Abstract
Estimates of production systems performance metrics, such as machine efficiency, e, system throughput, TP, lead time, LT, and work-in-process, WIP, are necessary for evaluating effectiveness of potential system modifications. Calculating these estimates requires machines MTBF and MTTR, which can be obtained by measuring up- and downtime realizations on the factory floor. A question arises: What is the smallest number of measurements required to ensure the desired accuracy of the induced estimates , (
), (
) and (
)? This paper provides an answer to this question in terms of serial lines with exponential machines. The approach is based on the theory of (
)-precise estimates (
) and (
), where
represents estimate's accuracy and
its probability. Specifically, the paper calculates (
)-precise estimates of
induced by (
) and (
), and evaluates the smallest number of machines' up- and downtime measurements, which ensure the desired precision of
. In addition, the paper develops a method for evaluating the smallest number of parts quality measurements to ensure (
)-precise estimate of machines' quality parameter q and the desired (
)-precise estimate of good parts throughput,
. The results obtained are intended for production systems managerial/engineering/research personnel as a tool for designing continuous improvement projects with analytically predicted outcomes.
Acknowledgments
The authors are grateful to three anonymous reviewers for their valuable comments and suggestions.
Disclosure Statement
Drs Semyon M. Meerkov and Liang Zhang have financial interests and/or other relationships with Smart Production Systems LLC, Ann Arbor, MI, USA.
Additional information
Funding
Notes on contributors
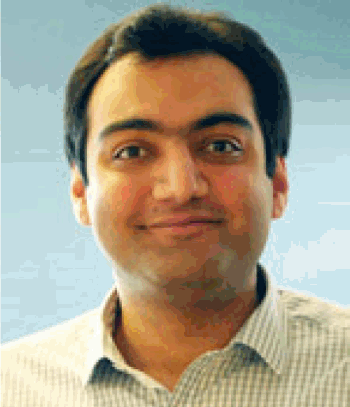
Pooya Alavian
Pooya Alavian was born in Mashhad, Iran in 1989. He received his B.S. (2011) and M.S. (2013) in Industrial Engineering from Sharif University, Tehran, Iran, and his Doctor of Engineering (2018) in Manufacturing Engineering from University of Michigan, Ann Arbor, MI. In 2018, he co-founded Smart Production Systems LLC, where he works as Chief Technology Officer on commercialization of the theory of Production Systems Engineering and his doctoral dissertation.
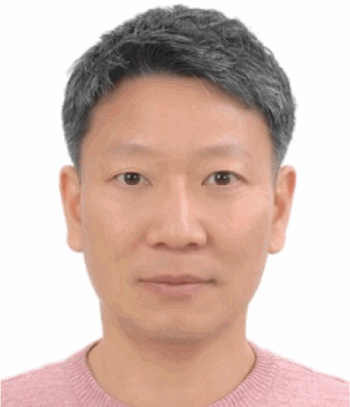
Yongsoon Eun
Yongsoon Eun received the B.A. degree in mathematics, and the B.S. and M.S.E. degrees in control and instrumentation engineering from Seoul National University, Seoul, Korea, in 1992, 1994, and 1997, respectively, and the Ph.D. degree in electrical engineering and computer science from the University of Michigan, Ann Arbor, MI, USA, in 2003. From 2003 to 2012, he was a Research Scientist with the Xerox Innovation Group, Webster, NY, USA. Currently he is a Professor with the Department of Information and Communication Engineering, DGIST, Korea. His research interests include control systems with nonlinear sensors and actuators, smart production systems, and resilient cyber-physical systems.
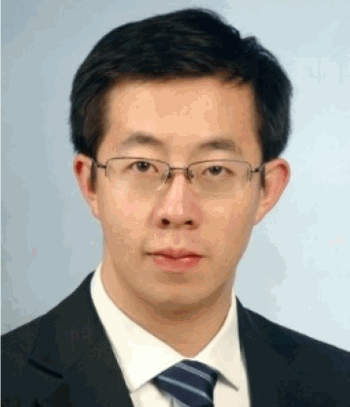
Kang Liu
Kang Liu received the B.S. degree in mechanical design, manufacturing and automation from Harbin Institute of Technology, Harbin, China, in 2016. He is currently pursuing the Ph.D. degree in electrical and computer engineering with the University of Michigan, Ann Arbor, MI, USA. His research interests include stochastic system modeling, analysis, optimization, control, and operations of production systems.

Semyon M. Meerkov
Semyon M. Meerkov received his MSEE degree from the Polytechnic of Kharkov, Ukraine, in 1962 and Ph.D. in Systems Science from the Institute of Control Sciences, Moscow, Russia, in 1966. He was with the Institute of Control Sciences until 1977. From 1979 to 1984 he was with the Department of Electrical and Computer Engineering, Illinois Institute of Technology, Chicago, IL. Since 1984 he has been a Professor at the Department of Electrical Engineering and Computer Science of the University of Michigan, Ann Arbor, MI. He held visiting positions at UCLA (1978-1979), Stanford (1991), Technion, Israel (Lady Davis Visiting Professor 1997-1998, 2008, and 2017), Tsinghua University, China (2008), and Ben-Gurion University, Israel (2011). He was the Editor-in-Chief of Mathematical Problems in Engineering (1995–2007), Department Editor for Manufacturing Systems of IIE Transactions (2002–2008) and Associate Editor of several other journals. Presently, he is on the Editorial Boards of Automation and Remote Control and International Journal of Production Research. He is a Foreign Member of the Russian Academy of Sciences and a Life Fellow of IEEE. His current research interests are in control of systems with nonlinear sensors and actuators; resilient monitoring and control under malicious attacks; production systems engineering; and smart production systems theory and applications. He is the President of Smart Production Systems LLC.
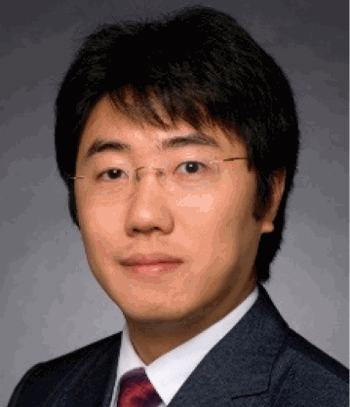
Liang Zhang
Liang Zhang received the B.E. and M.E. degrees from the Center for Intelligent and Networked Systems, Department of Automation, Tsinghua University, Beijing, China, in 2002 and 2004, respectively, and the Ph.D. degree in Electrical Engineering-Systems from the University of Michigan, Ann Arbor, MI, USA, in 2009. He was with the Department of Industrial and Manufacturing Engineering, University of Wisconsin-Milwaukee from 2009 to 2013.
He is currently an Associate Professor with the Department of Electrical and Computer Engineering, University of Connecticut, Storrs, CT, USA. His research interests include modeling, analysis, improvement, design, control, and energy-efficient operations of manufacturing systems.