Abstract
Sequencing decisions in mixed-model assembly lines are complicated by various uncertainty factors. This paper addresses a real-life uncertainty factor identified in a manufacturer of large vehicles, by modelling unreliable part delivery and quality. Stochastic optimisation is applied to find sequencing policies that improve the on-time performance of its mixed-model assembly lines. As schedulers have different levels of risk aversion, a risk-averse programme is further presented to protect against the decision maker’s chosen fraction of worst scenarios. Computational studies with Progressive Hedging as the solution method, and its lower bounding approach, demonstrate the high quality of resulting sequencing decisions and the time efficiency of the solution method.
Disclosure statement
No potential conflict of interest was reported by the author(s).
Additional information
Funding
Notes on contributors
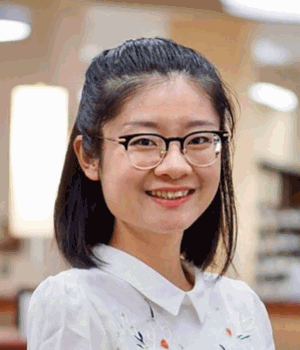
Ge Guo
Ge Guo is Assistant Professor of Decision Science at the University of Baltimore. She received her Ph.D. in Industrial Engineering from Iowa State University in 2018. Her research interests focus on stochastic optimisation applied in power systems and manufacturing systems.
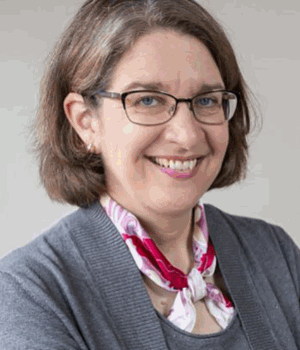
Sarah M. Ryan
Sarah M. Ryan is the Joseph Walkup Professor of Industrial and Manufacturing Systems Engineering at Iowa State University. Her research interests include planning and operation of manufacturing and service systems under uncertainty. Her work has been supported by the National Science Foundation, including a CAREER award, an AT&T Industrial Ecology Faculty Fellowship, the Department of Energy, and electric power systems consortia.