Abstract
Cellular structures (CSs) exhibit unique combinations of physical properties, including low weight, high structural strength, and substantial energy absorption, which could be useful in a variety of applications. Further, with the advent of additive manufacturing (AM), CSs are now easier to fabricate. While CSs and AM open up transformative opportunities, their potential for everyday use in industrial practice still lies largely idle. One of the major reasons is the lack of computational tools that allow us to automatically explore, verify, and optimise CSs and skin elements to create an optimised component that meets the exact specification. In this paper, we outline a periodic CS-based generative design pipeline that offers automated modelling, analysis, and inverse design solving of CS through the use of an integrated optimisation and finite-element analysis (FEA) framework. Specifically, a surrogate model-based optimisation scheme is proposed to design light-weight and high-strength functional parts by taking advantage of spatially varying conformal cubic periodic cellular structures.
Acknowledgments
All the research work was done at the University at Buffalo-SUNY.
Disclosure statement
No potential conflict of interest was reported by the author(s).
Notes
1 Aremu et al. (Citation2017) introduced three different internal CS creation strategies (sweeping, meshing, and trimming) in their work.
5
6 The three illustrative case study objects are also real-world products. For example, the fork end is a part of the Knuckle Joint, and the connecting rod is a part of a piston engine that connects the piston to the crankshaft. Our methods can be practical for real industrial designs.
7 The material used for the FE models is a general-purpose photocurable resin called from MarkerJuice Labs. The cured resin has Young's Modulus (E) of 209640 psi (1445.417 MPa) and Poisson's Ratio (ν) of 0.3272 (Wang and Rai Citation2016).
8 The optimisation starts with initial designs having ,
,
, and t = 0.08. To avoid zero volume fraction and period, we uniformly assign default volume faction of 0.35 (35%) and period of 1 to every unit cell when
and
. As shown in Figure , the initial structures are conformal CPCSs having uniform volume fraction of 0.35, period of 1, no rotations, and a shell with thickness of 0.08 in (2.032 mm).
9 The simulations in this paper are performed with consistent US units – i.e. inch and psi (). For the convenience of readers who are more familiar with SI units, we also convert the US units into consistent SI units – i.e. mm and MPa (N/mm
).
and
.
10 The generated volumetric mesh consists of all-tetrahedral elements. Our all-tetrahedral mesh generator is developed upon the distmesh library (Persson and Strang Citation2004), and a mesh optimiser (Taramón et al. Citation2019) is also included during the mesh generation to remove the zero-volume and sliver elements before FE simulations.
Additional information
Notes on contributors
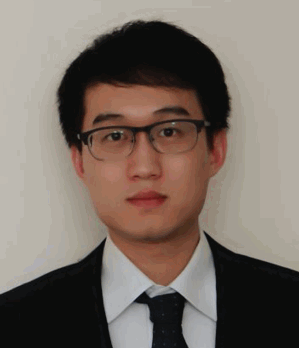
Jun Wang
Jun Wang is a Postdoctoral Associate of Mechanical Engineering at the University of Maryland, College Park. He received his Ph.D. and M.S. from the State University of New York at Buffalo and received his B.S. at Xi'an Jiaotong University, China. His research interests are Geometric Modeling, Simulation-Based Design, Data-Driven Design, and Generative Design. His current work is at the intersection of Machine Learning and Engineering Design. He has explored research areas in Computational Geometry, Design and Optimization, FEA, Additive Manufacturing, Machine Learning, and Computer Vision.
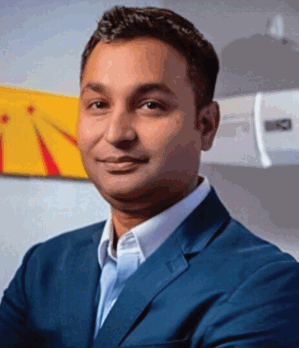
Rahul Rai
Rahul Rai received the B.Tech. degree from the National Institute of Foundry and Forge Technology (NIFFT), Ranchi, India, in 2000, the M.S. degree in manufacturing engineering from the Missouri University of Science and Technology (Missouri S&T), USA, in 2002, and the Ph.D. degree in mechanical engineering from The University of Texas at Austin, USA, in 2006. He is currently a Dean's Distinguished Professor in the Department of Automotive Engineering at the International Center for Automotive Engineering (ICAR) at Clemson University. Dr. Rai's research is focused on developing computational tools for Manufacturing, Cyber-Physical System (CPS) Design, Autonomy, Collaborative Human-Technology Systems, Diagnostics and Prognostics, and Extended Reality (XR) domains. By combining engineering innovations with methods from machine learning, AI, statistics and optimization, and geometric reasoning, his research strives to solve important problems in the above-mentioned domains. His research has been supported by NSF, DARPA, ONR, NSWC, DMDII, HP, NYSERDA, and NYSPII. He has authored over 100 articles to date in peer-reviewed conferences and journals covering a wide array of problems. He was a recipient of the 2009 HP Design Innovation and the 2017 ASME IDETC/CIE Young Engineer Award. He also received the 2019 PHM Society Conference Best Paper Award.