Abstract
This paper studies a two-agent scheduling problem on mixed batch machines in parallel. A mixed batch machine can process several jobs simultaneously as a batch, as long as the number of jobs in the batch does not exceed the machine capacity. The processing time of a mixed batch is the weighted sum of the maximum processing time and the total processing time of jobs in the batch. The objective is to minimise the weighted sum of two agents' makespans. We present four approximation algorithms based on two strategies: the machine-centric strategy and the agent-centric strategy. For each strategy, a full batch longest processing time (FBLPT) rule and a longest processing time greedy (LPTG) rule are used. We conduct theoretical analyses based on the worst-case performance ratio to provide the provable guarantees on the performances of the algorithms, and simulation analyses based on randomly generated instances to evaluate the average performances of the algorithms. Furthermore, we verify the consistency between the theoretical and simulation results. The algorithms using agent-centric strategy perform better than ones using machine-centric strategy. Finally, we provide managerial insights for the problem by analysing the technological parameters of batches, importance of agents, and demand seasonality.
Acknowledgments
The authors are grateful to the editors and anonymous reviewers for their valuable comments, which have significantly improved the quality of this work. The work of the first two authors was partly supported by the National Key R&D Program of China (Grant No. 2019YFB1703800), the National Natural Science Foundation of China (Grant Nos. 51675442 and 71931007). The work of the third author was partly supported by the University of Michigan MCubed Project Grant and the University of Michigan-Dearborn Scholars Grant.
Disclosure statement
No potential conflict of interest was reported by the author(s).
Additional information
Funding
Notes on contributors
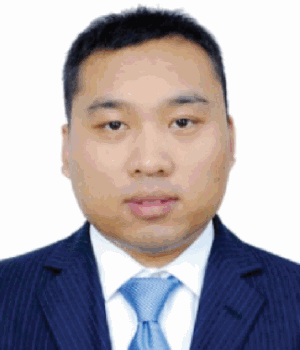
Guo-Qiang Fan
Guo-Qiang Fan is a Ph.D. candidate with the Department of Industrial Engineering, School of Mechanical Engineering, Northwestern Polytechnical University (NPU), Xi'an, China. His research focuses on algorithm design and analysis of scheduling.
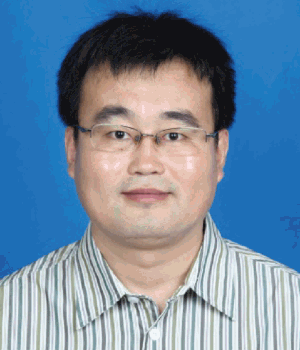
Jun-Qiang Wang
Jun-Qiang Wang is a Professor with the Department of Industrial Engineering, School of Mechanical Engineering, Northwestern Polytechnical University (NPU). He is also the Director of Performance Analysis Center of Production and Operations Systems (PacPos). He received a Ph.D. degree from the School of Mechanical Engineering, NPU, Xi'an, China, in 2006. His research interests include modelling, scheduling, analysis, and control of production and operations systems.
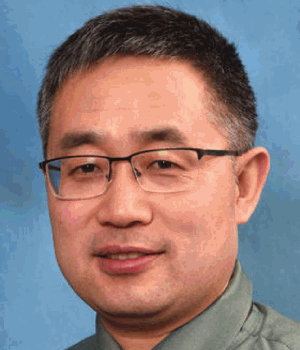
Zhixin Liu
Zhixin Liu is an Associate Professor of Decision Science at the University of Michigan-Dearborn College of Business. He received a B.S. degree in Computational Mathematics from Nankai University, a M.S. degree in Operations Research from Tsinghua University, and a Ph.D. degree in Operations Management from Ohio State University. His research interests include scheduling, capacity allocation, and pricing, specifically those with game issues. He is on the Editorial Review Board for Production and Operations Management.