Abstract
Machine learning methods are widely used for rolling bearing fault diagnosis. Most of them are based on a basic assumption that training and testing data are adequate and follow the same distribution. However, for bearings working under multiple working conditions, dynamic changes are inevitable and labelled vibration data are usually insufficient. To deal with the issues, a new fault diagnosis method using deformable convolutional neural network (CNN), deep long short-term memory (DLSTM) and transfer learning strategies is designed. Specifically, a model is constructed by integrating deformable CNN, DLSTM and dense layers. Among them, deformable CNN enhances the ability of standard CNNs for local feature extraction using fixed geometric structures. DLSTM further encodes the sequential information contained in the output of deformable CNN. Dense layers are applied to capture high-level features then classify the data samples as each fault type. The model is firstly pre-trained using data samples under one working condition. Then, transfer learning strategies are implemented to fine-tune the pre-trained model utilising very few samples of another working condition, enabling it to identify fault types of bearing under new condition. Experiments are conducted and results show that the presented model yields higher than comparative performance compared with state-of-the-art methods.
Disclosure statement
No potential conflict of interest was reported by the author(s).
Additional information
Funding
Notes on contributors

Zheng Wang
Wang Zheng is a Ph.D. student at the School of Mechanical Engineering, Shanghai Jiao Tong University. His research focuses on multiple AI topics in industry, including intelligent monitoring, intelligent fault diagnosis and intelligent product design. He has been specifically interested in various deep learning models used for dealing with both classification and regression problems based on sensor-collected data in the life cycle of industrial products. Current research interests include using deep reinforcement learning and multi-objective optimization for product design improvement.
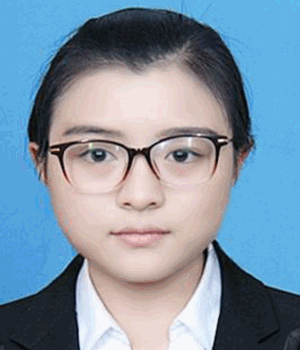
Qingxiu Liu
Liu Qingxiu is a master candidate in Department of Industrial Engineering and Management, Shanghai Jiao Tong University. Her research primarily focuses on the prognostics and health management of the wind turbine using deep learning methods, including anomaly detection, early fault isolation, performance assessment and health monitoring, etc. She has been specifically interested in analyzing the root cause of the wind turbine’s abnormal performance based on data-driven algorithms with evaluating the external environment.
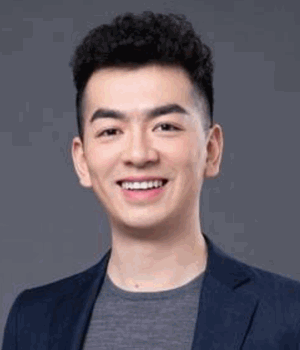
Hansi Chen
Chen Hans is a Ph.D. student at the School of Mechanical Engineering, Shanghai Jiao Tong University. His research spans a wide range, but currently focuses on the design improvement of large wind turbines based on condition monitoring data, i.e. SCADA data, looking at ways to find the root cause of product performance degradation from the data. Data mining and machine learning methods are used to extract useful information from the data to provide a basis for product iteration and design improvement.
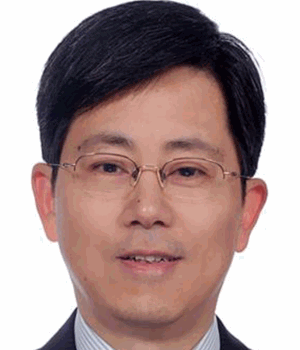
Xuening Chu
Chu Xuening is a Professor in Department of Industrial Engineering and Management, Shanghai Jiao Tong University. He is also a visiting professor at University of California, Berkeley, Aalborg University in Denmark and City University of Hong Kong. His research interests include intelligent design, intelligent diagnosis, product engineering and product service system. He has been granted more than 30 research projects by the National Hi-Tech R&D Program (863), the Natural Science Foundation of China, the Ministry of Education, the Science and Technology Commission of Shanghai Municipality, etc. He has published over 120 papers and supervised more than 80 graduate students. He won a number of scientific and technological achievement awards. He has been listed among Elsevier highly cited Chinese scholars since 2014.