Abstract
Multi-resource constrained dynamic workshop scheduling is a complex and challenging task in discrete manufacturing. In this paper, to obtain a high-performance scheduling in limited time, this problem is modelled into a Markov decision process, and solved by proximal policy optimisation algorithm, which can learn from the simulated workshop environment directly. A multi-modal hybrid neural network is used in the model to make good use of numerical state features representing workshop environment information and graphical state features representing constraint information during the learning process. Multi-label technique is used in this paper to decouple the output acts of jobs, machines, tools, and workers. Action mask technique coding the constraints is also used to prune invalid exploration. The experimental results show that compared with heuristic rules such as weighted shortest processing time, weighted modified due date, weighted cost over time, apparent tardiness cost and other reinforcement learning methods such as DeepRM and DeepRM2, the performance of the proposed method is at least better in scheduling penalty.
Disclosure statement
No potential conflict of interest was reported by the authors.
Additional information
Funding
Notes on contributors
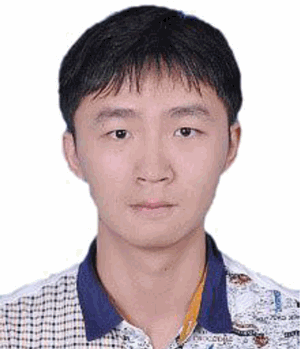
Peng Cheng Luo
Peng Cheng Luo received the B.S. degree in mechanical engineering and automation from the East China University of Science and Technology, Shanghai, China, in 2018, the M.S. degree in mechanical engineering from Tongji University, Shanghai, China, in 2021. His research interests include modelling and analysing of manufacturing systems with machine learning technics and their application to production scheduling.
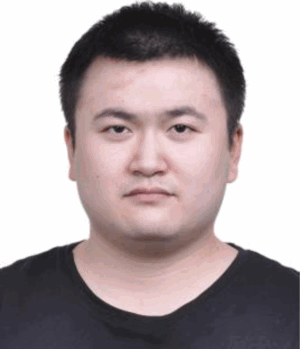
Huan Qian Xiong
Huan Qian Xiong received the B.S. degree in electronic science and technology from Tongji University, Shanghai, China, in 2018, the M.E. degree in integrated circuit engineering from Tongji University, Shanghai, China, in 2021. His research interests include antenna design, application of metasurfaces, and machine learning in multi-agent system.
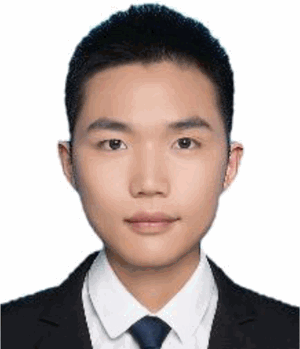
Bo Wen Zhang
Bo Wen Zhang received the B.S. degree in mechanical design, manufacturing and automation from Tongji University, Shanghai, China, in 2018, the M.E. degree in mechanical engineering from Tongji University, Shanghai, China, in 2021. His research interests include preventive maintenance and production scheduling of manufacturing systems.
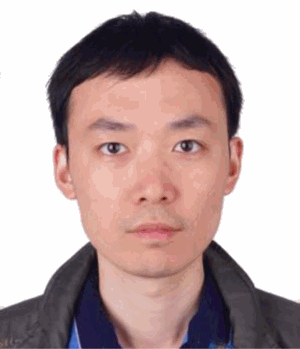
Jie Yang Peng
Jie Yang Peng received the B.S. degree in mechanical engineering from the East China University of Science and Technology, Shanghai, China, in 2013, the M.S. degree in mechanical engineering from Tongji University, Shanghai, China, in 2017. Since 2017, he has been working towards Ph.D. degree in Tongji University. His research interests include modelling and analysing of manufacturing facilities with machine learning technics and their application to preventive maintenance.
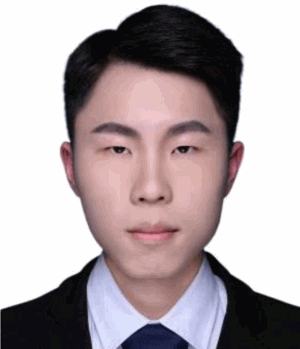
Zhao Feng Xiong
Zhao Feng Xiong received the B.S. degree in Electrical Engineering and its Automation from the Huazhong University of Science and Technology (Wenhua College), Wuhan, Hubei, China, in 2018. His research interests include distributed management and analysis of industrial IoT using cloud-native technologies.