ABSTRACT
The use of assistive technologies, such as digital instructions (DIs) and collaborative robots (cobots), can improve the productivity of assembly system. However, their implementation remains arbitrary. In this study, a decision support system based on tactical-level variables (i.e. throughput, operator and equipment cost, operation time and type) was proposed with the aim of suggesting when the introduction of assistive technologies becomes profitable. Four different assembly system configurations (i.e. manual assembly, manual assembly with the implementation of DIs, manual assembly with the implementation of cobots and manual assembly with the implementation of both DIs and cobots) were modelled by means of four different cost models and analysed in depth with a parametric analysis carried out by varying the tactical-level variables. The results suggested that, when the throughput is low, the introduction of cobots should be considered only in cases of high operation times, while the introduction of DIs and/or cobots is the best alternative when the throughput is high. Finally, the validity of the approach is proved by comparing the results suggested by the decision support system with those obtained from a case study.
Disclosure statement
No potential conflict of interest was reported by the author(s).
Additional information
Notes on contributors
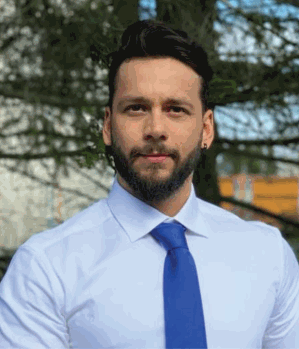
Mirco Peron
Mirco Peron is a researcher at the Department of Mechanical and Industrial Engineering (MTP) at NTNU (Norway) from May 2020, after receiving his PhD. His research topic is multi-disciplinary, involving industrial engineering and material science. He is working in the assessment of the mechanical performances of additively manufactured components and he is investigating the applicability of Industry 4.0 technologies, such as digital manufacturing, in the design of production and logistics systems.
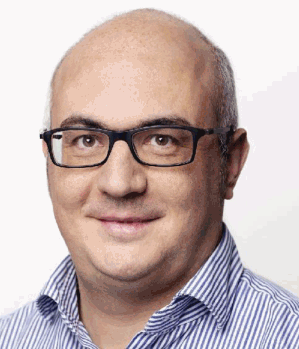
Fabio Sgarbossa
Fabio Sgarbossa is Full Professor of Industrial Logistics at the Department of Mechanical and Industrial Engineering (MTP) at NTNU (Norway) from October 2018. He was Associate Professor at University of Padova (Italy) where he also received his PhD in Industrial Engineering in 2010. He is leader of the Production Management Group at MTP and he is responsible of the Logistics 4.0 Lab at NTNU. He has been and he is involved in several European and National Projects. He is author and co-author of about 100 publications in relevant international journals, about industrial logistics, material handling, materials management, supply chain. He is member of Organising and Scientific Committees of several International Conferences, and he is member of editorial boards in relevant International Journals.
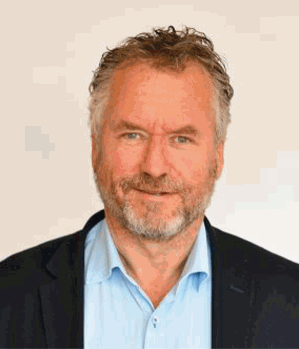
Jan Ola Strandhagen
Jan Ola Strandhagen is a Full Professor of at the Department of Mechanical and Industrial Engineering (MTP) at NTNU (Norway). His research deals with Manufacturing Strategy, Production Planning and Control and Simulation and Modelling, with the outputs of his research appearing in several peer-reviewed journals including computers in industry, International Journal of Production and Operations Management, and the International Journal of Production Research, Production Planning and Control among others. In the past Professor Strandhagen has been the research director at the NORMAN Research Centre, at SINTEF Technology and Society.