Abstract
Fault detection and diagnosis have always been the key techniques for safe and reliable operation of industrial processes. However, the high dimension and noise of process variables have brought great challenges to the fault detection model. In recent years, due to the powerful feature extraction ability, deep learning has been widely applied in process fault detection and diagnosis. However, these deep neural networks (DNNs) often need a large amount of label data for supervised training, or show poor performance in learning features under unsupervised-learning condition. This paper proposes a new DNN model, a one-dimension residual convolutional auto-encoder (1DRCAE), where unsupervised learning is used to extract representative features from complex industrial processes. 1DRCAE effectively integrates the one-dimensional convolutional kernel with an auto-encoder and is embedded residual learning block for effective feature extraction from one-dimensional data. The two statistics and squared prediction error are generated in the feature space and residual space of 1DRCAE, respectively. Finally, the feasibility and superiority of 1DRCAE are verified on a simulation process, Tennessee Eastman process, Fed-batch fermentation penicillin process, and a real-life case. The convolutional auto-encoder technique provides a new way for feature learning and fault detection on complex industrial processes.
Disclosure statement
No potential conflict of interest was reported by the author(s).
Additional information
Funding
Notes on contributors
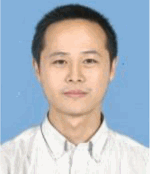
Jianbo Yu
Jianbo Yu, received the BE degree from the Department of Industrial Engineering, Zhejiang University of Technology, Zhejiang, China, in 2002, the ME degree from the Department of Mechanical Automation Engineering, Shanghai University, Shanghai, China, 2005, and the PhD degree from the Department of Industrial Engineering and Management, Shanghai Jiaotong University, Shanghai, China, 2009. In 2008, he was a visiting scholar at the Center for Intelligent Maintenance System, the University of Cincinnati, Cincinnati, Ohio, USA. From 2009 to 2013, he worked as an associate professor at the Department of Mechanical Automation Engineering, Shanghai University, Shanghai, China. Since 2016, he worked as a professor at the School of Mechanical Engineering, Tongji University, Shanghai, China. He is associate editor of IEEE Transactions on Instrumentation & Measurement, and the editorial board member of Recent Patents on Mechanical Engineering. His current research interests include intelligent condition-based maintenance, machine learning, quality control, and statistical analysis.
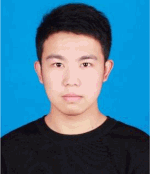
Xing Liu
Xing Liu received his BE degree in 2018 from the Department of Industrial Engineering, Nanjing University of Finance & Economics. Now he is a master’s degree candidate in Tongji University. His main research interests include machine learning, fault detection, and process monitoring.