Abstract
The multi-row facility layout problem (MRLP) is an important design problem often encountered in real life. Existing studies on MRLPs typically either ignore clearances between adjacent machines or consider only the minimum clearances. However, separating adjacent machines with clearances greater than the minimum ones may achieve lower material flow cost. In addition, current studies on MRLPs ignore the optimisation of layout area. In this paper, we study a multi-row facility layout problem with extra clearances (MRLP-EC), with the objectives of minimising material flow cost and layout area. A mixed integer programming formulation is established for MRLP-EC. A hybrid approach combining an improved multi-objective greedy randomised adaptive search procedure (mGRASP) and linear programming (LP) is proposed for the problem. The mGRASP is used to optimise machine sequences to obtain a set of non-dominated machine sequences. A segments-based dominance method is suggested to measure the dominance relationship of any pair of machine sequences. LP is used to optimise extra clearances between adjacent machines (i.e. the exact location of each machine) for each non-dominated machine sequence. The proposed approach is compared against an exact method and two multi-objective heuristics. Experiments show that the approach is effective for MRLP-EC and outperforms comparative approaches.
Acknowledgments
This work was supported in part by National Natural Science Foundation of China under Grants 61873040 and 61973042, and Beijing Natural Science Foundation under Grant 1202020, and BUPT Excellent Ph.D. Students Foundation.
Disclosure statement
No potential conflict of interest was reported by the author(s).
Additional information
Funding
Notes on contributors
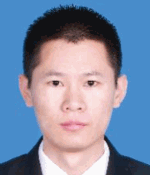
Xing Wan
Xing Wan received the master's degree in computer science and technology from Henan Polytechnic University, Jiaozuo, China, in 2017. He is currently pursuing the Ph.D. degree in computer science and technology from Beijing University of Posts and Telecommunications, Beijing, China. His research interests include facility layout and evolutionary computation.
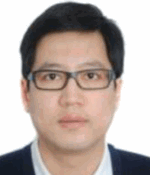
Xingquan Zuo
Xingquan Zuo is currently a Professor in School of Computer Science, Beijing University of Posts and Telecommunications. He received the Ph.D. degree in Control Theory and Control Engineering from Harbin Institute of Technology, Harbin, China, in 2004. From 2004 to 2006, he was a Postdoctoral Research Fellow in Automation Department of Tsinghua University. From 2012 to 2013, he was a Visiting Scholar in Industrial and System Engineering Department, Auburn University, AL, USA. His research interests are in optimisation and scheduling, data mining, artificial intelligence and intelligent transportation systems. He has published over 100 research papers in journals and conferences, two books and several book chapters.
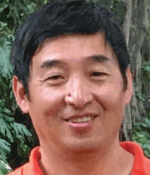
Xiaodong Li
Xiaodong Li received his B.Sc. degree from Xidian University, Xi'an, China, and Ph.D. degree in information science from University of Otago, Dunedin, New Zealand, respectively. He is a professor with the School of Science (Computer Science and Software Engineering), RMIT University, Melbourne, Australia. His research interests include machine learning, evolutionary computation, neural networks, data analytics, multiobjective optimisation, multimodal optimisation and swarm intelligence. He serves as an associate editor of the IEEE Transactions on Evolutionary Computation, Swarm Intelligence (Springer), and International Journal of Swarm Intelligence Research. He is a founding member of IEEE CIS Task Force on Swarm Intelligence, a vice chair of IEEE Task Force on Multi-modal Optimisation, and a former chair of IEEE CIS Task Force on Large Scale Global Optimisation. He is the recipient of 2013 ACM SIGEVO Impact Award and 2017 IEEE CIS ‘IEEE Transactions on Evolutionary Computation Outstanding Paper Award ’. He is an IEEE fellow.

Xinchao Zhao
Xinchao Zhao is currently a professor in School of Science, Beijing University of Posts and Telecommunications. He received B.S. and M.S. degrees from the Department of Mathematics, Capital Normal University, Beijing, China, in 1999 and 2002, Ph.D. degree from the Key Laboratory of Mathematics Mechanization, Chinese Academy of Sciences, Beijing, China, in 2005. From 2013 to 2014, he was a visiting scholar in School of Electrical Engineering and Computer Science, University of Essex, UK, and in School of Computer Science, University of Birmingham, UK. His research interests include evolutionary computation, swarm intelligence, operations research and engineering applications. He has published more than 100 research papers in EC&SI journals and conferences.