ABSTRACT
Ripple effect is a specific area of SC disruptions and a strong stressor to SC resilience. Research on the ripple effect analyses how one or more disruptive events propagate through the SC and impact its resilience and performance. The phenomenon of the ripple effect, immensely existing in practice, has received great research interest in recent years. Ripple effect management, modelling and assessment became visible research avenues with a growing number and scope of contributions. This Special Issue presents recent developments on the ripple effect in SCs. The Special Issue focuses on studies that address the ripple effect and provide a comprehensive picture of the state of the art and future perspectives. The methodologies comprise of mathematical optimisation, simulation, game theory, control theoretic, data-driven analytics, network complexity, reliability theory research, and empirical research. Even though a variety of valuable insights have been developed in this area in recent years, new research avenues and ripple effect taxonomies are identified for further exploring the ripple effect in the settings of the COVID-19 pandemic, SC viability, viable SC model, and reconfigurable SCs.
Supply chain engineering is one of the main issues in production research (Dolgui and Proth (Citation2010). Research on supply chain (SC) disruption management has focused on both empirical and analytical methodologies in understanding and appeasing risk (Ho et al. Citation2015; Ivanov Citation2018; Hosseini, Ivanov, and Dolgui Citation2019; Ivanov and Dolgui Citation2019; Yoon, Talluri, and Rosales Citation2020; Bier, Lange, and Glock Citation2020). Irrespective of the methodology utilised, majority of the work in this domain has concentrated on disruption impact on strategic/tactical/operational performance and stabilisation/recovery policies (Ivanov Citation2017, Citation2019; Li et al. Citation2019; Pournader, Kach, and Talluri Citation2020; Sawik Citation2020; Xu et al. Citation2020). A significant number of disruption studies have analysed how one or more events propagate through the SC and impact performance thus placing increased emphasis on the ripple effect in SC. Ripple effect is a specific area of SC disruptions and a strong stressor to SC resilience. The phenomenon of the ripple effect, immensely existing in practice, has received great research interest in recent years. The study by Ivanov and Dolgui (Citation2020c) provides the most recent overview of the ripple effect research in SCs. Ripple effect management, modelling and assessment became visible research avenues with a growing number and scope of contributions (Figure ).
We conducted a SCOPUS search based on the titles of the literature dealing with the ripple effect and in line with (Ivanov and Dolgui Citation2020c) as follows: (supply AND chain AND (ripple AND effect) OR (cascading AND failure) OR (risk AND transmission) OR (snowball AND effect) OR (risk AND propagation) OR (disruption AND propagation) OR (disturbance AND propagation) OR (structural AND dynamics). We limited the results to ‘Business and Management’, ‘Engineering’, and ‘Decision Science’ areas, and articles published in international journals. Figure shows that the number of articles published on the ripple effect-related topics in SC has been growing in 2012–2020.
The ripple effect occurs when a disruption, rather than remaining localised or being contained to one part of the SC, cascades downstream and impacts the performance of the entire SC (Dolgui, Ivanov, and Sokolov Citation2018). Ripple effect is followed by SC structural dynamics (Dolgui and Ivanov Citation2020; Ivanov Citation2018) and its impact might include lower revenues, delivery delays, loss of market share and reputation, etc., with adverse effects on the profitability of SC (Hosseini and Ivanov Citation2019; Gupta, Ivanov, and Choi Citation2020; Li et al. Citation2020). Some examples of the ripple effect are as follows:
Earthquake and tsunami in Japan in 2011 have disrupted multiple suppliers in the automotive industry and lead to production breaks and material shortages worldwide (Ivanov Citation2018).
In May 2017, production at BMW was disrupted as a consequence of a supply shortage of steering gears. BMW’s 1st tier supplier, Bosch, was unable to deliver the steering gears since an Italian 2nd tier supplier experienced production delays for certain steering parts due to internal machine breakdowns (Moetz, Stylos-Duesmann, and Ottoi Citation2019).
In Winter 2020, the COVID-19 virus outbreak resulted in production disruptions at many locations in China and missing deliveries from/to China impacting the global supply chains and even the stock values of many multi-national companies (Ghaffary and Molla Citation2020; Queiroz et al. Citation2020; Ivanov and Dolgui Citation2020a).
This Special Issue presents recent developments on the ripple effect in SCs. The Special Issue aims at delineating major features of the ripple effect and methodologies to mitigate the SC disruption propagations and recover. It focuses on studies that address the ripple effect and provide a comprehensive vision of the state of the art and future research perspectives. The methodologies comprise of mathematical optimisation, simulation, game theory, control theoretic, data-driven analytics, network complexity, reliability theory research, and empirical research. Even though a variety of valuable insights have been developed in this area in recent years, new research avenues and ripple effect taxonomies need to be identified for the near future.
Some recent studies on the ripple effect and the related SC resilience issues show the diversity of topics and methodologies applied to examination of this phenomenon, e.g. (Liberatore, Scaparra, and Daskin Citation2012; Mizgier, Jüttner, and Wagner Citation2013; Ghadge et al. Citation2013; Ivanov, Sokolov, and Dolgui Citation2014a, Citation2014b; Zeng and Xiao Citation2014; Swierczek Citation2014, Citation2016; Garvey, Carnovale, and Yeniyurt Citation2015; Han and Shin Citation2016; Sokolov et al. Citation2016; Tang et al. Citation2016; Levner and Ptuskin Citation2018; Scheibe and Blackhurst Citation2018; Ojha et al. Citation2018; Zhang, Chen, and Fang Citation2018; Pavlov et al. Citation2019; Sinha, Kumar, and Prakash Citation2019; Ivanov, Dolgui, and Sokolov Citation2019a; Dolgui, Ivanov, and Sokolov Citation2018, Citation2020a; Deng et al. Citation2019; Hosseini, Ivanov, and Dolgui Citation2020; Nguyen and Nof Citation2019; Garvey and Carnovale Citation2020; Kinra et al. Citation2020; Li and Zobel Citation2020). Recently, a Handbook on the Ripple Effects in the Supply Chain appeared showing a growing importance of this research field (Ivanov, Dolgui, and Sokolov Citation2019b). Besides, ripple effect has been seen as an important phenomenon in SC management evolution, e.g. in Industry 4.0 and digital SC systems (Ivanov and Dolgui Citation2020b; Ivanov et al. Citation2020; Ralston and Blackhurst Citation2020).
This Special Issue was established to furnish fresh and extend the existing insights in the ripple effect in SCs regarding the following questions:
Under what circumstances does one failure cause other failures?
Which structures of the SC are especially susceptible to the ripple effect?
What are the typical ripple effect scenarios and what are the most efficient and effective ways to respond to them?
provides an overview of the papers included in this special issue, their methodologies and major outcomes. In Table , the contributions shown in Table are divided into a three-level structure following the classification proposed by Ivanov and Dolgui (Citation2020c).
Given the reflections shown in Tables and , multiple ways to apply quantitative analysis to ripple effect modelling arise, e.g. considering:
disruption propagation in the SC;
dynamic recovery policies;
gradual capacity degradation and recovery;
multiple performance impact dimensions including financial and operational performance.
Table 1. Overview of the papers included in this special issue, their methodologies and major outcomes.
Table 2. Taxonomy of the existing studies on the ripple effect in SCs.
Finally, we point to some future research topics on the ripple effect in SCs which can be devoted to the following topics but are not limited to:
Network structures, ripple effect and resilience: What are the SC design structures that are more prone to the ripple effect?
Efficiency and resilience: contradictory or complementary goals in ripple effect mitigation?
Impact of digitalisation, Big Data analytics, and additive manufacturing on SC ripple effect and resilience: How to make use of available digital technology to improve resilience and mitigate the ripple effect?
Digital twins to manage the ripple effect: how to balance model-driven and data-driven approaches to mitigate and control the ripple effect?
Sourcing strategies and ripple effect
The interplay of flexibility and redundancy to ensure SC resilience and mitigate the ripple effect
Quantification and measuring of the ripple effect
Behavioural modelling of the ripple effect
Dynamic analysis of the SC ripple effect (simulation, control theory)
Interplay of financial and operational techniques in the ripple effect analysis
Ripple effect visualisation and control
Information coordination and ripple effect
Organisational theory and ripple effect
Competition and ripple effect
Sustainability and ripple effect: How to control the ripple effect with an efficient, sustainable resource utilisation?
Use cases with real company data allowing medium-term and long-term empirical observations of the ripple effects across the industry sectors and considering time lags between the disruption, ripple effect dispersal, and recovery
COVID-19 pandemic-driven ripple effects and SC viability.
The wider research community is invited to develop new studies in the above or closely related areas. Strong, new, and insightful conceptual and applications oriented studies on the ripple effect that add significantly to the existing body of knowledge will be particularly solicited in future IJPR publications. Especially, we encourage to explore the ripple effect in the setting of SC viability and viable SC model, reconfigurable SCs, and intertwined supply networks (Dolgui, Ivanov, and Sokolov Citation2020b; Hosseini, Ivanov, and Blackhurst Citation2020; Ivanov and Dolgui Citation2020a; Ivanov Citation2020).
Disclosure statement
No potential conflict of interest was reported by the author(s).
Additional information
Notes on contributors
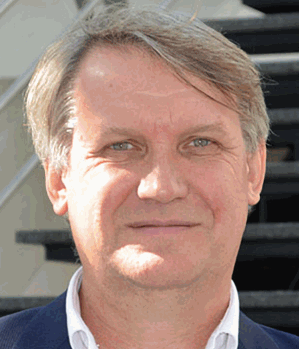
Alexandre Dolgui
Alexandre Dolgui is an IISE Fellow, Distinguished Professor, and the Head of Automation, Production and Computer Sciences Department at the IMT Atlantique, France. His research focuses on manufacturing line design, production planning and supply chain optimization. His main results are based on the exact mathematical programming methods and their intelligent coupling with heuristics and metaheuristics algorithms. He is the co-author of 5 books, the co-editor of 20 books or conference proceedings, the author of 249 refereed journal papers, 4 prefaces of books, 29 editorials and 37 book chapters as well as over 400 papers in conference proceedings. He is the Editor-in-Chief of the International Journal of Production Research (IJPR).
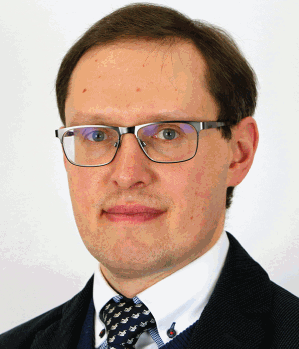
Dmitry Ivanov
Dmitry Ivanov is a professor of supply chain and operations management at Berlin School of Economics and Law (HWR Berlin). His publication list includes around 350 publications, including more than papers in international academic journals and a leading textbook Global Supply Chain and Operations Management. His main research interests and results span the resilience and ripple effect in supply chains, risk analytics, and digital twins. He co-edits International Journal of Integrated Supply Management (IJISM) and is an associate editor of the International Journal of Production Research (IJPR).
References
- Azaron, A., U. Venkatadri, and A. F. Doost. 2020. “Designing Profitable and Responsive Supply Chains Under Uncertainty.” International Journal of Production Research. doi:10.1080/00207543.2020.1785036.
- Bier, T., A. Lange, and C. H. Glock. 2020. “Methods for Mitigating Disruptions in Complex Supply Chain Structures: A Systematic Literature Review.” International Journal of Production Research 58 (6): 1835–1856. doi: 10.1080/00207543.2019.1687954
- Brintrup, A., V. Chauhan, and S. Perera. 2020. “The Relationship Between Nested Patterns and the Ripple Effect in Complex Supply Networks.” International Journal of Production Research.
- Choi, T.-M. 2020. “Facing Market Disruptions: Values of Elastic Logistics in Service Supply Chains.” International Journal of Production Research. doi:10.1080/00207543.2020.1722861.
- Deng, X., X. Yang, Y. Zhang, Y. Li, and Z. Lu. 2019. “Risk Propagation Mechanisms and Risk Management Strategies for a Sustainable Perishable Products Supply Chain.” Computers and Industrial Engineering 135: 1175–1187. doi: 10.1016/j.cie.2019.01.014
- Dolgui, A., and D. Ivanov. 2020. “Exploring Supply Chain Structural Dynamics: New Disruptive Technologies and Disruption Risks.” International Journal of Production Economics 229: 107886. doi: 10.1016/j.ijpe.2020.107886
- Dolgui, A., D. Ivanov, and M. Rozhkov. 2020a. “Does the Ripple Effect Influence the Bullwhip Effect? An Integrated Analysis of Structural and Operational Dynamics in the Supply Chain.” International Journal of Production Research 58 (5): 1285–1301. doi: 10.1080/00207543.2019.1627438
- Dolgui, A., D. Ivanov, and B. Sokolov. 2018. “Ripple Effect in the Supply Chain: An Analysis and Recent Literature.” International Journal of Production Research 56 (1–2): 414–430. doi: 10.1080/00207543.2017.1387680
- Dolgui, A., D. Ivanov, and B. Sokolov. 2020b. “Reconfigurable Supply Chain: The X-Network.” International Journal of Production Research 58 (13): 4138–4163. doi: 10.1080/00207543.2020.1774679
- Dolgui, A., and J.-M. Proth. 2010. Supply Chain Engineering: Useful Methods and Techniques. London: Springer.
- Dubey, R., A. Gunasekaran, S. J. Childe, S. F. Wamba, D. Roubaud, and C. Foropon. 2020. “Empirical Investigation of Data Analytics Capability and Organizational Flexibility as Complements to Supply Chain Resilience.” International Journal of Production Research, doi:10.1080/00207543.2019.1582820.
- Garvey, M. D., and S. Carnovale. 2020. “The Rippled Newsvendor: A New Inventory Framework for Modelling Supply Chain Risk Severity In The Presence of Risk Propagation.” International Journal of Production Economics 208: 107752. doi: 10.1016/j.ijpe.2020.107752
- Garvey, M. D., S. Carnovale, and S. Yeniyurt. 2015. “An Analytical Framework for Supply Network Risk Propagation: A Bayesian Network Approach.” European Journal of Operational Research 243 (2): 618–627. doi: 10.1016/j.ejor.2014.10.034
- Ghadge, A., S. Dani, M. Chester, and R. Kalawsky. 2013. “A Systems Thinking Approach for Modelling Supply Chain Risk Propagation.” Supply Chain Management: An International Journal 18 (5): 523–538. doi: 10.1108/SCM-11-2012-0366
- Ghaffary, S., and R. Molla. 2020. Apple, Facebook, and Google Stocks are Falling After Surge in Coronavirus Cases. Accessed February 27, 2020. https://www.vox.com/recode/2020/2/20/21145803/coronavirus-impacting-big-tech-business-apple-amazon-facebook-big-tech-impacted.
- Gholami-Zanjani, S. M., W. Klibi, M. S. Jabalameli, and M. S. Pishvaee. 2020. “A Robust Location-Inventory Model for Food Supply Chains Operating Under Disruptions with Ripple Effects.” International Journal of Production Research. in press
- Gupta, V., D. Ivanov, and T.-M. Choi. 2020. “Competitive Pricing of Substitute Products Under Supply Disruption.” Omega. doi:10.1016/j.omega.2020.102279.
- Han, J., and K. S. Shin. 2016. “Evaluation Mechanism for Structural Robustness of Supply Chain Considering Disruption Propagation.” International Journal of Production Research 54 (1): 135–151. doi: 10.1080/00207543.2015.1047977
- Ho, W., T. Zheng, H. Yildiz, and S. Talluri. 2015. “Supply Chain Risk Management: A Literature Review.” International Journal of Production Research 53 (16): 5031–5069. doi: 10.1080/00207543.2015.1030467
- Hosseini, S., and D. Ivanov. 2019. “Resilience Assessment of Supply Networks with Disruption Propagation Considerations: A Bayesian Network Approach.” Annals of Operations Research. doi:10.1007/s10479-019-03350-8.
- Hosseini, S., D. Ivanov, and J. Blackhurst. 2020. “Conceptualization and Measurement of Supply Chain Resilience in an Open-System Context.” IEEE Transactions on Engineering Management. doi:10.1109/TEM.2020.3026465.
- Hosseini, S., D. Ivanov, and A. Dolgui. 2019. “Review of Quantitative Methods for Supply Chain Resilience Analysis.” Transportation Research: Part E 125: 285–307. doi: 10.1016/j.tre.2019.03.001
- Hosseini, S., D. Ivanov, and A. Dolgui. 2020. “Ripple Effect Modeling of Supplier Disruption: Integrated Markov Chain and Dynamic Bayesian Network Approach.” International Journal of Production Research 58 (11): 3284–3303. doi: 10.1080/00207543.2019.1661538
- Ivanov, D. 2017. “Simulation-Based Ripple Effect Modelling in the Supply Chain.” International Journal of Production Research 55 (7): 2083–2101. doi: 10.1080/00207543.2016.1275873
- Ivanov, D. 2018. Structural Dynamics and Resilience in Supply Chain Risk Management. New York: Springer.
- Ivanov, D. 2019. “Disruption tails and revival policies: A simulation analysis of supply chain design and production-ordering systems in the recovery and post-disruption periods.” Computers and Industrial Engineering 127: 558–570. doi: 10.1016/j.cie.2018.10.043
- Ivanov, D. 2020. “Viable Supply Chain Model: Integrating Agility, Resilience and Sustainability Perspectives. Lessons from and Thinking Beyond the COVID-19 Pandemic.” Annals of Operations Research, doi:10.1007/s10479-020-03640-6.
- Ivanov, D., and A. Dolgui. 2019. “Low-Certainty-Need (LCN) Supply Chains: A New Perspective in Managing Disruption Risks and Resilience.” International Journal of Production Research 57 (15-16): 5119–5136. doi: 10.1080/00207543.2018.1521025
- Ivanov, D., and A. Dolgui. 2020a. “Viability of Intertwined Supply Networks: Extending the Supply Chain Resilience Angles Towards Survivability. A Position Paper Motivated by COVID-19 Outbreak.” International Journal of Production Research 58 (10): 2904–2915. doi: 10.1080/00207543.2020.1750727
- Ivanov, D., and A. Dolgui. 2020b. “A Digital Supply Chain Twin for Managing the Disruptions Risks and Resilience in the Era of Industry 4.0.” Production Planning and Control. doi:10.1080/09537287.2020.1768450.
- Ivanov, D., and A. Dolgui. 2020c. “OR-Methods for Coping with the Ripple Effect in Supply Chains During COVID-19 Pandemic: Managerial Insights and Research Implications.” International Journal of Production Economics, 107921. doi: 10.1016/j.ijpe.2020.107921
- Ivanov, D., A. Dolgui, and B. Sokolov. 2019a. “The Impact of Digital Technology and Industry 4.0 on the Ripple Effect and Supply Chain Risk Analytics.” International Journal of Production Research 57 (3): 829–846. doi: 10.1080/00207543.2018.1488086
- Ivanov, D., A. Dolgui, and B. Sokolov, eds. 2019b. Handbook of Ripple Effects in the Supply Chain. New York: Springer.
- Ivanov, D., B. Sokolov, and A. Dolgui. 2014a. “The Ripple Effect in Supply Chains: Trade-Off ‘Efficiency-Flexibility-Resilience’ in Disruption Management.” International Journal of Production Research 52 (7): 2154–2172. doi: 10.1080/00207543.2013.858836
- Ivanov, D., B. Sokolov, and A. Pavlov. 2014b. “Optimal Distribution (re)Planning in a Centralized Multi-Stage Network Under Conditions of Ripple Effect and Structure Dynamics.” European Journal of Operational Research 237 (2): 758–770. doi: 10.1016/j.ejor.2014.02.023
- Ivanov, D., C. S. Tang, A. Dolgui, D. Battini, and A. Das. 2020. “Researchers’ Perspectives on Industry 4.0: Multi-Disciplinary Analysis and Opportunities for Operations Management.” International Journal of Production Research. doi:10.1080/00207543.2020.1798035.
- Jahani, H., B. Abbasi, Z. Hosseinifard, M. Fadaki, and J. P. Minas. 2020. “Disruption Risk Management in Service-level Agreements.” International Journal of Production Research. doi:10.1080/00207543.2020.1748248.
- Kinra, A., D. Ivanov, A. Das, and A. Dolgui. 2020. “Ripple Effect Quantification by Supply Risk Exposure Assessment.” International Journal of Production Research 58 (19): 5559–5578. doi: 10.1080/00207543.2019.1675919
- Lee, B. W., J. Yoon, and S. J. Lee. 2020. “A Ripple Effect in Prehospital Stroke Patient Care.” International Journal of Production Research. doi:10.1080/00207543.2020.1825862.
- Lei, Z., M. K. Lim, L. Cui, and Y. Wang. 2020. “Modelling of Risk Transmission and Control Strategy in the Transnational Supply Chain.” International Journal of Production Research, 1–20. doi:10.1080/00207543.2019.1698782.
- Levner, E., and A. Ptuskin. 2018. “Entropy-Based Model for the Ripple Effect: Managing Environmental Risks in Supply Chains.” International Journal of Production Research 56 (7): 2539–2551. doi: 10.1080/00207543.2017.1374575
- Li, Y., K. Chen, S. Collignon, and D. Ivanov. 2020. “Ripple Effect in the Supply Chain Network: Forward and Backward Disruption Propagation, Network Health and Firm Vulnerability.” European Journal of Operational Research. doi:10.1016/j.ejor.2020.09.053.
- Li, Y., and C. W. Zobel. 2020. “Exploring Supply Chain Network Resilience in the Presence of the Ripple Effect.” International Journal of Production Economics. forthcoming
- Li, Y., C. W. Zobel, O. Seref, and D. C. Chatfield. 2019. “Network Characteristics and Supply Chain Resilience Under Conditions of Risk Propagation.” International Journal of Production Economics, doi:10.1016/j.ijpe.2019.107529.
- Liberatore, F., M. P. Scaparra, and M. S. Daskin. 2012. “Hedging Against Disruptions with Ripple Effects in Location Analysis.” Omega 40: 21–30. doi: 10.1016/j.omega.2011.03.003
- Liu, M., Z. Liu, F. Chu, F. Zheng, and C. Chu. 2020. “A New Robust Dynamic Bayesian Network Approach for Disruption Risk Assessment Under the Supply Chain Ripple Effect.” International Journal of Production Research. forthcoming
- Ma, S., Y. He, and R. Gu. 2020. “Dynamic Generic and Brand Advertising Decisions Under Supply Disruption.” International Journal of Production Research. doi:10.1080/00207543.2020.1812751.
- Mishra, D., Y. Dwivedi, N. Rana, and E. Hassini. 2020. “Evolution of Supply Chain Ripple Effect: A Bibliometric and Meta-Analytic View of the Constructs.” International Journal of Production Research. doi:10.1080/00207543.2019.1668073.
- Mizgier, K. J., M. P. Jüttner, and S. M. Wagner. 2013. “Bottleneck Identification in Supply Chain Networks.” International Journal of Production Research 51 (5): 1477–1490. doi: 10.1080/00207543.2012.695878
- Moetz, A., P. Stylos-Duesmann, and B. Ottoi. 2019. “Schedule Instability in Automotive Production Networks: The Development of a network-oriented Resequencing Method.” Proceedings of the 9th IFAC Conference on Manufacturing Modelling, Management and Control (MIM 2019), edited by Dmitry Ivanov, Alexandre Dolgui, Farouk Yalaoui, IFAC-PapersOnLine 52(13), 2810–2815.
- Nguyen, W. P. V., and S. Y. Nof. 2019. “Collaborative Response to Disruption Propagation (CRDP) in Cyber-Physical Systems and Complex Networks.” Decision Support Systems 117: 1–13. doi: 10.1016/j.dss.2018.11.005
- Ojha, R., A. Ghadge, M. K. Tiwari, and U. S. Bititci. 2018. “Bayesian Network Modelling for Supply Chain Risk Propagation.” International Journal of Production Research 56 (17): 5795–5819. doi: 10.1080/00207543.2018.1467059
- Özçelik, G., ÖF Yılmaz, and F. B. Yeni. 2020. “Robust Optimisation for Ripple Effect on Reverse Supply Chain: An Industrial Case Study.” International Journal of Production Research. doi:10.1080/00207543.2020.1740348.
- Pavlov, A., D. Ivanov, D. Pavlov, and A. Slinko. 2019. “Optimization of Network Redundancy and Contingency Planning in Sustainable and Resilient Supply Chain Resource Management Under Conditions Of Structural Dynamics.” Annals of Operations Research. doi:10.1007/s10479-019-03182-6.
- Pournader, M., A. Kach, and S. Talluri. 2020. “A Review of the Existing and Emerging Topics in Supply Chain Risk Management Literature.” Decision Sciences 51 (4): 867–919. doi: 10.1111/deci.12470
- Queiroz, M. M., D. Ivanov, A. Dolgui, and S. Fosso Wamba. 2020. “Impacts of Epidemic Outbreaks on Supply Chains: Mapping a Research Agenda Amid the COVID-19 Pandemic through a Structured Literature Review.” Annals of Operations Research. doi:10.1007/s10479-020-03685-7.
- Ralston, P., and J. Blackhurst. 2020. “Industry 4.0 and Resilience in the Supply Chain: A Driver of Capability Enhancement or Capability Loss?” International Journal of Production Research 58 (16): 5006–5019. doi: 10.1080/00207543.2020.1736724
- Sawik, T. 2020. Supply Chain Disruption Management. 2nd ed. New York: Springer.
- Scheibe, K. P., and J. Blackhurst. 2018. “Supply Chain Disruption Propagation: A Systemic Risk and Normal Accident Theory Perspective.” International Journal of Production Research 56 (1-2): 43–59. doi: 10.1080/00207543.2017.1355123
- Sinha, P., S. Kumar, and S. Prakash. 2019. “Measuring and Mitigating the Effects of Cost Disturbance Propagation in Multi-Echelon Apparel Supply Chains.” European Journal of Operational Research, doi:10.1016/j.ejor.2019.09.015.
- Sokolov, B., D. Ivanov, A. Dolgui, and A. Pavlov. 2016. “Structural Quantification of the Ripple Effect in the Supply Chain.” International Journal of Production Research 54 (1): 152–169. doi: 10.1080/00207543.2015.1055347
- Swierczek, A. 2014. “The Impact of Supply Chain Integration on the “Snowball Effect” in the Transmission of Disruptions: An Empirical Evaluation of the Model.” International Journal of Production Economics 157 (1): 89–104. doi: 10.1016/j.ijpe.2013.08.010
- Swierczek, A. 2016. “The “Snowball Effect” in the Transmission of Disruptions in Supply Chains: The Role of Intensity and Span of Integration.” International Journal of Logistics Management 27 (3): 1002–1038. doi: 10.1108/IJLM-08-2015-0133
- Tang, L., K. Jing, J. He, and H. E. Stanley. 2016. “Complex Interdependent Supply Chain Networks: Cascading Failure and Robustness.” Physica A 443: 58–69. doi: 10.1016/j.physa.2015.09.082
- Xu, S., X. Zhang, L. Feng, and W. Yang. 2020. “Disruption Risks in Supply Chain Management: A Literature Review Based on Bibliometric Analysis.” International Journal of Production Research 58 (11): 3508–3526. doi: 10.1080/00207543.2020.1717011
- Yoon, J., S. Talluri, and C. Rosales. 2020. “Procurement Decisions and Information Sharing Under Multi-tier Disruption Risk in a Supply Chain.” International Journal of Production Research 58 (5): 1362–1383. doi: 10.1080/00207543.2019.1634296
- Zeng, Y., and R. Xiao. 2014. “Modelling of Cluster Supply Network With Cascading Failure Spread and its Vulnerability Analysis.” International Journal of Production Research 52 (23): 6938–6953. doi: 10.1080/00207543.2014.917769
- Zhang, J., X. Chen, and C. Fang. 2018. “Transmission of a Supplier’s Disruption Risk Along the Supply Chain: A Further Investigation of the Chinese Automotive Industry.” Production Planning and Control 9 (9): 773–789. doi: 10.1080/09537287.2018.1470268