Abstract
We address a robotic flow shop scheduling problem where two part types are processed on each given set of dedicated machines. A single robot moving on a fixed rail transports one part at a time, and the processing times of the parts vary on the machines within a given time interval. We use a reinforcement learning (RL) approach to obtain efficient robot task sequences to minimise makespan. We model the problem with a Petri net used for a RLenvironment and develop a lower bound for the makespan. We then define states, actions, and rewards based on the Petri net model; further, we show that the RL approach works better than the first-in-first-out (FIFO) rule and the reverse sequence (RS), which is extensively used for cyclic scheduling of a robotic flow shop; moreover, the gap between the makespan from the proposed algorithm and a lower bound is not large; finally, the makespan from the RL method is compared to an optimal solution in a relaxed problem. This research shows the applicability of RL for the scheduling of robotic flow shops and its efficiency by comparing it to FIFO, RS and a lower bound. This work can be easily extended to several other variants of robotic flow shop scheduling problems.
Disclosure statement
No potential conflict of interest was reported by the author(s).
Additional information
Funding
Notes on contributors
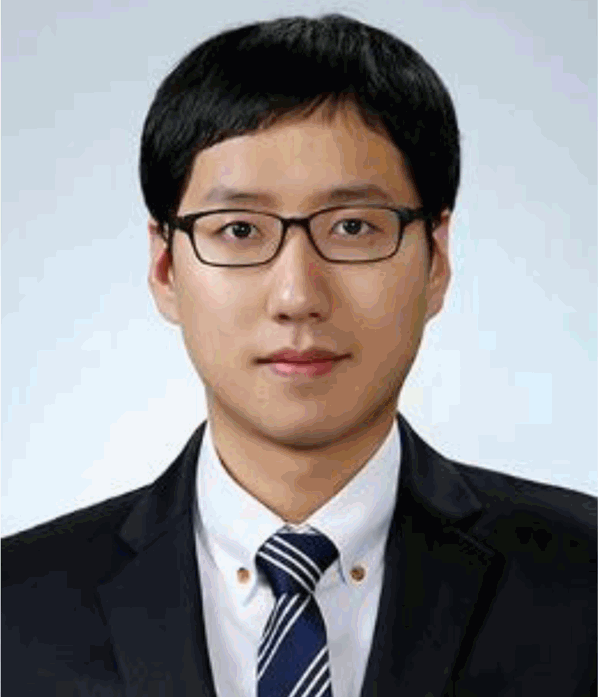
Jun-Ho Lee
Jun-Ho Lee received the B.S. and Ph.D. degrees from the Department of Industrial and Systems Engineering, Korea Advanced Institute of Science and Technology (KAIST), Daejeon, Republic of Korea, in 2008 and 2013, respectively. He was a Senior Researcher at Systems Engineering Team, Samsung Electronics, Republic of Korea, from 2013 to 2016, and was a Postdoctoral Researcher at the Department of Industrial and Systems Engineering, University of Wisconsin, Madison, WI, USA, from 2016 to 2017. He is currently an Assistant Professor with the School of Business, Chungnam National University, Republic of Korea. His research interests are in modelling, analysis, scheduling, and control of production and service systems.
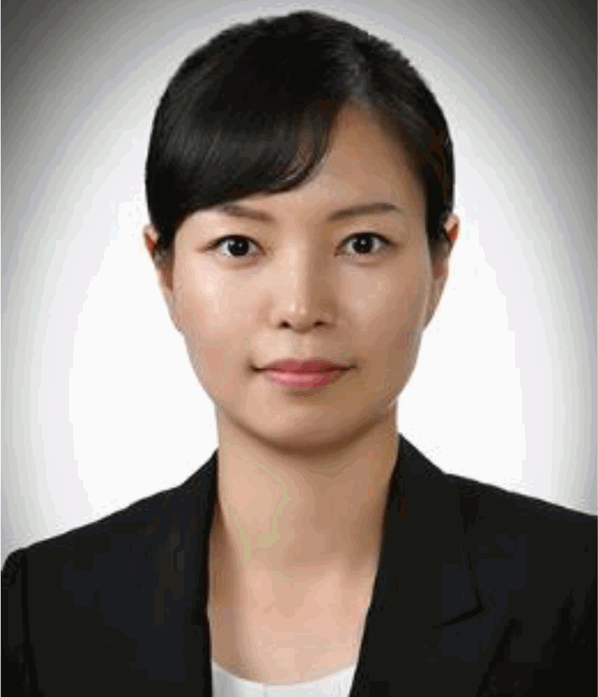
Hyun-Jung Kim
Hyun-Jung Kim received the Ph.D. degree in Industrial and Systems Engineering from Korea Advanced Institute of Science and Technology (KAIST), Daejeon, Republic of Korea in 2013. She was a Postdoctoral Researcher in the Department of Industrial Engineering & Operations Research, University of California, Berkeley, and was an Assistant Professor in the Department of Systems Management Engineering, Sungkyunkwan University, Republic of Korea. She is now an Assistant Professor with the Department of Industrial & Systems Engineering, KAIST. Her research interests include modelling, scheduling, and control of production systems.