ABSTRACT
Quality control is essential for modern business success. The traditional statistical process control (SPC), however, lacks efficacy in current high-variety low-volume industrial practices since the historical reference data in Phase I are usually too scarce to infer the in-control process parameters accurately. To solve this ‘small data’ challenge, a novel Bayesian process monitoring scheme via transfer learning is proposed to facilitate a cross-product data sharing. In particular, a joint prior distribution is taken to explicitly capture the relatedness between the process data of two similar products, through which the process information can be transferred from one product (source domain) to improve the Bayesian inference for the other product (target domain). The posteriors can be derived analytically in closed forms by using generalised hypergeometric functions, thereby leading to a computationally efficient control chart for the online real-time monitoring in Phase II. A user-specified parameter is also provided to enable a better theoretical understanding of the transferability matter and a free practical control of the transferred information across domains. Extensive numerical simulations and real example studies of an assembly process validate the superiority of our proposed scheme in terms of both the false alarm rate and detection capability.
Acknowledgments
The authors greatly acknowledge the insightful comments from the two anonymous referees and the associate editor which have led to a great improvement of this paper.
Disclosure statement
No potential conflict of interest was reported by the author(s).
Additional information
Funding
Notes on contributors
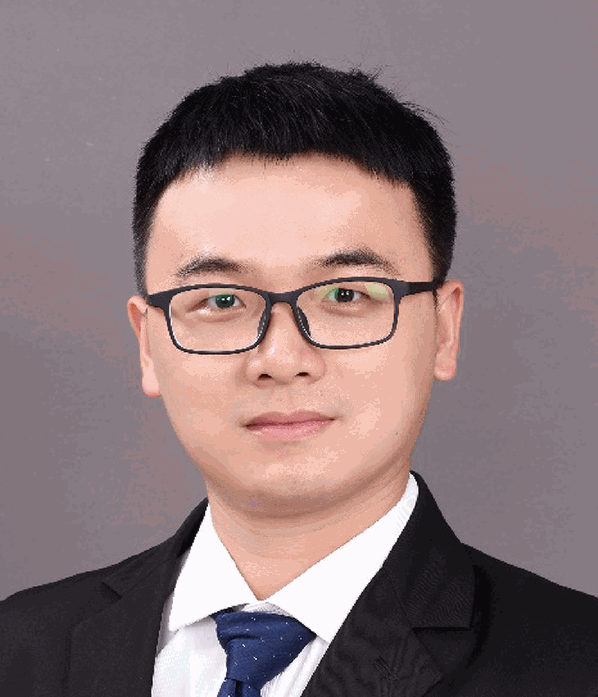
Kai Wang
Kai Wang received his Ph.D. degree in Industrial Engineering and Logistics Management from Hong Kong University of Science and Technology, Hong Kong, in 2018, and the B.S. degree in Industrial Engineering from Xi'an Jiaotong University, Shaanxi, China, in 2014. He is currently an Assistant Professor with the Department of Industrial Engineering, School of Management in Xi'an Jiaotong University, Xi'an China. His research focuses on statistical process control, industrial big data analytics, statistical machine learning and transfer learning.
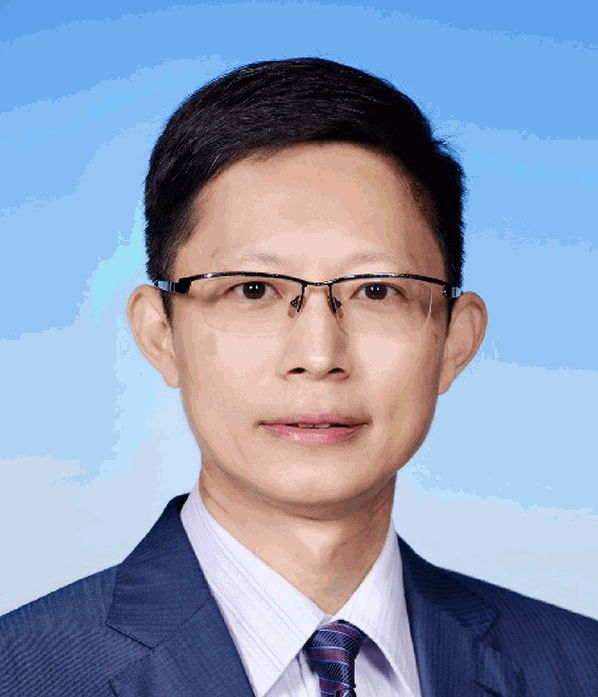
Fugee Tsung
Fugee Tsung received the Ph.D. and M.Sc. degrees from the University of Michigan, Ann Arbor, MI, USA., and the B.Sc. degree from National Taiwan University, Taipei, Taiwan. He is currently a Chair Professor with the Department of Industrial Engineering and Decision Analytics and the Director of the Quality and Data Analytics Laboratory in Hong Kong University of Science and Technology, Hong Kong. His research interests include quality analytics in advanced manufacturing and service processes, industrial big data and statistical process control, monitoring, and diagnosis.