Abstract
Intermittent demand occurs commonly for spare parts in the heavy-duty vehicle industry. Demand uncertainty and intermittency pose challenges to demand forecasting by conventional models. Support vector machine (SVM) models have been observed to yield competitive accuracy with existing models. However, there are still limitations for basic SVM models. First, the time-consuming computation does not bring a statistically significant accuracy improvement. Second, the forecasting-based inventory performance has not been sufficiently explored. Third, scarce explanations of model robustness are offered for demand forecasting. We build an adaptive univariate SVM (AUSVM) model to forecast intermittent demand. Its effectiveness, compared to 12 existing models and an improved neural-network, is demonstrated by real-world data from a heavy-duty vehicle spare-part company. AUSVM has an apparent advantage in computation time over basic SVM and neural networks. The computational results of the heavy-duty vehicle case indicate that, compared to well-known parametric models, AUSVM achieves a statistically significant accuracy improvement and better inventory performance for the group of non-smooth demand series. Discussions are presented on why AUSVM works for demand forecasting and inventory control of heavy-duty vehicle spare parts. Several insights are revealed for practitioners in the heavy-duty vehicle industry.
Acknowledgements
This work was supported by the National Natural Science Foundation of China under grant number 71673188 and grant number 71401181 and partially supported by the China Postdoctoral Science Foundation under grant number 2018M640397. We thank LetPub for its linguistic assistance. We thank Dr Yong Zhang from Xi'an Jiaotong University and Dr Yongchang Wei from Zhongnan University of Economics and Law for their suggestions on the inventory performance simulation. The authors are also grateful to the editors and anonymous reviewers for their constructive comments and valuable suggestions.
Disclosure statement
No potential conflict of interest was reported by the author(s).
Additional information
Notes on contributors
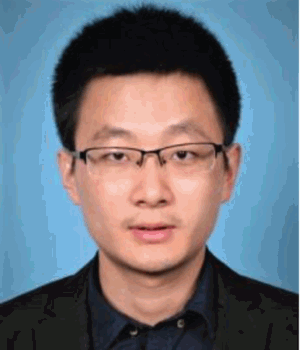
Peng Jiang
Dr. Peng Jiang is currently a Research Scientist at the Department of Systems Science, Institute of High Performance Computing (IHPC), Agency for Science, Technology and Research (A*STAR), Singapore. He received the bachelor's degree from Xi'an Jiaotong University, China in 2013 and the PhD degree from Shanghai Jiao Tong University, China in 2018. After that, he worked as postdoctoral fellow at Shanghai Jiao Tong University and National University of Singapore. His research interests include adaptive machine learning models and algorithms, the learning-based demand forecasting in supply chains, and system modelling for energy and environmental sustainability. His previous research has been published in the European Journal of Operational Research, Decision Support Systems, and IFAC World Congress.
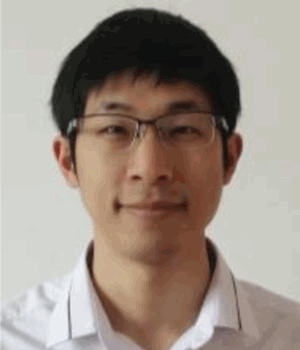
Yibin Huang
Mr. Yibin Huang is currently an Algorithm Engineer at Alibaba Group, China. He received both master's degree and the bachelor's degree from the Department of Industrial Engineering & Management at Shanghai Jiao Tong University, China in 2017 and 2020 separately. His research interests include data-driven models for time-series forecasting and probabilistic graphic models for fault diagnosis.
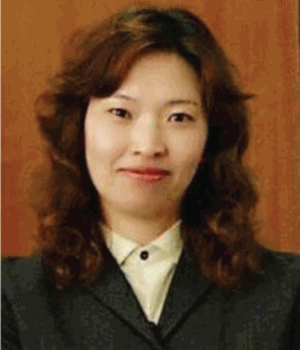
Xiao Liu
Dr. X. Liu received the M.Sc. degree in system engineering from the Northeastern University, China, in 1999, and the Ph.D. degree in industry system from the Université de technologie de Troyes (UTT), Troyes, France, in 2004. Currently, she is Professor in Industrial Engineering at Shanghai Jiao Tong University. Her research focuses on systems modelling and analysis, environmental and energy infrastructures resilient design method and optimisation, risk assessment, prediction, and robust optimisation. She has authored or coauthored more than one hundred technical papers, and currently serves on more than ten international research Journals.