ABSTRACT
Developing efficient performance evaluation methods is important to design and control complex production systems effectively. We present an approximation method (SLQNA) to predict the performance measures of queueing networks composed of multi-server stations operating under different service disciplines with correlated interarrival and service times with merge, split, and batching blocks separated with infinite capacity buffers. SLQNA yields the mean, coefficient of variation, and first-lag autocorrelation of the inter-departure times and the distribution of the time spent in the block, referred as the cycle time at each block. The method generates the training data by simulating different blocks for different parameters and uses Gaussian Process Regression to predict the inter-departure time and the cycle time distribution characteristics of each block in isolation. The predictions obtained for one block are fed into the next block in the network. The cycle time distributions of the blocks are used to approximate the distribution of the total time spent in the network (total cycle time). This approach eliminates the need to generate new data and train new models for each given network. We present SLQNA as a versatile, accurate, and efficient method to evaluate the cycle time distribution and other performance measures in queueing networks.
Disclosure statement
No potential conflict of interest was reported by the author(s).
Additional information
Funding
Notes on contributors
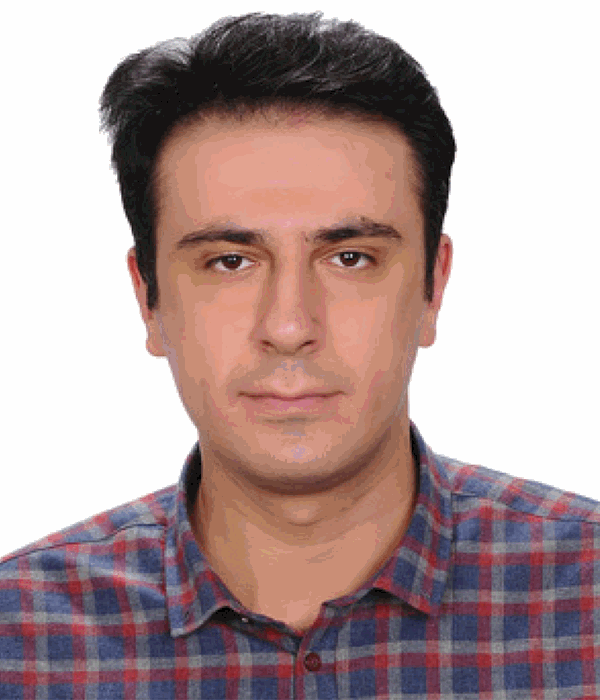
Siamak Khayyati
Siamak Khayyati is a Postdoctoral Fellow at Koç University. He received a BS degree in Industrial Engineering from Sharif University of Technology and PhD degree in Industrial Engineering and Operations Management from Koç University. His research interests are in design and control of production systems and artificial intelligence applications in manufacturing.
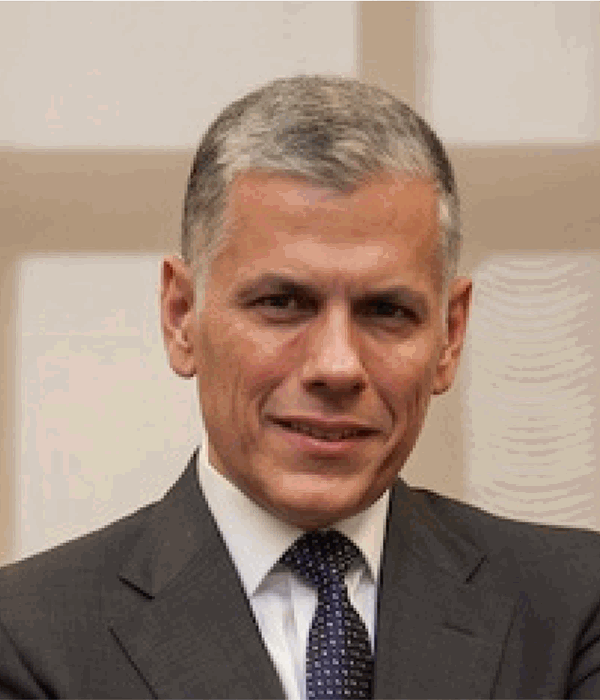
Barış Tan
Barış Tan is a Professor of Operations Management and Industrial Engineering at Koç University, Istanbul, Turkey. His areas of expertise are in design and control of production systems, supply chain management, and stochastic modeling. He received a BS degree in Electrical & Electronics Engineering from Bogazici University, and ME in Industrial and Systems Engineering, MSE in Manufacturing Systems, and PhD in Operations Research from the University of Florida.