Abstract
The rapid development of urbanisation and the ever-changing consumers’ demands are constantly changing the urban logistics industry, imposing challenges on logistics service providers to improve customer satisfaction which is one of the indicators for the sustainability of urban logistics. Existing customer satisfaction evaluations are based on a questionnaire survey, which is time-consuming and labour intensive. Moreover, the logistics data are confidential and can only be accessed by the stakeholders in existing logistics models, causing the problem of information non-transparency among logistics enterprises and the third authorities like banks and governments, which may hinder the sustainable development of urban logistics. In this paper, we propose a blockchain-based evaluation approach for customer satisfaction in the context of urban logistics. Four criteria affecting customer satisfaction in urban logistics are identified. A machine learning algorithm Long Short-Term Memory (LSTM) is adopted to predict customer satisfaction in the future period. The implementation is demonstrated to illustrate the proposed approach. A smart contract is designed for compensation and/or refund to customers when their satisfaction with the delivery services is at a low level.
Acknowledgement
This work was supported by National Natural Science Foundation of China under Grant [51405089]; Science and Technology Planning Project of Guangdong Province under [2015B010131008] and [2015B090921007]; and China Postdoctoral Science Foundation under [2018M630928]. It was also sponsored by the K. C. Wong Magna Fund in Ningbo University.
Disclosure statement
No potential conflict of interest was reported by the author(s).
Additional information
Funding
Notes on contributors
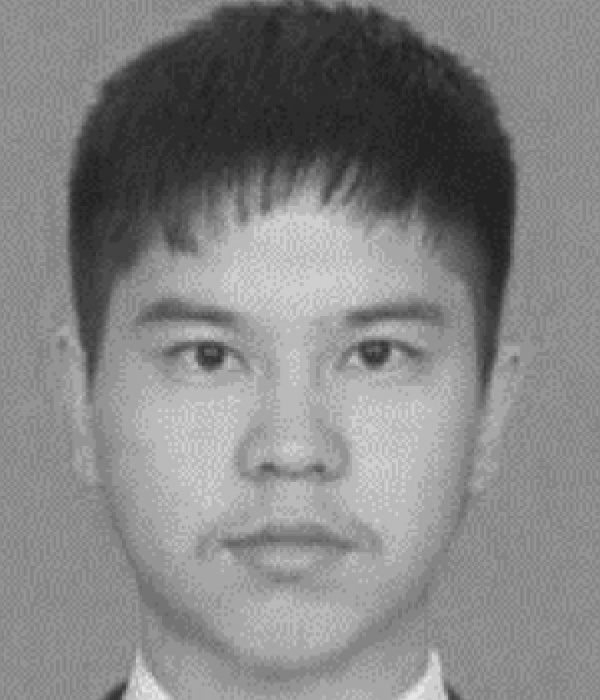
Zonggui Tian
Zonggui Tian received the B.Eng. degree in Mechanical Design, Manufacturing and Automation from Xiangtan University, Xiangtan, China, in 2015 and the M.Sc. degree in Mechanical Engineering at Guangdong University of Technology, Guangzhou, China in 2019, respectively. He is currently a research fellow at the College of Science and Technology, Ningbo University, China. His research interests include industrial blockchain and smart manufacturing.
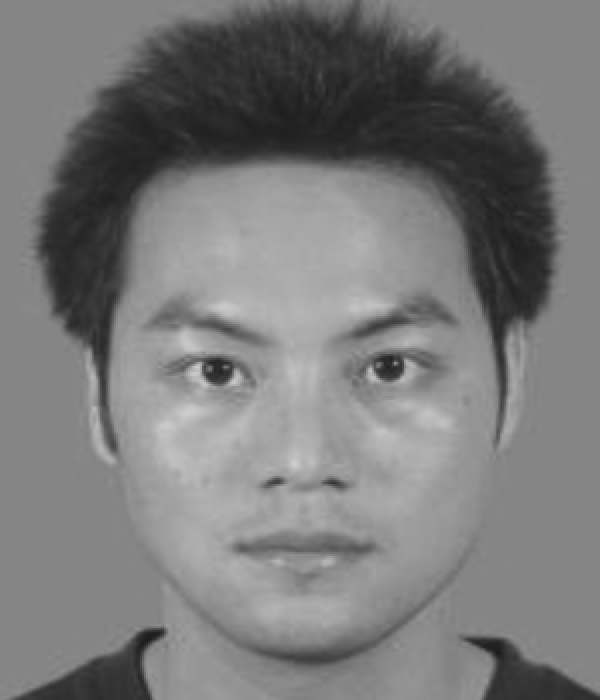
Ray Y. Zhong
Ray Y. Zhong received the Ph.D. degree in industrial and manufacturing systems engineering from the University of Hong Kong, China, in 2013, M.S. degree in signal and information processing from Guangdong University of Technology, China, in 2009 and B.S. degree in mathematics and computer science and technology from Gannan Normal University, China, in 2004. Dr. Zhong is current an Assistant Professor in Department of Industrial and Manufacturing Systems Engineering from the University of Hong Kong. He was a lecturer at Department of Mechanical Engineering, The University of Auckland from June 2016 to January 2019. From December 2015 to May 2016, he was a Research Assistant Professor with the Department of Industrial and Manufacturing Systems Engineering, The University of Hong Kong. His research interests include Big Data in manufacturing and RFID in Internet of Manufacturing Things. Dr. Zhong is member of IEEE, CIRP RA, IET, and ASME. He was a recipient of the Best Conference Paper Award in the 2018 and 2014 IEEE International Conference on Networking, Sensing and Control (ICNSC2018 and 2014), 2018 Best Paper of International Journal of Production Research and Certificate of Merit in the Hong Kong U-21 RFID Awards 2011, GS1 HK.
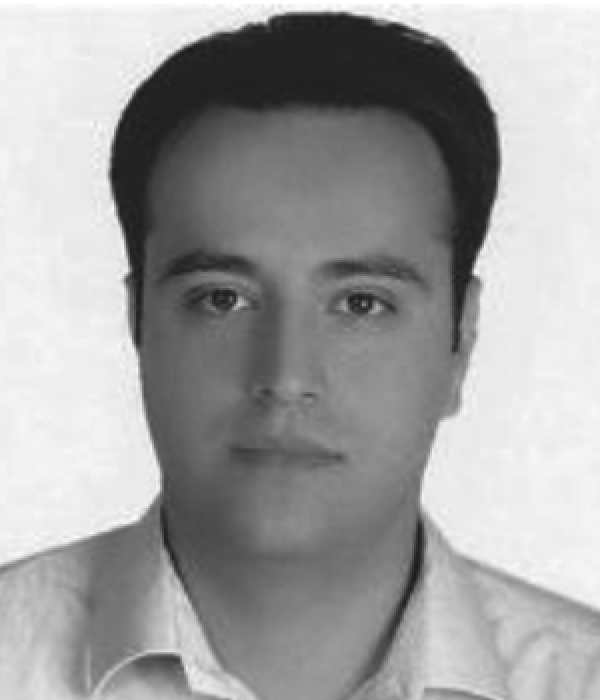
Ali Vatankhah Barenji
Ali Vatankhah Barenji received the M.Sc. and Ph.D. degrees in Mechanical Engineering from Eastern Mediterranean University, Cyprus, in 2013 and 2016, respectively. From 2014 to 2016, he was a Lecturer at the Mechanical Engineering Department of Eastern Mediterranean University. He was a Postdoctoral Fellow at the School of Electromechanical Engineering, Guangdong University of Technology, Guangzhou, China from November 2017 to October 2019. He is currently a Senior Research Scientist at H. Milton Stewart School of Industrial and Systems Engineering, Georgia Institute of Technology, Atlanta, USA. His research interests include multi-agent system, cloud manufacturing, smart manufacturing, blockchain and industrial internet of things.
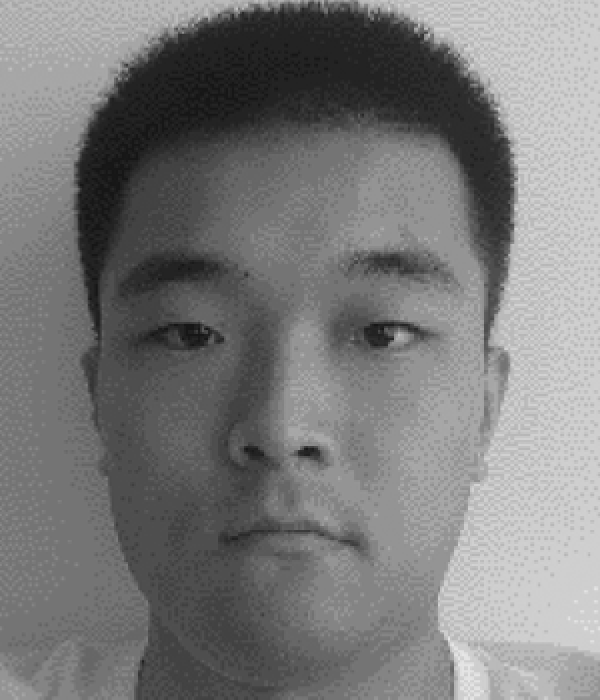
Y. T. Wang
Yitong Wang received the B.Eng. degree in Mechatronic Engineering from North University of China, Taiyuan, China, in 2018. He is currently pursuing the M.Sc. degree in Mechanical Engineering at Guangdong University of Technology, Guangzhou, China. His research interests include blockchain, supply chain management, and smart manufacturing.
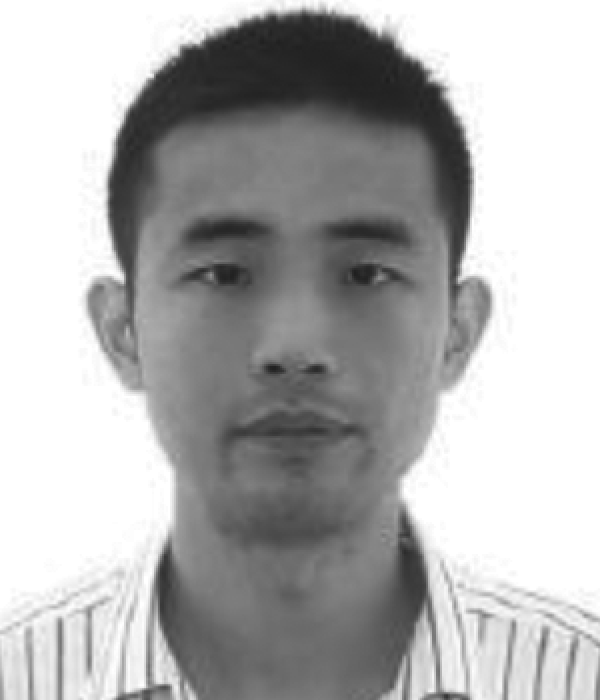
Zhi Li
Zhi Li received the B.Eng. degree in Mechanical Manufacturing and Automation from Huazhong University of Science and Technology, Wuhan, China, in 2005 and the Ph.D. degree in Industrial and Manufacturing Systems Engineering from the University of Hong Kong, Hong Kong, China, in 2013. He is currently a Distinguished Professor at the College of Science and Technology, Ningbo University, and an Associate Professor at the School of Electromechanical Engineering, Guangdong University of Technology. He has published more than 20 SCI journal papers. His research interests include industrial blockchain, artificial intelligence, product design and manufacturing internet of things. He is also the guest editor of two special issues in Robotics and Computer-Integrated Manufacturing and International Journal of Computer-Integrated Manufacturing.
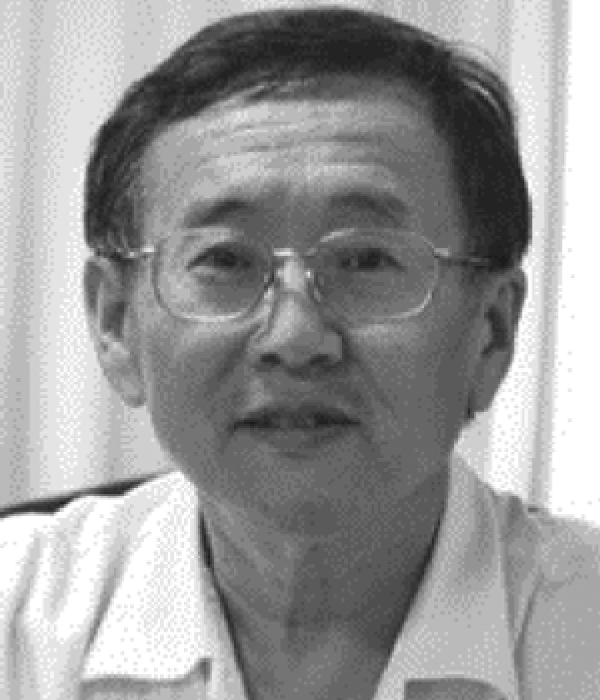
Yiming Rong
Yiming Rong received his Ph.D in Mechanical Engineering from University of Kentucky, Lexington, USA, in 1989. He is currently a professor of Mechanical and Energy Engineering in Southern University of Science and Technology, Shenzhen, China. Professor Rong is a tenured professor of Worcester Polytechnic Institute (WPI) in USA. He was named WPI Distinguished Professor for his outstanding scientific contributions. He is a fellow member of American Society of Mechanical Engineers, a senior member of Chinese Society of Mechanical Engineering, a member of Society of Manufacturing Engineers, a member of American Society of Metals and Heat Treatment Society, a member of American Society of Engineering Education. He was worked as a Professor in Tsinghua University from 2010 to 2015. Professor Rong's research interests include precision machining technology; Modelling, simulation and optimisation of metal material processing, and manufacturing system production planning and tooling technology. He has been responsible for more than 50 scientific research projects, and has been supported by American Science Foundation, Air Force Basic Research, Department of Energy and other federal agencies and major manufacturing companies (GM, Ford, Caterpillar, P&W, GE, Ingersoll and so on). After returning to China, he has presided over and participated in more than 10 projects supported by Natural Science Foundation, 973, 863, national major projects and industrial cooperation projects. He has published two academic monographs, over 300 research papers and applied patents.