Abstract
In a re-entrant job shop (RJS), an entity can visit the same resource type multiple times; this is called re-entrancy, which occurs frequently in actual industries. Re-entrancy causes an NP-hard problem and is dominated by heuristics-based production control. The stochastic arrivals due to re-entrancy require the design of an appropriate dispatching rule. Reinforcement learning (RL) is an efficient technique for establishing robust dispatching rules; however, only a few cases that coordinate RL-based production control with a digital twin (DT) have been reported. This study proposes a novel production control model that applies a DT and horizontal coordination with RL-based production control. The requirements for dispatching in the RJS and coordination between RL and the DT were defined. A suitable architectural framework, service composition, and systematic logic library schema were developed to exploit the advanced characteristics of the DT and improve the existing production control methods. This study is an early case of coordinating RL and DT, and the findings revealed that RL policy networks should be imported in the creation procedures rather than being synchronised to the DT. The results should be a valuable reference for research on other types of RL-based production control with regard to horizontal coordination.
Acknowledgements
This work was partly funded by the Korea Institute of Advancement of Technology (KIAT) and Ministry of Trade, Industry and Energy (MOTIE) under Grant [P0009839, Cyber Physical Assembly and Logistics System in Global Supply Chain]; and the Technology Innovation Program under Grant [20003957, Simulation and Optimization of Logistics Operation of Big Data Based Manufacturing Line] funded by the MOTIE.
Disclosure statement
No potential conflict of interest was reported by the author(s).
Additional information
Funding
Notes on contributors
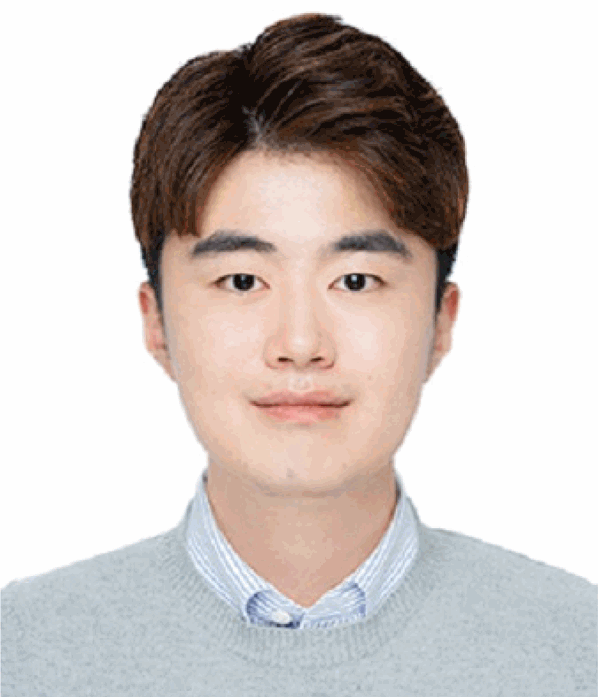
Kyu Tae Park
Park, Kyu Tae received the B.Sc. degree in Systems Management Engineering from Sungkyunkwan University. He is currently Ph. D. candidate in Department of Industrial Engineering at Sungkyunkwan University, and is currently senior researcher in MICUBE solution, Inc., Republic of Korea. His current research interests include cyber-physical production system, digital twin, distributed simulation, engineering interoperability, and production control.
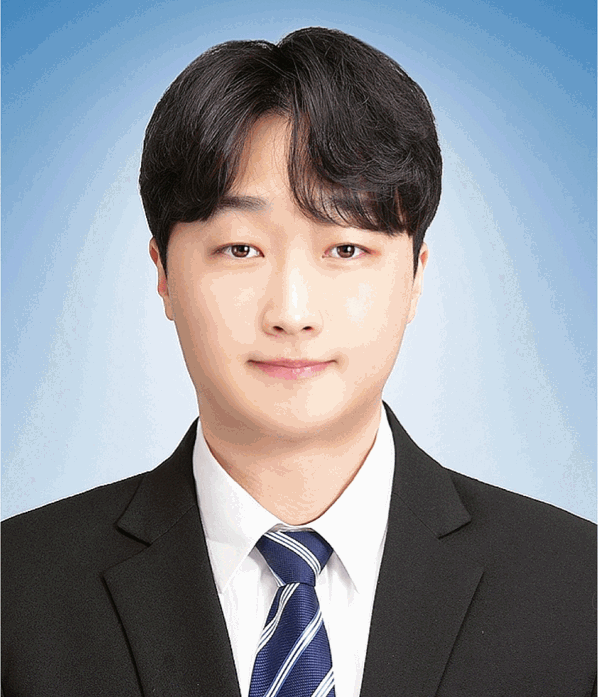
Seung-Woo Jeon
Jeon, Seung-Woo received the B.Sc. degree in Industrial Engineering from Gyeongsang National University, and the M.Sc. degree in Industrial Engineering from Sungkyunkwan University. His current research interests include cyber-physical production system, digital twin, automated modelling and simulation, reinforcement learning.
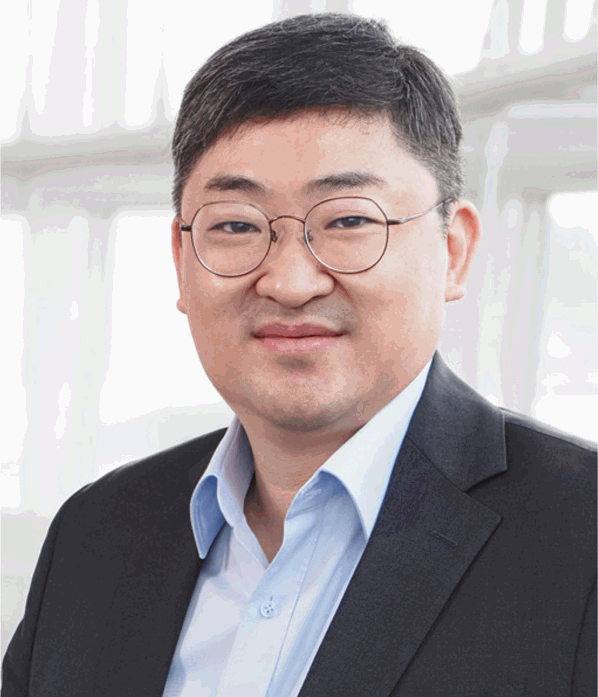
Sang Do Noh
Noh, Sang Do received the B.Sc. degree in Mechanical Engineering from KAIST, and the M.Sc. and Ph.D. degrees in Manufacturing Engineering from Seoul National University. He is currently a professor in Department of Industrial Engineering at Sungkyunkwan University. He served as a senior research engineer in Institute for Advanced Engineering, a visiting researcher in GM Global R&D Center, and a guest professor in KTH Royal Institute of Technology. His current research interests include CAD/CAPP/CAM, digital & virtual manufacturing, PLM, smart manufacturing, smart factory, cyber-physical production system, and digital twin.