Abstract
As E-Commerce develop, customers' tight delivery requirements are driving warehouses to operate more efficiently. This paper studies two types of class-based storage policies for a multi-dock unit-load warehouse: the within-aisle policy and the across-aisle policy. The aim is to determine the optimal class boundary and explore the dominance situation (i.e. which policy performs better in which situation). We first develop an expected response distance model for each policy based on a general layout of multiple docks. Then, closed-form optimal first zone boundary and warehouse dimension are given for the across-aisle policy. For within-aisle policy, an efficient algorithm is developed (based on the proved unimodality property) for obtaining its optimal first zone boundary. Besides, each policy's dominance situation is analysed for two typical situations. Numerical results show that both policies can bring up to 60% performance improvement compared with the random storage policy. Impacts of docks layout and warehouse dimensions on each policy's dominance situations are illustrated. It shows that the across-aisle policy performs better in the situation where the docks' and wall's centrelines are adjacent, and in the situation where the warehouse is narrow and deep. Managerial insights on policy selection and dock layout design are presented for warehouse managers.
Disclosure statement
No potential conflict of interest was reported by the author(s).
Additional information
Funding
Notes on contributors

Bilin Yu
Bilin Yu is a Master Candidate of Management Science and Engineering at the School of Management, University of Science and Technology of China (USTC). Her research interests include warehousing, material handling and logistics.
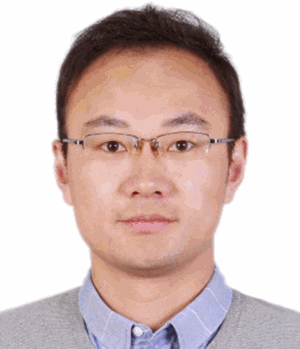
Hu Yu
Hu Yu is a Post-Doctor of Management Science and Engineering at the School of Management, University of Science and Technology of China (USTC). He received his B.S. degree in Information and Computing Science from Wuhan University of Technology, and Ph.D. degree in Management Science and Engineering from USTC in 2019. His current research interests are warehousing, algorithm design and optimisation.

Yugang Yu
Yugang Yu is Executive Dean and Yangtze Scholar Distinguished Professor of Logistics and Operations Management at the University of Science and Technology of China (USTC). He received his Ph.D. in Management Science and Engineering from USTC in 2003. His current research interests are warehousing, supply chain management, business analytics and business optimisation. He has published more than 100 papers in academic journals such as Manufacturing & Service Operations Management, Productions and Operations Management, Transportation Science, IISE Transactions, and International Journal of Production Research. His papers have been cited more than 3000 times, and Elsevier ranked him as one of ‘the most cited researchers in the Mainland of China’ from 2014 to 2019. His research results have also been patented several times in China. He is editorial board member of several journals such as Transportation Research Part E: Logistics and Transportation Review.