a keyword in this stage of the search. The result backs up the statement by Robinson et al. (Citation2012) that there are many computer-based simulation games to teach Lean, but they do not necessarily employ DES. Many of them merely use simulation as a keyword to refer to different types of games. The article by Cudney et al. (Citation2011) provides a specific example which shows computer simulation software being used to teach Lean concepts to engineering students, without specifying the type of simulation employed. However, their conclusions (that students get to experiment in a virtual world and see the impact of their decisions and the benefits of applying specific Lean methods and tools) would still be applicable if DES had been used. Other authors such as Chwif and Barretto (Citation2003) employ DES to teach operations management concepts to engineering students, but make no reference to Lean.
It is not surprising that most of the selected papers are from the industrial domain, for both Lean and simulation were initially developed to improve manufacturing systems. However, the increasing interest in areas such as construction and healthcare is apparent in this review, with many authors proposing standards for combining the two approaches and even providing case studies to prove the methods or frameworks proposed.
Analysis by main purpose of the combination
Both Robinson et al. (Citation2012) and Goienetxea Uriarte et al. (Citation2016) identify education, evaluation and facilitation as different purposes for combining DES and Lean. Baril et al. (Citation2016), referring to the article by Robinson et al. (Citation2012), note that these purposes align with predefined models, detailed models, and simple models respectively. The selected articles have been classified according to these purposes.
Figure (b) shows the classification of the selected papers by purpose. Most of them refer to the combination of Lean and simulation for evaluation purposes (both of the current and future state), which is the most common use of simulation models.
Very few focus only on the use of simulation for facilitation purposes in Lean events. Bhat, Gijo, and Jnanesh (Citation2014) present a combination of Lean and Six Sigma tools used to improve the registration process at an Indian hospital. They use simulation to facilitate discussions before Lean Six Sigma tool implementation. The article by De Oliveira Gomes and Trabasso (Citation2016) also sets out to define a simulation-aided continuous improvement method. The authors explain, step-by-step, how kaizen events can be complemented with simulation to develop continuous improvement projects. Robinson et al. (Citation2014), as in their earlier article (Robinson et al. Citation2012), also explain the benefits of using simulation to facilitate discussions during kaizen events.
Baril et al. (Citation2016) offer a different approach to Robinson et al. with their SimLean approach. Rather than developing a simple model during a kaizen event to support discussion (Robinson et al. Citation2012; Robinson et al. Citation2014), Baril et al. (Citation2016) propose the development of detailed simulation models before kaizen events ‘to avoid unnecessary discussions during the event’. The model, in this case, was developed in the ‘back office’. Although many authors included in this review do not explicitly state whether their models were ‘simple’ or ‘detailed’, it seems that most of them followed the approach of building detailed models to be used as a support during kaizen events.
Ramakrishnan, Al-Fandi, and Chen (Citation2009) present a framework focused on improving energy consumption via ‘Energy Kaizen’ in which simulation is employed to evaluate improvement alternatives. Ramakrishnan, Testani, and Orth (Citation2009) show how to follow the define, measure, analyse, improve, and control (DMAIC) steps in which different Lean tools (VSM, standard worksheet, etc.) are employed in the analysis phase during a kaizen event, and are then an input to the improvement phase where simulation is employed to evaluate different scenarios. Their article presents a house of Lean called a ‘kaizen framework’ by the authors, in which different tools are identified depending on the knowledge elements needed. The elements can include tools to ensure basic stability in the system (5S, visual management, etc.), structured problem solving (DMAIC), and flow opportunity analysis (VSM, simulation, etc.).
Only three papers were found in which DES was employed for Lean education. Esquenazi and Sacks (Citation2006) discuss the use of live simulation games and computer simulation games to teach Lean in the construction sector and present a case study using DES to teach Lean concepts. Shannon, Krumwiede, and Street (Citation2010) describe how they employed DES in engineering programmes at undergraduate and graduate levels to teach different Lean implementation strategies. Similarly, Constantino Delago et al. (Citation2016) present the use of DES to teach Lean methods to engineering students. The methods and tools they employed were VSM, 5S, SMED, pull flow and standardised work. The game was divided into different phases in which the students employed the simulation model to evaluate different possible improvements. These authors also proposed introducing different levels into the simulation model to make it more or less complex depending on the background and knowledge of the students.
Few of the selected articles dealt with the combination of Lean and simulation for more than one purpose. However, Robinson et al. (Citation2012) and later Goienetxea Uriarte et al. (Citation2016) highlight the benefits of combining Lean and simulation for evaluation, facilitation and educational purposes. Ali, Petersen, and De França (Citation2015) identify the possibility of employing simulation both for facilitation and for evaluation.
This analysis shows that regardless of the domain, the usual purpose of simulation is purely the evaluation of current or future states of the system, mainly related to system design projects. However, a few authors see the possibility of employing a combination of Lean and simulation over the whole lifecycle of a system, including not only an initial evaluation of the system’s design but also improvements to the system based on Lean principles. The combination of Lean and simulation could also be used to provide education about Lean in universities as well as in the industrial, healthcare or construction contexts, where it could facilitate the learning of Lean concepts.
Analysis by comprehensiveness
Comprehensiveness was classified based on six different ways of combining Lean and simulation. Figures (c) and (d) present how the articles were classified according to their level of comprehensiveness and ways of combination.
The vast majority of the selected articles, as shown in Figure (c), were rated as having low comprehensiveness. This is because their focus on evaluation meant that the only way of combining Lean and simulation were related to the use of simulation to evaluate the impact of Lean methods and tools before implementation (c2). Many authors also identified the need to introduce the analysis done via Lean methods and tools as an input to a simulation model (c1), including such methods as VSM, Yamazumi boards, fishbone diagrams, and 5 Whys. Most of the articles rated as having a medium level of comprehensiveness used a combination of c1 and c2.
The next most common combination is the application of Lean methods and tools after simulation (c3), either to draw a future VSM or to actually implement the Lean scenario tested by simulation. Using simulation to teach Lean concepts (c5) was seldom identified as a possible combination of the two concepts. The same applies to the use of simulation to facilitate discussions during Lean events (c4).
Several authors identified the need to take Lean principles into account in setting simulation objectives. Farrar, Abourizk, and Mao (Citation2004) proposed a framework to introduce Lean principles into simulation models in the construction sector. Other examples can be found in Al-Sudairi (Citation2007); Jia (Citation2010); Lu, Yang, and Wang (Citation2011); Yang and Lu (Citation2011) or Huang and Klassen (Citation2016). This point is extremely important to ensure a Lean result. However, it seems that the combination of Lean and simulation tended to focus only on the generic design or improvement project steps, using Lean methods and tools before and after the simulation process. Most of the papers followed an expert modelling approach when it came to building simulation models, with only simulation engineers being involved in the design and development of the model, rather than the facilitated modelling approach proposed by many authors (Franco and Montibeller Citation2010; Monks, Robinson, and Kotiadis Citation2016; Robinson et al. Citation2014). Using experts for modelling means that some of the fourteen Lean principles defined by Liker (Citation2004), such as those related to organisational learning which can define how the simulation process is conducted (c6), may be lost. The principles that are likely to be overlooked include: (1) going to Gemba, (2) making decisions by consensus, and (3) knowledge management, extending the knowledge gained during the simulation process to the project team. These issues are also highlighted in Goienetxea Uriarte et al. (Citation2016).
A more comprehensive approach is presented by Goienetxea Uriarte et al. (Citation2016), who reflect on the need for a broad view of the combination of Lean, simulation and optimisation, rather than a narrow focus on the use of simulation for evaluating Lean implementation. They argue that Lean can also play a role in supporting the simulation process and outline the benefits of including optimisation in the process. Another article with high comprehensiveness is presented by Shannon, Krumwiede, and Street (Citation2010), who describe a method to teach Lean concepts via DES. The authors use VSM as an input to simulation and future state VSM after simulation. They also describe the use of DES by engineering students to evaluate different Lean implementation strategies. Ramakrishnan, Testani, and Orth (Citation2009) also offer a comprehensive article focused on the use of simulation in kaizen events.
Analysis by clarity of structure
De Oliveira Gomes and Trabasso (Citation2016) state that many projects involving simulation follow a generic process such as the one defined by Banks et al. (Citation2014). However, ‘there is no formalised routine for the integration of process improvement and simulation activities’ (De Oliveira Gomes and Trabasso Citation2016). Jarkko et al. (Citation2013) make the same comment after analysing the literature on the combination of VSM and DES. Lin, Jin, and Chia (Citation2014) also state that the combination of simulation with Lean and Six Sigma tools has not been presented as a standard method. This analysis by degree of clarity of the structure tries to classify the selected papers according to the structure of the method presented.
Most of the papers have a medium level of clarity as shown in Figure (e), meaning that they present either a general flow chart or a brief description of each of the steps needed when conducting a study involving Lean and simulation. However, there are also some articles that present a clear structure for the proposed methods or frameworks. They provide a clear description of each of the steps and identify specific tools and Lean principles that can be employed at each step. For example, the book by El-Haik and Al-Aomar (Citation2006) presents a method called simulation-based Lean Six Sigma (3S-LSS) in which simulation, Lean and Six Sigma tools are identified in each step of the DMAIC process and a detailed description on how to conduct each step is provided. A similar approach is followed by Huang and Klassen (Citation2016), who present a detailed classification of different tools applicable for each stage of the DMAIC process. The thesis by Jia (Citation2010) also describes a detailed framework with seven steps for system design and improvement in which Lean and simulation are combined.
Analysis by clarity of role definition
Most of the selected articles do not define the people or roles that need to participate in each of the steps of the methods presented, as shown in Figure (f).
Some authors have partially identified the roles of the people involved. Jia (Citation2010), for example, notes the importance of defining the objectives of the simulation project and the need for management commitment, but offers no role identification for the different stages in simulation projects. Robinson et al. (Citation2012) and Bhat, Gijo, and Jnanesh (Citation2014) identify the roles of the people who participated in the case studies they present, but do not specify whether those roles are necessary in a more general context or for future studies following their method or framework. On the other hand, they do point out the importance of stakeholder engagement in simulation projects. Ramakrishnan, Testani, and Orth (Citation2009) identify the generic roles in each step of the kaizen events. Similarly, Baril et al. (Citation2016) identify the roles of the people who participated in their case study in healthcare in each of the DMAIC steps. These roles are general enough (hospital manager, Lean facilitator, clinic manager, intervention team, kaizen team, and university team) to be applicable to other healthcare applications and not just to the haematology-oncology case they present.
In the educational context, Shannon, Krumwiede, and Street (Citation2010) make a clear distinction between the role of the instructor and the role of the students, so their method will be easily replicable by other teachers. Constantino Delago et al. (Citation2016) also identify the activities of the students and the instructors, even if not as clearly as the previous paper.
The lack of role identification is especially noticeable when it comes to the identification of participants in the simulation process. Therefore, in the vast majority of cases, it can be assumed that the projects are undertaken mainly by simulation experts. This is in line with the statement by De Oliveira Gomes and Trabasso (Citation2016) that the lack of inclusion of companies’ collective knowledge in simulation projects is one of the key issues in continuous improvement projects. Goienetxea Uriarte et al. (Citation2016) also highlight the importance of including the project team and people with Lean knowledge in the simulation process. Several authors argue the benefits of a participative or facilitated approach in simulation projects (Franco and Montibeller Citation2010; Monks, Robinson, and Kotiadis Citation2016; Robinson et al. Citation2014). This is in line with the Lean principles related to organisational learning as presented by Liker (Citation2004). Baril et al. (Citation2016) also mention this. Ramakrishnan, Testani, and Orth (Citation2009) also indicate the engagement of the participants as one of the critical factors for successful kaizen events.
Stage 4: analysis of the future research perspectives and existing challenges
After reviewing the literature about Lean and simulation, the following trends, gaps, future research opportunities and existing challenges have been identified.
Trends and future directions
The existing trends have been identified as a result of the analysis done in stages 1–3. The main trends are described in the following subsections.
Increased interest in the combination of Lean and simulation, especially in the Industry 4.0 context
An increasing interest in the link between Lean and Industry 4.0 has been identified as a key research stream by Ciano et al. (Citation2019). Additionally, the first stage of this review showed that the literature dealing with the combination of Lean and simulation is growing rapidly, especially as regards specific methods such as DES. This trend is likely to continue in the context of Industry 4.0 when many companies are proactively undergoing major technological paradigm shift towards increased degree of digitalisation and automation (Goienetxea Uriarte, Ng, and Urenda Moris Citation2018; Ruiz Zúñiga, Urenda Moris, and Syberfeldt Citation2017; Wagner, Herrmann, and Thiede Citation2017). In contrast, other methods such as mathematical modelling seem to be not showing the same positive trend.
Stage 1 also showed that the implementation of Lean and simulation is growing rapidly in BRIC countries. This trend too is likely to continue.
Increased interest in the combination of Lean and simulation with optimisation
Different techniques, methods or management philosophies have been employed in combination with Lean (Bhamu and Singh Sangwan Citation2014; Hines, Rich, and Holweg Citation2004) or in combination with simulation (Pidd Citation2012; Jahangirian et al. Citation2010). Certainly, the combination of Lean and simulation also employs variants of the previous.
As highlighted by Jahangirian et al. (Citation2010), the use of so-called ‘intelligent simulation’ or SBO is increasing. This tendency was seen in stage 1 when analysing the combination of ‘lean’, ‘simulation’ and ‘optimisation’ and was also apparent from the analysis of existing frameworks for combining Lean and DES in stage 3. Several authors have identified the benefits of combining Lean, simulation and optimisation (Goienetxea Uriarte et al. Citation2016) or have included optimisation as a step in their frameworks (Al-Aomar Citation2011; El-Haik and Al-Aomar Citation2006; Jia Citation2010). This trend will continue as the use of simulation alone to build and test different what-if scenarios requires tedious manual efforts. Whenever the problem can be formulated as an optimisation one, it is possible to use optimisation algorithms to find near-optimal configurations automatically. For this type of problem, merely running what-if scenarios on the simulation models can be considered as a waste. Furthermore, given that one of the goals of Lean is to optimise the overall flow of the value stream and minimise waste through continuous improvements, virtual factories that allow successive changes to be evaluated, guided by the SBO approach (Jain, Shao, and Shin Citation2016), will likely to be the new, innovative toolset at the disposal of any Lean practitioner.
Increased interest in the combination of Lean and simulation with Six Sigma
Some authors prefer the combination of Lean Six Sigma and simulation. This is the case for El-Haik and Al-Aomar (Citation2006), who argue that the combination of Lean and Six Sigma (LSS) offers a better approach than their individual implementation. Based on the previous reference, Lin, Jin, and Chia (Citation2014) also present a method of simulation-based LSS. Bhat, Gijo, and Jnanesh (Citation2014) apply the same approach to the analysis and improvement of the registration process at a hospital, where different Lean methods and tools including VSM, 5S, fish-bone diagrams and Gemba walk are implemented together with different statistical tools from Six Sigma. The authors follow a DMAIC process, and at each stage they identify the role of Lean or Six Sigma tools and simulation. Aqlan et al. (Citation2018) use DES to prioritise LSS projects. Huang and Klassen (Citation2016) identify the LSS tools for each step in DMAIC where the role of simulation is also identified. A similar approach is followed by Baril et al. (Citation2016) and Ramakrishnan, Testani, and Orth (Citation2009) in which simulation is employed for facilitation purposes in kaizen events and the DMAIC steps are followed. This is in line with the existing trend on combining Lean with Six Sigma (Shah, Chandrasekaran, and Linderman Citation2008). However, there are some who criticise this approach (Bicheno and Holweg Citation2009; Liker Citation2004; Pepper and Spedding Citation2010).
Increased interest in the combination of Lean and simulation with sustainability
Given the increasing importance of environmental issues and sustainable manufacturing, many authors have started to adopt the Lean and green approach (Ciano et al. Citation2019), and introduced simulation to support it. Ramakrishnan, Al-Fandi, and Chen (Citation2009) present an ‘Energy Kaizen’ framework in which different Lean methods and tools such as VSM and kaizen events are employed to detect waste in the manufacturing process of high-end servers, including a ninth waste defined as energy waste. The authors use simulation to evaluate the impact of possible improvements detected in the kaizen events, with a strong focus on reducing so-called ‘green waste’. Paju et al. (Citation2010) present a methodology called Sustainable Manufacturing Mapping in which simulation is used to evaluate current and future state maps. Similarly, Baysan, Cevikcan, and Satoglu (Citation2013) use simulation to estimate the effect of different Lean methods on energy consumption. A method using simulation to estimate the impact of Lean and green strategies is presented in Greinacher et al. (Citation2016). This is a very interesting area for further research as sustainability is and will be a key pillar in the Industry 4.0 context (Kusiak Citation2018).
Gaps and future directions
Gaps in the field and opportunities for further research were identified in stages 2 and 3 and are described in the following subsections.
Lack of review papers on Lean and simulation
Surprisingly, only a few review papers dealing with the combination of Lean and simulation were found in stage 2. Further review articles are needed to support research in the area, including case studies in specific domains (industry, construction, healthcare or education), reviews of specific simulation tools (e.g. DES, SD and ABM) combined with Lean, or specific Lean methods and tools (e.g. VSM, JIT and Kanban) combined with simulation. Such reviews would be of interest to professionals, researchers, and academics.
Lack of comprehensive frameworks of combining Lean and simulation
Different frameworks have been found in the literature and are described in stage 3. However, there is a lack of comprehensive frameworks for this combination. This has also been identified as a shortcoming by Pinho and Mendes (Citation2017) in their review about the role of IT in Lean. Buer, Strandhagen, and Chan (Citation2018) also defend the need for a framework for the integration of Industry 4.0 technologies and Lean.
However, the articles reviewed in stage 3 of this study merely provide a method or framework, which usually consists of some process flow with different steps. Still, the introduction of a new methodology into an organisation is a complex matter. There is a need for guidelines on how and when to implement the new methodology in organisations (Jasti and Kodali Citation2015). Goienetxea Uriarte, Ng, and Urenda Moris (Citation2017) present a maturity model that provides some guidelines to implement Lean and simulation in organisations. Jia (Citation2010) does address the question of the knowledge required for implementation. However, a more comprehensive analysis about when, where, how and who should employ and deploy the presented frameworks is needed.
Additionally, no framework scored high on every criterion in stage 3. Each criterion was selected as being an important factor for the implementation of the framework or method. Therefore, the development of a comprehensive framework including all the criteria detailed in stage 3 might be interesting to be addressed by future research. This will definitely support organisations on including simulation as part of the Lean toolbox, to support continuous improvement activities, as well as to introduce Lean principles in the simulation process.
Lack of evaluation of the existing frameworks of combining Lean and simulation
There are no longitudinal studies in which a framework has been implemented in an organisation and employed in many projects. It appears that the papers which present specific case studies only tried the method for that one case, creating isolated cases where the developed framework will not necessarily be employed in the future. An exception is the article by Ramakrishnan, Testani, and Orth (Citation2009) in which the authors state that their framework has been applied in over twenty kaizen events within a two-year period.
Additionally, there is no evidence of the measurement of the performance of the proposed frameworks in real-world situations. Most of the articles test their proposed framework in specific single-case studies. However, they do not present any evaluation of its usability or performance, which we suggest should be addressed by more research.
Lack of the combined use of Lean and simulation for educational purposes
There is a lack of methods for educational purposes in which DES is employed to teach Lean. Taking into account that virtual factories are one of the key notions within Industry 4.0 (Mrugalska and Wyrwicka Citation2017), the role of the engineers will be more and more tending towards testing design and improvement possibilities in virtual environments. Therefore, the engineering curriculum would benefit by using techniques such as DES to teach and test Lean concepts. This presents an opportunity for future researchers and lecturers.
Lack of the combination of Lean and simulation in the entire lifecycle of the system
When it comes to the purpose for combining simulation and Lean, most of the articles focus on evaluation, mainly on the use of simulation to support the design stages of a system. Research on the required methods as well as empirical studies are needed on the use of simulation even to support Lean continuous improvement events, both to facilitate discussions and evaluate different improvement alternatives. Promoting, therefore, the extended use of simulation models through the different steps of the lifecycle of the system where Lean and simulation models are employed in the initial design stages, but the simulation models are also continuously updated in later stages to support continuous improvement.
Not taking into account Lean principles in the simulation process
Most of the articles treat Lean as a set of tools and ignore the principles underlying its management philosophy. The Lean tools are used as an input to or output from a simulation model. However, no consideration is given to how the Lean philosophy can support the simulation process. There is a need to address this aspect and include Lean principles (Liker Citation2004), especially those related to organisational learning, in the simulation process. It is also vital to take these principles into account even when defining the objectives to achieve via the simulation study.
Existing challenges
Different challenges have to be overcome for a successful combination of Lean and simulation and its adoption by different kind of organisations.
Lean alone presents some challenges for its implementation as discussed by different authors (Deflorin and Scherrer-Rathje Citation2012; Hines, Rich, and Holweg Citation2004). Others have analysed the existing challenges for the use of simulation in the industrial context (Fowler and Rose Citation2004) or healthcare context (Young et al. Citation2009). Some barriers to the combination of Lean and simulation are identified by Goienetxea Uriarte et al. (Citation2016). The following subsections analyse the existing technical, organisational, as well as people and culture related challenges for the combination.
Technical challenges on the combination of Lean and simulation
Taking into account that both Lean and simulation will be important components in the Industry 4.0 context, an integrated link between them will be essential to better support organisations in system design and improvement. A challenge will be to adapt the existing simulation software tools to support a higher integration with Lean tools and principles, not just limited to the inclusion of VSM as an input or output from the simulation model.
In the Industry 4.0 context, considerable advances are expected towards a robust simulation infrastructure to support decision-making in different levels of the organisation (Goienetxea Uriarte, Ng, and Urenda Moris Citation2018) with ‘real-time simulation-based problem-solving capability’ and ‘synchronized factory models’ (Fowler and Rose Citation2004). However, the high-level of expertise and the amount of time that is still required to develop simulation models (Diamond et al. Citation2002) does not facilitate the inclusive approach defended by Lean practitioners, neither does support the extended use of simulation. This is a challenge that should be addressed mainly by the simulation software companies towards easier-to-use platforms.
Additionally, to be able to overcome the challenge of the required competence to build simulation models, higher education institutions should include simulation as a regular tool within the engineering curricula. This education should ensure a clear focus on teaching Lean system configurations via simulation.
Organisational challenges on the combination of Lean and simulation
An adaptation of the organisational structures will be needed to embrace a closer collaboration between Lean and simulation practitioners. To depend on external resources or highly specialised internal departments to develop simulation models in an expert mode (Franco and Montibeller Citation2010) is not sustainable and does not provide organisational learning as defended by the Lean principles (Goienetxea Uriarte et al. Citation2016).
The existing working standards should also be adapted towards a more inclusive link between different Lean methods and tools and simulation (e.g. kaizen or VSM events including simulation, standard activities in system design should include different Lean tools and simulation, etc.). The periodical meetings to discuss system design and improvements should even include people with Lean and simulation knowledge.
On the other hand, both Lean and simulation have been used with more or less success in large organisations. However, small and medium enterprises (SMEs) may find the implementation of their combination still challenging, because Lean is still not that successfully implemented in these (Knol et al. Citation2018; McGovern, Small, and Hicks Citation2017; Pearce, Pons, and Neitzert Citation2018; Shah and Ward Citation2003), and simulation may also be rather new to them (Ivers, Byrne, and Byrne Citation2016).
An additional challenge to address is on whether the combination of Lean and simulation is applicable to different organisations and domains. The literature analysis in this paper shows that the approach has been employed in different domains, i.e. industry, healthcare, construction, and education. However, the discussion on the applicability of Lean in the contexts with high demand variation is open (Hines, Rich, and Holweg Citation2004), especially when its methods and tools do not include any consideration to variation. Therefore, it seems that simulation, by providing the possibility of a better analysis including system variation, can possibly make Lean concepts more applicable, particularly in environments where it previously was not considered as such.
People and culture related challenges on the combination of Lean and simulation
Any change in the working routines, the introduction of new tools, or combination of tools has a natural positive or negative reaction by the people affected by the change. Negative reactions may have different causes and represent a challenge to overcome by the organisations trying to introduce those new routines or tools. The implementation of the combination of Lean and simulation will not be an exception. Goienetxea Uriarte et al. (Citation2016) identified the barriers that may impact how this combination is implemented, those related to personal or cultural challenges are: (1) problems with a generation gap with senior Lean practitioners not believing in simulation results; (2) previous negative experiences with Lean or simulation projects; or (3) having fear of losing the Gemba when virtual tools are employed instead of having a trial and error in the real-world.
An additional challenge to overcome may be an opposition to implement a management philosophy, methods and tools initially developed for the industrial domain, in non-industrial domains.
Conclusions and future work
Lean is considered to be the most popular management philosophy and it will still be actual in the Industry 4.0 context. Simulation is, at the same time, one of the key technologies in this new industrial revolution. Taking into account that different authors have defended the benefits of combining Lean and simulation, this article presented a four-stage review of the literature on their combination. To the best of the authors’ knowledge, this is the first comprehensive review conducted in the combination of Lean and simulation.
The evolution of the combination of Lean and simulation is analysed in stage 1, showing considerable growing interest in the area, especially in the last two decades. Existing reviews of the literature are presented in stage 2, and a lack of reviews in the area is identified as an opportunity for further research. Frameworks and methodologies for the combination are classified and described in stage 3. These frameworks are analysed in terms of (1) domain; (2) purpose of the combination; (3) level of comprehensiveness and way of combination of Lean and simulation; (4) clarity in structure and (5) role definition. Specific comments are also made on each framework.
The existing research perspectives and challenges are identified in stage 4. The main trends identified are the increased interest in the combination of Lean and simulation in the Industry 4.0 context and in their combination with optimisation, Six Sigma, as well as sustainability. The number of articles in these areas is likely to continue to grow.
Gaps and future research opportunities are also highlighted, including: (1) lack of reviews on the combination of Lean and simulation focused on the application domain, Lean tools, or specific types of simulation; (2) lack of comprehensive frameworks in the combination of Lean and simulation; (3) lack of framework performance and usability evaluations; (4) lack of the combined use of Lean and simulation for educational purposes; (5) lack of the combination of Lean and simulation in the entire lifecycle of the system; and (6) not taking into account Lean principles in the simulation process.
Additionally, technical, organisational, as well as people and culture related challenges on the combination of Lean and simulation have also been discussed. The technical challenges include the need for a higher integration of Lean tools and principles in the existing simulation software, as well as a need for easier-to-use simulation platforms to facilitate its extended use among Lean practitioners. The organisational challenges include the need for an adaptation of organisational structures and existing working standards to fully benefit from the combination. The implementation of the combination will also be challenging for SMEs, where both Lean and simulation are not commonly employed, and for organisations with high demand variation, which not necessarily consider Lean as an applicable philosophy. In regard to the people and culture related challenges, the reaction to change the traditional way of working will need to be handled by the organisations wanting to implement the combination of Lean and simulation. This will be especially critical in non-industrial domains.
Given that the literature reviewed relates to a number of domains (industry, construction, healthcare, and education) and purposes (evaluation, facilitation, and education), this article should be of interest to many professionals, academics and researchers working with Lean and/or simulation. The list of specific frameworks provided in stage 3 may be useful to both large organisations and SMEs.
As regards the methodology employed in this review, the authors believe that the combined use of text mining and the filtering tools available in databases allows for a more effective search of the relevant articles.
In future work, we would like to extend this study to analyse how Lean and simulation are used to support organisational decision-making processes, particularly in relation to real-world cases reported in the literature.
Acknowledgments
The authors gratefully acknowledge the Swedish Knowledge Foundation (KK-Stiftelsen) and the School of Engineering Science at the University of Skövde, for their financial support.
Disclosure statement
No potential conflict of interest was reported by the authors.
ORCID
Ainhoa Goienetxea Uriarte http://orcid.org/0000-0003-4604-6429
Amos H.C. Ng http://orcid.org/0000-0003-0111-1776
Correction Statement
This article has been republished with minor changes. These changes do not impact the academic content of the article.
Additional information
Funding
References
- Abdulmalek, F. A., and J. Rajgopal. 2007. “Analyzing the Benefits of Lean Manufacturing and Value Stream Mapping Via Simulation: A Process Sector Case Study.” International Journal of Production Economics 107 (1): 223–236. doi:10.1016/j.ijpe.2006.09.009.
- Abo-Hamad, W., J. Crowe, and A. Arisha. 2012. “Towards Leaner Healthcare Facility: Application of Simulation Modelling and Value Stream Mapping.” 1st international workshop on innovative simulation for health care, IWISH 2012, International multidisciplinary modeling and simulation multiconference, I3M 2012, Vienna.
- Al-Aomar, R. 2011. “Handling Multi-Lean Measures With Simulation and Simulated Annealing.” Journal of the Franklin Institute 348 (7): 1506–1522. doi:10.1016/j.jfranklin.2010.05.002.
- Al-Fandi, L., S. S. Lam, and S. Ramakrishnan. 2011. “A Framework to Reduce Problem Complexity Using Lean Concepts with Simulation.” 61st annual conference and expo of the institute of industrial engineers, Reno, NV.
- Al-Sudairi, A. A. 2007. “Evaluating the Effect of Construction Process Characteristics to the Applicability of Lean Principles.” Construction Innovation 7 (1): 99–121. doi:10.1108/14714170710721322.
- Ali, N. B., K. Petersen, and B. B. N. De França. 2015. “Evaluation of Simulation-Assisted Value Stream Mapping for Software Product Development: Two Industrial Cases.” Information and Software Technology 68: 45–61. doi:10.1016/j.infsof.2015.08.005.
- Anand, G., and R. Kodali. 2010a. “Analysis of Lean Manufacturing Frameworks.” Journal of Advanced Manufacturing Systems 9 (1): 1–30. doi:10.1142/S0219686710001776.
- Anand, G., and R. Kodali. 2010b. “Development of a Framework for Implementation of Lean Manufacturing Systems.” International Journal of Management Practice 4 (1): 95–116. doi:10.1504/IJMP.2010.029705.
- April, J., F. Glover, J. P. Kelly, and M. Laguna. 2003. “Practical Introduction to Simulation Optimization.” Proceedings of the 2003 winter simulation conference: Driving innovation, New Orleans, LA.
- Aqlan, F., S. Ramakrishnan, L. Al-Fandi, and C. Saha. 2018. “A Framework for Selecting and Evaluating Process Improvement Projects Using Simulation and Optimization Techniques.” 2017 winter simulation conference, WSC 2017.
- Atieh, A. M., H. Kaylani, A. Almuhtady, and O. Al-Tamimi. 2016. “A Value Stream Mapping and Simulation Hybrid Approach: Application to Glass Industry.” International Journal of Advanced Manufacturing Technology 84 (5–8): 1573–1586. doi:10.1007/s00170-015-7805-8.
- Badurdeen, F., P. Marksberry, A. Hall, and B. Gregory. 2010. “Teaching Lean Manufacturing with Simulations and Games: A Survey and Future Directions.” Simulation and Gaming 41 (4): 465–486. doi:10.1177/1046878109334331.
- Bal, A., C. Ceylan, and C. Taçoğlu. 2017. “Using Value Stream Mapping and Discrete Event Simulation to Improve Efficiency of Emergency Departments.” International Journal of Healthcare Management 10 (3): 196–206. doi:10.1080/20479700.2017.1304323.
- Banks, J., J. S. Carson, II, B. L. Nelson, and D. M. Nicol. 2014. Discrete-Event Simulation. 5th ed. England: Pearson Education.
- Baril, C., V. Gascon, J. Miller, and N. Côté. 2016. “Use of a Discrete-Event Simulation in a Kaizen Event: A Case Study in Healthcare.” European Journal of Operational Research 249 (1): 327–339. doi:10.1016/j.ejor.2015.08.036.
- Barman, S., M. D. Hanna, and R. L. LaForge. 2001. “Perceived Relevance and Quality of POM Journals: A Decade Later.” Journal of Operations Management 19 (3): 367–385. doi:10.1016/S0272-6963(00)00060-7.
- Baysan, S., E. Cevikcan, and ŞI Satoglu. 2013. “Assessment of Energy Efficiency in Lean Transformation: A Simulation Based Improvement Methodology.” Green Energy and Technology 23. doi:10.1007/978-1-4471-5143-2_18.
- Ben-Alon, L., and R. Sacks. 2015. “Simulating and Vizualising Emergent Production in Construction (Epic) Using Agents and BIM.” Proceedings of IGLC 23, 23rd annual conference of the international group for lean construction: Global knowledge – global solutions.
- Bhamu, J., and K. Singh Sangwan. 2014. “Lean Manufacturing: Literature Review and Research Issues.” International Journal of Operations & Production Management 34 (7): 876–940. doi:10.1108/IJOPM-08-2012-0315.
- Bhat, S., E. V. Gijo, and N. A. Jnanesh. 2014. “Application of Lean Six Sigma Methodology in the Registration Process of a Hospital.” International Journal of Productivity and Performance Management 63 (5): 613–643. doi:10.1108/IJPPM-11-2013-0191.
- Bicheno, J., and M. Holweg. 2009. The Lean Toolbox: The Essential Guide to Lean Transformation. 4th ed. Buckingham: PICSIE Books.
- Borshchev, A. 2014. “Multi-method Modelling: AnyLogic.” In Discrete Event Simulation and System Dynamics for Management Decision Making, edited by S. Brailsford, L. Churilov, and B. Dangerfield, 248–279. Chichester: Wiley.
- Brailsford, S. C., P. R. Harper, B. Patel, and M. Pitt. 2009. “An Analysis of the Academic Literature on Simulation and Modelling in Health Care.” Journal of Simulation 3 (3): 130–140. doi:10.1057/jos200910.
- Buer, S. V., J. O. Strandhagen, and F. T. S. Chan. 2018. “The Link Between Industry 4.0 and Lean Manufacturing: Mapping Current Research and Establishing a Research Agenda.” International Journal of Production Research 56 (8): 2924–2940. doi:10.1080/00207543.2018.1442945.
- Chattinnawat, W. 2016. “Application of Material Flow Cost Accounting Analysis to Increase Pajama Production Efficiency: A Case Study of Confederate International Co., Ltd.” 6th international conference on industrial engineering and operations management in Kuala Lumpur, IEOM 2016.
- Chay, T. F., Y. C. Xu, A. Tiwari, and F. Chay. 2015. “Towards Lean Transformation: The Analysis of Lean Implementation Frameworks.” Journal of Manufacturing Technology Management 26 (7): 1031–1052. doi:10.1108/JMTM-10-2013-0143.
- Chu, C. H., and W. L. Shih. 1992. “Simulation Studies in Jit Production.” International Journal of Production Research 30 (11): 2573–2586. doi:10.1080/00207549208948177.
- Chwif, L., and M. Barretto. 2003. “Simulation Models as an Aid for the Teaching and Learning Process in Operations Management.” Proceedings of the 2003 winter simulation conference, December 7–10.
- Ciano, M. P., R. Pozzi, T. Rossi, and F. Strozzi. 2019. “How IJPR has Addressed ‘Lean’: A Literature Review Using Bibliometric Tools.” International Journal of Production Research, In press. doi:10.1080/00207543.2019.1566667.
- Constantino Delago, L., M. E. F. H. S. Machado, F. Oliveira de Brito, G. Casarini Landgraf, M. de Andrade Shcroeder, and C. Torezzan. 2016. “Learning Lean Philosophy Through 3D Game-Based Simulation.” Proceedings of the 2016 winter simulation conference, December 11–14.
- Cudney, E. A., S. M. Corns, J. A. Farris, S. Gent, S. E. Grasman, and I. G. Guardiola. 2011. “Enhancing Undergraduate Engineering Education of Lean Methods Using Simulation Learning Modules Within a Virtual Environment.” ASEE annual conference and exposition, conference proceedings.
- Da Silva, T. M., and J. C. E. Ferreira. 2014. “Value Stream Mapping and Discrete Event Simulation Applied to Reduce Waste in a Company That Manufactures a Family of Automotive Parts.” 24th international conference on flexible automation and intelligent manufacturing, FAIM 2014.
- Deb, K. 2001. Multi-Objective Optimization Using Evolutionary Algorithms. 3rd ed. Wiley-Interscience Series in Systems and Optimization. Wiltshire: Wiley.
- Deflorin, P., and M. Scherrer-Rathje. 2012. “Challenges in the Transformation to Lean Production from Different Manufacturing-Process Choices: A Path-Dependent Perspective.” International Journal of Production Research 50 (14): 3956–3973. doi:10.1080/00207543.2011.613862.
- Deif, A. 2010. “Computer Simulation to Manage Lean Manufacturing Systems.” 2010 2nd international conference on computer engineering and technology, ICCET 2010, Chengdu.
- de Jong, S. J., and W. W. A. Beelaerts van Blokland. 2016. “Measuring Lean Implementation for Maintenance Service Companies.” International Journal of Lean Six Sigma 7 (1): 35–61. doi:10.1108/IJLSS-12-2014-0039.
- De Oliveira Gomes, V. E., and L. G. Trabasso. 2016. “A Proposal Simulation Method Towards Continuous Improvement in Discrete Manufacturing.” 49th CIRP conference on manufacturing systems, CIRP-CMS 2016.
- Diamond, R., C. R. Harrell, J. O. Henriksen, W. B. Nordgren, C. Dennis Pegden, M. W. Rohrer, A. P. Waller, and A. M. Law. 2002. “The Current and Future Status of Simulation Software (Panel).” Proceedings of the 2002 winter simulation conference, San Diego, CA.
- El-Haik, B., and R. Al-Aomar. 2006. Simulation-Based Lean Six-Sigma and Design for Six-Sigma. Hoboken, NJ: Wiley.
- Esquenazi, A., and R. Sacks. 2006. “Evaluation of Lean Improvements in Residential Construction Using Computer Simulation.” 14th annual conference of the international group for lean construction, IGLC-14, Santiago.
- Farrar, J. M., S. M. Abourizk, and X. Mao. 2004. “Generic Implementation of Lean Concepts in Simulation Models.” Lean Construction Journal 1 (October): 1–23.
- Ferrin, D. M., M. J. Miller, and D. Muthler. 2005. “Lean Sigma and Simulation, So What’s the Correlation? V2.” Proceedings of the 2005 winter simulation conference. Orlando, FL.
- Figueira, G., and B. Almada-Lobo. 2014. “Hybrid Simulation-Optimization Methods: A Taxonomy and Discussion.” Simulation Modelling Practice and Theory 46: 118–134.
- Fontanini, P. S. P., F. A. Picchi, S. A. Loureiro, O. F. Lima, Jr., and A. T. Folch. 2008. “Simulating a Construction Supply Chain: Preliminary Case Study of Pre-Cast Elements.” 16th annual conference of the international group for lean construction, IGLC16, Manchester.
- Fowler, J. W., and O. Rose. 2004. “Grand Challenges in Modeling and Simulation of Complex Manufacturing Systems.” Simulation 80 (9): 469–476. doi:10.1177/0037549704044324.
- Franco, L. A., and G. Montibeller. 2010. “Facilitated Modelling in Operational Research.” European Journal of Operational Research 205 (3): 489–500. doi:10.1016/j.ejor.2009.09.030.
- Gaddi, M., N. Garbellini, and F. Garibaldo. 2017. Industry 4.0 and Its Consequences for Work and Labour. Bologna: Fondazione Claudio Sabattini.
- Garza-Reyes, J. A., I. Oraifige, H. Soriano-Meier, P. L. Forrester, and D. Harmanto. 2012. “The Development of a Lean Park Homes Production Process Using Process Flow and Simulation Methods.” Journal of Manufacturing Technology Management 23 (2): 178–197. doi:10.1108/17410381211202188.
- Goienetxea Uriarte, A., A. H. C. Ng, and M. Urenda Moris. 2017. “Lean, Simulation and Optimization: A Maturity Model.” International conference on industrial engineering and engineering management, IEEE IEEM 2017, Singapore.
- Goienetxea Uriarte, A., A. H. C. Ng, and M. Urenda Moris. 2018. “Supporting the Lean Journey with Simulation and Optimization in the Context of Industry 4.0.” Procedia Manufacturing 25: 586–593. doi:10.1016/j.promfg.2018.06.097.
- Goienetxea Uriarte, A., T. Sellgren, A. H. C. Ng, and M. Urenda Moris. 2019. “Introducing Simulation and Optimization in the Lean Continuous Improvement Standards in an Automotive Company.” Winter simulation conference, WSC 2018, Gothenburg.
- Goienetxea Uriarte, A., M. Urenda Moris, A. H. C. Ng, and J. Oscarsson. 2016. “Lean, Simulation and Optimization: A Win-Win Combination.” Winter simulation conference, WSC 2015, Huntington Beach, CA.
- Goldsman, D., R. E. Nance, and J. R. Wilson. 2010. “A Brief History of Simulation Revisited.” Winter simulation conference, WSC 2010, Baltimore, MD.
- Greinacher, S., E. Moser, J. Freier, J. Müller, and G. Lanza. 2016. “Simulation-Based Methodology for the Application of Lean and Green Strategies Depending on External Change Driver Influence.” 23rd CIRP conference on life cycle engineering, LCE 2016.
- Gurumurthy, A., and R. Kodali. 2011. “Design of Lean Manufacturing Systems Using Value Stream Mapping with Simulation: A Case Study.” Journal of Manufacturing Technology Management 22 (4): 444–473. doi:10.1108/17410381111126409.
- Hines, P., N. Rich, and M. Holweg. 2004. “Learning to Evolve: A Review of Contemporary Lean Thinking.” International Journal of Operations & Production Management 24 (10): 994–1011. doi:10.1108/01443570410558049.
- Hirsch-Kreinsen, H. 2016. “Industry 4.0” as Promising Technology: Emergence, Semantics and Ambivalent Character. Sociological Working Papers. Vol. 48. Dortmund: Technical University of Dortmund.
- Hobbs, D. P. 2004. Lean Manufacturing Implementation: A Complete Execution Manual for Any Size Manufacturer. Boca Ratón: J. Ross Pub.
- Hosseini, S. A. A., A. Nikakhtar, K. Y. Wong, and A. Zavichi. 2012. “Implementing Lean Construction Theory Into Construction Processes’ Waste Management.” International conference on sustainable design and construction 2011: Integrating sustainability practices in the construction industry, ICSDC 2011, Kansas City, MO.
- Huang, Y., and K. J. Klassen. 2016. “Using Six Sigma, Lean, and Simulation to Improve the Phlebotomy Process.” Quality Management Journal 23 (2): 6–21. doi:10.1080/10686967.2016.11918468.
- IMTR. 2000. Integrated Manufacturing Technology Roadmapping Project: Modeling & Simulation. Tennessee: Integrated Manufacturing Technology Initiative Inc.
- Ivers, A. M., J. Byrne, and P. J. Byrne. 2016. “Analysis of SME Data Readiness: A Simulation Perspective.” Journal of Small Business and Enterprise Development 23 (1): 163–188. doi:10.1108/JSBED-03-2014-0046.
- Jahangirian, M., T. Eldabi, A. Naseer, L. K. Stergioulas, and T. Young. 2010. “Simulation in Manufacturing and Business: A Review.” European Journal of Operational Research 203 (1): 1–13. doi:10.1016/j.ejor.2009.06.004.
- Jain, S., D. Lechevalier, J. Woo, and S. J. Shin. 2016. “Towards a Virtual Factory Prototype.” Winter simulation conference, WSC 2015, Huntington Beach, CA.
- Jain, S., G. Shao, and S. J. Shin. 2016. “Virtual Factory Based Data Analytics.” IEEE international conference on control and automation, Kathmandu, Nepal.
- Jarkko, E., W. Lu, S. Lars, and O. Thomas. 2013. “Discrete Event Simulation Enhanced Value Stream Mapping: An Industrialized Construction Case Study.” Lean Construction Journal 2013: 47–65.
- Jasti, N. V. K., and R. Kodali. 2015. “Lean Production: Literature Review and Trends.” International Journal of Production Research 53 (3): 867–885. doi:10.1080/00207543.2014.937508.
- Jeong, W., S. Chang, J. W. Son, and J. S. Yi. 2016. “BIM-Integrated Construction Operation Simulation for Just-in-Time Production Management.” Sustainability (Switzerland) 8: 11. doi:10.3390/su8111106.
- Jia, Y. 2010. “SimLean: A Reference Framework for Embedding Simulation in Lean Projects.” Ph.D. Thesis., Sheffield Hallam University.
- Jia, Y., and T. Perera. 2009. “Embedding Simulation in Lean Projects.” 7th international industrial simulation conference, ISC 2009, Loughborough.
- Kaylani, H., A. Almuhtady, and A. M. Atieh. 2016. “Novel Approach to Enhance the Performance of Production Systems Using Lean Tools.” Jordan Journal of Mechanical and Industrial Engineering 10 (3): 215–229.
- Khadem, M., S. A. Ali, and H. Seifoddini. 2008. “Efficacy of Lean Metrics in Evaluating the Performance of Manufacturing Systems.” International Journal of Industrial Engineering: Theory Applications and Practice 15 (2): 176–184.
- Knol, W. H., J. Slomp, R. L. J. Schouteten, and K. Lauche. 2018. “Implementing Lean Practices in Manufacturing SMEs: Testing ‘Critical Success Factors’ Using Necessary Condition Analysis.” International Journal of Production Research 56 (11): 3955–3973. doi:10.1080/00207543.2017.1419583.
- Koulouriotis, D. E., A. S. Xanthopoulos, and V. D. Tourassis. 2010. “Simulation Optimisation of Pull Control Policies for Serial Manufacturing Lines and Assembly Manufacturing Systems Using Genetic Algorithms.” International Journal of Production Research 48 (10): 2887–2912. doi:10.1080/00207540802603759.
- Kusiak, A. 2018. “Smart Manufacturing.” International Journal of Production Research 56 (1-2): 508–517. doi:10.1080/00207543.2017.1351644.
- Law, A. M. 2007. Simulation Modeling and Analysis. 4th ed. New York: McGraw-Hill.
- Lian, Y. H., and H. Van Landeghem. 2007. “Analysing the Effects of Lean Manufacturing Using a Value Stream Mapping-Based Simulation Generator.” International Journal of Production Research 45 (13): 3037–3058. doi:10.1080/00207540600791590.
- Liker, J. K. 1996. Becoming Lean. Portland: Productivity Press.
- Liker, J. K. 2004. The Toyota Way: 14 Management Principles from the World’s Greatest Manufacturer. Madison, WI: McGraw-Hill.
- Lin, W. D., X. Jin, and S. Y. Chia. 2014. “Simulation Based Lean Six Sigma Approach to Reduce Patients Waiting Time in an Outpatient Eye Clinic.” IEEE international conference on industrial engineering and engineering management.
- Liu, Y. S., L. N. Tang, Y. Z. Ma, and T. Yang. 2018. “TFT-LCD Module Cell Layout Design Using Simulation and Fuzzy Multiple Attribute Group Decision-Making Approach.” Applied Soft Computing 68: 873–888. doi:10.1016/j.asoc.2017.10.026.
- Lu, J. C., T. Yang, and C. Y. Wang. 2011. “A Lean Pull System Design Analysed by Value Stream Mapping and Multiple Criteria Decision-Making Method Under Demand Uncertainty.” International Journal of Computer Integrated Manufacturing 24 (3): 211–228. doi:10.1080/0951192X.2010.551283.
- Ma, L., and R. Sacks. 2016. “Agent-Based Simulation of Construction Workflows Using a Relational Data Model.” 24th annual conference of the international group for lean construction, IGLC 2016.
- Mao, X., and X. Zhang. 2008. “Construction Process Reengineering by Integrating Lean Principles and Computer Simulation Techniques.” Journal of Construction Engineering and Management 134 (5): 371–381. doi:10.1061/(ASCE)0733–9364(2008)134:5(371).
- Marvel, J. H., and C. R. Standridge. 2009. “Simulation-Enhanced Lean Design Process.” Journal of Industrial Engineering and Management 2 (1): 90–113.
- McGovern, T., A. Small, and C. Hicks. 2017. “Diffusion of Process Improvement Methods in European SMEs.” International Journal of Operations & Production Management 37 (5): 607–629. doi:10.1108/IJOPM-11-2015-0694.
- Meyer, A., and B. Amberg. 2018. “Transport Concept Selection Considering Supplier Milk Runs: An Integrated Model and a Case Study from the Automotive Industry.” Transportation Research Part E: Logistics and Transportation Review 113: 147–169. doi:10.1016/j.tre.2017.07.004.
- Mielczarek, B., and J. Uziałko-Mydlikowska. 2012. “Application of Computer Simulation Modeling in the Health Care Sector: A Survey.” Simulation 88 (2): 197–216. doi:10.1177/0037549710387802.
- Mirdad, W. K., and C. I. Eseonu. 2015. “A Conceptual Map of the Lean Nomenclature: Comparing Expert Classification to the Lean Literature.” EMJ – Engineering Management Journal 27 (4): 188–202. doi:10.1080/10429247.2015.1082068.
- Moghadam, M., B. Barkokebas, and M. Al-Hussein. 2014. “Post-Simulation Visualization Application for Production Improvement of Modular Construction Manufacturing.” 31st international symposium on automation and robotics in construction and mining, ISARC 2014.
- Mohamad, E., M. A. Ibrahim, A. S. Shibghatullah, M. A. A. Rahman, M. A. Sulaiman, A. A. A. Rahman, S. Abdullah, and M. R. Salleh. 2016. “A Simulation-Based Approach for Lean Manufacturing Tools Implementation: A Review.” ARPN Journal of Engineering and Applied Sciences 11 (5): 3400–3406.
- Mongeon, P., and A. Paul-Hus. 2016. “The Journal Coverage of Web of Science and Scopuss: A Comparative Analysis.” Scientometrics 106: 213–228. doi:10.1007/s11192-015-1765-5.
- Monks, T., S. Robinson, and K. Kotiadis. 2016. “Can Involving Clients in Simulation Studies Help Them Solve Their Future Problems? A Transfer of Learning Experiment.” European Journal of Operational Research 249 (3): 919–930. doi:10.1016/j.ejor.2015.08.037.
- Mostafa, S., N. Chileshe, and T. Abdelhamid. 2016. “Lean and Agile Integration Within Offsite Construction Using Discrete Event Simulation: A Systematic Literature Review.” Construction Innovation 16 (4): 483–525. doi:10.1108/CI-09-2014-0043.
- Mrugalska, B., and M. K. Wyrwicka. 2017. “Towards Lean Production in Industry 4.0.” Procedia Engineering 182: 466–473.
- Neeraj, A., Z. K. Rybkowski, J. L. Fernández-Solís, R. C. Hill, C. Tsao, B. Seed, and D. Heinemeier. 2016. “Framework Linking Lean Simulations to Their Applications on Construction Projects.” 24th annual conference of the international group for lean construction, IGLC 2016.
- Negahban, A., and J. S. Smith. 2014. “Simulation for Manufacturing System Design and Operation: Literature Review and Analysis.” Journal of Manufacturing Systems 33 (2): 241–261. doi:10.1016/j.jmsy.2013.12.007.
- Olson, J. E. 2005. “Top-25-Business-School Professors Rate Journals in Operations Management and Related Fields.” Interfaces 35 (4): 323–338. doi:10.1287/inte.1050.0149.
- Omogbai, O., and K. Salonitis. 2016. “Manufacturing System Lean Improvement Design Using Discrete Event Simulation.” Procedia CIRP 57: 195–200.
- O’Neil, J. 2001. “Building Better Global Economic BRICs.” Global Economics 66: 1–16.
- Paju, M., J. Heilala, M. Hentula, A. Heikkilä, B. Johansson, S. Leong, and K. Lyons. 2010. “Framework and Indicators for a Sustainable Manufacturing Mapping Methodology.” Proceedings – winter simulation conference.
- Pearce, A., D. Pons, and T. Neitzert. 2018. “Implementing Lean: Outcomes from SME Case Studies.” Operations Research Perspectives 5: 94–104. doi:10.1016/j.orp.2018.02.002.
- Pepper, M. P. J., and T. A. Spedding. 2010. “The Evolution of Lean Six Sigma.” International Journal of Quality & Reliability Management 27 (2): 138–155.
- Pidd, M. 2012. “Mixing Other Methods With Simulation Is Not Big Deal.” Winter simulation conference, Berlin.
- Pinho, C., and L. Mendes. 2017. “IT in Lean-Based Manufacturing Industries: Systematic Literature Review and Research Issues.” International Journal of Production Research 55 (24): 7524–7540. doi:10.1080/00207543.2017.1384585.
- Poshdar, M., V. A. González, M. O’Sullivan, M. Shahbazpour, C. G. Walker, and H. Golzarpoor. 2016. “The Role of Conceptual Modeling in Lean Construction Simulation.” 24th annual conference of the international group for lean construction, IGLC 2016.
- Ramakrishnan, S., L. Al-Fandi, and J. Chen. 2009. “A Simulation Based Framework to Study the Impact of Lean Techniques on Green Supply Chains.” 30th annual national conference of the American society for engineering management 2009, ASEM 2009.
- Ramakrishnan, S., M. V. Testani, and R. Orth. 2009. “Critical Factors for Sustainable Kaizen Events: People, Process and Technology.” 30th annual national conference of the American society for engineering management 2009, ASEM 2009, Springfield, MO.
- Rane, A. B., D. S. S. Sudhakar, V. K. Sunnapwar, and S. Rane. 2015. “Improving the Performance of Assembly Line: Review with Case Study.” 2015 International conference on nascent technologies in the engineering field, ICNTE 2015.
- Robinson, S. 2005. “Discrete-Event Simulation: From the Pioneers to the Present, What Next?” Journal of the Operational Research Society 56 (6): 619–629. doi:10.1057/palgrave.jors.2601864.
- Robinson, S., Z. J. Radnor, N. Burgess, and C. Worthington. 2012. “SimLean: Utilising Simulation in the Implementation of Lean in Healthcare.” European Journal of Operational Research 219 (1): 188–197.
- Robinson, S., C. Worthington, N. Burgess, and Z. J. Radnor. 2014. “Facilitated Modelling With Discrete-Event Simulation: Reality or Myth?” European Journal of Operational Research 234 (1): 231–240. doi:10.1016/j.ejor.2012.12.024.
- Ruiz Zúñiga, E., M. Urenda Moris, and A. Syberfeldt. 2017. “Integrating Simulation-Based Optimization, Lean, and the Concepts of Industry 4.0.” Winter simulation conference, Las Vegas.
- Sabaghi, M., R. Rostamzadeh, and C. Mascle. 2015. “Kanban and Value Stream Mapping Analysis in Lean Manufacturing Philosophy Via Simulation: A Plastic Fabrication (Case Study).” International Journal of Services and Operations Management 20 (1): 118–140. doi:10.1504/IJSOM.2015.065977.
- Samant, S., V. K. Mittal, and R. Prakash. 2018. “Resource Optimisation for an Automobile Chassis Manufacturer Through Value Stream Mapping Enhanced with Simulation Technique and Constraint Programming.” International Journal of Industrial and Systems Engineering 28 (3): 379–401. doi:10.1504/IJISE.2018.089746.
- Schmidtke, D., U. Heiser, and O. Hinrichsen. 2014. “A Simulation-Enhanced Value Stream Mapping Approach for Optimisation of Complex Production Environments.” International Journal of Production Research 52 (20): 6146–6160. doi:10.1080/00207543.2014.917770.
- Shah, R., A. Chandrasekaran, and K. Linderman. 2008. “In Pursuit of Implementation Patterns: The Context of Lean and Six Sigma.” International Journal of Production Research 46 (23): 6679–6699.
- Shah, R., and P. T. Ward. 2003. “Lean Manufacturing: Context, Practice Bundles, and Performance.” Journal of Operations Management 21 (2): 129–149. doi:10.1016/S0272-6963(02)00108-0.
- Shannon, P. W., K. R. Krumwiede, and J. N. Street. 2010. “Using Simulation to Explore Lean Manufacturing Implementation Strategies.” Journal of Management Education 34 (2): 280–302. doi:10.1177/1052562909358964.
- Smith, J. S. 2003. “Survey on the Use of Simulation for Manufacturing System Design and Operation.” Journal of Manufacturing Systems 22 (2): 157–171.
- Solding, P., and P. Gullander. 2009. “Concepts for Simulation Based Value Stream Mapping.” 2009 winter simulation conference, WSC 2009, Austin, TX.
- Standridge, C. R., and J. H. Marvel. 2006. “Why Lean Needs Simulation.” 2006 winter simulation conference, WSC, Monterey, CA.
- Tekin, E., and I. Sabuncuoglu. 2004. “Simulation Optimization: A Comprehensive Review on Theory and Applications.” IIE Transactions (Institute of Industrial Engineers) 36 (11): 1067–1081. doi:10.1080/07408170490500654.
- Theoharakis, V., C. Voss, G. C. Hadjinicola, and A. C. Soteriou. 2007. “Insights Into Factors Affecting Production and Operations Management (POM) Journal Evaluation.” Journal of Operations Management 25 (4): 932–955. doi:10.1016/j.jom.2006.09.002.
- Tilson, V., G. Dobson, C. E. Haas, and D. Tilson. 2014. “Mathematical Modeling to Reduce Waste of Compounded Sterile Products in Hospital Pharmacies.” Hospital Pharmacy 49 (7): 616–627. doi:10.1310/hpj4907-616.
- Tokola, H., E. Niemi, and V. Väistö. 2016. “Lean Manufacturing Methods in Simulation Literature: Review and Association Analysis.” Winter simulation conference, WSC 2015, Huntington Beach, CA.
- Tranfield, D., D. Denyer, and P. Smart. 2003. “Towards a Methodology for Developing Evidence-Informed Management Knowledge by Means of Systematic Review.” British Journal of Management 14 (3): 207–222. doi:10.1111/1467-8551.00375.
- Van Eck, N. J., and L. Waltman. 2010. “Software Survey: Vosviewer, a Computer Program for Bibliometric Mapping.” Scientometrics 84 (2): 523–538. doi:10.1007/s11192-009-0146-3.
- Van Lent, W. A., P. Vanberkel, and W. H. Van Harten. 2012. “A Review on the Relation Between Simulation and Improvement in Hospitals.” BMC Medical Informatics and Decision Making 12 (1), doi:10.1186/1472-6947-12-18.
- Wagner, T., C. Herrmann, and S. Thiede. 2017. “Industry 4.0 Impacts on Lean Production Systems.” Procedia CIRP 63 (Supplement C): 125–131.
- Wang, L. F., and L. Y. Shi. 2013. “Simulation Optimization: A Review on Theory and Applications.” Zidonghua Xuebao/Acta Automatica Sinica 39 (11): 1957–1968. doi:10.3724/SP.J.1004.2013.01957.
- Womack, J. P., D. T. Jones, and D. Roos. 1990. The Machine That Changed the World: Based on the Massachusetts Institute of Technology 5-Million Dollar 5-Year Study on the Future of the Auto-Mobile. New York: Rawson Associates.
- Yadav, O. P., B. P. Nepal, M. M. Rahaman, and V. Lal. 2017. “Lean Implementation and Organizational Transformation: A Literature Review.” EMJ – Engineering Management Journal 29 (1): 2–16. doi:10.1080/10429247.2016.1263914.
- Yang, T., and J. C. Lu. 2011. “The Use of a Multiple Attribute Decision-Making Method and Value Stream Mapping in Solving the Pacemaker Location Problem.” International Journal of Production Research 49 (10): 2793–2817. doi:10.1080/00207541003801267.
- Yang, T., T. K. Wang, V. C. Li, and C. L. Su. 2015. “The Optimization of Total Laboratory Automation by Simulation of a Pull-Strategy.” Journal of Medical Systems 39 (1), doi:10.1007/s10916-014-0162-6.
- Young, T., J. Eatock, M. Jahangirian, A. Naseer, and R. Lilford. 2009. “Three Critical Challenges for Modeling and Simulation in Healthcare.” Winter simulation conference, WSC 2009, Austin, TX.