Abstract
Industry desires a digital thread of information that aligns as-designed, as-planned, as-executed, and as-inspected viewpoints. An experiment was conducted to test selected open data standards' ability to integrate the lifecycle stages of engineering design, manufacturing, and quality assurance through a thorough implementation of a small-scale model-based enterprise. The research team set out to answer: from design, through production, and final inspections, what are the hurdles that a manufacturer would face during the development of a fully linked and integrated information chain? The research team was not able to fully link all the required information, but value for the industry was still identified. This paper presents the results of the experiment, provides guidance on how to overcome or mitigate identified challenges, and discusses the benefits or incentives to be gained from tracing or linking information through multiple stages a product lifecycle.
Acknowledgments
We wish to thank Capvidia, Origin International, and Mitutoyo America Corporation for their generous support and use of their software for completing the inspection tasks of this project. We also wish to thank William Bernstein, Larry Maggiano, Vijay Srinivasan, and the journal peer-reviewers for their review and comments.
Disclosure statement
No potential conflict of interest was reported by the author(s).
Notes
1 The 2012 edition of ASME Y14.41 was selected because the latest edition was not publicly available at the time the models were generated in this work.
2 More information available at https://www.cax-if.org/
Additional information
Notes on contributors
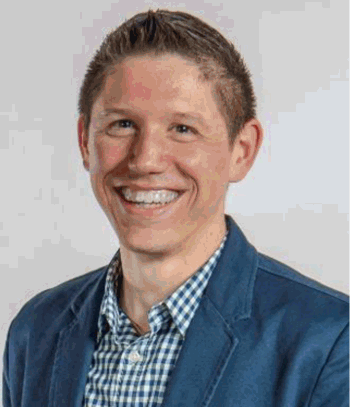
T. D. Hedberg
Dr. Thomas Hedberg, Jr. is a mission-area lead at the Applied Research Laboratory for Intelligence and Security (ARLIS). Dr. Hedberg joined ARLIS from the National Institute of Standards and Technology (NIST), where he was the Program Manager of the Model-Based Enterprise (MBE) program and the Co-Leader of the Smart Manufacturing Systems Test Bed, which earned him the U.S. Department of Commerce Gold Medal. Dr. Hedberg is the Chair of the ASME MBE Standards Committee, a member of the several ASME Y14 committees, and a past member of ISO TC184/SC4/WGs 11, 12, 15, 21 and the ISO TC184/SC4 Quality Committee. Dr. Hedberg earned a B.S. in Aeronautical and Astronautical Engineering from Purdue University, a M.Eng. in Engineering Management from the Pennsylvania State University, and a Ph.D. in Industrial and Systems Engineering from the Virginia Polytechnic Institute and State University.
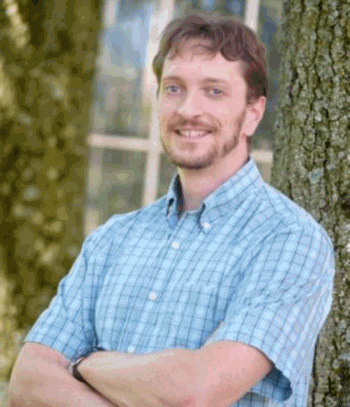
M. E. Sharp
Dr. Michael E. Sharp is a Reliability Engineer at the National Institute of Standards and Technology (NIST) located in Gaithersburg, MD. He received a B.S (2007), M.S. (2009), and Ph.D. (2012) in Nuclear Engineering from the University of Tennessee, Knoxville, TN, USA. His research interests include signal analytics, machine learning, artificial intelligence, optimisation, and natural language processing. Michael has worked on a wide array of projects including image processing for elemental material recognition, navel reliability monitoring, and manufacturing robotics diagnostic monitoring. He currently works with the NIST Systems Integration Division for Smart manufacturing.
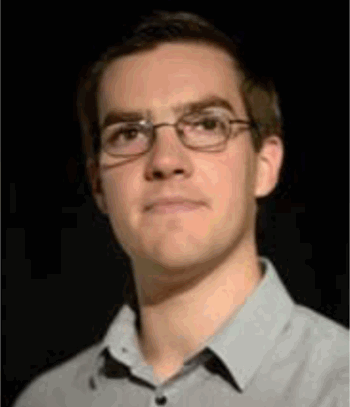
T. M. M. Maw
Dr. Toby Maw is a Senior Research Engineer in the Digital Engineering group at the Manufacturing Technology Centre (MTC). His research background stemmed from a PhD in experimental physics from Loughborough University in the field of the characterisation of novel materials. He has 9 years' metrology experience having worked at Nikon Metrology for 3 years in the coordinate measurement machine software development team before joining the MTC, where he now leads the Metrology 4.0 research area within the Metrology and NDT department. Toby has managed and technically led cross disciplinary teams in projects across multiple sectors, specialising in the areas of Digital Manufacturing, Digital Measurement Integration and Model Based Enterprise (MBE).

M. M. Helu
Dr. Moneer Helu is the Leader of the Life Cycle Engineering Group in the Systems Integration Division of the Engineering Laboratory at the National Institute of Standards and Technology (NIST). He manages the Model-Based Enterprise program, Model-Based Manufacturing Capability Definition project, and NIST Smart Manufacturing Systems Test Bed. His current research focuses on improving agility and flexibility in manufacturing by enabling operational control based on the measured capability of a manufacturing system. He has also made contributions in the areas of green manufacturing, process monitoring, prognostics and diagnostics, and manufacturing data interoperability and management. Helu is a member of the Executive Committee, Standards Committee, and Technical Review Board for MTConnect, Executive Committee of the ASME Manufacturing Engineering Division, and a Corporate Member of the International Academy for Production Engineering (CIRP).
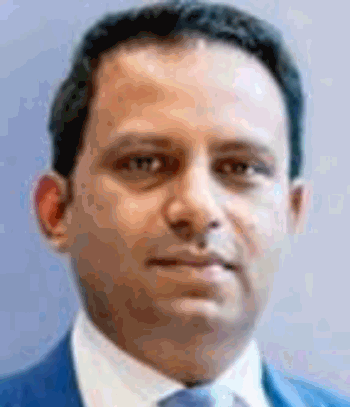
M. M. Rahman
Dr. Mostafizur Rahman is one of the Technical Specialists for Data and Information System theme and his current research focuses on manufacturing informatics, Digital Engineering, and Industry 4.0. Rahman has over 18 years of research and development experience in the area of Computer Science, Artificial Intelligence, Machine Learning and Data Mining. He was awarded his PhD in Medical Data Mining and Machine Learning from University of Hull, UK, and MSc in Artificial Intelligence from Dalarna University Sweden. Rahman has worked in both academic and commercial organisations. He is also technically leading a number of industrial and collaborative (UK and EU) research projects primarily looking at data analytics, machine learning, Industry 4.0 solutions for manufacturing.
S. Jadhav
Dr. Swati Jadhav is an Advanced Research Engineer at the Manufacturing Technology Centre (MTC). Dr. Jadhav is experienced with Computer Science, Data Mining, Machine Learning, Artificial Intelligence, Data Science, Data Analytics, and Predictive Analytics algorithms.
J. J. Whicker
Mr. James Whicker is a Research Engineer in Metrology and NDT at the Manufacturing Technology Centre (MTC). He is an experienced research and development engineer. Mr. Whicker's work includes several technical areas including experimental design and programming.
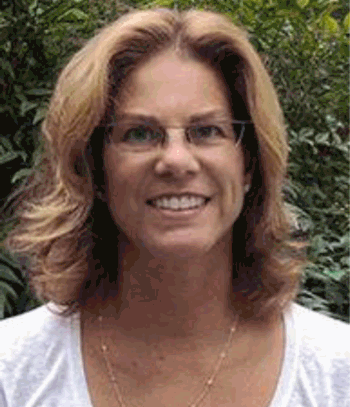
A. Barnard Feeney
Ms. Allison Barnard Feeney leads the Systems Engineering Group in the National Institute of Standards and Technology's Engineering Laboratory. She recently completed a five-year $50M program that addressed national problems related to advanced manufacturing such as cybersecurity and wireless systems for industrial environments, integration of languages and models for lifecycle engineering, and prognostics and health management of smart manufacturing systems. Ms. Barnard Feeney is a key contributor to the development, testing, and adoption of STEP (ISO 10303) product data standards and is the Chair of the US Technical Advisory Group to ISO TC 184/ SC 4, and the US member of SC 4's Policy and Planning Committee. Ms. Barnard Feeney has been honoured with the Department of Commerce Gold Medal (2019) and Silver Medal (2005), the NIST Bronze Medal (2019), and the 2018 ANSI Finegan Standards Medal.