Abstract
The Line Feeding Problem (LFP) involves the delivery of components to the production area. Previous models minimise the delivery costs and optimally assign each component to a line feeding mode between line stocking, kitting, and sequencing but cannot provide easily comprehensible guidelines. We use the Classification And Regression Tree (CART) algorithm to develop, in a supervised way, a decision tree based on problems that are solved with a Mixed Integer Programming (MIP) model for training purposes. Based on selected attributes of the components and the manufacturing environment, the decision tree suggests a line feeding mode for every component. For a synthetically determined training and evaluation data set, we find that the classification tree can predict the line feeding mode with an average classification accuracy of 78.49%. After the decision tree is implemented and a line feeding mode is selected for each component, an infeasible solution might occur. We develop a repair approach that solves this problem with an average cost deviation from the optimal solution of 0.38%.
Acknowledgments
This work was partially supported by the Fondazione Ing. Aldo Gini. Furthermore, we would like to thank the editor and two anonymous reviewers for the careful reading of our article and Monica Reggiani for the opportunity to discuss our work, her suggestions, and comments.
Disclosure statement
No potential conflict of interest was reported by the author(s).
Additional information
Funding
Notes on contributors
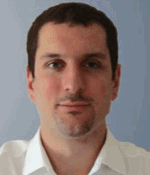
Francesco Zangaro
Francesco Zangaro is a Ph.D. candidate at the Ph.D. School of Mechatronics and Product Innovation Engineering of the University of Padua (UniPD) and at the Chair of Logistics and Supply Chain Management of the Technical University of Munich (TUM). His research interests are in part feeding optimisation and in-house logistics improvements.
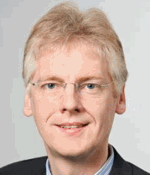
Stefan Minner
Stefan Minner is a Full Professor for Logistics and Supply Chain Management at the School of Management, Technical University of Munich (TUM). Currently, Stefan Minner is the Editor-in-Chief of the International Journal of Production Economics. His research interests are in business analytics, global supply chain design, transportation optimisation and inventory management.
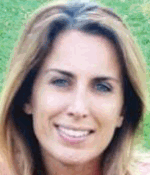
Daria Battini
Daria Battini is a full professor of Industrial Facilities Design and Industrial Logistics at the University of Padua (Italy) and she is the Coordinator of the Doctoral Course in Mechatronics and Product Innovation Engineering. She investigates supply chain design and management, industrial system optimisation, manufacturing system management for sustainability. She is the author and co-author of more than 100 publications in relevant international journals, about industrial logistics, material handling, materials management, supply chain, assembly system design.