Abstract
Production research literature and industry practice have started to pay increasing attention to the Industry 4.0 (I4.0) phenomenon. Scholars and practitioners identified a strong link between this paradigm and the well-known Lean Production (LP) paradigm. Most studies consider LP as a prerequisite of I4.0 and I4.0 as a tool to overcome LP limits and boost its practices. However, so far, these effects have been studied only at a high level, without an in-depth and comprehensive pairwise analysis at a practice-technology level. Moreover, few empirical studies have been carried out on this topic. Our paper attempts to fill these gaps by conducting a multiple case studies research to explain the one-to-one relationships between LP techniques and I4.0 technologies, and vice versa. More specifically, the one-to-one analysis examines the enabling effect of LP on I4.0 and the empowering effect of I4.0 on LP. Based on the empirical analyses, we propose a framework on the relationships between the two paradigms structured into six areas drawn from previous research (i.e. manufacturing equipment and processes, shop-floor management, workforce management, new product development, supplier relationships, customer relationships). Such representation clarifies the interdependence of the two paradigms in the whole supply chain.
1. Introduction
The last four decades of operations management and production research literature have been characterised by an increasing interest in the lean production (LP) phenomenon (Ciano et al. Citation2019). On one hand, LP has been proved to eliminate wastes, reduce set-up and lead times, reduce inventories, and improve performance (Pozzi et al. Citation2018; Kovács Citation2020; Tortorella, Fettermann, et al. Citation2020; Sancha et al. Citation2020). On the other hand, it can improve the employees’ morale and satisfaction, communication, and decision-making attitude (Jasti and Kodali Citation2015; Hopp Citation2018; Ciano et al. Citation2019).
All these LP benefits lead to costs and efforts reduction and contribute to reaching customer satisfaction (Jasti and Kodali Citation2015; Kovács Citation2020). However, nowadays, the market is increasingly competitive and requires companies to improve rapidly; moreover, it is challenging the industry with the rapid increase of highly customised products’ demand (Kolberg, Knobloch, and Zühlke Citation2017; Buer, Strandhagen, and Chan Citation2018). LP is facing several challenges from an integration perspective; indeed, the acquisition of exact customer needs is getting more and more complex, pull production must face rapid changes in scheduling, and often the set-up time reduction is based exclusively on human experience (Sanders, Elangeswaran, and Wulfsberg Citation2016). The current context implies complex systems and a consequent high-volume data that call for the adoption of new solutions able to exploit the potential of information and communication technologies (Kolberg, Knobloch, and Zühlke Citation2017; Buer, Strandhagen, and Chan Citation2018; Yin, Stecke, and Li Citation2018).
The literature considers the potentialities of Industry 4.0 (I4.0) able to overcome the LP's limits in facing the aforementioned issues (Kolberg and Zühlke Citation2015; Kolberg, Knobloch, and Zühlke Citation2017). Indeed, I4.0 is seen as the ‘usage of intelligent products and processes, which enables autonomous data collection and analysis as well as the interaction between products, processes, suppliers, and customers through the internet’ (Buer, Strandhagen, and Chan Citation2018). Several studies demonstrate that I4.0 technologies significantly improve industrial performance, most of all, flexibility, productivity, delivery time, cost, and quality (Moeuf et al. Citation2018, Citation2020). Furthermore, I4.0 is attracting many companies since these benefits can be translated into the promise of an individual and customised production at the same cost of mass production (Wang et al. Citation2016). Indeed, the integration of smart machines and components into a digital network, at the basis of I4.0, creates a so-called cyber-physical system that enables a modular and changeable production (Kolberg, Knobloch, and Zühlke Citation2017), as the prerequisite of an individual single-item production (Matt and Rauch Citation2013; Kolberg and Zühlke Citation2015).
However, I4.0 does not mean the sunset of lean. On the contrary, literature recognises a strong interdependency between the two paradigms (e.g. Sanders, Elangeswaran, and Wulfsberg Citation2016; Buer, Strandhagen, and Chan Citation2018; Tortorella and Fettermann Citation2018; Kamble, Gunasekaran, and Dhone Citation2020; Rossini et al. Citation2019; Rosin et al. Citation2020). Indeed, on the one hand, the LP's high streamlined process orientation with defined tasks and times, its standardisation of work and places, and its emphasis on visual control and transparency facilitate the implementation of the I4.0 information sharing and automation (Kolberg and Zühlke Citation2015; Dombrowski, Richter, and Krenkel Citation2017). On the other hand, several studies pointed out that I4.0 technologies can boost LP practices (Buer, Strandhagen, and Chan Citation2018). For instance, factory integration, sensors, and IoT technologies can improve Kanban, shorten cycle time, and make milk-runs more efficient (Hofmann and Rüsch Citation2017). Moreover, these technologies allow the real-time collection of data that can empower the value-stream-mapping and fasten the wastes’ detection (see among others: Meudt, Metternich, and Abele Citation2017; Mrugalska and Wyrwicka Citation2017).
The investigation of the mutual support between the two paradigms is however still characterised by some gaps. Even though scholars agree on the enabling effect of implementing LP before I4.0, so far this effect has been studied just at a high level, without investigating the specific LP practices that cause it (Buer, Strandhagen, and Chan Citation2018). Moreover, there is still a lack of an in-depth and comprehensive pairwise analysis at a practice-technology level, able to clarify the roles of both paradigms in a LP-I4.0 transformation (Rossini et al. Citation2019). These gaps are also related to the general scarcity of empirical studies about the topic (Buer, Strandhagen, and Chan Citation2018; Pagliosa, Tortorella, and Ferreira Citation2019). Indeed, according to a recent review, only 14 percent of the studies in the field are empirical and actually verified how the relationships occur (Pagliosa, Tortorella, and Ferreira Citation2019).
All these aspects led to the lack of a holistic integration framework (Buer, Strandhagen, and Chan Citation2018), especially of one considering the link between I4.0 technologies and LP practices according to the entire value chain (Pagliosa, Tortorella, and Ferreira Citation2019).
This paper aims to fill these gaps by distinguishing and characterising the possible effects of the two paradigms on each other in enabling, i.e. the creation of opportunities for something to become able or competent, and/or empowering, i.e. the achievement of a sense of self-efficacy and of best version (Dunst, Trivette, and Deal Citation1988). Given their definitions, and the fact that an empowering effect implies that its objectives are already present or possible (Dunst, Trivette, and Deal Citation1988), we consider the effect of LP on I4.0 enabling, and the effect of I4.0 on LP empowering. The analysis of these effects is conducted in a one-to-one way, answering the following research questions:
RQ1: How do LP techniques and practices enable I4.0 technologies and characteristics?
RQ2: How do I4.0 technologies and characteristics empower LP techniques and practices?
The results are obtained through the analysis of eight case studies conducted in manufacturing companies that apply both paradigms.
Our paper significantly contributes to both production research theory and practice. Indeed, it fills the content gaps depicting a detailed picture of the relationship between LP and I4.0 in both directions. Moreover, the results lead to the formalisation of an integration framework that encompasses all the areas of the value chain. The disclosure of the practice-technology relationship and the insights given by the companies can help practitioners in understanding the requirements and in anticipating the effects of the specific directions of the LP-I4.0 transformation.
The article is organised as follows: Section 2 defines the domain of LP and I4.0 and outlines their relationship through a literature review. Section 3 describes the research method and the analysed sample. The results from the case studies are presented in section 4. Section 5 discusses the main findings in light of extant literature, while section 6 summarises and concludes the article.
2. Background
This section aims first to explain the main concepts of both LP and I4.0 paradigms and to collect the key techniques and technologies to be investigated. Then, it aims to depict the state of the art of the scientific production that focuses on the effect of LP on I4.0 and the effect of I4.0 on LP.
2.1. Main concepts
2.1.1. Lean production practices and techniques
In over forty years of study, the body of knowledge on LP has expanded considerably to include dedicated journals, various special issues and many literature reviews on it. According to the high recognised reviews by Bhamu and Sangwan (Citation2014) and by Jasti and Kodali (Citation2015), in literature LP is generally addressed from two points of view, namely from a theoretical perspective related to guiding principles and general goals (Womack and Jones Citation1996; Spear and Bowen Citation1999), or from the practical perspective of a set of practices and techniques that can be observed directly (Shah and Ward Citation2003). The scope of this study leads to deepening the understanding of LP practices and techniques, the tools through which LP helps companies to achieve all the above-mentioned benefits, and to strive towards operational excellence (Möldner, Garza-Reyes, and Kumar Citation2020).
LP practices have been extensively adapted and implemented in several sectors with the general expectation that by adopting the same set of practices, the companies can obtain similar benefits that will distinguish them in their businesses (Tortorella, Fettermann, et al. Citation2020).
In literature, the LP practices were often categorised into bundles (Sancha et al. Citation2020; Bevilacqua, Ciarapica, and De Sanctis Citation2017). Flynn, Sakakibara, and Schroeder (Citation1995) focused on the practices related to Just-in-Time (JIT) and Total Quality Management (TQM). Sakakibara et al. (Citation1997) divided the practices into infrastructure practices (quality management, workforce management, manufacturing strategy, organisational characteristics, product design) and JIT practices (set-up time reduction, schedule flexibility, maintenance, equipment layout, kanban and JIT supplier relationships). For McLachlin (Citation1997), LP practices can be associated to the JIT flows, Human Resource Management (HRM) and empowerment, Total Productive Maintenance (TPM) and equipment management, and TQM. According to Van Assen and de Mast (Citation2019), these four bundles of practices are still the most addressed by literature.
Looking at the tradition of LP literature, Jasti and Kodali (Citation2015) affirmed that it can be classified into four research streams, i.e. lean manufacturing, lean product development, lean supply chain management and lean enterprise. The first three streams concern the application of LP in the related fields, the last one emphasises the application of LP across the entire value chain of a company (Womack and Jones Citation1994). Indeed, a lean enterprise is a company that selects the best practices in all functional areas and the management of external relationships (Karlsson and Åhlström Citation1996).
Based on this last concept, the seminal study by Panizzolo (Citation1998) suggested a scheme for classifying the LM practices composed of six areas of intervention of LP that encompass all the internal and the external pivotal aspects: the manufacturing area, other internal areas such as product design and development and human resources, and relationships with external actors belonging to the supply chain, namely suppliers and customers. Later, this scheme was recalled by Doolen and Hacker (Citation2005) and by Bai, Satir, and Sarkis (Citation2019); both studies updated the techniques and practices corresponding to the six areas identified by Panizzolo (Citation1998). In particular, the recent study of Bai, Satir, and Sarkis (Citation2019) included the findings of many works attempting to classify LM practices, namely Bortolotti, Boscari, and Danese (Citation2015); Sezen, Karakadilar, and Buyukozkan (Citation2012); Saurin, Marodin, and Ribeiro (Citation2011); Pettersen (Citation2009); Shah and Ward (Citation2007); Shah and Ward (Citation2003); Cua, McKone, and Schroeder (Citation2001). The general classification brought by Panizzolo (Citation1998) and the following studies adopting his view assign to the area manufacturing equipment and process, all the techniques related to the set-up and cycle time reduction, to the work standardisation and the pursuit of quality. Another area, devoted to the shop floor management, includes the techniques regarding the pull flow, the lot size reduction, and the production scheduling. The external actors belonging to the supply chain, i.e. suppliers and customers, have two distinct areas, i.e. supplier relationships and customer relationships. The internal relationships with the workforce were characterised by a set of LP practices referring to their commitment and their organisation, like multifunctional teamwork. Finally, the area about new product development included the techniques concerning the standardisation of the components and the design for manufacturing.
Nowadays, in addition to internal LP practices within individual manufacturing organisations, existing studies and industry pay attention to LP practices in the context of manufacturing supply chains (Meng Citation2019). Therefore, to adopt a comprehensive value chain perspective, considering both internal and external areas, this study refers to a list of LP techniques and practices classified into the related impact areas, drawn from Panizzolo (Citation1998), Doolen and Hacker (Citation2005), Buer, Strandhagen, and Chan (Citation2018), Bai, Satir, and Sarkis (Citation2019), and their main references, as reported in Table .
Table 1. LP practices and techniques classified into six impact areas.
2.1.2. Industry 4.0 technologies and characteristics
I4.0 is a label referred to the cyber-physical production system based on the integration, the transparency and the availability of heterogeneous knowledge, for meeting the market's requirements (Lu Citation2017). I4.0 is not limited to direct manufacturing in the company, but it concerns the whole value chain (Lu Citation2017; Rojko Citation2017; Tortorella and Fettermann Citation2018; Frank, Dalenogare, and Ayala Citation2019). Moreover, it can track and support the entire lifecycle of systems and products introducing new business and services (Rojko Citation2017; Dalenogare et al. Citation2018). Furthermore, I4.0 significantly affects production dynamics; its technologies can indeed substitute the conventional forecast based production planning with real-time planning together with dynamic self-optimisation (Moeuf et al. Citation2020; Sanders, Elangeswaran, and Wulfsberg Citation2016).
The concept of I4.0 is very complex and comprehensive, and literature does not provide a univocal definition (Buer, Strandhagen, and Chan Citation2018; Piccarozzi, Aquilani, and Gatti Citation2018; Culot et al. Citation2020). Among the many, Pan et al. (Citation2015) focused on the fact that I4.0 represents the ability of industrial components and actors to communicate. This focus on the link between elements was highlighted by Kovács and Kot (Citation2016) as well; they argued that the core point of I4.0 is the introduction of network-linked intelligent systems able to realise a self-regulating production. Burritt and Christ (Citation2016), Lu (Citation2017), Frank, Dalenogare, and Ayala (Citation2019), Bai, Satir, and Sarkis (Citation2019) and many other authors proposed a definition of I4.0 based on its combining nature, considering I4.0 as an umbrella term to describe a group of connected technological advances for increasing the digitisation of the business.
This last aspect was underlined by the key work in the I4.0 formalisation developed by Rüßmann et al. (Citation2015), who identified nine technological ‘pillars’ of I4.0: (i) Autonomous Robots, (ii) Simulation, (iii) Horizontal and Vertical Integration, (iv) Industrial Internet of Things (IIoT), (v) Cybersecurity, (vi) Cloud, (vii) Additive Manufacturing, (viii) Augmented Reality, and (ix) Big Data and Analytics. The pillars are also confirmed by the review of I4.0 definitions performed by Culot et al. (Citation2020), as well as by other reviews on Industry 4.0 (i.e. Liao et al. Citation2017; Machado, Winroth, and Ribeiro da Silva Citation2020; Parente et al. Citation2020).
Autonomous Robots include: Collaborative Robots, that perform repetitive and non-ergonomic tasks in direct collaboration with the operator (Romero et al. Citation2016); Automated Guided Vehicles (AGV), that realise decentralised coordination of supplies (Stock and Seliger Citation2016); and Smart Machines that communicate with robots and products and make consequent decisions (Davenport and Kirby Citation2016).
Simulation can leverage real-time data to mirror the physical world in a virtual model (Rüßmann et al.Citation2015), a concept that also leads to the Digital Twin (DT) (Uhlemann, Lehmann, and Steinhilper Citation2017). DT represents an achievement even for maintenance; indeed, training or scenarios simulations can be performed in a safe context replicating the ‘real’ environment (Tuegel et al. Citation2011; Negri, Fumagalli, and Macchi Citation2017; Liu, Meyendorf, and Mrad Citation2018).
Horizontal and Vertical System Integration guarantees the truly automated value chain (Rüßmann et al. Citation2015). Horizontal Integration, across the whole value chain, and Vertical Integration, within the company, are possible due to MES/ERP software, sensors and IIoT for data sharing (Lasi et al. Citation2014; Ivanov, Dolgui, and Sokolov Citation2019). This might also allow to enhancing the ripple effect control along supply chains (Ivanov, Dolgui, and Sokolov Citation2019). IIoT allows the interconnection at the basis of the integration enriching some devices with embedded computing. Some examples of IIoT are RFID, sensors, tags, global positioning systems (Shrouf, Ordieres, and Miragliotta Citation2014), real-time scanning through smartphones (Xu, Xu, and Li Citation2018), and smart bins (Thürer et al. Citation2016).
With interconnection, companies need protection from cyber-attacks. Some Cybersecurity systems listed in literature are encryption, cryptography, virus scanners, signature scanner, ICT Anomaly Detection/IDS (Flatt et al. Citation2016).
Data sharing and data analytics can be facilitated by the adoption of Cloud-based software. Indeed, cloud computing enables ubiquitous and on-demand network access to a shared collection of resources (Mell and Grance Citation2011).
Additive Manufacturing, a layer-by-layer build-up of parts (Herzog et al. Citation2016), such as 3D Printings, allows a quickly customised single-item or small batches production. In this regard, smart material with shape memory can be used to achieve the desired characteristics (Dilberoglu et al. Citation2017).
Augmented Reality (AR), as an overlap of computer-generated sensory input in a physical real-world, and Virtual Reality (VR), as a completely artificial environment created by software (Chavan Citation2014), might be applied for different purposes, like warehouse management, layout testing, operators’ training, and remote maintenance (Rüßmann et al. Citation2015; Büttner et al. Citation2017).
Big Data collected in the smart factory allows process optimisation. Data Analytics divides into Descriptive, Predictive and Prescriptive. Descriptive Analytics describes the current situation and helps in the diagnosis of the problems; Predictive Analytics predict future events; while Prescriptive Analytics concerns decision-making mechanisms (Nguyen et al. Citation2018). Artificial Intelligence can exploit the collected data to make the system self-aware, self-adaptive, proactive and prescriptive (Kibria et al. Citation2018).
Various studies argue that Industry 4.0 offers unique opportunities to redesign production processes as well as business models, this way significantly enhance firm performance (Moeuf et al. Citation2020; Hahn Citation2020; Culot et al. Citation2020).
Table provides an overview of the main I4.0 technologies and characteristics considered by this study, classified into the nine pillars presented above.
Table 2. I4.0 technologies or characteristics.
2.2. The effect of lean production on Industry 4.0
The history of LP is characterised by a tendency towards autonomation since its early stages; indeed, its founder, Taiichi Ohno, stated that repetitive value-adding processes should be automated to improve the information flow and meet the market demands (Kolberg, Knobloch, and Zühlke Citation2017). In this regard, Ohno adopted the term ‘autonomation’ (Ohno Citation1988). Since autonomy is a crucial theme in I4.0, LP can be considered as its precursor.
According to Wang et al. (Citation2016), a production process already based on LP can be an easier environment for the introduction of I4.0. Other papers stated or demonstrated the same concept (Parente et al. Citation2020). Kolberg and Zühlke (Citation2015) and Dombrowski, Richter, and Krenkel (Citation2017) highlighted the need for the LP process orientation and defined tasks and times to implement the I4.0 autonomation and information exchange and to lower the risks of integration. Indeed, only standardised processes allow the identification of errors and the consequent troubleshooting, essential for a valuable synchronisation of the various processes (Butollo, Jürgens, and Krzywdzinski Citation2018). Evidence by Tortorella and Fettermann (Citation2018) proved that the success of I4.0 is significantly associated with the level of LP adoption. Pessl, Sorko, and Mayer (Citation2017) confirmed the importance of LP suggesting its adoption as a fundamental strategy in a roadmap for I4.0 implementation. Erol et al. (Citation2016) pointed out that LP as a basic competence should be transferred as well to the technical level. Mayr et al. (Citation2018) added that LP enables I4.0 even because of the competencies that a LP decision-maker has in considering customer value and in avoiding wastes. They also highlighted the potential of LP reduction of product and process complexity to enable the efficient and economic use of I4.0 technologies. Even the more recent paper by Rossini et al. (Citation2019) emphasises the need for a previous LP adoption to successfully introduce I4.0. Pagliosa, Tortorella, and Ferreira (Citation2019) highlighted that VSM can be potentially beneficial for the adoption of I4.0 since it provides a structured approach to identify improvement opportunities.
Therefore, literature seems to consider LP as a prerequisite to the I4.0 implementation (Ciano et al. Citation2019). Nevertheless, Buer, Strandhagen, and Chan (Citation2018) identified a lack of investigation about the facilitating effect of LP on I4.0. Indeed, even if scholars agree on the general enabling effect of I4.0 on LP, the knowledge of how it happens is still immature (Wagner, Herrmann, and Thiede Citation2017; Buer, Strandhagen, and Chan Citation2018; Rossini et al. Citation2019).
2.3. The effect of Industry 4.0 on lean production
Literature focusing on the effect of I4.0 on LP is richer than the one focused on the opposite direction. However, few articles considered a comprehensive set of technologies or practices (see Mayr et al. Citation2018; Rosin et al. Citation2020; Pagliosa, Tortorella, and Ferreira Citation2019). Indeed, most studies considered limited and specific aspects. For instance, Kietzmann, Pitt, and Berthon (Citation2015) highlighted that 3D Printers can be considered a new form of Kanban, as they can intervene in the inventory management replicating JIT items on-demand. Lu, Li, and Tian (Citation2015) stated that the adoption of 3D Printing helps in equipment maintenance, re-manufacturing and on-site maintenance. Regarding SMED, Feldmann and Gorj (Citation2017) argued that, since Additive Manufacturing is not product-specific, the set-up time for selection, search and adjustment of pieces and tools are avoided.
According to Negahban and Smith (Citation2014), Simulation empowers JIT, because it allows to test and optimise the AGV's routes. Rosin et al. (Citation2020) found that the ability of Simulation to detect bottlenecks ensures JIT continuous flow. Simulation can be also used for the identification of ideal Kanban parameters like stock, delivery frequency and lot size (Goienetxea Uriarte, Ng, and Urenda Moris Citation2020). DT, instead, can reproduce realistic production plants helpful in maintenance activities (Mayr et al. Citation2018).
JIT, and especially One-Piece-Flow, can be promoted by Collaborative Robots included in lean assembly lines allowing the increase of production capacity and hence productivity (Gil-Vilda et al. Citation2017).
According to Rosin et al. (Citation2020), the majority of the studies about the support of I4.0 on LP focus on IIoT. Indeed, they are essential to retrieve information, identification and assignment of spaces, materials, pieces and tools (Mayr et al. Citation2018). Literature particularly focuses on the benefits that IIoT and Cyber-Physical Systems can bring to VSM (Buer, Strandhagen, and Chan Citation2018; Pagliosa, Tortorella, and Ferreira Citation2019; Kamble, Gunasekaran, and Dhone Citation2020), Continuous Flow, Pull/Kanban strategy, and VM (Rosin et al. Citation2020). Real-time data collected by IIoT can bring to the so-called VSM 4.0 (Meudt, Metternich, and Abele Citation2017), a dynamic picture of the shop floor (Buer, Strandhagen, and Chan Citation2018; Tortorella, Pradhan, et al. Citation2020). The JIT continuous flow and the Pull/Kanban strategy are ensured by the IIoT ability to track products in real-time and to send instant progress updates (Rosin et al. Citation2020). Smart Bins recognise in real-time their filling level and the system sends a virtual Kanban to trigger replenishments (Kolberg and Zühlke Citation2015). IIoT can empower VM thanks to the visual monitoring information available to employees (Rosin et al. Citation2020). The studies by Kolberg and Zühlke (Citation2015), Ma, Wang, and Zhao (Citation2017), and Kolberg, Knobloch, and Zühlke (Citation2017) focused on the role of Integration and IIoT for the empowerment of Jidoka, especially Andon. The latter study underlined the positive effect of IIoT and Integration on JIT, Heijunka and Continuous Improvement.
Other papers focus on the importance of IIoT devices to collect data for the prediction and the prescription of maintenance programmes (e.g. Lee et al. Citation2015; Khoshafian and Rostetter Citation2015; Sanders et al. Citation2017; Kipper et al. Citation2020).
Mayr et al. (Citation2018) highlighted the importance of Big Data and Analytics in JIT, indeed the analysis of real-time process information provides insights into parameters, trends, and rules for the production system. In this direction, Huo, Zhang, and Chan (Citation2020) propose a fuzzy control system that uses real-time data made available by Industry 4.0 technology to determine when to rebalance an assembly line and how to adjust production rates to achieve higher utilisation of machines and lower buffer levels.
Mayr et al. (Citation2018) stated that AR and VR are important for JIT, TPM, SMED, VSM and Poka-Yoke. They mainly focused on the possibility of AR to replace physical boards to guide operators and on the use of VR to train operators for maintenance objectives. Similarly, Mourtzis et al. (Citation2019) report on an AR-based maintenance system that provided an increased capacity to a robotics company.
Considering the supply chain level, Núñez-Merino et al. (Citation2020) investigated by using a Systematic Literature Review (SLR) the relationships between Information and Digital Technologies (IDT) of Industry 4.0 and Lean Supply Chain Management. According to the authors, when extended to the supply chain level, a large number of IDT of Industry 4.0 and several Lean practices are used at the same time. As an example, IoT use in Lean Supply Chain Management usually occurs together with RFID, Cyber-Physical System (CPS), Cloud Computing and Big-Data affecting positively different LP practices. These benefits cover agility, flexibility, information sharing, collaboration, coordination, reduced costs, faster deliveries, reduced inventory levels as well as errors, enabling traceability and inventory control, reduced risk, improved service quality as well as customer satisfaction (Núñez-Merino et al. Citation2020).
Among the others, Buer, Strandhagen, and Chan Citation2018 pointed out that one underexplored aspect in literature is the effect of I4.0 on the so-called ‘soft’ LP techniques, namely the ones regarding Workplace and VM and Human Resources.
In conclusion, it can be argued that the relationship between the two paradigms has been studied mostly at a high level, with a poor investigation at a practice-technology level (Rossini et al. Citation2019). As can be seen, some studies deepened the relationship but referring to specific and limited aspects. The studies by Mayr et al. (Citation2018), Rosin et al. (Citation2020) and Pagliosa, Tortorella, and Ferreira (Citation2019) attempted to conduct a comprehensive pairwise investigation. However, the first two studies focused just on the effect of I4.0 on LP, a research area that has more contribution than the one referring to the opposite direction (Buer, Strandhagen, and Chan Citation2018). Pagliosa, Tortorella, and Ferreira (Citation2019) proposed synergy levels in both directions, but referring to LP they explained only the ones of VSM and Takt-Time on the general paradigm of I4.0.
3. Research method
3.1. Case study approach and sampling
Driven by the goal of our paper (theory building) and the nature of the RQs (i.e. ‘how’ questions, see the Introduction section), we adopted a multiple case study methodology. This research methodology, ‘oriented towards exploration, discovery, and inductive logic’ (Patton Citation2002), is indeed suited to the development of data grounded testable theories (Eisenhardt Citation1989; Voss, Tsikriktsis, and Frohlich Citation2002).
Our unit of analysis is the company within a manufacturing value chain. Considering the focus of our study (i.e. the relationship between I4.0 and LP) the population of interest consists of companies that have adopted both these paradigms. We adopted a theoretical sampling method (Eisenhardt Citation1989; Patton Citation2002) and selected heterogeneous cases in terms of firm size (both SMEs and large companiesFootnote1), manufacturing industries (e.g. manufacture of fabricated metal products, electromechanical and electronic components, pumps, cableways, and sealing solutions), and level of adoption of I4.0 (both ‘normal’ adopters and lighthouse plants) and homogeneous cases in terms of country (Italy). The sampled companies consist of different players of the manufacturing value chain (e.g. case D is a supplier of case H, and case C is a provider of MES and other I4.0 technologies). Despite the focus on the manufacturing value chain and a single country might reduce the possibility to generalise findings, it ensures that variation is not caused by extraneous or confounding variables (e.g. Saunders, Lewis, and Thornhill Citation2003).
Table provides data about the sampled companies, using code names to protect identity. Appendix A shows in detail the specific LP principles and techniques as well as I4.0 technologies adopted by each company.
Table 3. Sampled companies.
3.2. Data collection
Data were mainly collected through face-to-face semi-structured interviews. Archival data (e.g. annual reports, websites, and internal documents on LP or I4.0 implementation projects) were also used to triangulate the empirical evidence.
Based on the review of the relevant literature (see section 2), we defined an interview protocol organised in four main sections: (1) general information about the company and the interviewee; (2) adoption of LP principles and techniques; (3) adoption of I4.0 technologies; (4) relationships between LP and I4.0. In section 2, we provided to the interviewees a detailed list of LP principles and techniques classified into six impact areas (see Table ) and asked them whether, when, and in which department each technique was adopted. In section 3, we provided instead to the interviewees a detailed list of I4.0 technologies or characteristics (see Table ) and asked them whether, when, and in which department/activity each technology was adopted. Finally, in section 4, we asked to the interviewees to identify and explain all possible one-to-one links between LP practices and I4.0 technologies (in both directions), i.e. whether and how each LP technique/practice has enabled the adoption of each I4.0 technology/characteristics and whether and how each I4.0 technology/characteristic has empowered each LP technique and practice. The interview protocol is reported in Appendix B.
In each company, we interviewed at least two managers (including the production and operations manager) to enhance validity (Yin Citation2003) and reliability of the collected data (Voss, Tsikriktsis, and Frohlich Citation2002). Each interview lasted 90–120 min. All interviews were recorded and transcribed verbatim.
3.3. Data analysis
We created a database for each case consisting of the interview transcripts, field notes, and archival data. As suggested by Eisenhardt (Citation1989) and Voss, Tsikriktsis, and Frohlich (Citation2002), we then analysed the data following a two-step procedure (i.e. within-case analysis and cross-case analysis). Due to length limitation, we however reported in the paper only the results of the cross-case analysis.
In the within-case analysis, we developed for each case two tables, following the suggestions of Miles and Huberman (Citation1994) for qualitative data analysis. In the first one, we put in the lines the LP practices/techniques mentioned by the interviewees, in the columns the I4.0 technologies mentioned, and in the intersections the explanation of the enabling effects of LP practices on I4.0 technologies provided by the interviewees (if any) (an excerpt of this table can be found in Appendix C – Table C.1). In the second table, we put instead in the lines the I4.0 technologies mentioned by the interviewees, in the columns the LP practices/techniques mentioned, and in the intersections the explanation of the empowering effects of I4.0 technologies on LP practices/techniques. Coding and data analysis were conducted manually by two authors to ensure inter-coder reliability (Duriau, Reger, and Pfarrer Citation2007). These two authors and an additional one who was assigned the role of ‘resident devil's advocate’ discussed and resolved any disagreements.
In the cross-case analysis, we then combined the above-mentioned tables into two summary tables with the results of all analysed cases concerning (a) the enabling effects of LP practices on I4.0 technologies (RQ1) (an excerpt of this table can be found in Appendix C – Table C.2) and (b) the empowering effect of I4.0 technologies on LP practices/techniques (RQ2). Finally, we combined the findings of the different cases and developed two summary cross-case analysis tables that are reported in Tables and . Two I4.0 technologies initially considered (i.e. smart bins and smart materials) were then removed from the list since none of the analysed cases implemented them (see Appendix A).
Table 4. The enabling effect of LP practices and techniques on I4.0 technologies or characteristics.
Table 5. The empowering effect of I4.0 technologies or characteristics on LP practices or techniques.
3.4. Validity and reliability
Various strategies suggested by Yin (Citation2003) and Eisenhardt (Citation1989) were adopted to enhance the validity and reliability of the results. To ensure construct validity, we triangulated empirical evidence from different sources, mainly interviews and archival data (such as annual reports, websites, and internal documents provided by the sampled companies), and from multiple respondents (see Section 3.2). Furthermore, the coding and data analysis were carried out by two researchers/authors separately and then compared (see Section 3.3); the few disagreements in the initial coding/analyses were widely discussed until a final agreed decision was reached. Internal validity was instead enabled by pattern matching, i.e. we iteratively compared the findings of the within-case analysis with the proposed model (whose final version is reported in Figure ), until when theoretical saturation was reached. To increase external validity (i.e. the generalizability of the results) we adopted a theoretical sampling method (Eisenhardt Citation1989; Patton Citation2002), explained in detail in Section 3.1, and we identified commonalities and differences in the analysed cases (Section 3.3). Finally, reliability was ensured by defining and following a case study protocol during field research and analysis (see Section 3.2 and 3.3) as well as by creating a case study database containing interview transcripts, field notes, and archival data.
4. Results
The results of the case studies are presented below in two paragraphs corresponding to the two possible directions of the relationship between LP and I4.0. Tables and summarise their characteristics. LP practices and techniques and I4.0 technologies or characteristics not adopted by any of the companies (see Appendix A) have been excluded from the analysis and are not present in the tables. If none of the companies recognised an enabling/empowering effect of a specific item of a paradigm on a specific one of the other paradigm, the relevant row shows the entry ‘no direct effect identified.’
4.1. The enabling effect of LP practices and techniques on I4.0 technologies
All the case studies investigated in this work confirmed the agreed statement of LP as a prerequisite of I4.0. Supporting this view, in all the cases LP has been in general adopted before I4.0. However, Case A reported a particular situation in which a premature introduction of I4.0 technology called for a step back to the LP techniques. Indeed, the company introduced Smart Machines and Collaborative Robots that increased productivity. Still, the layout was neither optimised nor standardised, so the increased production was often addressed to the wrong place, work-in-progress were piled up disorderly, and different lots were mixed up. Therefore, the company understood the need for the application of LP techniques for workplace and plant standardisation, namely 5S and VM.
Case D, E, and H understood that Lean Layout could be the basis in which including I4.0, indeed they applied cell design and then introduced Autonomous Robots or Smart Machines such as to facilitate the flow. For instance, Case D introduced Collaborative Robots in the U-shape cell in the position in which the line could be more balanced and the flow more continuous.
In summary, all the interviewees argued something similar to the statement of the manager of case H: ‘If a company does not apply LP before introducing technologies and digital innovations, the results would be just a digitalisation of existing wastes.’
Considering RQ1, the cases provided deep insights about the one-to-one enabling effects of LP techniques on I4.0 technologies. These enabling effects are summarised in Table and presented in detail below.
Some LP techniques can have an enabling effect on the introduction of I4.0 in general. First examples are represented by VSM and Kaizen (see cases C, E, F, G, and H). Indeed, VSM can be adopted to identify the pivotal areas within the value stream in which invest with any I4.0 for boosting the performance. For instance, case G, belonging to the pharmaceutical industry, stated that ‘through VSM, we understood the crucial point in our process (i.e. the cleanroom) and applied I4.0 technologies to optimise order recognition as well as set-up activities, speeding up the entire phase’.
Kaizen events, instead, involve people and facilitate their acceptance of I4.0 implementation creating shared occasions to bring in new ideas. Kaizen events are characterised by Multifunctional Teams that can adopt different Problem Solving and Elimination of Waste techniques to solve an inefficiency or to improve an area. This is what happened in case G, in which, during a Kaizen event, a Multifunctional Team adopted PDCA and A3 to design new carts for facilitating collaborative robots in picking plaques from shearing machines.
Kaizen and Multifunctional Team are often considered ‘soft’ LP techniques (Buer, Strandhagen, and Chan Citation2018), however the analysed case studies prove that their contribution to I4.0 can be very effective. Buer, Strandhagen, and Chan (Citation2018) classified as ‘soft’ also 5S and VM. However, also these other ‘soft’ techniques are proven to have strong enabling effects on I4.0. Indeed, according to case E:
The principle of everything at the right place, the so-called ‘set in order’ of 5S, is necessary for the picking and the supply of materials and products in an automated way. Searching for specific items in a chaotic warehouse trying to use real-time scanning with smartphones would lead to find more inconsistencies than matches.
In cases C, E, F, and H JIT and One-Piece-Flow were fundamental practices for enabling tracking and tracing of pieces in real-time, contributing to the transparency aimed by Vertical Integration, and, if present, Horizontal Integration. Indeed, as stated by case F:
The One-Piece-Flow principle and the connection of machines to the ERP-system both enable the introduction of a production flow that facilitates the I4.0 tracking and tracing of pieces in real-time. This increases transparency allowing to identify quality errors in early phases and consequently to reduce reworks and/or scraps.
In cases B, C, E, F, G, and H, VSM, instead enabled Vertical Integration. Indeed, it allowed defining the value-adding tasks to be standardised in electronic Job-Element-Sheets (JES) visible on monitors at the working stations. The electronic JES allow the operator to insert up-to-date information in the MES and ERP promoting the integration. Skipping from a phase to the following one after completion, the system can record the employed time. If workers stop the station because of defects or tools breakage, the system will record the machine downtime and its causes allowing the updating of KPIs and related root cause analyses.
In the same cases, KPIs to be calculated with Descriptive Analytics are defined by the LP concept of Overall Equipment Effectiveness (OEE). OEE defines data to be collected, namely the ones related to availability, performance, and quality. Case C took advantage of I4.0 creating a new business division that provides other companies with new software for the shop floor integration whose several monitoring parameters are based on this LP parameter.
Regarding Horizontal Integration, case E and H identified in the practices of keeping long term relationships with suppliers and involving them as a fundamental basis. Indeed, companies can trust suppliers in sharing platforms about the real-time status of material consumption and in letting them manage electronic Kanban. On the other hand, involved suppliers are more willing to give such services.
4.2. The empowering effect of I4.0 technologies or characteristics on LP techniques
Unlike the previous analysis, the cases proved that almost all the I4.0 technologies or characteristics have specific empowering effects on LP practices or techniques (Table ). Only Cybersecurity is excluded. Cloud has no empirical evidence to empower LP, but some interviewees recognised that it might empower the techniques belonging to the shop-floor management area and the relationships with suppliers and customers due to the on-demand shared data availability (case C, E, G, H).
All the cases identified the empowering effects of Smart Machines on Andon, SMED, and Standardised Work and the one of Product Simulation on Kaizen.
Smart Machines can detect anomalies in the process through sensors or cameras and call for repair via smartphones, smartwatches, or screens empowering Andon.
They can also receive information about materials, products, or work phase and prepare the right set-up of the machines empowering SMED. For instance, in case D, a Smart Machine represents the last and single station for the packaging phase. It recognises different orders with barcode scanning, and it gives workers the right components for the manual assembly of the boxes, drastically reducing the time for picking the right component.
Receiving information corresponding to the precise product and work phase allows displaying the relative JES on monitors. These virtual JES can be easily updated if any product changes configuration or if the line balancing finds a new configuration.
Product Simulation meets the purposes of continuous improvement; indeed, it allows to understand which configuration can reduce scraps, material consumption, and phases.
Regarding the other pillars, Autonomous Robots have an empowering effect on several LP techniques. Collaborative Robots can speed up One-Piece-Flow (cases E and H). For instance, in case H, a YuMi® robot is included in the production line for assembling disconnect switches one by one faster than human workers used to do, allowing a more balanced line. They can also empower employees’ commitment by handling all the repetitive and alienating activities they used to do (case A, E, G, and H). For instance, in case A Collaborative Robots substituted human workers in the repetitive action of picking metal sheets from the press. Only cases E and H adopted Autonomous AGV. Both the cases noticed an empowering effect on the supply of materials to workstations and supermarkets managed through Kanban. Case H adopted them also for the itinerant pre-assembly, which allows reducing machine tooling, empowering SMED and JIT production. Indeed, the AGV visits all the stations of the supermarket according to the identified route for the specific product, and the workers add every time a sequential component to work in progress. Furthermore, cases C, D, E, F, and H proved the empowering effect of Smart Machines on a Kanban system. This effect is due to the real-time track of the material consumption that makes instantly the Kanban system aware of the need for replenishment. Cases D and H identified in similar ways an empowering effect of Smart Machines on Poka-Yoke practices. Case D argued that:
The machine to mount clips on filter units recognizes the defect through a camera and opens only the gate of the wastebasket. Moreover, the machine does not allow to go on until the wrong piece is discarded. Once human errors were normal and understandable, now they do not happen anymore.
Process Simulation, instead, allows trying different layout configurations for choosing the one that better facilitates the process flow (cases C, D, E, G, H), or even One-Piece-Flow (cases C, D, E, H). Often, the company can have a virtual walk within the simulated environment for testing if the configuration allows a fluid process (cases G and H). It also allows for finding the right scheduling to respect JIT practices (cases E and H).
Case H is the only one that adopts DT (appendix A). The company identified an interesting empowering effect of DT on a multifunctional team, especially on teams with people located in different plants that share ideas and monitor the development of strategies and new products in a virtual simulated Obeya-Room. The company also highlighted an empowering effect of DT on TPM. Indeed, with machine DT Simulation, it is possible to try different maintenance solutions in a safe condition and thus allowing to forecast future maintenance. For instance, the company replicates an electric panel in VR for these purposes.
Machines integrated with MES/ERP allow real-time information record and sharing that empower Kanban (cases C, E, and H), JIT (cases C, D, E, and H), Andon (cases A, B, C, D, E, G, H), and Statistical Process Control (cases B, C, E, G, and H). Considering Kanban, material consumption is always recorded, and, in case of warehouse integration, it triggers pick-to-light systems (with smartphones or lights in the shelf). In the case of Autonomous AGV adoption, it directs the material handling. Due to this Vertical Integration, the flow is transparent, and there is real-time awareness of the capacity and availability of the machines that allow reacting better to plan deviations empowering JIT. The Real-Time Tracking can identify and immediately communicate anomalies or brake-downs respecting the Andon principle. In cases C and H, this Andon 4.0 also communicates through wearable smartwatches. Finally, data collection allowed by Vertical Integration allows calculating real-time KPIs (especially all the components of the OEE, namely availability, performance, and quality).
The Horizontal Integration, implying data shared with suppliers and/or partners through IIoT systems, can empower supplier involvement (cases E, G, and H) and the direct management of Kanban by the suppliers themselves (case H).
About IIoT, case G adopts RFID in presses for recognising the die and setting all the corresponding parameters. This practice reduces set-up time significantly empowering SMED. A similar match is performed by cases A, F, and H for the recognition of the right tool for the right machine, a kind of Poka-Yoke 4.0.
Another IIoT, the RTLS (real-time location system), is adopted by cases D, G, and H to map movements creating spaghetti charts or heat maps that give information about the need for a re-layout. Case E adopts pick-to-light in the warehouse facilitating the picking and hence empowering Kanban. Case C and H adopt scanning with smartphones to point machines for monitoring KPIs in real-time. Case C explained that
by pointing the keyboard of the machine, it is possible to access not only the real-time KPIs, but also the historical ones. So far, we are only displaying KPIs, but this latter aspect could be used in the future for maintenance purposes; indeed, the access to historical breakdowns directly on-site could be useful.
AR is fully adopted only by case H, in which glasses and smartphones give interactive maintenance instruction overlapped to the physical world. Case A has the project of introducing Microsoft HoloLens for receiving maintenance instructions by the machinery constructor.
Six out of the eight case studies, namely cases B, C, E, F, G, and H, perform Data Analytics, but only Descriptive/Diagnostics. This technology empowers the Statistical Process Control, indeed it provides real-time KPIs’ calculation, especially OEE in these cases. The analysis of the defects, the bottlenecks, and wastes promote continuous improvement (Kaizen), as well. All the KPIs and the performed analysis also give the material to display in the visual board during the 5-minutes meetings, empowering the employees’ commitment and the waste reduction techniques.
Only case H performs Predictive and Prescriptive Data Analytics and both to empower TPM's plans. Case H also exploits data adopting a multi-objective genetic algorithm for levelling the workstations workload according to the Heijunka principle, fundamental for the company.
The manager of case H highlighted an interesting effect of I4.0 on workers, indeed he stated:
The first attempts of LP implementation found resistance and mistrust. I4.0, instead, is making all the workers enthusiastic. Tablets, smartwatches, overlapped objects, autonomous AGV, etc. are accepted because everything has the shape of a game. In our company, this ‘gaming’ effect made the introduction of I4.0 technologies considerably less difficult than the adoption of traditional LP techniques.
5. Discussion
This section discusses the results of the one-to-one relationships between I4.0 technologies and LP techniques according to the six LP impact areas. Furthermore, the original results of our paper compared to previous studies are summarised in Section 5.7.
The results of the 8 case studies are summarised and depicted graphically in Figure . It follows the structure and symbology of a conventional Vale Stream Map to highlight how an analysis of individual cases has revealed the importance of lean and I4.0 throughout the value chain of a manufacturing company. The upper part of each impact area, coloured in light grey, depicts the enabling effects of LP techniques to I4.0 technologies (RQ1). The lower part with the white background visualises the empowering effects of I4.0 technologies to LP techniques (RQ2).
The underlined enabling or empowering effects represent the original findings not found by previous studies.
5.1. Manufacturing equipment and processes
Considering RQ1, our study gives evidence that VSM enables a Vertical Integration of manufacturing processes. Moreover, VSM can be considered as a prerequisite to identify the focal areas and processes of a company to support with I4.0 technology. More in detail, VSM allows identifying the value-adding tasks to be standardised in electronic JES. The interaction with them allows information update, as explained in section 4.1. Therefore, VSM sets the basis for the Integration of different processes as well as different information systems (MES and ERP) confirmed by recent works (e.g. Sony Citation2018; Kolberg, Knobloch, and Zühlke Citation2017).
Based on our results, Statistical Process Control has an enabling effect on Descriptive Analytics. This is in line with the outcomes of Jugulum (Citation2016) that highlighted the importance of data quality to perform high-quality analytics.
The cases show that 5S and VM bring rules and standardisation that allow an appropriate implementation of I4.0 technologies used for the management of materials, like Smartphones, AR as well as Autonomous Robots and AGVs. On the other hand, literature also reports that AR can assist in carrying out 5S more efficiently (Mayr et al. Citation2018), confirming mutual support between the two paradigms. Our results show also that a Lean Layout can be considered as a fundamental prerequisite to obtaining efficient routing of Autonomous AGV.
Considering RQ2, our results reveal that Autonomous Robots empower SMED, Poka-Yoke, Andon and Standardised Work. Considering the early detection of problems and error prevention, our findings confirm that Smart Machines can recognise an improper use of equipment in advance and therefore empower the LP concept of Poka-Yoke (Li, Ai, and Sun Citation2013). Similarly, the fact that Smart Machines can detect anomalies within the production process in real-time confirms that they empower Andon (Ma, Wang, and Zhao Citation2017). In this direction, our results reveal that Horizontal and Vertical System Integration empower Andon and Statistical Process Control. In particular, machines integrated with MES and ERP systems can immediately communicate anomalies or breakdowns as well as calculate KPIs in real-time. In line with research performed by Kolberg and Zühlke (Citation2015), in case of problems/failures, employees equipped with smartwatches are notified immediately and independently from their location. Similarly, according to our results, IIoT empowers Poka-yoke, SMED and Statistical Process Control. Here, companies reported using RFID to avoid the use of a wrong tool by a machine and that the RFID tag recognises the die allowing an autonomous set-up of all parameters. This is in line with the research of Mayr et al. (Citation2018), presenting the concept of Poka-Yoke 4.0. Besides, in our case studies, Additive Manufacturing allows the production of Poka-Yoke tools like jigs and clamps, which confirms Lu, Li, and Tian (Citation2015). Considering Statistical Process Control, companies reported the use of smartphones for real-time control of machine's KPIs. This confirms Georgakopolous et al. (Citation2016) who outlined the opportunity of IIoT to better understand production processes and how to improve them. Based on our results, Simulation enables TPM through a machine DT that tries different maintenance solutions in a safe environment to forecast optimal maintenance strategies. This is in line with Tuegel et al. (Citation2011), who proposed the use of DT for predicting the life of aircraft structures to assure their structural integrity. In addition, our research reveals that Process Simulation allows trying different layout configurations through a virtual walk in the simulated environment reaching a Lean Layout. This aspect enriches the research area concerning Process Simulation to reach an efficient layout configuration focusing on the evaluation of parameters like machine utilisation, waiting time and throughput obtained from different simulation experiments (Negahban and Smith Citation2014). Furthermore, our results reveal that global positioning systems like RTLS can map the movement within a factory advising about the need to implement a re-layout. Here, no other scientific works were identified stating this fact. Moreover, VR was found to be used for testing virtually different layout configurations allowing a fluid production flow and comfortable motions. Our results confirmed also the use of AR to train and give interactive maintenance assignments facilitating TPM (Mayr et al. Citation2018). Furthermore, Big Data Analytics empowers TPM by predicting maintenance actions as well as prescribing a maintenance plan. This supports Lee et al. (Citation2013) and Lee et al. (Citation2015), who found that a prediction capability allows maintenance to be more cost-effective. According to Khoshafian and Rostetter (Citation2015), digital prescriptive maintenance is empowered by Big Data and Analytics, avoiding potential failures that could have serious consequences for people, environment, and equipment. Moreover, Big Data and Analytics, and specifically Descriptive Diagnostics empower Statistical Process Control by providing a calculation of KPIs in real-time. Our results confirm also the use of the OEE indicator in manufacturing where advanced analytics can be used to pinpoint possible root causes for bottlenecks (Lade, Ghosh, and Srinivasan Citation2017).
5.2. Shop-floor management
Considering RQ1, our results show that One-Piece-Flow and JIT, promoting to obtain low levels of WIP, enable a tracking and tracing of products in real-time and hence, the Vertical Integration of processes within a company. Moreover, according to our results, continuous improvement processes facilitate the acceptance of I4.0 implementations.
Considering RQ2, our results reveal that Autonomous Robots empower One-Piece-Flow, Kanban and JIT. They support Kaspar and Schneider (Citation2015), who stated that Autonomous AGVs empower Kanban since material supply at the shop floor level can be realised by using a one-container-system without the necessity to refill several containers with the same material. Similarly, the results confirm that Autonomous AGV can further contribute to a JIT delivery to the workplace because the material to be refilled at the workstation arrives in the exact moment when it is needed, as stated by Mayr et al. (Citation2018). In this direction, scientific works by Kolberg and Zühlke (Citation2015) as well as Kolberg, Knobloch, and Zühlke (Citation2017) showed that Smart Machines empower Kanban mentioning the example of displays with graphical user interfaces connected to the production line and MES, which can drastically reduce the effort for updating conventional Kanban boards. In addition, our results reveal that Process Simulation allows trying different layout configurations through a virtual walk in the simulated environment reaching a One-Piece-Flow and even JIT production (Aigbedo and Monden Citation1996; Rosin et al. Citation2020).
Our research confirms the fact that Horizontal and Vertical System Integration and specifically Machines integrated with MES and ERP empower Kanban and JIT (Kolberg and Zühlke Citation2015; Kolberg, Knobloch, and Zühlke Citation2017; Mayr et al. Citation2018; Rosin et al. Citation2020) by continuously measuring material consumption and by having a real-time awareness of machines capacity and availability.
Similarly, our results confirm that IIoT empowers Kanban by using smartphones to support pick-to-light in warehouses, as stated by Mayr et al. (Citation2018).
Considering Big Data and Analytics, our results identified that Artificial Intelligence is used to level the workstations workload according to the Heijunka principle. This was also underpinned by Hou, Katayama, and Hwang (Citation2015) that used Genetic Algorithms to maximise line efficiency and minimise the variation of work overload time in a mixed-model straight/U-shaped assembly line using Heijunka.
5.3. Workforce management
Considering RQ1, problem-solving in a multifunctional team and contests for innovative ideas can enable the introduction of I4.0 in general. Here, no other enabling effect of LP on I4.0 were identified.
On the other hand, regarding RQ2, our results reveal that Simulation and specifically DT applications allow to create a virtual Obeya-Room to enable Multifunctional Teams with people located in different places. Here, no reference from other scientific works was identified that underpins this fact. Moreover, according to our results, Autonomous Robots empower Employees Commitment by relieving workers from repetitive and alienating tasks while increasing the level of occupational safety (Giuliani et al. Citation2010). Similarly, the case study analysis identifies that Big Data and Analytics and specifically Descriptive Diagnostics is used to display KPIs updated in real-time on visual boards, which can be used during the 5 min meeting empowering Employee Commitment.
5.4. New product development
Similarly, to the previous paragraph, this impact area contributes most to the RQ2. Although the literature identifies potential use of Additive Manufacturing for small series favouring Kanban and JIT, the cases mostly demonstrate its use for prototyping in the fields of Modularity and Customisation. Here, the case studies report the empowering effect of Additive Manufacturing for the production of small Customised pre-series, in line with Kietzmann, Pitt, and Berthon (Citation2015) who depicted the huge potential of Additive Manufacturing in the field of medical prosthetics. Moreover, the case studies adopted Additive Manufacturing to manufacture prototypes to test if Modular and standardised elements can meet customer requirements. Scientific references also underpin the empowering effect on Customisation (Zangiacomi et al. Citation2020), by substantiating the fact of 3D printers used to create prototypes that test whether standard and modular components can meet customer requirements. Here, especially the application in the field of using 3D printing for producing prosthetics can be mentioned (Bijadi et al. Citation2017; Hussain et al. Citation2018).
5.5. Supplier relationships
Based on our results and concerning RQ1, Long-Term Supplier relationships as well as Supplier Involvement enable a Horizontal Integration of supply chains, reporting the examples of shared platforms communicating the status of material consumption in real-time as well as suppliers that manage autonomously electronic Kanban. The companies reported that involved suppliers are more willing to give such a service. In literature, Camarinha-Matos, Fornasiero, and Afsarmanesh (Citation2017) highlight the importance of collaborative organisational structures, processes, and mechanisms as enablers of Horizontal Integrations of supply chains.
Considering RQ2, Horizontal and Vertical System Integration and specifically data shared with suppliers and/or partners through IIoT systems empower Supplier Involvement. The case studies reported examples of electronic platforms that share information about warehouses as well as other KPIs in real-time that allow suppliers to organise in a better way their deliveries. The usage of common knowledge-sharing platforms that favour Horizontal Integration of Suppliers has also be confirmed in literature by Chen (Citation2017).
5.6. Customer relationships
Answering to RQ2, the case study results report that Simulation and specifically Product Simulation empower Modularity and Customisation because it allows to understand if standardised as well as customised components meet specific customer requirements. Similarly, as reported in the field of New Product Development, Additive Manufacturing empowers the production of Customised pre-series and test if Modular and standardised prototypical elements can meet customer requirements.
5.7. Summary of original results
Figure summarises the findings of our paper, emphasising the results that, to the best of our knowledge, have not been found by previous studies.
Considering RQ1, the following results have not been found by previous studies. Statistical Process Control enables Descriptive Analytics. Elimination of Waste enables Autonomous Robots. 5S and VM enable Real Time scanning with smartphone, AR as well as Autonomous AGV. Lean Layout enables Autonomous AGV. One Piece Flow and JIT enable Vertical Integration.
Regarding RQ2, the following results have not been found by previous studies. Autonomous Robots empower SMED and Standardised Work. Horizontal and Vertical System Integration empower Statistical Process Control. IIoT empowers SMED and Lean Layout. AR empowers Lean Layout. Big Data and Analytics empower Waste Reduction. Additive Manufacturing empowers Modularity. Simulation empowers Multifunctional Team. Big Data and Analytics empower Employees Commitment.
6. Conclusion
6.1. Contribution to theory
This paper contributes to the production research literature on the relationships between I4.0 and LP in various significant ways.
First, we do a significant theory-building effort and propose a comprehensive framework that shows the one-to-one relationships between I4.0 technologies and lean practices and vice-versa (Figure ), focusing not only on the company itself but also on the whole value chain (Panizzolo Citation1998; Hines, Holweg, and Rich Citation2004; Pagliosa, Tortorella, and Ferreira Citation2019; Rojko Citation2017). This pairwise framework for a LP-I4.0 integration at a practice-technology level, which was missing in extant literature (Buer, Strandhagen, and Chan Citation2018; Rossini et al. Citation2019), represents in our view a significant theoretical advancement since it highlights in a synthetic but comprehensive way all the relevant constructs (i.e. I4.0 technologies and lean principles and practices) and their interrelationships (Gioia and Pitre Citation1990; Corley and Gioia Citation2011). Further research is however needed to further validate the framework, extend it to other geographical and industrial contexts, and empirically test all the hypothesised relationships in a wider, more heterogeneous and statistically significant sample (see Section 6.3).
Second, our paper confirms previous studies that postulate that the relationship between I4.0 technologies and LP is in both directions, i.e. (a) I4.0 affects LP and (b) LP affects I4.0 (e.g. Buer, Strandhagen, and Chan Citation2018; Pagliosa, Tortorella, and Ferreira Citation2019; Rossini et al. Citation2019), but it adds that the two paths have a different nature. More in detail, while LP enables I4.0 (creating opportunities for its development), I4.0 empowers LP (leading to the achievement of self-efficacy and of best version). This classification of enablement and empowerment (Dunst, Trivette, and Deal Citation1988) allows an easier and more direct understanding of the links between the two paradigms.
Third, we highlight a set of potential relationships between I4.0 and LP, that were not highlighted by previous research (see the underlined items in Figure ). Some examples of these relationships are the empowering effects of Autonomous Robots and IIoT on SMED and Standardised Work, of Big Data and Analytics on Employees Commitment, and of AR on Lean Layout, as well as the enabling effects of Statistical Process Control on Descriptive Analytics, of Lean Layout on Autonomous AGV, and of One Piece Flow and JIT on Vertical Integration (see Section 5.7 for further details). While these identified relationships deserve certainly to be tested on a wider and more heterogeneous sample, their identification might in our view guide future research in this field.
Finally, our study highlights the impact of I4.0 on the so-called ‘soft’ LP techniques, which according to a recent review (i.e. Buer, Strandhagen, and Chan Citation2018) represents a significant research gap. The analysis (Table ) found specific effects of I4.0 technologies or characteristics on Kaizen and Teamwork, and in general, insights from companies support the idea that the gaming atmosphere led by I4.0 can empower and make the adoption of all the LP techniques and practices more pleasant.
6.2. Contribution to practice
The findings of our study have also significant managerial implications.
First, we identify the key concepts in the I4.0 and LP fields (i.e. I4.0 technologies and LP principles/techniques, respectively) (see Tables and ). These concepts might be used by managers to assess the implementation level of I4.0 and/or LP in their companies.
Second, the proposed framework that summarises the one-to-one relationships between I4.0 technologies and lean practices and vice-versa (see Figure ) shows to managers all the possible synergies among the two manufacturing paradigms. It can be used as an assessment tool by both, companies that have already adopted one of the two paradigms, to exploit the possible links and synergies among I4.0 technologies and LP practices/techniques (or vice versa), and by companies that have adopted none of them and in this case to identify possible lacunae (or gaps) that should be addressed before implementing I4.0 (or LP). As an example, companies should re-design their plant layout (lean layout) before adopting autonomous AGV. Automating a (logistics) process that is not (yet) optimised/efficient would indeed lead only to limited performance improvement.
Third, the eight analysed cases allow us to present some insights and tangible experiences of the interdependence of LP and I4.0 in specific contexts, that can inspire managers in similar situations. For instance, managers can understand the right tools of both paradigms to deal with layout problems, with customisation's requirements, with material handling issues, etc.
6.3. Limitations and future research
This study presents some limitations. First, while the multiple case study (and the qualitative data analyses) are widely recognised as a valid research method for theory building purposes (Eisenhardt Citation1989; Voss, Tsikriktsis, and Frohlich Citation2002) and we performed several actions to enhance the validity and the reliability (see section 3), caution is needed to statistically generalise the results of our study to a wider population. Furthermore, some results were reported by a single case and perhaps they can be due to subjective situations dictated by the context. Nevertheless, the insights given by these companies, detailed in section 4, can provide interesting points of view that future research could verify.
The framework with the one-to-one relationships between Industry 4.0 technologies and Lean Production techniques (Figure ) should, therefore, be further validated with additional case studies in other contexts as well as empirically tested, for instance with a survey on a wider and statistically significant sample.
Moreover, once the relationships will be validated, there is the need to understand the implication they have in terms of performance, a pivotal aspect that can help companies to understand the importance and the measurable advantages of specific practices or technologies. Other studies can also deepen the understanding of the LP practices or the I4.0 technologies for which the sample here considered did not recognise any direct effect on specific items of the other paradigm. In addition, future studies could focus on a temporal perspective, adding, in the light of the one-to-one relationships depicted in this paper, the stages a company should follow in the LP-I4.0 transformation journey.
Second, case studies based on a retrospective longitudinal approach, like ours, are characterised by two potential problems: lack of memory and post-rationalization (Voss, Johnson, and Godsell Citation2016). We however adopted various strategies to minimise these issues (e.g. proper selection of interviewees and assessment of their knowledge about the topic and triangulation of evidence among different sources and multiple interviewees). Future research might employ ‘real’ longitudinal case studies or action researches to analyse the relationships between the two paradigms during the implementation of either Industry 4.0 or Lean production.
Third, the sample consisted of eight Italian manufacturing companies. While Italy is one of the most important manufacturing countries in Europe (together with Germany, France and UK) and has significantly invested in I4.0 in the last few years (e.g. in ‘Piano Nazionale Industria 4.0’), future research – both based on qualitative (e.g. case studies) and quantitative (e.g. survey) methods – should include a wider sample of companies in different countries and sectors in order to confirm/validate our results. Contextual factors (e.g. country, industry, level of I4.0 or LP adoption) that might potentially moderate the one-to-one relationships between Industry 4.0 technologies and Lean Production techniques could also be investigated in future research.
Supplemental Material
Download MS Word (54 KB)Acknowledgement
This work was supported by the European Union’s Horizon 2020 research and innovation program under the Marie Skłodowska Curie grant agreement No. 734713 (Project title: SME 4.0—Industry). The author(s) thank(s) the Department of Innovation, Research and University of the Autonomous Province of Bozen/Bolzano for covering the Open Access publication costs.
Disclosure statement
No potential conflict of interest was reported by the author(s).
Additional information
Notes on contributors
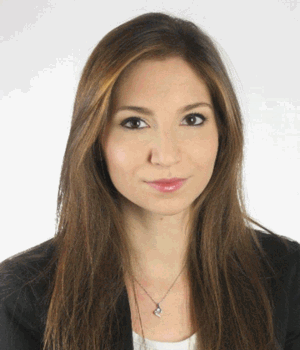
Maria Pia Ciano
Maria Pia Ciano is enrolled in the last year of the Ph.D. at LIUC - Cattaneo University. She holds a Master's Degree in Management Engineering for Industrial Production at the same university following the path of excellence in Lean Manufacturing. Her main topics of interest and research are: Lean manufacturing, design of production systems, Industry 4.0 and data analytics and, finally, evaluation of the advantages that a company can obtain from the digitization of the factory. She participates in projects about production flows, performance improvement, layouts redesign and Industry 4.0 adoption and sinergy with Lean. These projects gave her the opportunity to get in contact with other notions, such as innovation and circular economy, and a better understanding of specific contexts. She is facing the main topic of her PhD thesis, i.e., the link between Lean and industry 4.0, in collaboration with the Free University of Bozen and Fraunhofer IAO of Stuttgart.

Patrick Dallasega
Patrick Dallasega is an Assistant Professor of Factory Planning and Project Management at the Faculty of Science and Technology of the Free University of Bolzano (Italy). He studied at the Free University of Bolzano (Italy), at the Polytechnic University of Turin (Italy) and got his PhD at the University of Stuttgart (Germany). From 2005 until 2010, he worked in the fields of plant and factory planning in a local food processing industry. Later, from 2012 until 2015, he was employed as Research Associate at Fraunhofer Italia Research where he managed and executed several research projects for private and public customers. Since 2016, he has been employed as Assistant Professor at the Free University of Bozen-Bolzano. His main research interests are in, supply chain management, Industry 4.0, lean construction, lean manufacturing and production planning and control in MTO and ETO enterprises.
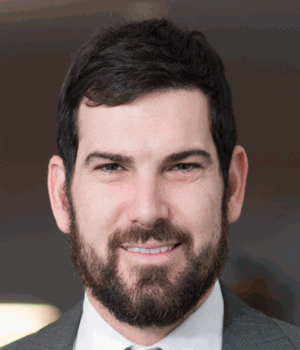
Guido Orzes
Guido Orzes is Assistant Professor in Management Engineering at the Free University of Bozen-Bolzano (Italy). He was also Honorary Research Fellow at the University of Exeter Business School (UK) and visiting scholar at the Worcester Polytechnic Institute (USA). His research focuses on international sourcing and manufacturing and their social and environmental implications. On these topics, has published more than 100 scientific works in leading operations management and international business journals (e.g., International Journal of Operations and Production Management, International Journal of Production Economics, International Business Review and Journal of Purchasing and Supply Management) as well as in conference proceedings and books. Dr. Orzes is involved in various EU-funded research projects on global operations management and Industry 4.0, including SME 4.0 - Industry 4.0 for SME (Marie Skłodowska-Curie RISE), European Monitor on Reshoring (funded by the EU agency Eurofound) and A21Digital Tyrol Veneto (Interreg V-A Italia-Austria). He is also Associate Editor of the Journal of Purchasing and Supply Management and member of the board of the European division of the Decision Science Institute.

Tommaso Rossi
Tommaso Rossi, PhD, is Full Professor of ‘Smart Factory’ at the Industrial Engineering School of LIUC – Cattaneo University, where he holds the courses of ‘Operations Management’ and ‘Data Science for Management’. He is lecturer of ‘Decision Support Systems for Designing and Managing Production Plants’ at Politecnico di Milano. His main research interests are related to: network design and opti-mization, lean manufacturing, finite capacity materials management policies, industry 4.0 and model-ling and simulation of production and logistics systems. On these topics, he has carried out projects for several companies and is author of more than 30 papers on classified international journals.
Notes
1 According to the definition of the European Union Commission (recommendation 2003/361/EC).
References
- Aigbedo, H., and Y. Monden. 1996. “A Simulation Analysis for Two-Level Sequence-Scheduling for Just-in-Time (JIT) Mixed-Model Assembly Lines.” International Journal of Production Research 34 (11): 3107–3124.
- Bai, C., A. Satir, and J. Sarkis. 2019. “Investing in Lean Manufacturing Practices: An Environmental and Operational Perspective.” International Journal of Production Research 57 (4): 1037–1051.
- Bevilacqua, M., F. E. Ciarapica, and I. De Sanctis. 2017. “Lean Practices Implementation and Their Relationships with Operational Responsiveness and Company Performance: an Italian Study.” International Journal of Production Research 55 (3): 769–794.
- Bhamu, J., and K. S. Sangwan. 2014. “Lean Manufacturing: Literature Review and Research Issues.” International Journal of Operations & Production Management 34 (7): 876–940.
- Bijadi, S., E. de Bruijn, E. Y. Tempelman, and J. Oberdorf. 2017. “Application of Multi-Material 3D Printing for Improved Functionality and Modularity of Open Source Low-Cost Prosthetics: A Case Study.” In Frontiers in Biomedical Devices, V001T10A003. Minneapolis, Minnesota, USA: American Society of Mechanical Engineers. Vol. 40672.https://doi.org/10.1115/DMD2017-3540.
- Bortolotti, T., S. Boscari, and P. Danese. 2015. “Successful Lean Implementation: Organizational Culture and Soft Lean Practices.” International Journal of Production Economics 160: 182–201.
- Buer, S. V., J. O. Strandhagen, and F. T. Chan. 2018. “The Link Between Industry 4.0 and Lean Manufacturing: Mapping Current Research and Establishing a Research Agenda.” International Journal of Production Research 56 (8): 2924–2940.
- Burritt, R., and K. Christ. 2016. “Industry 4.0 and Environmental Accounting: A New Revolution?” Asian Journal of Sustainability and Social Responsibility 1 (1): 23–38.
- Butollo, F., U. Jürgens, and M. Krzywdzinski. 2018. “From Lean Production to Industrie 4.0. More Autonomy for Employees?” (No. SP III 2018-303). WZB Discussion Paper.
- Büttner, S., H. Mucha, M. Funk, T. Kosch, M. Aehnelt, S. Robert, and C. Röcker. 2017. “The Design Space of Augmented and Virtual Reality Applications for Assistive Environments in Manufacturing: A Visual Approach.” In Proceedings of the 10th International Conference on PErvasive Technologies Related to Assistive Environments, 433–440. ACM, June.
- Camarinha-Matos, L. M., R. Fornasiero, and H. Afsarmanesh. 2017. “Collaborative Networks as a Core Enabler of Industry 4.0.” In Working Conference on Virtual Enterprises, 3–17. Cham: Springer.
- Chavan, S. R. 2014. Augmented Reality vs. Virtual Reality: Differences and Similarities.
- Chen, Y. 2017. “Integrated and Intelligent Manufacturing: Perspectives and Enablers.” Engineering 3 (5): 588–595.
- Ciano, M. P., R. Pozzi, T. Rossi, and F. Strozzi. 2019. “How IJPR has Addressed ‘Lean’: A Literature Review Using Bibliometric Tools.” International Journal of Production Research 57 (15-16): 5284–5317. doi:10.1080/00207543.2019.1566667.
- Corley, K. G., and D. A. Gioia. 2011. “Building Theory About Theory Building: What Constitutes a Theoretical Contribution?” Academy of Management Review 36 (1): 12–32.
- Cua, K. O., K. E. McKone, and R. G. Schroeder. 2001. “Relationships between Implementation of TQM, JIT, and TPM and Manufacturing Performance.” Journal of Operations Management 19 (6): 675–694.
- Culot, G., G. Nassimbeni, G. Orzes, and M. Sartor. 2020. “Behind the Definition of Industry 4.0: Analysis and Open Questions.” International Journal of Production Economics 226. doi:10.1016/j.ijpe.2020.107617.
- Dalenogare, L. S., G. B. Benitez, N. F. Ayala, and A. G. Frank. 2018. “The Expected Contribution of Industry 4.0 Technologies for Industrial Performance.” International Journal of Production Economics 204: 383–394.
- Davenport, T. H., and J. Kirby. 2016. “Just how Smart are Smart Machines?” MIT Sloan Management Review 57 (3): 21.
- Dilberoglu, U. M., B. Gharehpapagh, U. Yaman, and M. Dolen. 2017. “The Role of Additive Manufacturing in the era of Industry 4.0.” Procedia Manufacturing 11: 545–554.
- Dombrowski, U., T. Richter, and P. Krenkel. 2017. “Interdependencies of Industrie 4.0 & Lean Production Systems: A Use Cases Analysis.” Procedia Manufacturing 11: 1061–1068.
- Doolen, T. L., and M. E. Hacker. 2005. “A Review of Lean Assessment in Organizations: an Exploratory Study of Lean Practices by Electronics Manufacturers.” Journal of Manufacturing Systems 24 (1): 55–67.
- Dunst, C. J., C. M. Trivette, and A. G. Deal. 1988. Enabling and Empowering Families: Principles and Guidelines for Practice, Brookline Books. Cambridge, MA: Brookline Books.
- Duriau, V. J., R. K. Reger, and M. D. Pfarrer. 2007. “A Content Analysis of the Content Analysis Literature in Organization Studies: Research Themes, Data Sources, and Methodological Refinements.” Organizational Research Methods 10 (1): 5–34.
- Eisenhardt, K. M. 1989. “Building Theories From Case Study Research.” Academy of Management Review 14 (4): 532–550.
- Erol, S., A. Jäger, P. Hold, K. Ott, and W. Sihn. 2016. “Tangible Industry 4.0: A Scenario-Based Approach to Learning for the Future of Production.” Procedia CiRp 54: 13–18.
- Feldmann, C., and A. Gorj. 2017. 3D-Druck und Lean Production. Schlanke Produktionssysteme mit additiver Fertigung. Wiesbaden: Spriger Gabler.
- Flatt, H., S. Schriegel, J. Jasperneite, H. Trsek, and H. Adamczyk. 2016. “Analysis of the Cyber-Security of Industry 4.0 Technologies Based on RAMI 4.0 and Identification of Requirements.” In 2016 IEEE 21st International Conference on Emerging Technologies and Factory Automation (ETFA), 1–4. IEEE, September.
- Flynn, B. B., S. Sakakibara, and R. G. Schroeder. 1995. “Relationship Between JIT and TQM: Practices and Performance.” Academy of Management Journal 38 (5): 1325–1360.
- Frank, A. G., L. S. Dalenogare, and N. F. Ayala. 2019. “Industry 4.0 Technologies: Implementation Patterns in Manufacturing Companies.” International Journal of Production Economics 210: 15–26.
- Georgakopoulos, D., P. P. Jayaraman, M. Fazia, M. Villari, and R. Ranjan. 2016. “Internet of Things and Edge Cloud Computing Roadmap for Manufacturing.” IEEE Cloud Computing 3 (4): 66–73.
- Gil-Vilda, F., A. Sune, J. A. Yagüe-Fabra, C. Crespo, and H. Serrano. 2017. “Integration of a Collaborative Robot in a U-Shaped Production Line: A Real Case Study.” Procedia Manufacturing 13: 109–115.
- Gioia, D. A., and E. Pitre. 1990. “Multiparadigm Perspectives on Theory Building.” Academy of Management Review 15 (4): 584–602.
- Giuliani, M., C. Lenz, T. Müller, M. Rickert, and A. Knoll. 2010. “Design Principles for Safety in Human-Robot Interaction.” International Journal of Social Robotics 2 (3): 253–274.
- Goienetxea Uriarte, A., A. H. Ng, and M. Urenda Moris. 2020. “Bringing Together Lean and Simulation: A Comprehensive Review.” International Journal of Production Research 58 (1): 87–117.
- Hahn, G. J. 2020. “Industry 4.0: a Supply Chain Innovation Perspective.” International Journal of Production Research 58 (5): 1425–1441.
- Herzog, D., V. Seyda, E. Wycisk, and C. Emmelmann. 2016. “Additive Manufacturing of Metals.” Acta Materialia 117: 371–392.
- Hines, P., M. Holweg, and N. Rich. 2004. “Learning to Evolve.” International Journal of Operations & Production Management 24 (10): 994–1011.
- Hofmann, E., and M. Rüsch. 2017. “Industry 4.0 and the Current Status as Well as Future Prospects on Logistics.” Computers in Industry 89: 23–34.
- Hopp, W. J. 2018. “Positive Lean: Merging the Science of Efficiency with the Psychology of Work.” International Journal of Production Research 56 (1–2): 398–413.
- Hou, Z. Z., H. Katayama, and R. Hwang. 2015. “On Heijunka Design of Assembly Load Balancing Problem: Genetic Algorithm and Ameliorative Procedure-Combined Approach.” International Journal of Intelligent Information Systems 4 (2): 49.
- Huo, J., J. Zhang, and F. T. S. Chan. 2020. “A Fuzzy Control System for Assembly Line Balancing with a Three-State Degradation Process in the era of Industry 4.0.” International Journal of Production Research, 1–18. doi:10.1080/00207543.2020.1786186.
- Hussain, I., Z. Iqbal, M. Malvezzi, L. Seneviratne, D. Gan, and D. Prattichizzo. 2018. “Modeling and Prototyping of a Soft Prosthetic Hand Exploiting Joint Compliance and Modularity.” In 2018 IEEE International Conference on Robotics and Biomimetics (ROBIO), 65–70. IEEE.
- Ivanov, D., A. Dolgui, and B. Sokolov. 2019. “The Impact of Digital Technology and Industry 4.0 on the Ripple Effect and Supply Chain Risk Analytics.” International Journal of Production Research 57 (3): 829–846.
- Jasti, N. V. K., and R. Kodali. 2015. “Lean Production: Literature Review and Trends.” International Journal of Production Research 53 (3): 867–885.
- Jugulum, R. 2016. “Importance of Data Quality for Analytics.” In Quality in the 21st Century, 23–31. Cham: Springer.
- Kamble, S., A. Gunasekaran, and N. C. Dhone. 2020. “Industry 4.0 and Lean Manufacturing Practices for Sustainable Organisational Performance in Indian Manufacturing Companies.” International Journal of Production Research 58 (5): 1319–1337.
- Karlsson, C., and P. Åhlström. 1996. “Assessing Changes Towards Lean Production.” International Journal of Operations & Production Management 16 (2): 24–41.
- Kaspar, S., and M. Schneider. 2015. “Lean und Industrie 4.0 in der Intralogistik: Effizienzsteigerung durch Kombination der beiden Ansätze.” Productivity Management 20 (5): 17–20.
- Khoshafian, S., and C. Rostetter. 2015. Digital Prescriptive Maintenance. Internet of Things, Process of Everything, BPM Everywhere, 1–20.
- Kibria, M. G., K. Nguyen, G. P. Villardi, O. Zhao, K. Ishizu, and F. Kojima. 2018. “Big Data Analytics, Machine Learning, and Artificial Intelligence in Next-Generation Wireless Networks.” IEEE Access 6: 32328–32338.
- Kietzmann, J., L. Pitt, and P. Berthon. 2015. “Disruptions, Decisions, and Destinations: Enter the Age of 3-D Printing and Additive Manufacturing.” Business Horizons 58 (2): 209–215.
- Kipper, L. M., L. B. Furstenau, D. Hoppe, R. Frozza, and S. Iepsen. 2020. “Scopus Scientific Mapping Production in Industry 4.0 (2011–2018): A Bibliometric Analysis.” International Journal of Production Research 58 (6): 1605–1627.
- Kolberg, D., J. Knobloch, and D. Zühlke. 2017. “Towards a Lean Automation Interface for Workstations.” International Journal of Production Research 55 (10): 2845–2856.
- Kolberg, D., and D. Zühlke. 2015. “Lean Automation Enabled by Industry 4.0 Technologies.” IFAC-PapersOnLine 48 (3): 1870–1875.
- Kovács, G. 2020. “Combination of Lean Value-Oriented Conception and Facility Layout Design for Even More Significant Efficiency Improvement and Cost Reduction.” International Journal of Production Research 58 (10): 2916–2936. doi:10.1080/00207543.2020.1712490.
- Kovács, G., and S. Kot. 2016. “New Logistics and Production Trends as the Effect of Global Economy Changes.” Polish Journal of Management Studies 14 (2): 115–126.
- Lade, P., R. Ghosh, and S. Srinivasan. 2017. “Manufacturing Analytics and Industrial Internet of Things.” IEEE Intelligent Systems 32 (3): 74–79.
- Lasi, H., P. Fettke, H. G. Kemper, T. Feld, and M. Hoffmann. 2014. “Industry 4.0.” Business and Information Systems Engineering 6 (4): 239–242.
- Lee, J., H. D. Ardakani, S. Yang, and B. Bagheri. 2015. “Industrial big Data Analytics and Cyber-Physical Systems for Future Maintenance and Service Innovation.” Procedia Cirp 38: 3–7.
- Lee, J., E. Lapira, B. Bagheri, and H. A. Kao. 2013. “Recent Advances and Trends in Predictive Manufacturing Systems in Big Data Environment.” Manufacturing Letters 1 (1): 38–41.
- Li, Y., J. Ai, and C. Sun. 2013. “Online Fabric Defect Inspection Using Smart Visual Sensors.” Sensors 13 (4): 4659–4673.
- Liao, Y., F. Deschamps, E. D. F. R. Loures, and L. F. P. Ramos. 2017. “Past, Present and Future of Industry 4.0-a Systematic Literature Review and Research Agenda Proposal.” International Journal of Production Research 55 (12): 3609–3629.
- Liu, Z., N. Meyendorf, and N. Mrad. 2018, April. “The Role of Data Fusion in Predictive Maintenance Using Digital Twin.” In AIP Conference Proceedings, Vol. 1949, No. 1, 020023. AIP Publishing.
- Lu, Y. 2017. “Industry 4.0: A Survey on Technologies, Applications and Open Research Issues.” Journal of Industrial Information Integration 6: 1–10.
- Lu, B., D. Li, and X. Tian. 2015. “Development Trends in Additive Manufacturing and 3D Printing.” Engineering 1 (1): 85–89.
- Ma, J., Q. Wang, and Z. Zhao. 2017. “SLAE–CPS: Smart Lean Automation Engine Enabled by Cyber-Physical Systems Technologies.” Sensors 17 (7): 1500.
- Machado, C. G., M. P. Winroth, and E. H. D. Ribeiro da Silva. 2020. “Sustainable Manufacturing in Industry 4.0: an Emerging Research Agenda.” International Journal of Production Research 58 (5): 1462–1484.
- Matt, D. T., and E. Rauch. 2013. “Design of a Network of Scalable Modular Manufacturing Systems to Support Geographically Distributed Production of Mass Customized Goods.” Procedia CIRP 12: 438–443.
- Mayr, A., M. Weigelt, A. Kühl, S. Grimm, A. Erll, M. Potzel, and J. Franke. 2018. “Lean 4.0-A Conceptual Conjunction of Lean Management and Industry 4.0.” Procedia Cirp 72: 622–628.
- McLachlin, R. 1997. “Management Initiatives and Just-in-Time Manufacturing.” Journal of Operations Management 15 (4): 271–292.
- Mell, P., and T. Grance. 2011. The NIST Definition of Cloud Computing.
- Meng, X. 2019. “Lean Management in the Context of Construction Supply Chains.” International Journal of Production Research 57 (11): 3784–3798.
- Meudt, T., J. Metternich, and E. Abele. 2017. “Value Stream Mapping 4.0: Holistic Examination of Value Stream and Information Logistics in Production.” CIRP Annals – Manufacturing Technology 66 (1): 413–416.
- Miles, M. B., and M. Huberman. 1994. Qualitative Data Analysis: A Sourcebook of New Methods. Beverly Hills, CA: Sage Publications.
- Moeuf, A., S. Lamouri, R. Pellerin, S. Tamayo-Giraldo, E. Tobon-Valencia, and R. Eburdy. 2020. “Identification of Critical Success Factors, Risks and Opportunities of Industry 4.0 in SMEs.” International Journal of Production Research 58 (5): 1384–1400.
- Moeuf, A., R. Pellerin, S. Lamouri, S. Tamayo-Giraldo, and R. Barbaray. 2018. “The Industrial Management of SMEs in the era of Industry 4.0.” International Journal of Production Research 56 (3): 1118–1136.
- Möldner, A. K., J. A. Garza-Reyes, and V. Kumar. 2020. “Exploring Lean Manufacturing Practices’ Influence on Process Innovation Performance.” Journal of Business Research 106: 233–249.
- Mourtzis, D., S. Fotia, N. Boli, and E. Vlachou. 2019. “Modelling and Quantification of Industry 4.0 Manufacturing Complexity Based on Information Theory: a Robotics Case Study.” International Journal of Production Research 57 (22): 6908–6921.
- Mrugalska, B., and M. K. Wyrwicka. 2017. “Towards Lean Production in Industry 4.0.” Procedia Engineering 182: 466–473.
- Negahban, A., and J. S. Smith. 2014. “Simulation for Manufacturing System Design and Operation: Literature Review and Analysis.” Journal of Manufacturing Systems 33 (2): 241–261.
- Negri, E., L. Fumagalli, and M. Macchi. 2017. “A Review of the Roles of Digital Twin in cps-Based Production Systems.” Procedia Manufacturing 11: 939–948.
- Nguyen, T., Z. H. O. U. Li, V. Spiegler, P. Ieromonachou, and Y. Lin. 2018. “Big Data Analytics in Supply Chain Management: A State-of-the-art Literature Review.” Computers and Operations Research 98: 254.
- Núñez-Merino, M., J. M. Maqueira-Marín, J. Moyano-Fuentes, and P. J. Martínez-Jurado. 2020. “Information and Digital Technologies of Industry 4.0 and Lean Supply Chain Management: A Systematic Literature Review.” International Journal of Production Research 58 (16): 5034–5061.
- Ohno, T. 1988. Toyota Production System: Beyond Large-Scale Production. CRC Press.
- Pagliosa, M., G. Tortorella, and J. C. E. Ferreira. 2019. “Industry 4.0 and Lean Manufacturing: A systematic literature review and future research directions.” Journal of Manufacturing Technology Management. doi:10.1108/JMTM-12-2018-0446.
- Pan, M., J. Sikorski, C. A. Kastner, J. Akroyd, S. Mosbach, R. Lau, and M. Kraft. 2015. “Applying Industry 4.0 to the Jurong Island eco-Industrial Park.” Energy Procedia 75: 1536–1541.
- Panizzolo, R. 1998. “Applying the Lessons Learned From 27 Lean Manufacturers.: The Relevance of Relationships Management.” International Journal of Production Economics 55 (3): 223–240.
- Parente, M., G. Figueira, P. Amorim, and A. Marques. 2020. “Production Scheduling in the Context of Industry 4.0: Review and Trends.” International Journal of Production Research 58 (17): 5401–5431. doi:10.1080/00207543.2020.1718794.
- Patton, M. Q. 2002. Qualitative Research & Evaluation Methods. London: Sage Publications.
- Pessl, E., S. R. Sorko, and B. Mayer. 2017. “Roadmap Industry 4.0–Implementation Guideline for Enterprises. International Journal of Science.” Technology and Society 5 (6): 193–202.
- Pettersen, J. 2009. “Defining Lean Production: Some Conceptual and Practical Issues.” The TQM Journal 21 (2): 127–142.
- Piccarozzi, M., B. Aquilani, and C. Gatti. 2018. “Industry 4.0 in Management Studies: A Systematic Literature Review.” Sustainability 10 (10): 3821.
- Pozzi, R., F. Strozzi, T. Rossi, and C. Noè. 2018. “Quantifying the Benefits of the Lean Thinking Adoption by the Beer Game Supply Chain.” International Journal of Operational Research 32 (3): 350–363.
- Rojko, A. 2017. “Industry 4.0 Concept: Background and Overview.” International Journal of Interactive Mobile Technologies (iJIM) 11 (5): 77–90.
- Romero, D., J. Stahre, T. Wuest, O. Noran, P. Bernus, Å Fast-Berglund, and D. Gorecky. 2016. “Towards an Operator 4.0 Typology: A Human-Centric Perspective on the Fourth Industrial Revolution Technologies.” In International Conference on Computers and Industrial Engineering (CIE46) Proceedings, October.
- Rosin, F., P. Forget, S. Lamouri, and R. Pellerin. 2020. “Impacts of Industry 4.0 Technologies on Lean Principles.” International Journal of Production Research 58 (6): 1644–1661.
- Rossini, M., F. Costa, G. L. Tortorella, and A. Portioli-Staudacher. 2019. “The Interrelation Between Industry 4.0 and Lean Production: an Empirical Study on European Manufacturers.” The International Journal of Advanced Manufacturing Technology 102 (9–12): 3963–3976.
- Rüßmann, M., M. Lorenz, P. Gerbert, M. Waldner, J. Justus, P. Engel, and M. Harnisch. 2015. “Industry 4.0: The Future of Productivity and Growth in Manufacturing Industries.” Boston Consulting Group 9 (1): 54–89.
- Sakakibara, S., B. B. Flynn, R. G. Schroeder, and W. T. Morris. 1997. “The Impact of Just-in-Time Manufacturing and its Infrastructure on Manufacturing Performance.” Management Science 43 (9): 1246–1257.
- Sancha, C., F. Wiengarten, A. Longoni, and M. Pagell. 2020. “The Moderating Role of Temporary Work on the Performance of Lean Manufacturing Systems.” International Journal of Production Research 58 (14): 4285–4305.
- Sanders, A., C. Elangeswaran, and J. P. Wulfsberg. 2016. “Industry 4.0 Implies Lean Manufacturing: Research Activities in Industry 4.0 Function as Enablers for Lean Manufacturing.” Journal of Industrial Engineering and Management (JIEM) 9 (3): 811–833.
- Sanders, A., K. R. Subramanian, T. Redlich, and J. P. Wulfsberg. 2017. “Industry 4.0 and Lean Management–Synergy or Contradiction?” In IFIP International Conference on Advances in Production Management Systems, 341–349. Cham: Springer, September.
- Saunders, M., P. Lewis, and A. Thornhill. 2003. Research Methods for Business Students. 3rd ed. Harlow: Pearson Education Limited.
- Saurin, T. A., G. A. Marodin, and J. L. D. Ribeiro. 2011. “A Framework for Assessing the use of Lean Production Practices in Manufacturing Cells.” International Journal of Production Research 49 (11): 3211–3230.
- Sezen, B., I. S. Karakadilar, and G. Buyukozkan. 2012. “Proposition of a Model for Measuring Adherence to Lean Practices: Applied to Turkish Automotive Part Suppliers.” International Journal of Production Research 50 (14): 3878–3894.
- Shah, R., and P. T. Ward. 2003. “Lean Manufacturing: Context, Practice Bundles, and Performance.” Journal of Operations Management 21 (2): 129–149.
- Shah, R., and P. T. Ward. 2007. “Defining and Developing Measures of Lean Production.” Journal of Operations Management 25 (4): 785–805.
- Shrouf, F., J. Ordieres, and G. Miragliotta. 2014. “Smart Factories in Industry 4.0: A Review of the Concept and of Energy Management Approached in Production Based on the Internet of Things Paradigm.” In 2014 IEEE International Conference on Industrial Engineering and Engineering Management, 697–701. IEEE, December.
- Sony, M. 2018. “Industry 4.0 and Lean Management: A Proposed Integration Model and Research Propositions.” Production and Manufacturing Research 6 (1): 416–432.
- Spear, S., and H. K. Bowen. 1999. “Decoding the DNA of the Toyota Production System.” Harvard Business Review 77: 96–108.
- Stock, T., and G. Seliger. 2016. “Opportunities of Sustainable Manufacturing in Industry 4.0.” Procedia Cirp 40: 536–541.
- Thürer, M., Y. H. Pan, T. Qu, H. Luo, C. D. Li, and G. Q. Huang. 2016. “Internet of Things (IoT) Driven Kanban System for Reverse Logistics: Solid Waste Collection.” Journal of Intelligent Manufacturing 30 (7): 2621–2630.
- Tortorella, G. L., and D. Fettermann. 2018. “Implementation of Industry 4.0 and Lean Production in Brazilian Manufacturing Companies.” International Journal of Production Research 56 (8): 2975–2987.
- Tortorella, G. L., D. Fettermann, P. A. Cauchick Miguel, and R. Sawhney. 2020. “Learning Organisation and Lean Production: an Empirical Research on Their Relationship.” International Journal of Production Research 58 (12): 3650–3666.
- Tortorella, G. L., N. Pradhan, E. Macias de Anda, S. Trevino Martinez, R. Sawhney, and M. Kumar. 2020. “Designing Lean Value Streams in the Fourth Industrial Revolution era: Proposition of Technology-Integrated Guidelines.” International Journal of Production Research, 1–14. doi:10.1080/00207543.2020.1743893.
- Tuegel, E. J., A. R. Ingraffea, T. G. Eason, and S. M. Spottswood. 2011. “Reengineering Aircraft Structural Life Prediction Using a Digital Twin.” International Journal of Aerospace Engineering. doi:10.1155/2011/154798.
- Uhlemann, T. H. J., C. Lehmann, and R. Steinhilper. 2017. “The Digital Twin: Realizing the Cyber-Physical Production System for Industry 4.0.” Procedia Cirp 61: 335–340.
- Van Assen, M., and J. de Mast. 2019. “Visual Performance Management as a Fitness Factor for Lean.” International Journal of Production Research 57 (1): 285–297.
- Voss, C., M. Johnson, and J. Godsell. 2016. “Case Research.” In Research 23 Methods for Operations Management, edited by C. Karlsson, 2nd ed., 165–197. London: Routledge.
- Voss, C., N. Tsikriktsis, and M. Frohlich. 2002. “Case Research in Operations Management.” International Journal of Operations & Production Management 22 (2): 195–219.
- Wagner, T., C. Herrmann, and S. Thiede. 2017. “Industry 4.0 Impacts on Lean Production Systems.” Procedia CIRP 63: 125–131.
- Wang, B., J. Y. Zhao, Z. G. Wan, J. H. Ma, H. Li, and J. Ma. 2016. “Lean Intelligent Production System and Value Stream Practice.” Paper Presented at the 3rd International Conference on Economics and Management, ICEM 2016, Jiangsu.
- Womack, J. P., and D. T. Jones. 1994. “From Lean Production to the Lean Enterprise.” Harvard Business Review 72 (2): 93–103.
- Womack, J. P., and D. T. Jones. 1996. Lean Thinking. New York, NY: Simon and Schuster.
- Xu, L. D., E. L. Xu, and L. Li. 2018. “Industry 4.0: State of the art and Future Trends.” International Journal of Production Research 56 (8): 2941–2962.
- Yin, R. K. 2003. Case Study Research: Design and Methods. Thousand Oaks, CA: Sage Publications.
- Yin, Y., K. E. Stecke, and D. Li. 2018. “The Evolution of Production Systems From Industry 2.0 Through Industry 4.0.” International Journal of Production Research 56 (1–2): 848–861.
- Zangiacomi, A., E. Pessot, R. Fornasiero, M. Bertetti, and M. Sacco. 2020. “Moving Towards Digitalization: a Multiple Case Study in Manufacturing.” Production Planning & Control 31 (2–3): 143–157.