ABSTRACT
This paper proposes a per-machine linear dispatching rule learning approach to improve the scheduling of re-entrant flow shop such as semiconductor fab. Finding an optimal schedule of a complex manufacturing system is intractable; hence, a dispatching rule as a heuristic approach is widely used in actual practice. Also, to develop a good dispatching rule, an automated methodology for developing heuristics, also known as a hyper-heuristic, has been studied extensively. However, most of the literature has focused on finding a single-sophisticated dispatching rule, in which every machine uses the same rule. Such an approach often shows suboptimal performance when the optimal dispatching rule is different on each machine. To solve this problem, we introduce a simple and effective per-machine dispatching rule learning approach, in which each machine has one linear dispatching rule that is optimised by the Gradient-based Evolutionary Strategy (GES). This method is sample-efficient and can be applied to non-differentiable objective functions such as average Cycle Time. The proposed approach was mainly compared to two popular methods based on Genetic Programming (GP) and the Genetic Algorithm (GA) on a four-station and eight-machine re-entrant flow shop. Numerical results show that the proposed approach outperforms widely used methods.
Disclosure statement
No potential conflict of interest was reported by the author(s).
Additional information
Funding
Notes on contributors
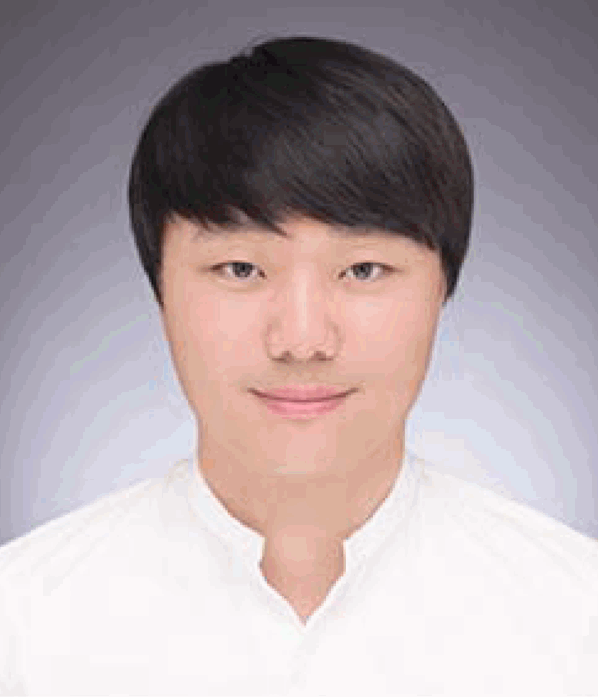
Namyong Kim
Namyong Kim is a Staff Engineer in the Data and Information Technology (DIT) Center of Samsung Electronics DS, Hwaseong, South Korea. He received a BS from Korea University, Seoul, South Korea, in 2012, and a PhD in industrial systems and engineering department from the Korea Advanced Institute of Science and Technology (KAIST), Daejeon, South Korea, in 2020, His research interests include machine learning, simulation, scheduling, and AI-based manufacturing systems.
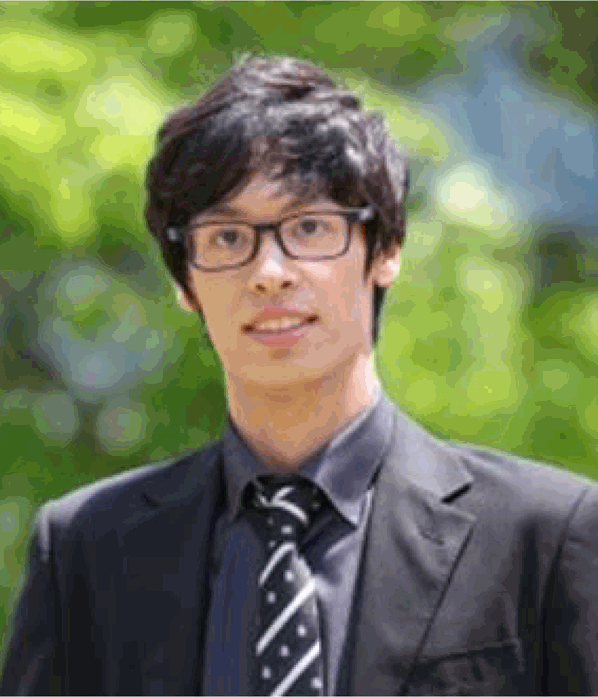
Stephane Barde
Stephane Barde received his B.S., M.S., and Ph.D. in industrial systems and engineering department from the Korea Advanced Institute of Science and Technology (KAIST), Daejeon, South Korea, in 2014, 2017, and 2021 respectively. He is currently a Staff Engineer in the AI task force division of Samsung Electronics DS, Hwaseong, South Korea. His research interests include operations research, machine learning, and AI applied in manufacturing systems.
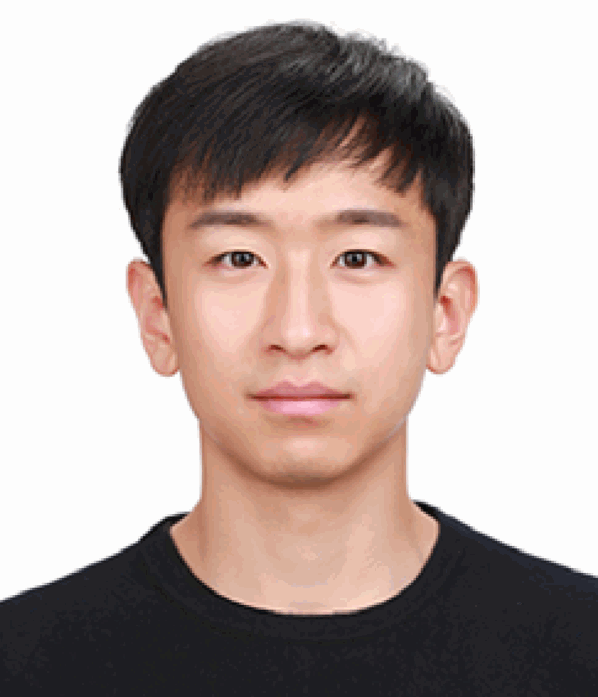
Kiwook Bae
Kiwook Bae received the B.S. and M.S. degree in industrial and systems engineering from the Korea Advanced Institute of Science and Technology (KAIST), Daejeon, South Korea, in 2014 and his master degree in industrial and system engineering from the KAIST in 2015. He is currently employed at the Samsung Electronics DS, Hwaseong, South Korea. His research interests include deep learning and AI-based application for operation system.

Hayong Shin
Hayong Shin is a professor in the Department of Industrial & Systems Engineering at KAIST (Korea Advanced Institute of Science and Technology). Before joining KAIST, He worked for Chrysler Corp., CubicTek Co. and LG Electronics, developing commercial and in-house engineering software solutions. He received a BS from Seoul National University in 1985, an MS and a PhD from KAIST in 1987 and 1991, all in industrial engineering. His recent research interests are in the area of machine learning, simulation, geometric modelling for manufacturing system applications. He can be reached at [email protected].