Abstract
In this paper, we develop a maintenance model for systems subjected to multiple correlated degradation processes, where a multivariate stochastic process is used to model the degradation processes, and the covariance matrix is employed to describe the interactions among the processes. The system is considered failed when any of its degradation features hits the pre-specified threshold. Due to the dormancy of degradation-based failures, inspection is implemented to detect the hidden failures. The failed systems are replaced upon inspection. We assume an imperfect inspection, in such a way that a failure can only be detected with a specific probability. Based on the degradation processes, system reliability is evaluated to serve as the foundation, followed by a maintenance model to reduce the economic losses. We provide theoretical boundaries of the cost-optimal inspection intervals, which are then integrated into the optimisation algorithm to relieve the computational burden. Finally, a fatigue crack propagation process is employed as an example to illustrate the effectiveness and robustness of the developed maintenance policy. Numerical results imply that the inspection inaccuracy contributes significantly to the operating cost and it is suggested that more effort should be paid to improve the inspection accuracy.
Acknowledgements
The authors would like to thank the associate editor and three anonymous reviewers for their constructive comments that substantially help to improve the paper. This work was supported by the National Natural Science Foundation of China (61873096, 62073145, 71971181, 72002149), Guangdong Basic and Applied Basic Research Foundation (2020A1515011057), and Guangdong Technology International Cooperation Project Application (2020A0505100024).
Disclosure statement
No potential conflict of interest was reported by the author(s).
Additional information
Funding
Notes on contributors
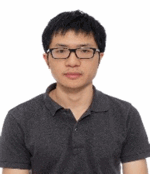
Bin Liu
Bin Liu received the B.S. degree in automation from Zhejiang University, China, and the Ph.D. degree in industrial engineering from the City University of Hong Kong, Hong Kong. He was a Postdoctoral Fellow with the University of Waterloo, Canada. He is currently a Lecturer with the Department of Management Science, University of Strathclyde, Glasgow, U.K. His research interests include risk analysis, reliability and maintenance modelling, decision-making under uncertainty, and data analysis.

Xiujie Zhao
Xiujie Zhao is an associate professor with the College of Management and Economics, Tianjin University, Tianjin, China. He received the B.E. degree from Tsinghua University, China, in 2013, the M.S. degree from the Pennsylvania State University, University Park, PA, USA, in 2015 and the Ph.D. degree from City University of Hong Kong in 2018, all in industrial engineering. His research interests include industrial statistics, degradation modelling, maintenance optimisation and risk management. His papers have appeared in European Journal of Operational Research, Journal of Quality Technology, IISE Transactions, among others.
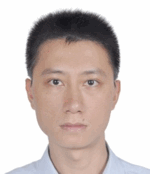
Yiqi Liu
Yiqi Liu was born in Haikou, China in 1983. He received B.S. and M.S. degrees in control engineering from the Chemical University of Technology, Beijing, in 2006 and 2009, respectively, a Ph.D. degree in control engineering from the South China University of Technology, Guangzhou, China in 2013. From 2013 to 2016, he was a lecturer at the South China University of Technology. Since 2016, he has been an Associate Professor in the Department of Automation at the South China University of Technology. He is the author of more than 60 peer-review articles. His research interests include soft sensors, fault diagnosis, control and wastewater treatment. He was a recipient of Marie Curie Actions Individual Fellowships in 2019, Hong Kong Scholar Fellowships in 2016, the Chinese Scholarship Council Award in 2011, and the Deutscher Akademischer Austausch Dienst Visiting Fellowships in 2015.

Phuc Do
Phuc Do is currently Associate Professor at Lorraine University (UL/CRAN laboratory) since 2011. He received his PhD in systems optimisation and dependability in 2008 from Troyes University of Technology (France). He defended his HDR (Habilitation à diriger des recherches) in 2019. His research interests include stochastic modelling for reliability prognostic, optimisation of maintenance policies (prescriptive maintenance, predictive maintenance, prognostics-based maintenance decision-making, opportunistic and dynamic grouping maintenance), reliability importance measures and their related applications. He has published over 70 research publications in international journals and conferences.