Abstract
The literature discusses data science (DS) as a very promising set of techniques and tools to support lean production (LP) practices. DS could aid manufacturing companies in transforming massive real-time data into meaningful knowledge, increasing process transparency and product quality information and supporting improvement activities through data-driven decision-making. However, no attempt has been made in the literature to formalise the links between DS and LP practices. Thus, this study aims to overcome this gap by clarifying the DS techniques and tools that can support LP practices and how to apply them. This study employs a quantitative bibliometric method – specifically, a keyword co-occurrence network analysis – on a set of papers extracted from Scopus. The results obtained allowed the researchers to identify a set of DS techniques and tools that can support LP practices and to develop a model to guide their implementation based on the typical improvement implementation stages of the plan-do-check-act cycle. The model shows how to use DS techniques and tools in LP for: identifying areas for improvement and subsequent implementation (plan); enabling a better knowledge and process management (do); identifying/predicting potential problems and employing statistical process control (check); providing remedial actions and effectively applying process improvement (act).
Disclosure statement
No potential conflict of interest was reported by the author(s).
Correction Statement
This article has been corrected with minor changes. These changes do not impact the academic content of the article.
Notes
Additional information
Notes on contributors
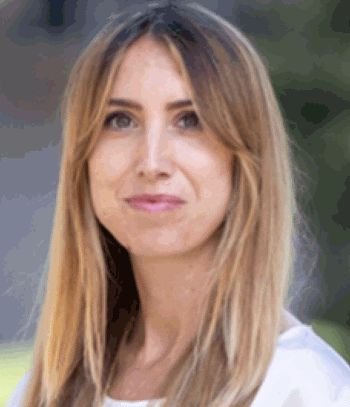
Rossella Pozzi
Rossella Pozzi, Ph.D. is Assistant Professor at the School of Industrial Engineering of Carlo Cattaneo University (LIUC). She teaches Operations management, Lean Manufacturing, Quality management and Data Analytics at LIUC, and Operations Management at the School of Management of Politecnico di Milano. Her main research interests are related to: lean manufacturing, finite capacity materials management policies, industry 4.0 and modelling and simulation of production and logistics systems. She is author of more than 20 papers on classified international journals.
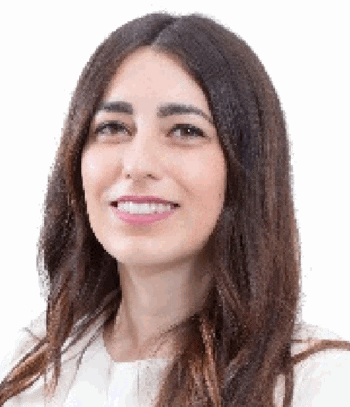
Violetta Giada Cannas
Violetta Giada Cannas, Ph.D. is a post graduate research fellow at the School of Industrial Engineering of Carlo Cattaneo University (LIUC). She teaches Operations management, Quality management and Data Analytics at LIUC, and Operations Management at the School of Management of Politecnico di Milano. She is coordinator of the i-FAB LIUC, a learning factory for the adoption of Industry 4.0 technologies in a lean managed assembly system. Her research interests include supply chain and production management, lean management and Industry 4.0, with a particular focus on companies operating in the engineer-to-order context.
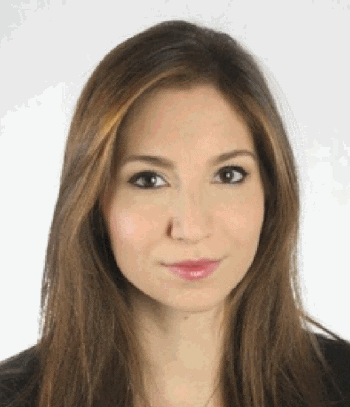
Maria Pia Ciano
Maria Pia Ciano completed a PhD at LIUC – Cattaneo University with a thesis on the link between Lean Production techniques and Industry 4.0 technologies. She addressed the main topic of her PhD thesis in collaboration with the Free University of Bolzano and the Fraunhofer IAO of Stuttgart. She participates in projects on production flows, performance improvement, layout redesign and the adoption of Industry 4.0 and synergy with Lean. These projects have given her the opportunity to get in touch with other notions, such as Innovation and Circular Economy, and a better understanding of specific contexts. She teaches courses on Lean Management, Operations Management and their links with Circular Economy principles.