Abstract
Supply chain segmentation and inventory classification, specifically, are considered a competitive advantage in many industries. Approaches like the ABC-XYZ analysis are commonly used in practice to classify SKUs based on simple rules for ranking even though simplified rules-of-thumb may lead to sub-optimal decisions and higher costs. We thus propose a cost-based, multi-dimensional inventory classification scheme for assigning SKUs to classes of replenishment policies that prescribe a group service level, a demand distribution, and an inventory control rule. We further provide an extension for classification under an overall service constraint. Our methodological approach is based on machine learning classifiers and we employ a genetic algorithm to train cost-minimising decision trees which allow for easy understanding and reproduction of classification decisions. Cost- and operational focus, simple application, and interpretability are our main contributions to the inventory classification literature. We evaluate the approach on three industry data sets and show that the classification trees result in an average cost increase of only 1.01% (3.70% with an overall service constraint) over the cost-optimal classification, where no tree structure is enforced. Once trees are constructed, unseen data can be classified out-of-sample with an average cost increase of 1.85% (7.68%) over the optimal cost of classification.
Acknowledgments
We would like to thank the two anonymous reviewers for their effort and time taken to review our work. The comments greatly helped to improve the content and presentation of the paper.
Disclosure statement
No potential conflict of interest was reported by the author(s).
Additional information
Notes on contributors
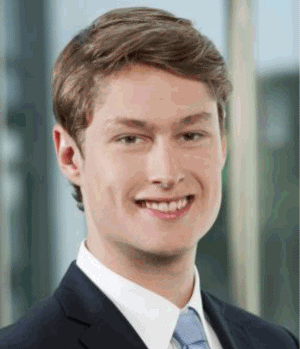
Josef Svoboda
Josef Svoboda is a Ph.D. candidate at the Chair for Logistics and Supply Chain Management at the School of Management, Technical University of Munich (TUM). His research interests are stochastic inventory control and machine learning applications in inventory management.
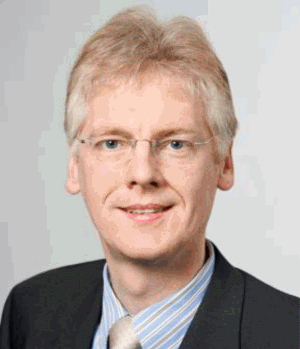
Stefan Minner
Stefan Minner is a Full Professor for Logistics and Supply Chain Management at the School of Management, Technical University of Munich (TUM). Currently, Stefan Minner is the Editor-in-Chief of the International Journal of Production Economics. His research interests are in business analytics, global supply chain design, transportation optimisation and inventory management.