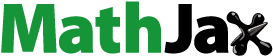
Abstract
Virtual metrology (VM) involves estimating a product’s quality directly from production process data without physically measuring it. This enables the product quality of each unit of production to be monitored in real time, while preserving the process efficiency. Initially developed for the semiconductor industry, VM has recently been examined for use in other industrial fields. VM is enabled by components such as quality estimators and drift detectors. It enhances various industrial applications such as machine control and sampling decision systems. The literature lacks a comprehensive summary and a systematic review of the state of the art in VM. To fill this gap, this study followed a systematic methodology to conduct a complete and structured literature review of VM. This paper presents a detailed analysis of the 199 papers that we identified based on our search and selection criteria. Following our methodological framework, we assigned these papers to categories and highlighted shortcomings. Finally, we discussed VM’s use in various industrial fields, underlining its potential for every manufacturing industry. This study and the proposed framework are useful for both practitioners and academicians since they raise crucial theoretical and managerial questions as well as provide suggestions for further research on this critical topic.
1. Introduction
The contemporary manufacturing domain is shifting to more sustainable manufacturing practices in order to more efficiently cope with complex market desires. Market demands have increased significantly while the product life cycle and the manufacturing cycle have reduced significantly, introducing new challenges for manufacturers (Psarommatis and Kiritsis Citation2018; Kiraci et al. Citation2017). Furthermore, product customisation has forced manufacturers to reduce batch sizes, which has increased defect rates (Ferretti et al. Citation2013; Zhou et al. Citation2017). The key factor in achieving sustainable manufacturing is the assurance of product quality (Psarommatis et al. Citation2020). Therefore, manufacturers must measure and qualify the quality of their products at each stage of production (Chun and Bidanda Citation2013Citation2020 2]; Inman et al. Citation2003). The two main quality control strategies are systematic control – every product is measured – and batch control – when just a few products from each batch are measured and assumed to be representative of the whole batch. Additionally, as stated by Psarommatis et al. the measuring process can be performed in two ways physical measurement or virtual measurement (Psarommatis et al. Citation2020). Physically inspecting parts is time-consuming and expensive; therefore, manufacturing companies currently prefer batch control to systematic control because of the cost as well as the performance that can be achieved. However, batch control has a major disadvantage in that defective products may reach the consumer, which can result in devastating costs to the company (Cheah, Shahbudin, and Taib Citation2011; Tannock and Saelem Citation2007). Systematic control is the optimal practice for ensuring that all products conform to the desired quality characteristics (Psarommatis, Gharaei, and Kiritsis Citation2020; Psarommatis and Kiritsis Citation2019). Virtual inspection has become a reality due to modern technological advancements, and it has enabled manufacturers to apply systematic control without the cost and time disadvantages of physical inspection (Psarommatis et al. Citation2020). The concept of a virtual inspection lies within a broader concept called virtual metrology (VM), which enables the relaxation of batch control homogeneity assumption while preserving production efficiency.
VM lies within the zero-defect manufacturing (ZDM) approach. ZDM proposes several different methods with the shared goal of decreasing and mitigating failures in manufacturing processes – that is, it emphasises doing things right the first time. These methods can be classified as detection, prediction and repair, and prevention methods (Psarommatis et al. Citation2020). VM is a part of the detection class of methods as it enables the detection of defects that have already occurred. Furthermore, automatic machine control, which can repair or even prevent defects, is a production application enabled by VM (Jebri et al. Citation2017b). The present study focused on ZDM because of its advanced capabilities compared with traditional quality improvement methods, such as Six Sigma, Lean Manufacturing, the Theory of Constraints, and Total Quality Management. The superiority of ZDM lies in the fact that after each defect is detected, production methods learn from it for the future, which does not occur in traditional quality improvement methods (Psarommatis et al. Citation2020). ZDM can be implemented through two approaches, namely the product-oriented and process-oriented approaches (Psarommatis et al. Citation2020). Both approaches lead to ZDM but they have different starting points. VM is a product-centred approach, which means that it is focused on product quality, and the process quality will be derived from the product quality. For this systematic review, the following definition of VM was adopted:
VM involves estimating the characteristics that define a product’s quality directly from production process data and mostly using data-driven algorithms. The approach is product-centred, meaning that it exploits data from the means of production to create information about the product (Kuo and Kusiak Citation2019). VM can also incorporate a drift detector to add a safeguard against process evolution, and it may also include the features of updatability and adaptability. Quality estimation can be performed by a sampling decision system (SDS) to optimise the real measurement frequency and by using a control algorithm to close the loop on the production mean.
In addition, VM falls within a larger group of approaches described as soft metrology (Vallejo et al. Citation2019), which brings together concepts such as soft sensors that focus on chemical and continuous manufacturing applications. VM was initially developed for the field of semiconductor production. Today, however, VM is of interest to the entire manufacturing domain as it can significantly improve the sustainability of a manufacturing network (Psarommatis Citation2021; Psarommatis and Kiritsis Citation2019; Cheng et al. Citation2011; Michl et al. Citation2011). VM is a fairly new concept, which is verified by the fact that the first paper to define VM was published in 2005 (Chen et al. Citation2005). Figure illustrates the results of a bibliometric analysis on the number of publications per year, revealing a clear and steady upward trend. This trend is reasonable and expected as more manufacturers and researchers are understanding the need for alternative, more efficient methods of measuring the quality of their products.
The present article provides a complete, structured, systematic review of the field of VM. It proposes a framework with which to structure and popularise all research activities linked with VM, and reviews the technical shortcomings of all of its components, applications, and fields of use. State-of-the-art (SOTA) machine learning (ML) is also leveraged to highlight new opportunities for VM, as are other high-potential new fields of application. The objectives of our analysis were defined by the following research questions:
Q1: How are the different components and applications of VM structured together?
Q2: What is the state of the art of VM, including its components and applications?
Q3: What are the shortcomings of VM in terms of component, applications and industries?
This study and the proposed framework are useful for both practitioners and academicians since they raise central theoretical and managerial questions as well as provide suggestions for further research on this critical yet complex topic. The implications are detailed further as follows:
This structured, systematic review article was written to be useful to all practitioners of VM through a framework for mapping the whole research field. It provides an overall view of VM that could be used as an aid for new implementations. The SOTA of each component and application was studied to guide the development of practitioners’ algorithms and their decisions on approaches.
This study provides researchers with a framework and terminology with which to organise and combine different research fields. It could be used to encourage research into the auxiliary components and applications of VM, such as multi-stage architecture and real-time control. Future research opportunities, supported by SOTA ML, are presented to highlight unexplored yet promising avenues for future research. The paper’s product-centred definition of VM could be used to provide direction to future research in the field.
For newcomers to the field of VM, this paper is an ideal first step toward understanding the field and its definitions. It popularises the vocabulary and, thanks to its description of the SOTA, provides simple guidelines to follow for implementing a basic prototype VM algorithm.
The remainder of this paper is structured as follows. Section 2 describes the research method of the study, and Section 3 presents the proposed VM framework and its components. Section 4 provides an analysis and discussion of the components of the VM framework. Next, Section 5 discusses the advancements and opportunities of VM industrial applications. Finally, Section 6 concludes by highlighting the main findings and outcomes of the study as well as by mapping out directions for further research.
2. Research methodology
This systematic literature review used procedures (Kitchenham Citation2004) exemplified in an earlier review (Rashid, Anwar, and Khan Citation2015). This section defines the methodology to make the process replicable and objective.
2.1. Data sources and search strategy
After the removal of duplicates, the literature on the SOTA of VM comprised 352 papers found through searching the Scopus, Web of Science, IEEE, and Engineer Village databases. A literature search querying phase usually forces keyword assumptions; however, for a complete review of the SOTA of VM, we used the unique query keyword of ‘Virtual Metrology.’ The date range of the systematic review was from 2004 – when the first paper to propose the term VM was published – to November 2020.
2.2. Article selection
Once the 352 papers containing the keyword of ‘Virtual Metrology’ had been collected, four rejection criteria were defined to make the systematic review repeatable:
Accessibility: Papers not accessible in English were not considered.
Crucial effects: Articles ignored by the VM community would have negatively affected the variance of our statistical constructs. To avoid such effects, papers published more than 3 years previously and never cited were removed.
Repetition: To ensure statistical quality and decrease potential bias, conference papers that had been republished as journal papers were removed.
Subject relevance: For consistency, all the papers compared had to respect VM product-centred paradigms and be oriented toward an industrial topic. For instance, a paper dealing with the estimation of a machine’s production efficiency and not with the quality of the final product would have been excluded from this review.
The effects of each of these selection–rejection criteria are summarised in Figure . In the bibliometric analysis, most of the papers were found to deal with semiconductor applications. However, the search was not limited to only this field because of the potential of VM in other manufacturing domains.
2.3. Quality assessment of the retained articles
A total of 199 papers were extracted according to the aforementioned criteria, and then they were classified depending on their topic and application. Every component and application of VM was studied, either exhaustively or in a quantitative manner. For the component and application counting too few papers to extract trends, an exhaustive review was performed; otherwise, a more quantitative approach to studying VM was employed. In this case, in addition to studying the statistics, some significant papers were also examined qualitatively. The criteria used to select them were as follows:
ML relevance: The proposed VM approach corresponded to commonly used, SOTA machine learning approaches.
Research impact: The paper was in the top 25% of most cited papers in its domain of VM based on its Scopus citation record.
Recentness: The paper was published in 2018 or earlier.
Originality: The paper proposed an original, high-potential solution for resolving a known problem.
To be highlighted as significant research, a paper needed to be ML-relevant and fulfill at least one of the other criteria – either 2, 3, or 4. The fourth criterion enabled the consideration of under-rated solutions from another perspective. Every manufacturing application of VM was reviewed quantitatively, and they are presented later in the discussion section.
2.4. Data extraction and synthesis
To answer our research questions, 11 categories of VM were dichotomised into two groups. The technical group contained the components and applications of VM, as described in the framework presented in Table . The group of VM manufacturing applications classified industrial operations into the two categories in Table . Each paper was nonexclusively classified into one or more categories. To document the systematically reviewed papers, all of the papers are cited either in the text (for highlighted papers) or in the citation table in the Annex.
Table 1. Summary of technical group categories.
Table 2. Summary of manufacturing application categories.
3. Virtual metrology framework
Based on the analysis of the literature, the components and applications of VM were structured as a framework that depicted their interconnections. This framework is presented in Figure .
The proposed framework was aimed at comprehensively connecting all of the components and applications of VM. The elements of the framework are summarised as follows: The VM block takes the raw process data as inputs. Those inputs then undergo preprocessing before being processed by the quality estimator and the drift detector. This preprocessing step, presented in Section 4.1, includes the nonexhaustive removal of outliers, reduction of dimensionality, and normalisation of raw data. The quality estimator, presented in Section 4.2, extracts information from the preprocessed data to estimate the product quality. The drift detector, presented in Section 4.3, detects abnormal behaviour in the preprocessing data and raises internal alarms when a drift is uncovered. All of these techniques can be enhanced to enable the VM to be updatable or more data-efficient. The updatability feature is discussed in Section 4.5, and the adaptability feature is discussed in Section 4.6. The data-efficiency enhancement provided by the adaptability feature enables faster prototyping and implementation of a new VM model. The implementation of VM in an industrial environment induces the need to define two connections: The first is the horizontal interconnection of the different VMs implemented along the production life cycle of the product, which is discussed in Section 4.7; the second is the vertical connection of the VM to the manufacturing execution system, known as a Fab-wide architecture, which is discussed in Section 4.9. Finally, the VM output is composed of a quality estimation and a drift detection alarm, which are used by two main applications: First, the SDS, presented in Section 4.4, optimises the product measurement rate; then, the production machine can be controlled based on the quality estimated, which is discussed in Section 4.8. Noteworthily, other Industry 4.0 applications can benefit from VM quality estimation (Dreyfus and Kyritsis Citation2018). For instance, predictive maintenance could benefit from VM since product quality is strongly linked to machines’ health (Roeder et al. Citation2012; Hsieh et al. Citation2013; Hsieh, Cheng, and Yang Citation2012). Vice-versa, VM can benefit significantly from predictive maintenance through the forecasting of upcoming concept drift (Moyne et al. Citation2014). Indeed, the causes of machine failure are also causes of quality drift. However, because nearly no research exists on the link between those applications and VM, they were omitted from the proposed framework.
As depicted in Figure , each element of the framework can be classified by its role. This explains the place it holds in the implementation of VM.
The most basic elements are those that extract information from the data. Most of the time, these are supervised or unsupervised ML algorithms, which obtain value from the process or from each other. They are referred to as techniques.
Features are technique modifications for achieving secondary objectives, such as reducing the minimum size of the training set or making the techniques updatable. They often come as technique add-ons, which still require technique modification to be implemented.
Architectures define the way VM is connected to the environment. They deal with the data flux horizontally – that is, all the operations required to manufacture the product – and vertically – that is, the enterprise software system.
Applications are external elements that use techniques to enhance or provide new benefits. As they are external from VM, they are not components. Every application can be described in the same way as VM, with techniques, features, and architectures.
It is worthwhile classifying the framework’s elements by their necessity. Necessity is defined as the element’s importance for the implementation of VM as presented in Figure .
The core concept group includes the elements that are strictly necessary for building VM and estimating product quality. The group is composed exclusively of techniques that are sufficient for implementing a basic VM solution. These techniques should be designed and implemented first.
The essential group includes the elements required for building a lasting VM solution. As with any Industry 4.0 solution, the VM environment evolves with time, and therefore, every technique needs to be updated. The essential group includes all of the elements that enable what could be called technique maintenance. These elements are not necessary for short-term VM implementation but are necessary for long-term VM implementation. For industrial deployment, essential elements must be taken into consideration.
The auxiliary group contains the optional elements that create value but are not necessary for basic or long-term VM implementations. Such elements are still worth considering for enhancing the system in terms of industrial implementation or application.
4. Analysis and discussion of virtual metrology elements
4.1. Data preprocessing
Data preprocessing is a technique that transforms data to make it compatible with other techniques and also enhances the accuracy of quality estimation. Data preprocessing is a required technique for implementing VM, and thus, it is part of the core concept group. Preprocessing includes multiple operations that can all be performed offline and sometimes online, such as the preparation of the initial data, assessment of missing data (sometimes online), outlier removal (online), data normalisation (online), and feature engineering (sometimes online).
Even though all of the retained papers described the use of preprocessing – as a fundamental step of ML – few explained which preprocessing phases were used, and even fewer explicitly mentioned which algorithms they employed. This explains why fewer papers were cited in our preprocessing statistics than in our quality estimation statistics and also why only outlier removal and feature selection were discussed. Moreover, this lack of transparency may also give rise to problems related to experiment repeatability.
4.1.1. Outlier removal
Outliers are aberrant data points lying outside of the expected distribution, which can lower the precision of the quality estimator if considered. Detecting and removing them from the dataset is a thoroughly discussed preprocessing operation in the VM field. The most used outlier removal approach is promptly described in Table .
Table 3. Outlier removal algorithms.
Manual outlier detection is the simplest and most used approach. Features can be plotted independently or not (Chen et al. Citation2020) for visualising potential outliers. This method is purely offline, which is an important limitation for VM implementation. Statistics-based methods are also highly popular as they are the simplest online approach. A specific clustering method named ART2 was developed for VM outlier detection (Huang et al. Citation2014). ART2 is widely used for VM as it is included in the automatic VM framework (Cheng et al. Citation2016). Lastly, a ML field called novelty detection proposes advanced solutions for detecting outliers. Some of those solutions have been tested successfully for VM (Chou, Wu, and Chen Citation2010; Kang, Kim, and Cho Citation2014; Kim et al. Citation2015). The main advantage of novelty detection is that it can deal with data streams. Indeed, whereas the other solutions assume that the distribution they learned is fixed, a data stream accepts that it will evolve with time, adding a new challenge – whether it is ‘an aberrant point, an outlier, or just the evolution of the normality.’ Detecting aberrant points is not performed simply to increase the accuracy but also – and especially – to protect the quality estimator from the time-variation of its environment. This is a major challenge that should be prioritised more.
The field of novelty detection abounds with more advanced solutions that have yet to be studied for VM applications (Faria et al. Citation2016). Outlier detection is highly connected to drift detection, which is discussed later in Section 4.3.
4.1.2. Dimensionality reduction
Dimensionality reduction consists of the modification of the feature’s space, from a high dimensional space toward a low dimensional space, while maintaining the maximum amount of meaningful information. Working with a high dimensional feature space is detrimental to VM application due to the twin curses of dimensionality and computational intractability. Moreover, keeping features uncorrelated with the output can reduce the model’s overall effectiveness (Kuhn and Johnson Citation2013; Kim and Kang Citation2019). The VM feature’s space size usually has a very high dimension due to the many process sensors sampling at a high frequency for several minutes at a time. This explains why dimensionality reduction is a fundamental preprocessing step in VM.
Dimensionality reduction can be achieved by removing less meaningful features, using feature selection, or transforming all of the features toward a lower dimensional space using feature extraction. Finally, dimensionality reduction can also be performed by reducing the size of each feature, as with data quantisation.
Feature selection combines the dimensionality reduction approaches that preserve features by simply decreasing the feature space dimension. In other words, redundant or irrelevant features are removed. One advantage of feature selection is its transparency. Indeed, information about a feature’s usefulness can, for example, explain the modification of the process itself. Feature-selection methods are usually divided into three main categories: filter, wrapper, and embedded methods. The most used feature selection algorithm is presented in Table . Alternatively, feature selection could also simply be conducted by a process expert.
Table 4. Feature selection algorithms based on a similar table in Vallejo et al. (Citation2019).
Although computationally demanding, wrapper methods are highly popular due to their capability for high dimensionality reduction. In the reviewed papers, 59% of wrapper methods were used with linear approaches such as multiple linear regression (MLR) or partial least squares (PLS) as inference algorithms, whereas 41% were combined with nonlinear approaches such as neural networks (NNs), Gaussian process regression (GPR), or support vector regression (SVR). Taking into account the high number of iterations required to converge, weak learners such as linear learners were often used. The use of nonlinear learners highlights the nonlinearity of most VM problems. Indeed, applying linear algorithms to nonlinear problems will lead to underperformance. Similarly, filter methods are not used anymore. By contrast, the popularity of embedded methods has grown significantly, carried by multiple recent publications that have used ensemble methods as quality estimators.
Feature-extraction approaches transform an initial feature space into a smaller one. This transformation permits the removal of any hidden redundancy between features, which can be more efficient than feature selection in terms of reducing dimensionality. Moreover, the newly extracted feature space can increase the precision of the quality estimator and reduce the overall noise. The greatest limitation of the feature-extraction approach is the difficulty in extracting process knowledge from the new feature space once it has been transformed. Moreover, unlike the feature-selection approach, which transforms the feature space, the feature-extraction approach must be implemented both offline and online, thus increasing the calculation time. Feature selection and extraction are equally used in SOTA VM. The most used feature extraction algorithm is presented in Table .
Table 5. Feature-extraction algorithms.
PCA and PLS are widely used methods for VM that are restrained by their linear assumption. To ease this limitation, a kernel can be associated with them to explore nonlinear relationships, a method usually called kernel PCA or kernel PLS (Kang et al. Citation2009; Chou, Wu, and Chen Citation2010; Kang et al. Citation2011). Notably, all existing nonlinear feature-extraction approaches, including CNNs and autoencoders, have been described for the first time since 2017, reflecting the need to move toward nonlinear algorithms. Moreover, thanks to the fast evolution of CNN applications in the field of ML, the use of CNNs has also been increasing in VM applications, representing more than 70% of dimensionality reduction algorithms described in 2020.
Data quantisation, very commonly used for VM, reduces the dataset size by binning it by features and representing the bins using statistics. In addition to the most commonly generated statistics (such as mean, median, and maximum), excellent results have been reported for the segregation of steady-state and transient periods for building new statistics, such as steady-state level and duration, settling time, and rise time (Ul Haq and Djurdjanovic Citation2019; Chen et al. Citation2019). Binning can also be performed at a fixed frequency or by focusing on specific zones of interest that correspond to production recipe steps (Hirai and Kano Citation2015). Reducing the size of the dataset often comes at the price of a great loss of information, which may reduce the inference accuracy. However, some approaches require a qualitative feature and may have greater accuracy.
4.2. Quality estimation
Quality estimation is one of the core concepts of VM techniques. It is aimed at estimating product quality using measurable process variables. Defining a product’s quality is application-dependent, but it usually has a single output, such as etching depth for plasma-etching applications. Moreover, quality estimations are often defined as continuous values, which encourages the use of regression algorithms for inference tasks. However, some papers have evaluated classification approaches that simply describe product quality as within tolerance or out of tolerance (Tilouche, Bassetto, and Nia Citation2014; Chou, Wu, and Chen Citation2010). Using classification for quality estimation is often called fault detection and classification. For instance, novel detection algorithms have been applied for discrete VM estimations of quality (Kim et al. Citation2012). Most of the approaches used in VM are data-driven ML approaches. Discrete VM is restrictive and should only be used if data is too scarce to train a regression algorithm. Even if prior knowledge can, in some cases, be considered, algorithms learn thanks to data-driven representations of the system (i.e. a dataset). The approaches presented here are supervised as they always use the input–output couple to learn. Numerous algorithms are used to perform quality estimation, and they are presented in Table .
Table 6. Quality estimation algorithms based on a similar table in Vallejo et al. (Citation2019).
Linear regressors are currently the most frequently used algorithms for quality estimation; however, although they provide fast inferences, they do not achieve high accuracy and can be unstable with nonlinear problems. Their popularity may be driven by their data efficiency in an industry where data is scarce considering continually changing production-machine health. Their popularity may also be driven by their noise rejection ability.
The second most used algorithm family is NNs. Even if MLP was for long the most used approach, today it is under-represented, replaced by more advanced neural network architectures. Recurrent NNs (RNNs) use time dependencies to increase their accuracy when dealing with time series. However, RNNs suffer from heavy data-efficiency issues, which explains their lack of popularity. Moreover, recent research demonstrated that CNNs can also capture time dependencies in a highly data-efficient manner and with greater accuracy (Bai, Zico Kolter, and Koltun Citation2018), enabling greater accuracy and robustness against drifts, which could explain their recent popularity in the VM field. Attention mechanism algorithms (Vaswani et al. Citation2017) have become a highly popular approach for replacing RNNs in SOTA ML. Future research on attention mechanisms for VM applications could be interesting. Papers featuring Bayesian NNs (BNNs) were particularly well represented in 2020 because BNNs have brought many new, valuable capabilities to the table, such as prior knowledge transfer from computer-aided manufacturing tools (Chen et al. Citation2019) and causality links. Causal inference is another popular topic in the field of VM as it ensures tremendous interpretability, enabling rapid correction after the detection of sources of process failure (Kim et al. Citation2019). Hybrid architectures had excellent results in SOTA VM, such as a combination of an RNN and a CNN for enhancing robustness against concept drift (Bum, Chang, and Kim 2020). Noteworthily, thanks to the evolution of calculation power, NN architectures and hyper parameters tend to now be optimised by genetic algorithms for VM applications (Jia et al. Citation2018; Yang et al. Citation2019). Such NN variations hold tremendous opportunities for enhancing the accuracy, precision, and interpretability of quality estimators; thus, they should be studied further.
The third most frequently used approach involves kernel methods. The use of SVR is declining while GPR is becoming ever more popular. GPR is a high-potential approach in the field of VM as it provides in-built uncertainty quantification, which is a critical advantage because it greatly simplifies the implementation of perennial VM solutions. GPR’s greatest limitation is its lack of scalability, which can be limited if combined with the right dimensionality reduction algorithm such as lasso (Chan et al. Citation2018) or a CNN (Wu et al. Citation2020).
Despite a significant number of studies in 2020 adopting ensemble methods, they remain the least used approaches for quality estimation. The bagging algorithm has crucial advantages as it can not only estimate uncertainty like GPR but can also be updated simply through the addition or removal of an individual from the ensemble. Stacking is known to perform better than single learners for some problems (Džeroski and Ženko Citation2004), which tend to be validated for VM (Chen et al. Citation2020; Hsieh et al. Citation2020; Li, Wu, and Yu Citation2019). Most ensemble methods permit the easy implementation of essential group components, and although they are recent, they should be considered by practitioners for industrial applications.
4.3. Drift detection
The quality estimator learns a concept defined by the representation of the studied physical equations, namely the learning dataset. This concept is generally time-dependent. Its evolution is called concept drift (Widmer and Kubat Citation1996), and if not considered, it will constantly decrease the accuracy of quality estimations. When dealing with an issue as critical as product quality estimation, it is necessary to ensure the correctness of conjectures. This problem is known as the applicability or manufacturability problem (Jonathan, Cheng, and Cheng Citation2007). The technique of drift detection (DD) is part of the essential group, and it is a necessary element for making VM perennial in time. DD assumes the role of the alarm by detecting concept drifts based on the inputs and feeding the information into the SDS. DD outputs a Boolean that indicates whether a drift is actually occurring. Implementing DD involves two steps, the first of which is detecting an abnormality, and the second is characterising the concept drift. These two steps, including the algorithms used, are described in detail in the following two subsections.
4.3.1. Detecting an abnormality
The first group of algorithms used to detect drift in SOTA VM is called uncertainty quantification. Very often assuming Gaussianity, these algorithms identify a standard deviation of the quality estimation, capturing its ‘confidence.’ Based on a problem-dependent heuristic threshold, the abnormality can be detected. One advantage of calculating the confidence is that besides DD, it can be leveraged to enhance both the quality estimators (Azamfar, Li, and Lee Citation2020) and machine control algorithms (W. T. Yang et al. Citation2020; Kim, Jang, and Kim Citation2020). The automatic virtual metrology (AVM) solutions propose an add-on heuristic approach called the reliance index, which is based on the crossover of two different quality estimators (Cheng et al. Citation2008). This used to be the most popular method for detecting abnormality, but many other interesting alternatives are available today. For instance, regression approaches with in-built uncertainty quantification such as GPR and bagging are convincing for VM applications (Cai et al. Citation2020). Moreover, in SOTA ML, multiple methods are yet to be studied, such as BNNs with uncertainty quantification, concrete dropout (Lakshminarayanan, Pritzel, and Blundell Citation2017; Caldeira and Nord Citation2020), and deep ensembles (Kabir et al. Citation2018).
As discussed in the outlier detection section, the second group of algorithms mostly originate from SOTA novelty detection. They are one-class classification or clustering algorithms. Generally, with the threshold indirectly included in their hyper parameters, they directly output a Boolean value for detecting an abnormality. They are known to be highly efficient at detecting drift (Faria et al. Citation2016). Despite the central role of DD in the essential group, it has not been thoroughly studied for VM, and numerous algorithms remain to be explored.
4.3.2. Characterising a concept drift
Detected abnormalities must be classified as either outliers or concept drift. These are different entities because outliers are not representative of the physical distributions (Wadewale and Desai Citation2015). Once a drift has been characterised, the product must be measured to update the quality estimator. However, measurements are expensive and measuring outliers is useless. Furthermore, including outliers in the training set is detrimental. To discriminate concept drift from outliers, the simplest solution used in VM is to measure output only if multiple successive abnormal samples have been detected (Cheng et al. Citation2016). Once the measurement has been performed, another VM approach suggests a second verification of whether the output is abnormal; if the output is normal and the input is abnormal, then the sample is considered an outlier (Kim et al. Citation2015). Numerous methods exist for characterising a concept drift that remain to be explored, particularly those originating from SOTA novelty detection.
4.4. Sampling decision system
The SDS is the main application of VM because it enables the production measurement rate to be adapted based on the virtual quality estimation. Its decisions are built around the quality estimator output, the DD output, and the time elapsed since the last measurement. SDS strategies simply based on time are called passive or time-based strategies, whereas SDS strategies only based on VM output are called active or event-based strategies. SDS strategies based on both time and VM output are called hybrid strategies. Figure , which is inspired by a figure by Kang and Kang (Citation2017) illustrates the different types of sampling strategies; They are also described in Table .
Table 7. Sampling decision system strategies.
Event-based strategies compensate for the two aforementioned limits of time-based strategies, as the measurement decision is based on the specific quality of each product. The main event-based SDS strategy is called uncertainty sampling (Lewis and Gale Citation1994). It consists of triggering a measurement when the estimation is no longer trusted (Kurz, De Luca, and Pilz Citation2015; Susto Citation2017), out of tolerance (Wan, Honari, and McLoone Citation2013), or impossible, as with just-in-time (JIT) learning extrapolation problems (Jebri et al. Citation2017a). The first approach, namely uncertainty sampling, has been integrated into all SDSs across the SOTA papers on VM. Event-based strategies should never be used alone because there are always sources of deviation that are hidden and then not visible for the DD, making the whole system fallible.
Today, time-based strategies are the main strategies used in production. The frequency at which measurements should be conducted are either optimised through a traditional statistical process control approach or simply defined heuristically. Most of the time, these strategies under-perform in terms of product quality because they assume a homogeneous batch, and also in terms of process efficiency because they assume the system to be time-independent.
Hybrid SDS strategies perform the best because they have the advantages of both time- and event-based strategies. A fixed-time-based frequency accounts for hidden drifts, while an event-based frequency takes care of the other types of drift (Cheng et al. Citation2015). Some approaches propose adapting the time-based frequency in function of the event occurrence (Cheng et al. Citation2016). By doing so, one can leverage some room for optimisation from the fixed-frequency hybrid strategy; however, a theoretical background is nowadays missing to ensure that the model update frequency follows the hidden context evolution. In fact, this background is also missing when setting up the fixed-frequency hybrid strategy, which indicates great research opportunities.
4.5. Updatability feature
Once a labelled example is available, the updatability feature enables all techniques to be updated, including preprocessing (Hyun Baek et al. Citation2014). The updatability feature enters the essential group as it enables VM to deal with concept drifts. To do so, it alters the way the learning set is stocked thanks to an external algorithm, thus avoiding the limitations of a huge learning set. The updatability features are described in Table . The stability–plasticity dilemma (SPD) is fundamental for differentiating the various methods of updating (Jaber Citation2013). A highly stable method will have high inertia and adapt slowly, whereas, a method with high plasticity will adapt quicker to new concepts but may become unstable.
Table 8. Updatability algorithms.
Moving window (MW) is the most commonly used approach for dealing with data streams for VM thanks to its simplicity and its ‘forgetting capability.’ Various MW ameliorations exist, such as adaptive MW or JIT learning MW (Urhan and Alakent Citation2020), although only a few studies have examined them for VM (Ringwood et al. Citation2010; Wu, Cheng, and Kong Citation2012).
JIT learning is popular for VM. It enables very large learning sets to be dealt with by retraining the inference algorithm at each inference on a small subset selected using different clustering algorithms (Lee, Kang, and Cho Citation2014; Cheng and Chiu Citation2004). However, it is highly limited by its lack of ability to ‘forget,’ making it impossible to use for applications that are not purely cyclical. Moreover, even if sometimes applied with slow learners (Chan et al. Citation2018), JIT learning needs to be applied online to be combined with fast learners, which explains why it is nearly exclusively used with linear algorithms. One-third of linear approaches use JIT learning, whereas all other approaches use MW.
One means of supplementing an updating algorithm – if one has prior knowledge about the physical system – is to model concept drifts separately to decompose the problem (Iskandar and Moyne Citation2016) with, for instance, NNs (Chang et al. Citation2006) or wavelet transform (Chang Citation2010). For example, maintenance – a common cause of concept drift – has been modelled multiple times (Lin, Hsu, and Yu Citation2014; Lynn, Ringwood, and MacGearailt Citation2012; Park et al. Citation2020). This method is rarely sufficient as all of the causes of drift are generally unknown; it should be implemented as a complement to MW or JIT approaches.
Besides these methods found in SOTA VM papers, ensemble methods are often used for their high stability and plasticity. Indeed, ensemble methods can be updated easily by adding or removing new members; they would probably outperform the currently used methods for VM (Ditzler et al. Citation2015).
4.6. Adaptability feature
The adaptability feature aims to diminish the set-up time of a quality estimator, for new or different products, by reducing the number and size of the features required to train that quality estimator. In doing so, it increases VM’s agility and responsiveness, which is increasingly useful in a world where personalisation and product diversity have become the norm. Multiple approaches have been studied to reach those goals, and they are listed in Table .
Table 9. Adaptability approaches.
Constant system identification has been applied for VM (Pan et al. Citation2011; Tieng et al. Citation2017). However, it remains very limited as very few products differ from a simple constant.
Another method called transfer learning enables the learning time and the learning set size to be reduced by presetting optimised parameters with a learning algorithm that has already been trained on a similar task. Enhanced accuracy and computational efficiency have been found for VM applications (Kang Citation2018).
When multiple quality estimators must be jointly developed, multi-task learning can be implemented to reduce both the learning time and the learning set size. In SOTA VM, multi-task learning is applied to linear algorithms, ensemble methods, and kernel methods, and its data efficiency has been proven (Park et al. Citation2018). However, for different tasks to be jointly learned, they must be selected wisely as this approach could become counter-effective if the difference between them is too great.
Another approach is called semisupervised learning, which automatically generates labelled data from unlabelled data to diminish the learning set size. For VM, all of the tested approaches label new points based on a consensus ensemble of homogeneous learners trained on different subsets of the dataset, as with the co-training algorithm (Nguyen et al. Citation2017; Citation2020), or an ensemble of heterogeneous learners trained on the same dataset, as with the SAFER algorithm (Kim et al. Citation2019). This approach has been noticed to be highly counter-effective if drift occurs, as this will lead to a biased consensus and the inclusion of false information in the training set.
Adaptability features are a crucial topic as labelled data is scarce and expensive in the industry, which is underdeveloped today. Thus, great research opportunities exist in this area.
4.7. Multi-stage architecture
VM’s main goal is to estimate a product’s quality based on production process data. Current quality usually depends on the quality of past operations. In other words, the quality at operation n-1 affects operation n, and this is true along the production line. The multi-stage architecture in operation defines VM’s interconnectedness; it deals with the integration and connection of multiple VM points along the product’s production line to enhance the overall accuracy of quality estimation. Multiple multi-stage architectures exist; the present review proposes a three-group classification described in Table , and illustrated in Figure .
Table 10. Multi-stage architectures.
A serial architecture improves VM accuracy without increasing the feature space of each VM point much (Su et al. Citation2008). It has been commonly used for VM and its applications, such as machine control, where it takes both current estimations and estimations from previous operations as inputs (Khan, Moyne, and Tilbury Citation2007).
A hub architecture can increase VM’s accuracy and is advantageous when the quality of past operations is not measurable or of low accuracy (Chen et al. Citation2020). Moreover, developing and maintaining VM involve financial costs, which in some cases must be minimised. This architecture can be used either as a quality control point or as a final quality control verification. The hub architecture’s main limitation comes from the curse of dimensionality as the feature space grows with each past operation considered, which is limiting in the real-time implementations.
A cascade architecture has pros and cons that are highly dependent on the final architecture. For example, one cascade architecture proposes independent VMs at each point of operation along the production line, but only feeds their estimations to the VM point for the last operation. This last VM point uses all preceding estimations plus the data from the last operation to estimate the product’s final quality (Susto et al. Citation2015; Schirru et al. Citation2011). As a guideline, the information flux should follow the operations depending on their correlation. Because most manufacturing operation dependence is not serial, most implementations should use cascade architectures. However, research in this field is scant. Therefore, more research must be conducted to compare the different multi-stage architectures and to determine how to develop them.
4.8. Machine control
Automatic machine control is an application of VM that leverages quality estimation and DD to control the means of production. By doing so, the production process can be stabilised and robustified. Most control approaches involve run-to-run control – they update process parameters at the end of each production run. This does not control the quality of each part but attempts to centre the quality distribution around tolerance. VM can therefore deal with process drift (Moyne, Del Castillo, and Hurwitz Citation2000) at the batch level, but it cannot ensure the quality of every piece produced. Multiple control algorithms are used with VM, and they are presented in Table .
Table 11. Control algorithms.
Table 12. Fab-wide architectures.
The vast majority of control algorithms for VM use EWMA or dEWMA, which are exclusively implemented in run-to-run. Multiple variations have been studied for SOTA VM. The output quality measures can, for instance, be included in an EWMA filter when available (Lin, Tseng, and Wang Citation2013). Those newly included physical measures have a high weighting compared with virtual measures and they are used as temporary baselines. Some research using EWMA/dEWMA filters has taken information regarding uncertainty quantification into account. This can be used to fine-tune the weighting parameter in real time. High uncertainty will increase the weighting of actual measurements and vice-versa. It has also been shown to increase controller performance.
Another active research topic is the controller’s robustness against concept drift. One approach that was proposed is to feedforward the measured quality to modify the controller gains. This allows a slow incremental drift to be detected and compensated for (Khakifirooz, Fathi, and Chien Citation2018). Another approach integrates knowledge about equipment condition into a dEWMA, which is estimated by a dynamic Bayesian network (Yang et al. Citation2020). The main limitation of the EWMA/dEWMA filter is its assumption of linearity and its run-to-run implementation.
Model predictive control (MPC) is one of the few approaches that combines VM with online, real-time control (Lynn, Macgearailt, and Ringwood Citation2012). Unfortunately, MPC is heavily dependent on the system simulation, which is mostly unavailable in VM applications. Another real-time approach merges the quality estimator and the controller by learning the inverse model, thus estimating the process parameters to achieve the desired quality of the output. Thus, quality is not estimated directly but the control task is performed. In this approach, a generative adversarial network is employed to train the CNN used for inference and to deal with concept drift caused by regular updates (Kim, Jang, and Kim Citation2020).
Real-time consideration may seem useless for the main VM application, namely SDS, which explains the popularity of run-to-run control. However, from a control perspective, real-time quality estimation is the holy grail as it would enable the real-time control of production apparatuses in terms of quality, which would be a huge step toward the ZDM paradigm (Ringwood et al. Citation2010). Although a dense body of knowledge on control theory exists, much research still needs to be done with a particular focus on real-time nonlinear approaches that incorporate information regarding uncertainty quantification.
4.9. Fab-wide architecture
The need for Fab-wide architectures is a fundamental aspect of any industrial big-data approach. A Fab-wide architecture is defined to connect the different organs of an industrial VM implementation. Its location is either on the shop floor, on a computer on the production machine, either on the intranet, on the manufacturing execution system (MES), or on the cloud. The different architectures are presented in Table .
The generic VM architecture is the simplest that exists and should be the first step for new industrial VM implementations. The VM unit is as integrated as possible with the production apparatus (Huang et al. Citation2007). It enables real-time capabilities while being highly flexible; it also diminishes the risk of data-packet asynchronicity problems or even losses.
MES VM architectures introduce three new components, which are shared for all of the different VM units. The model generation server consists of a multi-tenant server that can receive and run multiple VM creation models on different virtual machines simultaneously, thus ensuring user isolation (Hung et al. Citation2017). The central database stocks all data and models. Using main memory database technology for VM applications has produced better results than disk-resident technology (Hung et al. Citation2012). The VM management server deals with the control and maintenance of VM models (Cheng, Huang, and Kao Citation2012; Hung et al. Citation2012). This approach enables better overall control of VM capability across a whole fab.
A hot trend in Industry 4.0. is products as services, where product providers rent services, maintain control of systems, and ensure their maintenance. The manufacturing industry or client rents VM solutions, outsourcing their implementation, operation, and maintenance problems. To implement products as services, VM solutions must be moved to the cloud. The partial cloud VM architecture pushes the model creation server, VM manager, and central database to the cloud (Cheng, Huang, and Kao Citation2012a, Citation2012b; Hsieh et al. Citation2019; Hung et al. Citation2013). In this case, the VM operation is kept close to the production apparatus, enabling real-time implementation. This final approach seems to be the optimal compromise because it successfully separates the online and offline parts.
The full cloud VM architecture places every possible component in the cloud, including the VM algorithm. The only part that remains on the machine is the data collection device (Huang et al. Citation2015). Adding VM in a server dramatically increases the server’s resistance to software failure thanks to the easy replacement of virtual machines; computational power can also easily be higher. On the other hand, real-time VM is compromised due to the lag between the cloud and shop floor.
In terms of implementation, research has proposed multiple solutions for industrial VM. Apache Hadoop is a big-data-friendly, open-source ecosystem that can improve on the query processing speeds and data storage efficiency of handmade solutions (Moyne, Samantaray, and Armacost Citation2016). Another solution is Microsoft’s Azure Machine Learning Studio, which provides a drag-and-drop way to train, deploy, and manage VM solutions (Tang Hsuan et al. Citation2020). Noteworthily, some Fab-wide architectures foresee not only the inclusion of VM but also other technologies such as predictive maintenance or ontology structuring.
5. Virtual metrology industrial application and discussion
VM is a generic ZDM tool that can theoretically be applied to every manufacturing application. Originally designed for semiconductor manufacturing, VM has recently been applied in a multitude of industrial fields to various processes with different dynamics. It is impossible to find one algorithm for every component in the VM framework that will fit all of the different dynamics. Accordingly, this section discuss the advancement and opportunities in those industrial applications. It is organised in 2 subsections, first discussing the semiconductor application and finally the other manufacturing domains.
5.1. Semiconductor manufacturing
SOTA VM for semiconductor applications can be grouped into the following five operations: photolithography, chemical vapour deposition (CVD), plasma-enhanced chemical vapour deposition (PECVD), plasma-etching, and chemical-mechanical planarisation (CMP). All of the citations for semiconductor manufacturing VM papers, classified by applications, are provided in Table 8 of the Annex.
The most studied semiconductor operation incorporating VM is plasma-etching, which usually involves removing a photoresist layer. As discussed in a 2010 review, this is a high-value-added operation usually run in open-loop, thus providing a strong motivation to control the quality of that loop using retroaction (Ringwood et al. Citation2010). Following the review’s recommendation, multiple control approaches have been tested based on VM, such as real-time MPC, which was highly successful and acted as a proof of concept (Lynn, Macgearailt, and Ringwood Citation2012). Unfortunately, no other real-time implementation of VM has emerged from the SOTA. One might think that with the emergence of ZDM, real-time consideration will soon become a hot topic. Regarding plasma-etching, it is noteworthy that the use of plasma information (PI) as an input has enabled online control and opened new opportunities for VM; PI is still very commonly used (Park et al. Citation2020). Speaking of features, there is still a great deal of emphasis on dimensionality reduction with a preference for feature extractions. Plasma-etching is no different from other semiconductor applications in its strong need to reduce the number of processed inputs. As concluded by a 2009 review (Zeng and Spanos Citation2009), due to nonlinearity, the best and most commonly used algorithm for the quality estimator is a NN. MLP was and still is the most popular choice. However, there is a notable increase in interest in CNN applications, which achieve increased accuracy and robustness compared with MLP (Tsutsui and Matsuzawa Citation2019). Indeed, plasma-etching is not spared from concept drift such as residue deposition (Ringwood et al. Citation2010), justifying the potential of CNNs. On the other hand, the implementation of the essential group component is the optimal approach for dealing with concept drift, yet it remains under-researched (Susto Citation2017).
The second most studied operation is CVD, along with its variant PECVD. Notably, most papers have not explicitly described the exact industrial operations involved, and thus, they remain vague. PECVD is an exception and is usually differentiated from CVD, although no clear differences exist between the algorithms used for both techniques. CVD is used to produce thin-films, either for adding a new layer or for doping an existing one. As with plasma-etching, great effort is expended to diminish the feature space size, with an emphasis on feature extraction algorithms. In terms of the quality estimator, a 2014 review on VM for CVD explained that the use of SVR is optimal for this application (Purwins et al. Citation2014). Since then, kernel methods have become the most used methods, among which GPR is the most popular. As GPR is not a scalable algorithm, its rise can be explained by the development of feature extraction algorithms. In terms of VM technical applications, very few papers have focused on machine control (Lin, Hsu, and Yu Citation2014) for CVD. Because it enhances VM’s impact on the shop floor, it can only be beneficial for implementing CVD control, which motivates further research. Conversely, the implementation of essential group components is an active topic for CVD research. The most used approaches are MW for updatability, diverse DD methods, and SDS, demonstrating the clear presence of concept drift in this industrial application.
CMP is the third most studied application. It is used to planarise and clean the top layer, to finish the wafer, or to prepare it for its next operation. CMP can be viewed as a bridge between semiconductor manufacturing and other manufacturing domains. Indeed, planarisation can also indicate ‘polishing,’ which is an operation found in many production industries. This application is quite recent as 50% of CMP VM papers have been published since 2018. Another point that makes CMP unique is that dimensionality reduction does not seem to be an problem. Very little research has been conducted on this subject for CMP, and most papers have not explained the use of one. Quality estimators are quasi-exclusively implemented with NNs but surprisingly not MLP. More advanced architectures are used such as RNNs, CNNs, or BNNs. BNN applications are mostly applied to CMP. CNNs and ensemble algorithm seem to be the approaches with the most potential for quality estimation across the industrial applications. Dealing with drift is another active topic, but remarkably the most used updatability feature is JIT learning, not MW. In terms of control, both classic controllers such as dEWMA and highly innovative controllers such as the one based on genetic adversarial network (GAN; Kim, Jang, and Kim Citation2020) have been discussed. CMP is like the laboratory of VM for semiconductor manufacturing because many new technologies and algorithms have been tested with CMP, yet they still need to be tested for all of the other industrial applications such as CNNs, BNNs, GANs, and more. It is the VM field to watch as it is the most active and innovative.
Finally, the least studied operation incorporating VM is photolithography, which shapes the final substrate. Very few papers have been published on its different components, making the resulting discussion less objective. Moreover, very little has been said in terms of pre-processing, concept drift handling, and applications. However, MLP is the most commonly used approach for quality estimation, indicating potentially high nonlinearity.
Lastly, it was found that since 2015, the scientific focus on CMP and more marginally represented semiconductor applications has grown significantly, such as copper-clad laminate (Kim et al. Citation2017), physical vapour deposition (Chen et al. Citation2020), laser chip manufacturing (Hou et al. Citation2019), roll-to-roll manufacturing (Jin, Shui, and Shpitalni Citation2019; Shui, Jin, and Ni Citation2019), and wafer sawing (Hsieh et al. Citation2020) among others. This clearly emphasises the future opportunities for VM in new, less studied semiconductor applications.
5.2. Other manufacturing domains
Beyond the semiconductor domain, VM is also adopted in other domains, such as the following:
Machining operations, including milling, turning, drilling, and spark machining
Metal additive manufacturing
Carbon fibre manufacturing
All of the citations for papers outside of the semiconductor-manufacturing domain are available Annex 9.
In the field of machining operations, the major challenge for VM is the drastic decrease in dataset signal-to-noise ratios compared with semiconductor manufacturing. The production environment is noisy with severe vibrations and temperature changes, which makes advanced preprocessing critical. Wavelet denoising, as described previously, is an interesting approach under these conditions (Yang, Tieng, and Cheng Citation2016). In terms of preprocessing, as for semiconductor operations, machining operations must deal with numerous features, which necessitates efficient feature selection (Chen et al. Citation2019a, Citation2019b).
In terms of quality estimation, 100% of the papers describing VM in machining settings have used MLP as their inference algorithm. Indeed, MLP can deal with noise and leverage its presence to avoid overfitting (Rolnick et al. Citation2017). Furthermore, the use of MLP can be explained by the high nonlinearity of machining operations. To reduce complexity, prior knowledge can be injected as post-processing. For instance, a deformation model of a hole can be used to enhance the accuracy of VM in a drilling operation (Tieng et al. Citation2018). Finally, one application, namely run-to-run control, has already been tested on machining operations successfully (Huang and Lv Citation2020).
Research on the use of VM in other manufacturing domains besides semiconductors only began in 2017. Since then, 12 papers have been published on this topic, which is more than 10% of the published papers on VM in that time, demonstrating increasing interest. There are tremendous research opportunities on the use of VM in all manufacturing fields. The few results that have been presented can be viewed as proof of concept on the applicability of VM to the discussed manufacturing operations. The next step will be to implement the tested framework elements on different machines in order to validate the prior results and test untested algorithms, approaches and architectures, such as those in the essential and auxiliary groups.
6. Concluding remarks
This systematic review performed a comprehensive analysis of the literature on VM and then proposed a VM framework. VM is structured by the proposed framework, which is composed of the following components: preprocessing, quality estimation, DD, an SDS, the updatability feature, the adaptability feature, a multi-stage architecture, machine control, and a fab-wide architecture. Each element was defined in terms of its roles and importance. The detailed analysis performed in this study revealed the following key findings:
The use of nonlinear algorithms for dimensionality reduction and quality estimation is now the standard.
Numerous high-potential approaches have been used for quality estimation. They bring new capabilities such as causality study for BNNs, automatic feature extraction for CNNs, uncertainty estimation, and simple updating for ensemble methods.
The drift rejection capability of VM is enabled by three essential steps: DD, the SDS, and updating.
An event-based SDS should not be used because of its sensitivity to hidden contexts. A hybrid SDS should always be used with VM.
Three families exist for multi-stage architecture: serial, hub, and cascade architectures.
Machine control based on VM is dominated by run-to-run approaches. Very few research studies have focused on real-time solutions.
Driven by the product-as-services trend, the latest research on fabrication-wide VM architectures has focused on cloud implementation.
CMP is the laboratory of VM, featuring all the newest approaches such as CNNs and BNNs. It is also the bridge between semiconductor manufacturing and other manufacturing domains.
An increasing number of new VM industrial applications have been studied both for semiconductors and other manufacturing domains.
Beyond all of these considerations, VM-related research should also be conducted on the different patterns illustrated by our study and by the proposed framework. First, real-time machine control is an Industry 4.0 application of very high value, and is mainly enabled by VM. There is still much work to be done on this topic since only a few papers have addressed it. Another insight is that VM cannot be sustainable in an industrial environment without a proper drift rejection capability. DD, an SDS, and the updatability feature are promising research topics for VM. Furthermore, once in the industrial environment, VM must be applied to numerous machines and operations. VM adaptability decreases implementation needs in terms of time and data, and is an under-researched high-value feature that will gain importance with time. Thanks to numerous proofs of concept of VM applications in new industrial operations such as machining and additive manufacturing, numerous research opportunities exist for replicating and enhancing their results. Moreover, quality estimation algorithm benchmarking on public datasets has not been conducted in the VM field for numerous applications. Finally, the link between VM and predictive maintenance is another under-researched topic. Applicative papers that demonstrate the benefit of this interaction are of great interest.
Disclosure statement
No potential conflict of interest was reported by the author(s).
Additional information
Notes on contributors
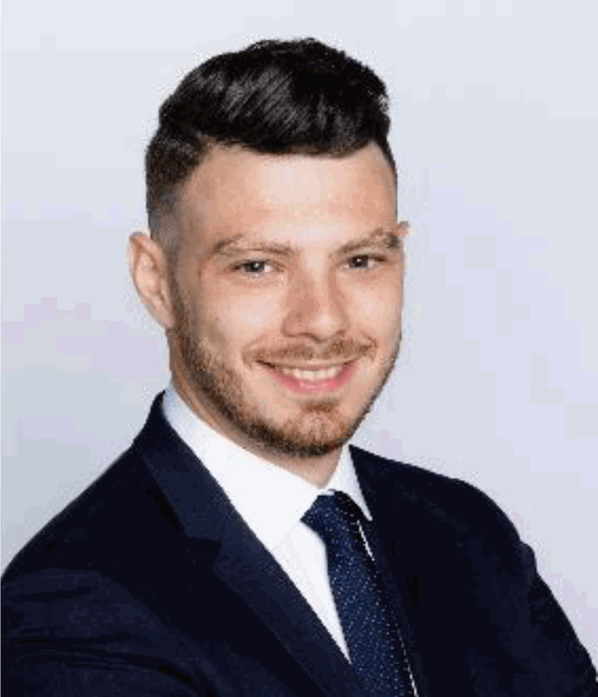
Paul-Arthur Dreyfus
Paul-Arthur Dreyfus received the M.S degree in mechanical engineering with a specialisation in control and machine learning from the Swiss Federal Institute of Technology in Lausanne (EPFL), Switzerland, in 2017. He is currently pursuing a Ph.D. degree at the ICT for Sustainable Manufacturing group, at the same university. Already awarded with Best Paper awards in international conferences, Paul-Arthur’s research is made in tight collaboration with a well-known Swiss watch manufacturer allowing him to pay major attention to real industrial needs and constraints. His domain of expertise is mainly about Industry 4.0 technologies and tools including product quality estimation, machines connectivity, and long-term sustainability of Industry 4.0 solutions. Paul-Arthur also has a strong background in methods and tools used for Industry 4.0. applications such as machine learning, simulation, and control.
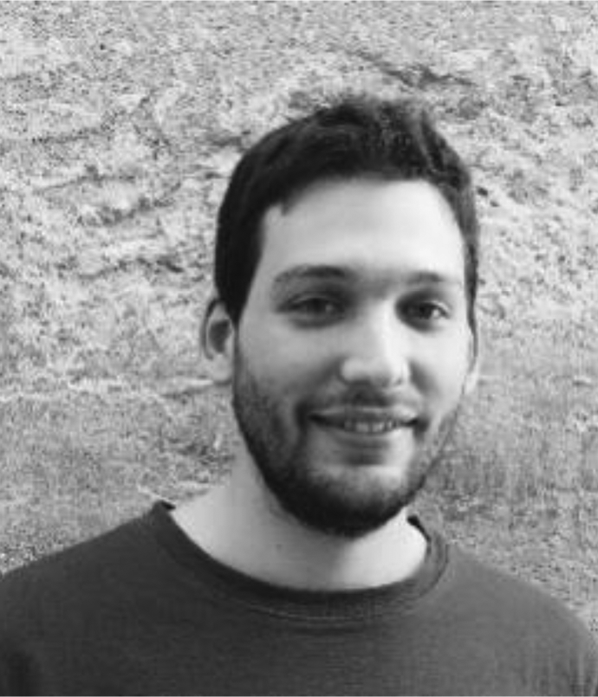
Foivos Psarommatis
Foivos Psarommatis is a passionate and active researcher in the area of quality improvement in manufacturing systems at École polytechnique fédérale de Lausanne - EPFL. More specifically is a pioneer in the area of Zero Defect Manufacturing (ZDM), as is the first who modernised and set the foundation of modern ZDM. His scientific interests, motivation and vision are around on how ZDM can be applied efficiently to production systems, focusing on the decision making, scheduling and design of a system or a product, with ultimate goal to achieve true sustainable manufacturing.
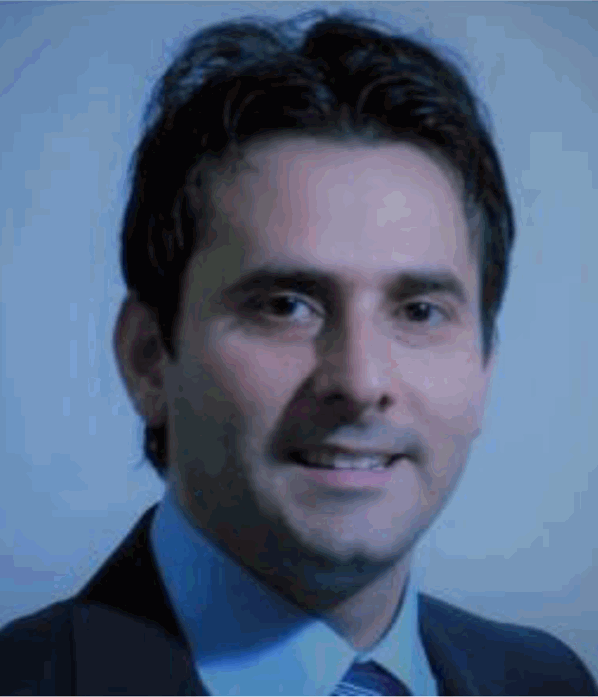
Gokan May
Gökan May is an Assistant Professor at the University of North Florida, and Editorial Board Member of the World Manufacturing Forum. His major fields of research in the main area of data-driven smart and sustainable manufacturing include energy efficient manufacturing, zero-defect manufacturing, and predictive maintenance. Dr. Gökan May has published more than 35 papers in international journals and conference proceedings and has been involved in several industry-academia collaboration projects. Before joining UNF, he was a Postdoctoral Researcher at École Polytechnique Fédérale de Lausanne (2015–2020), and a Fellow of the World Economic Forum’s Global Future Council on Production (2018–2020). He received his Ph.D. in 2014 (Summa Cum Laude) and M.Sc. degree in 2010 (with Highest Honours) in Management, Economics and Industrial Engineering from Politecnico di Milano. During 2013, he worked as a Researcher at the Pennsylvania State University in the Department of Industrial and Manufacturing Engineering.
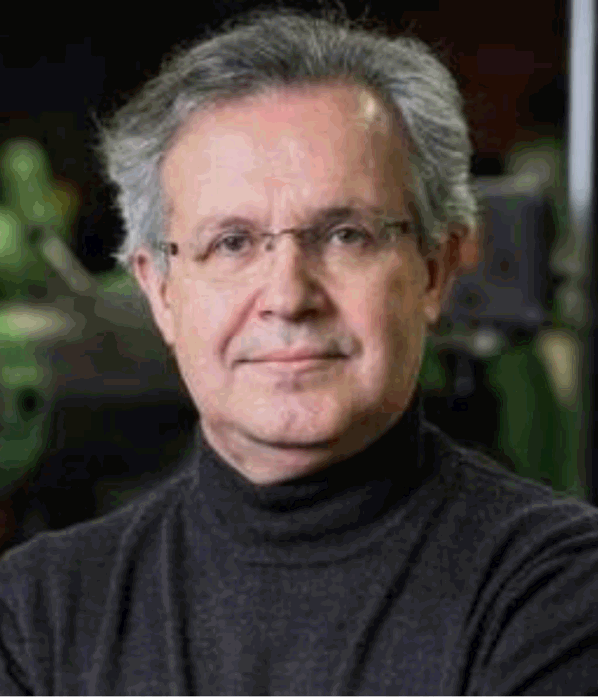
Dimitris Kiritsis
Dimitris Kiritsis (Kyritsis) is Faculty Member at the Institute of Mechanical Engineering of the School of Engineering of EPFL, Switzerland, where he is leading a research group on ICT for Sustainable Manufacturing. His research interests are Closed Loop Lifecycle Management, Sustainable Manufacturing IIoT, Semantic Technologies, Industrial Ontologies and Data Analytics for Engineering Applications. Dimitris serves as Director of the doctoral Programme of EPFL on Robotics, Control and Intelligent Systems (EDRS). He served as Guest Professor at the IMS Centre of the University of Cincinnati, and Invited Professor at the University of Technology of Compiègne, the University of Technology of Belfort-Montbéliard and at ParisTech ENSAM Paris. Dimitris is actively involved in EU research programmes in the area of Factories of the Future and Enabling ICT for Sustainable Manufacturing. He has more than 220 publications. From September 2013 to September 2019 Dimitris was the Chair of IFIP WG5.7 – Advanced Production Management Systems. From 2013 to 2017 he was member of the Advisory Group of the European Council on Leadership on Enabling Industrial Technologies – AG LEIT-NMBP. He is also founding fellow member of the International Society for Engineering Asset Management (ISEAM and among the initiators and co-founders of the IOF (Industrial Ontologies Foundry). Since 2019 Dimitris is member of the WEF Global Future Council of Advanced Manufacturing and Production
References
- Azamfar, Moslem, Xiang Li, and Jay Lee. 2020. “Deep Learning-Based Domain Adaptation Method for Fault Diagnosis in Semiconductor Manufacturing.” IEEE Transactions on Semiconductor Manufacturing 33 (3): 445–453. doi:https://doi.org/10.1109/TSM.2020.2995548.
- Bai, Shaojie, J. Zico Kolter, and Vladlen Koltun. 2018. “An Empirical Evaluation of Generic Convolutional and Recurrent Networks for Sequence Modeling.” Preprint. https://arxiv.org/pdf/1803.01271.pdf.
- Cai, Haoshu, Jianshe Feng, Qibo Yang, Wenzhe Li, Xiang Li, and Jay Lee. 2020. “A Virtual Metrology Method with Prediction Uncertainty Based on Gaussian Process for Chemical Mechanical Planarization.” Computers in Industry 119: 103228. doi:https://doi.org/10.1016/j.compind.2020.103228.
- Caldeira, João, and Brian Nord. 2020. “Deeply Uncertain: Comparing Methods of Uncertainty Quantification in Deep Learning Algorithms.” Machine Learning: Science and Technology 2 (1): 015002. doi:https://doi.org/10.1088/2632-2153/aba6f3.
- Chan, Lester Lik Teck, Xiaofei Wu, Junghui Chen, Lei Xie, and Chun-I Chen. 2018. “Just-In-Time Modeling With Variable Shrinkage Based on Gaussian Processes for Semiconductor Manufacturing.” IEEE Transactions on Semiconductor Manufacturing 31 (3): 335–342. doi:https://doi.org/10.1109/TSM.2018.2826012.
- Chang, Yaw Jen. 2010. “Wavelet-Based Virtual Metrology Technique.” In Proceedings of the 2010 IEEE International Conference on Mechatronics and Automation, Xi’an, August 4–7, 2010, 367–371. Xi’an: IEEE. doi:https://doi.org/10.1109/ICMA.2010.5588327.
- Chang, Yaw-Jen, Yuan Kang, Chih-Liang Hsu, Chi-Tim Chang, and Tat Yan Chan. 2006. “Virtual Metrology Technique for Semiconductor Manufacturing.” In The 2006 IEEE International Joint Conference on Neural Network Proceedings, Vancouver, July 16–21, 2006, 5289–5293. Vancouver, BC: IEEE. doi:https://doi.org/10.1109/IJCNN.2006.247284.
- Cheah, Soo-Jin, Amirul Shah Shahbudin, and Fauziah Taib. 2011. “Tracking Hidden Quality Costs in a Manufacturing Company: An Action Research.” International Journal of Quality and Reliability Management 28 (4): 405–425. doi:https://doi.org/10.1108/02656711111121816.
- Chen, Chao Chun, Min Hsiung Hung, Benny Suryajaya, Yu Chuan Lin, Haw Ching Yang, Hsien Cheng Huang, and Fan Tien Cheng. 2019a. “A Novel Efficient Big Data Processing Scheme for Feature Extraction in Electrical Discharge Machining.” IEEE Robotics and Automation Letters 4 (2): 910–917. doi:https://doi.org/10.1109/LRA.2019.2891498.
- Chen, Chun Han, Parag Parashar, Chandni Akbar, Sze Ming Fu, Ming Ying Syu, and Albert Lin. 2019b. “Physics-Prior Bayesian Neural Networks in Semiconductor Processing.” IEEE Access 7: 130168–79. doi:https://doi.org/10.1109/ACCESS.2019.2940130.
- Chen, Ping Hsu, Sunny Wu, Junshien Lin, Francis Ko, Henry Lo, Jean Wang, C. H. Yu, and M. S. Liang. 2005. “Virtual Metrology: A Solution for Wafer to Wafer Advanced Process Control.” In ISSM 2005, IEEE International Symposium on Semiconductor Manufacturing, San Jose, September 13–15, 2005, 155–157. San Jose, CA: IEEE. doi:https://doi.org/10.1109/ISSM.2005.1513322.
- Chen, Ching-Hsien, Wei-Dong Zhao, Timothy Pang, and Yi-Zheng Lin. 2020. “Virtual Metrology of Semiconductor PVD Process Based on Combination of Tree-Based Ensemble Model.” ISA Transactions 103: 192–202. doi:https://doi.org/10.1016/j.isatra.2020.03.031.
- Cheng, Fan Tien, Jonathan Yung-Cheng Chang, Hsien-Cheng Huang, Chi-An Kao, Ying-Lin Chen, and Ju-Lei Peng. 2011. “Benefit Model of Virtual Metrology and Integrating AVM into MES.” IEEE Transaction on Semiconductor Manufacturing 24 (2): 261–272. doi:https://doi.org/10.1109/TSM.2011.2104372.
- Cheng, Fan Tien, Chun Fang Chen, Yao Sheng Hsieh, Hsuan Heng Huang, and Chu Chieh Wu. 2015. “Intelligent Sampling Decision Scheme Based on the AVM System.” International Journal of Production Research 53 (7): 2073–2088. doi:https://doi.org/10.1080/00207543.2014.955924.
- Cheng, Fan Tien, Yeh Tung Chen, Yu Chuan Su, and Deng Lin Zeng. 2008. “Evaluating Reliance Level of a Virtual Metrology System.” IEEE Transactions on Semiconductor Manufacturing 21 (1): 92–102. doi:https://doi.org/10.1109/TSM.2007.914373.
- Cheng, Cheng, and Min Sen Chiu. 2004. “A New Data-Based Methodology for Nonlinear Process Modeling.” Chemical Engineering Science 59 (13): 2801–2810. doi:https://doi.org/10.1016/j.ces.2004.04.020.
- Cheng, Fan Tien, Yao Sheng Hsieh, Chun Fang Chen, and Jhao Rong Lyu. 2016a. “Automated Sampling Decision Scheme for the AVM System.” International Journal of Production Research 54 (21): 6351–6366. doi:https://doi.org/10.1080/00207543.2015.1072649.
- Cheng, Fan Tien, Hsien Cheng Huang, and Chi An Kao. 2012a. “Developing an Automatic Virtual Metrology System.” IEEE Transactions on Automation Science and Engineering 9 (1): 181–188. doi:https://doi.org/10.1109/TASE.2011.2169405.
- Cheng, Jui Yu, Min Hsiung Hung, Shih Sung Lin, and Fan Tien Cheng. 2012b. “New Remote Monitoring and Control System Architectures Based on Cloud Computing.” Advanced Materials Research 579: 312–329. doi:https://doi.org/10.4028/www.scientific.net/AMR.579.312.
- Cheng, Fan Tien, Hao Tieng, Haw Ching Yang, Min-Hsiung Hung, Yu-Chuan Lin, Chun-Fan Wei, and Zih-Yan Shieh. 2016b. “Industry 4.1 for Wheel Machining Automation.” IEEE Robotics and Automation Letters 1 (1): 332–339. doi:https://doi.org/10.1109/LRA.2016.2517208.
- Chou, Pao-Hua, Menq-Jiun Wu, and Kuang-Ku Chen. 2010. “Integrating Support Vector Machine and Genetic Algorithm to Implement Dynamic Wafer Quality Prediction System.” Expert Systems with Applications 37 (6): 4413–4424. doi:https://doi.org/10.1016/j.eswa.2009.11.087.
- Chun, Youngjae, and Bopaya Bidanda. 2013. “Sustainable manufacturing and the role of the International Journal of Production Research.” International Journal of Production Research 51 (23-24): 7448–7455. https://doi.org/https://doi.org/10.1080/00207543.2012.762135.
- Ditzler, Gregory, Manuel Roveri, Cesare Alippi, and Robi Polikar. 2015. “Learning in Nonstationary Environments: A Survey.” IEEE Computational Intelligence Magazine 10 (4): 12–25. doi:https://doi.org/10.1109/MCI.2015.2471196.
- Dreyfus, Paul Arthur, and Dimitrios Kyritsis. 2018. “A Framework Based on Predictive Maintenance, Zero-Defect Manufacturing and Scheduling Under Uncertainty Tools, to Optimize Production Capacities of High-End Quality Products.” In IFIP Advances in Information and Communication Technology, edited by Ilkyeong Moon, Gyu M. Lee, Jinwoo Park, Dimitris Kiritsis, and Gregor von Cieminski, 296–303. Cham: Springer.
- Džeroski, Saso, and Bernard Ženko. 2004. “Is Combining Classifiers with Stacking Better Than Selecting the Best One?” Machine Learning 54 (3): 255–273. doi:https://doi.org/10.1023/B:MACH.0000015881.36452.6e.
- Faria, Elaine R., Isabel J. C. R. Gonçalves, André C. P. L. F. de Carvalho, and João Gama. 2016. “Novelty Detection in Data Streams.” Artificial Intelligence Review 45 (2): 235–269. doi:https://doi.org/10.1007/s10462-015-9444-8.
- Ferretti, S., D. Caputo, M. Penza, and D. M. D’Addona. 2013. “Monitoring Systems for Zero Defect Manufacturing.” Procedia CIRP 12: 258–263. doi:https://doi.org/10.1016/j.procir.2013.09.045.
- Hirai, Toshiya, and Manabu Kano. 2015. “Adaptive Virtual Metrology Design for Semiconductor Dry Etching Process Through Locally Weighted Partial Least Squares.” IEEE Transactions on Semiconductor Manufacturing 28 (2): 137–144. doi:https://doi.org/10.1109/TSM.2015.2409299.
- Hou, Dennis, Tuo Liu, Yen Ting Pan, and Janpu Hou. 2019. “AI on Edge Device for Laser Chip Defect Detection.” In 2019 IEEE 9th Annual Computing and Communication Workshop and Conference, Las Vegas, January 7–9, 2019, 247–251. Las Vegas, NV: IEEE. doi:https://doi.org/10.1109/CCWC.2019.8666503.
- Hsieh, Yao Sheng, Fan Tien Cheng, Hsien Cheng Huang, Chung Ren Wang, Saint Chi Wang, and Haw Ching Yang. 2013. “VM-Based Baseline Predictive Maintenance Scheme.” IEEE Transactions on Semiconductor Manufacturing 26 (1): 132–144. doi:https://doi.org/10.1109/TSM.2012.2218837.
- Hsieh, Yao Sheng, Fan Tien Cheng, and Haw Ching Yang. 2012. “Virtual-Metrology-Based FDC Scheme.” In IEEE International Conference on Automation Science and Engineering, Seoul, August 20–24, 2012, 80–85. Seoul: IEEE. doi:https://doi.org/10.1109/CoASE.2012.6386371.
- Hsieh, Yu-Ming, Chin Yi Lin, Yu Ru Yang, Min Hsiung Hung, and Fan Tien Cheng. 2019. “Automatic Virtual Metrology for Carbon Fiber Manufacturing.” IEEE Robotics and Automation Letters 4 (3): 2730–2737. doi:https://doi.org/10.1109/LRA.2019.2917384.
- Hsieh, Yu-Ming, Rung Lu, Jing-Wen Lu, Fan-Tien Cheng, and Muhammad Adnan. 2020. “Automated Classification Scheme Plus AVM for Wafer Sawing Processes.” IEEE Robotics and Automation Letters 5 (3): 4525–4532. doi:https://doi.org/10.1109/lra.2020.3000678.
- Huang, Yi-Ting, Fan-Tien Cheng, Yu-Hsuan Shih, and Yen-Lun Chen. 2014. “Advanced ART2 Scheme for Enhancing Metrology-Data-Quality Evaluation.” Journal of the Chinese Institute of Engineers 37 (8): 1065–1080. doi:https://doi.org/10.1080/02533839.2014.912773.
- Huang, Hsien Cheng, Yu Chuan Lin, Min Hsiung Hung, Chia Chun Tu, and Fan Tien Cheng. 2015. “Development of Cloud-Based Automatic Virtual Metrology System for Semiconductor Industry.” Robotics and Computer-Integrated Manufacturing 34: 30–43. doi:https://doi.org/10.1016/j.rcim.2015.01.005.
- Huang, Delin, and Jun Lv. 2020. “Run-to-Run Control of Batch Production Process in Manufacturing Systems Based on Online Measurement.” Computers and Industrial Engineering 141: 106298. doi:https://doi.org/10.1016/j.cie.2020.106298.
- Huang, Hsien Cheng, Yu Chuan Su, Fan Tien Cheng, and Jia Mau Jian. 2007. “Development of a Generic Virtual Metrology Framework.” In 2007 IEEE International Conference on Automation Science and Engineering, Scottsdale, September 22–25, 2007, 282–287. Scottsdale, AZ: IEEE. doi:https://doi.org/10.1109/COASE.2007.4341746.
- Hung, Min Hsiung, Chun Fang Chen, Hsien Cheng Huang, Haw Ching Yang, and Fan Tien Cheng. 2012a. “Development of an AVM System Implementation Framework.” IEEE Transactions on Semiconductor Manufacturing 25 (4): 598–613. doi:https://doi.org/10.1109/TSM.2012.2206061.
- Hung, Min Hsiung, Yu Yung Li, Yu Chuan Lin, Chun Fan Wei, Haw Ching Yang, and Fan Tien Cheng. 2017. “Development of a Novel Cloud-Based Multi-Tenant Model Creation Service for Automatic Virtual Metrology.” Robotics and Computer-Integrated Manufacturing 44: 174–189. doi:https://doi.org/10.1016/j.rcim.2016.09.003.
- Hung, Min Hsiung, Yu Chuan Lin, Hsien Cheng Huang, Min Hsuan Hsieh, Haw Ching Yang, and Fan Tien Cheng. 2013. “Development of an Advanced Manufacturing Cloud for Machine Tool Industry Based on AVM Technology.” In 2013 IEEE International Conference on Automation Science and Engineering, Madison, August 17–20, 2013, 189–194. Madison, WI: IEEE. doi:https://doi.org/10.1109/CoASE.2013.6654048.
- Hung, Min Hsiung, Wen Huang Tsai, Haw Ching Yang, Yi Jhong Kao, and Fan Tien Cheng. 2012b. “A Novel Automatic Virtual Metrology System Architecture for TFT-LCD Industry Based on Main Memory Database.” Robotics and Computer-Integrated Manufacturing 28 (4): 559–568. doi:https://doi.org/10.1016/j.rcim.2012.01.002.
- Hyun Baek, Kye, Kiwook Song, Chonghun Han, Gilheyun Choi, Han Ku Cho, and Thomas F. Edgar. 2014. “Implementation of a Robust Virtual Metrology for Plasma Etching Through Effective Variable Selection and Recursive Update Technology.” Journal of Vacuum Science & Technology B, Nanotechnology and Microelectronics: Materials, Processing, Measurement, and Phenomena 32 (1): 012203. doi:https://doi.org/10.1116/1.4862254.
- Inman, Robert R, Dennis E. Blumenfeld, Ningjian Huang, and Jingshan Li. 2010. “Designing Production Systems for Quality: Research Opportunities from an Automotive Industry Perspective.” International Journal of Production Research 41 (9): 1953–1971. https://doi.org/https://doi.org/10.1080/0020754031000077293.
- Iskandar, Jimmy, and James Moyne. 2016. “Maintenance of Virtual Metrology Models.” In 2016 27th Annual SEMI Advanced Semiconductor Manufacturing Conference (ASMC), Saratoga Springs, May 16–19, 2016, 393–398. Saratoga Springs, NY: IEEE. doi:https://doi.org/10.1109/ASMC.2016.7491083.
- Jaber, Ghazal. 2013. “An Approach for Online Learning in the Presence of Concept Change.” Doctoral thesis, Université Paris Sud. https://tel.archives-ouvertes.fr/tel-00907486/document.
- Jebri, Mohammed Ali, El Mostafa el Adel, Guilaume Graton, Mustapha Ouladsine, and Jacques Pinaton. 2017a. “The Impact of the Virtual Metrology on a Run-to-Run Control for a Chemical Mechanical Planarization Process.” IFAC-PapersOnLine 50 (1): 6154–6159. doi:https://doi.org/10.1016/j.ifacol.2017.08.980.
- Jebri, Mohammed Ali, El Mostafa el Adel, Guilaume Graton, Mustapha Ouladsine, and Jacques Pinaton. 2017b. “Virtual Metrology Applied in Run-to-Run Control for a Chemical Mechanical Planarization Process.” Journal of Physics: Conference Series 783 (1): 012042. doi:https://doi.org/10.1088/1742-6596/783/1/012042.
- Jia, Xiaodong, Yuan Di, Jianshe Feng, Qibo Yang, Honghao Dai, and Jay Lee. 2018. “Adaptive Virtual Metrology for Semiconductor Chemical Mechanical Planarization Process Using GMDH-Type Polynomial Neural Networks.” Journal of Process Control 62: 44–54. doi:https://doi.org/10.1016/j.jprocont.2017.12.004.
- Jin, Xiaoning, Huanyi Shui, and Moshe Shpitalni. 2019. “Virtual Sensing and Virtual Metrology for Spatial Error Monitoring of Roll-to-Roll Manufacturing Systems.” CIRP Annals-Manufacturing Technology 68 (1): 491–494. doi:https://doi.org/10.1016/j.cirp.2019.04.108.
- Jonathan, Chang, Yung Cheng, and Fan Tien Cheng. 2006. “Manufacturability of Multivariate Applications in the Semiconductor Industry.” In 2006 IEEE International Conference on Automation Science and Engineering, Shanghai, October 8–10, 2006, 230–235. doi:https://doi.org/10.1109/COASE.2006.326885.
- Kabir, H. M. Dipu, Abbas Khosravi, Mohammad Anwar Hosen, and Saeid Nahavandi. 2018. “Neural Network-Based Uncertainty Quantification: A Survey of Methodologies and Applications.” IEEE Access 6: 36218–36234. doi:https://doi.org/10.1109/ACCESS.2018.2836917.
- Kang, Seokho. 2018. “On Effectiveness of Transfer Learning Approach for Neural Network-Based Virtual Metrology Modeling.” IEEE Transactions on Semiconductor Manufacturing 31 (1): 149–155. doi:https://doi.org/10.1109/TSM.2017.2787550.
- Kang, Seokho, and Pilsung Kang. 2017. “An Intelligent Virtual Metrology System with Adaptive Update for Semiconductor Manufacturing.” Journal of Process Control 52: 66–74. doi:https://doi.org/10.1016/j.jprocont.2017.02.002.
- Kang, Pilsung, Dongil Kim, and Sungzoon Cho. 2014. “Evaluating the Reliability Level of Virtual Metrology Results for Flexible Process Control: A Novelty Detection-Based Approach.” Pattern Analysis and Applications 17 (4): 863–881. doi:https://doi.org/10.1007/s10044-014-0386-6.
- Kang, Pilsung, Dongil Kim, Hyoung Joo Lee, Seungyong Doh, and Sungzoon Cho. 2011. “Virtual Metrology for Run-to-Run Control in Semiconductor Manufacturing.” Expert Systems with Applications 38 (3): 2508–2522. doi:https://doi.org/10.1016/j.eswa.2010.08.040.
- Kang, Pilsung, Hyoung Joo Lee, Sungzoon Cho, Dongil Kim, Jinwoo Park, Chan Kyoo Park, and Seungyong Doh. 2009. “A Virtual Metrology System for Semiconductor Manufacturing.” Expert Systems with Applications 36 (10): 12554–12561. doi:https://doi.org/10.1016/j.eswa.2009.05.053.
- Khakifirooz, Marzieh, Mahdi Fathi, and Chen Fu Chien. 2018. “Modelling and Decision Support System for Intelligent Manufacturing: An Empirical Study for Feedforward-Feedback Learning-Based Run-to-Run Controller for Semiconductor Dry-Etching Process.” International Journal of Industrial Engineering 25 (6): 828–842. https://journals.sfu.ca/ijietap/index.php/ijie/article/view/4871.
- Khan, Aftab A., James R. Moyne, and Dawn M. Tilbury. 2007. “An Approach for Factory-Wide Control Utilizing Virtual Metrology.” IEEE Transactions on Semiconductor Manufacturing 20 (4): 364–375. doi:https://doi.org/10.1109/TSM.2007.907609.
- Kim, Sinyoung, Jaeyeon Jang, and Chang Ouk Kim. 2020. “A Run-to-Run Controller for a Chemical Mechanical Planarization Process Using Least Squares Generative Adversarial Networks.” Journal of Intelligent Manufacturing, doi:https://doi.org/10.1007/s10845-020-01639-1.
- Kim, Dongil, and Seokho Kang. 2019. “Effect of Irrelevant Variables on Faulty Wafer Detection in Semiconductor Manufacturing.” Energies 12 (13): 2530. doi:https://doi.org/10.3390/en12132530.
- Kim, Dongil, Pilsung Kang, Sungzoon Cho, Hyoung Joo Lee, and Seungyong Doh. 2012. “Machine Learning-Based Novelty Detection for Faulty Wafer Detection in Semiconductor Manufacturing.” Expert Systems with Applications 39 (4): 4075–4083. doi:https://doi.org/10.1016/j.eswa.2011.09.088.
- Kim, Misuk, Seokho Kang, Jehyuk Lee, Hyunchang Cho, Sungzoon Cho, and Jee Su Park. 2017. “Virtual Metrology for Copper-Clad Laminate Manufacturing.” Computers and Industrial Engineering 109: 280–287. doi:https://doi.org/10.1016/j.cie.2017.04.016.
- Kim, Dongil, Pilsung Kang, Seung-Kyung Lee, Seokho Kang, Seungyong Doh, and Sungzoon Cho. 2015. “Improvement of Virtual Metrology Performance by Removing Metrology Noises in a Training Dataset.” Pattern Analysis and Applications 18 (1): 173–189. doi:https://doi.org/10.1007/s10044-013-0363-5.
- Kim, Kyung-Jun, Kyu-Jin Kim, Chi-Hyuck Jun, Il-Gyo Chong, and Geun-Young Song. 2019a. “Variable Selection Under Missing Values and Unlabeled Data in Semiconductor Processes.” IEEE Transactions on Semiconductor Manufacturing 32 (1): 121–128. doi:https://doi.org/10.1109/TSM.2018.2881286.
- Kim, Dongil, Jeongin Koo, Hyein Kim, Seokho Kang, Sang Hyun Lee, and Jeong Tae Kang. 2019b. “Rapid Fault Cause Identification in Surface Mount Technology Processes Based on Factory-Wide Data Analysis.” International Journal of Distributed Sensor Networks 15 (2): 1–15. doi:https://doi.org/10.1177/1550147719832802.
- Kiraci, Ercihan, P. Franciosa, J. A. Warnett, and Mark A. Williams. 2017. “Non-Reproducible Alignment and Fitting Algorithm Effects on Laser Radar Measurement.” In 2017 IEEE International Instrumentation and Measurement Technology Conference (I2MTC), Turin, May 22–25, 2017, 1–5. Turin: IEEE doi:https://doi.org/10.1109/I2MTC.2017.7969967.
- Kitchenham, Barbara. 2004. Procedures for Performing Systematic Reviews. Keele: Keele University. http://www.elizabete.com.br/rs/Tutorial_IHC_2012_files/Conceitos_RevisaoSistematica_kitchenham_2004.pdf.
- Kuhn, Max, and Kjell Johnson. 2013. Applied Predictive Modeling. New York: Springer. doi:https://doi.org/10.1007/978-1-4614-6849-3
- Kuo, Yong-Hong, and Andrew Kusiak. 2019. “From Data to Big Data in Production Research: The Past and Future Trends.” International Journal of Production Research 57 (15-16): 4828–4853. https://doi.org/https://doi.org/10.1080/00207543.2018.1443230.
- Kurz, Daniel, Cristina De Luca, and Jürgen Pilz. 2015. “A Sampling Decision System for Virtual Metrology in Semiconductor Manufacturing.” IEEE Transactions on Automation Science and Engineering 12 (1): 75–83. doi:https://doi.org/10.1109/TASE.2014.2360214.
- Lakshminarayanan, Balaji, Alexander Pritzel, and Charles Blundell. 2017. “Simple and Scalable Predictive Uncertainty Estimation Using Deep Ensembles.” In Proceedings of the 31st International Conference on Neural Information Processing Systems, Long Beach, December 4–9, 2017, 6405–6416. Long Beach, CA: Curran Associates. https://dl.acm.org/doi/https://doi.org/10.5555/3295222.3295387.
- Lee, Seung kyung, Pilsung Kang, and Sungzoon Cho. 2014. “Probabilistic Local Reconstruction for K-NN Regression and its Application to Virtual Metrology in Semiconductor Manufacturing.” Neurocomputing 131: 427–439. doi:https://doi.org/10.1016/j.neucom.2013.10.001.
- Lee, Ki Bum, and Chang Ouk Kim. 2020. “Recurrent Feature-Incorporated Convolutional Neural Network for Virtual Metrology of the Chemical Mechanical Planarization Process.” Journal of Intelligent Manufacturing 31 (1): 73–86. https://doi.org/https://doi.org/10.1007/s10845-018-1437-4.
- Lewis, David D., and William A. Gale. 1994. “A Sequential Algorithm for Training Text Classifiers.” In SIGIR ‘94, edited by Bruce W. Croft, and C. J. Van Rijsbergen, 3–12. London: Springer.
- Li, Zhixiong, Dazhong Wu, and Tianyu Yu. 2019. “Prediction of Material Removal Rate for Chemical Mechanical Planarization Using Decision Tree-Based Ensemble Learning.” Journal of Manufacturing Science and Engineering 141 (3): 031003. doi:https://doi.org/10.1115/1.4042051.
- Lin, Kuo Yi, Chia Yu Hsu, and Hui Chun Yu. 2014. “A Virtual Metrology Approach for Maintenance Compensation to Improve Yield in Semiconductor Manufacturing.” International Journal of Computational Intelligence Systems 7 (Sup2): 66–73. doi:https://doi.org/10.1080/18756891.2014.947116.
- Lin, Chien-Hua, Sheng-Tsaing Tseng, and Hsiang-Fan Wang. 2013. “Modified EWMA Controller Subject to Metrology Delay.” IIE Transactions 45 (4): 409–421. doi:https://doi.org/10.1080/0740817X.2012.689242.
- Lynn, Shane A., Niall Macgearailt, and John V. Ringwood. 2012a. “Real-Time Virtual Metrology and Control for Plasma Etch.” Journal of Process Control 22 (4): 666–676. doi:https://doi.org/10.1016/j.jprocont.2012.01.012.
- Lynn, Shane A, John V. Ringwood, and Niall MacGearailt. 2010. “Weighted Windowed PLS Models for Virtual Metrology of an Industrial Plasma Etch Process.” In 2010 IEEE International Conference on Industrial Technology, Via del Mar, March 14–17, 2010, 309–314. Via del Mar, Chile: IEEE. doi:https://doi.org/10.1109/ICIT.2010.5472698.
- Lynn, Shane A., John Ringwood, and Niall MacGearailt. 2012b. “Global and Local Virtual Metrology Models for a Plasma Etch Process.” IEEE Transactions on Semiconductor Manufacturing 25 (1): 94–103. doi:https://doi.org/10.1109/TSM.2011.2176759.
- Michl, Markus, Matthias Koitzsh, Jochen Merhof, Humbert Noll, Alexander Nemecek, Alfred Honold, Gerhard Kleineidam, and Holger Lebrecht. 2011. “Implementing Virtual Metrology into Semiconductor Production – An Investment Assessment.” In Proceedings of the 2011 Winter Simulation Conference (WSC), Phoenix, December 11–14, 2011, 2017–2018. Phoenix, AZ: IEEE. doi:https://doi.org/10.1109/WSC.2011.6147915.
- Moyne, James, Enrique Del Castillo, and Arnon Max Hurwitz. 2000. Run-to-Run Control in Semiconductor Manufacturing. Florida: CRC Press.
- Moyne, James, Jamini Samantaray, and Michael Armacost. 2016. “Big Data Capabilities Applied to Semiconductor Manufacturing Advanced Process Control.” IEEE Transactions on Semiconductor Manufacturing 29 (4): 283–291. doi:https://doi.org/10.1109/TSM.2016.2574130.
- Moyne, James, Manjunath Yedatore, Jimmy Iskandar, Parris Hawkins, and John Scoville. 2014. “Chamber Matching Across Multiple Dimensions Utilizing Predictive Maintenance, Equipment Health Monitoring, Virtual Metrology and Run-To-Run Control.” In 25th Annual SEMI Advanced Semiconductor Manufacturing Conference (ASMC 2014), Saratoga Springs, May 19–21, 2014, 86–91. Saratoga Springs, NY: IEEE. doi:https://doi.org/10.1109/ASMC.2014.6846983.
- Nguyen, Cuong Manh, Xin Li, Ronald de Shawn Blanton, and Xiang Li. 2017. “Partial Co-Training for Virtual Metrology.” In 2017 22nd IEEE International Conference on Emerging Technologies and Factory Automation (ETFA), Limassol, September 12–15, 2017, 1–8. Limassol, Cyprus: IEEE. doi:https://doi.org/10.1109/ETFA.2017.8247660.
- Nguyen, Cuong Manh, Xin Li, Ronald de Shawn Blanton, and Xiang Li. 2020. “Partial Bayesian Co-Training for Virtual Metrology.” IEEE Transactions on Industrial Informatics 16 (5): 2937–2945. doi:https://doi.org/10.1109/TII.2019.2903718.
- Pan, Tian-Hong, Bi-Qi Sheng, David Shan-Hill Wong, and Shi-Shang Jang. 2011. “A Virtual Metrology System for Predicting End-of-Line Electrical Properties Using a MANCOVA Model with Tools Clustering.” IEEE Transactions on Industrial Informatics 7 (2): 187–195. doi:https://doi.org/10.1109/TII.2010.2098416.
- Park, Seolhye, Yongsuk Jang, Taewon Cha, Yeongil Noh, Younghoon Choi, Juyoung Lee, Jaegu Seong, et al. 2020. “Predictive Control of the Plasma Processes in the OLED Display Mass Production Referring to the Discontinuity Qualifying PI-VM.” Physics of Plasmas 27 (8): 083507. doi:https://doi.org/10.1063/1.5135312.
- Park, Chanhee, Younghoon Kim, Youngjoon Park, and Seoung Bum Kim. 2018. “Multitask Learning for Virtual Metrology in Semiconductor Manufacturing Systems.” Computers and Industrial Engineering 123: 209–219. doi:https://doi.org/10.1016/j.cie.2018.06.024.
- Psarommatis, Foivos. 2021. “A Generic Methodology and a Digital Twin for Zero Defect Manufacturing (ZDM) Performance Mapping Towards Design for ZDM.” Journal of Manufacturing Systems 59: 507–521. doi:https://doi.org/10.1016/j.jmsy.2021.03.021.
- Psarommatis, Foivos, Ali Gharaei, and Dimitris Kiritsis. 2020a. “Identification of the Critical Reaction Times for Re-Scheduling Flexible Job Shops for Different Types of Unexpected Events.” Procedia CIRP 93: 903–908. doi:https://doi.org/10.1016/j.procir.2020.03.038.
- Psarommatis, Foivos, and Dimitris Kiritsis. 2018. “A Scheduling Tool for Achieving Zero Defect Manufacturing (ZDM): A Conceptual Framework.” In Advances in Production Management Systems: Smart Manufacturing for Industry 4.0, edited by Ilkyeong Moon, Gyu M. Lee, Jinwoo Park, Dimitris Kiritsis, and Gregor Von Cieminski, 271–278. Cham: Springer.
- Psarommatis, Foivos, and Dimitris Kiritsis. 2019. “Identification of the Inspection Specifications for Achieving Zero Defect Manufacturing.” In Advances in Production Management Systems: Production Management for the Factory of the Future, edited by Farhad Ameri, Kathryn E. Stecke, Gregor Von Cieminski, and Dimitris Kiritsis, 267–273. Cham: Springer.
- Psarommatis, Foivos, Gökan May, Paul Arthur Dreyfus, and Dimitris Kiritsis. 2020b. “Zero Defect Manufacturing: State-of-the-Art Review, Shortcomings and Future Directions in Research.” International Journal of Production Research 58 (1): 1–17. doi:https://doi.org/10.1080/00207543.2019.1605228.
- Psarommatis, Foivos, Sylvain Prouvost, Gökan May, and Dimitris Kiritsis. 2020c. “Product Quality Improvement Policies in Industry 4.0: Characteristics, Enabling Factors, Barriers, and Evolution Toward Zero Defect Manufacturing.” Frontiers in Computer Science 2 (26), doi:https://doi.org/10.3389/fcomp.2020.00026.
- Purwins, Hendrik, Bernd Barak, Ahmed Nagi, Reiner Engel, Uwe Hockele, Andreas Kyek, Srikanth Cherla, Benjamin Lenz, Gunter Pfeifer, and Kurt Weinzierl. 2014. “Regression Methods for Virtual Metrology of Layer Thickness in Chemical Vapor Deposition.” IEEE/ASME Transactions on Mechatronics 19 (1): 1–8. doi:https://doi.org/10.1109/TMECH.2013.2273435.
- Rashid, Muhammad, Muhammad Waseem Anwar, and Aamir M. Khan. 2015. “Toward the Tools Selection in Model Based System Engineering for Embedded Systems - A Systematic Literature Review.” Journal of Systems and Software 106: 150–163. doi:https://doi.org/10.1016/j.jss.2015.04.089.
- Ringwood, John V., Shane Lynn, Giorgio Bacelli, Beibei Ma, Emanuele Ragnoli, and Sean McLoone. 2010. “Estimation and Control in Semiconductor Etch: Practice and Possibilities.” IEEE Transactions on Semiconductor Manufacturing 23 (1): 87–98. doi:https://doi.org/10.1109/TSM.2009.2039250.
- Roeder, Georg, Andreas Mattes, Markus Pfeffer, Martin Schellenberger, Lothar Pfitzner, Alexander Knapp, and Heribert Mühlberger. 2012. “Framework for Integration of Virtual Metrology and Predictive Maintenance.” In 2012 SEMI Advanced Semiconductor Manufacturing Conference, Saratoga Springs, May 15–17, 2012, 288–293. Saratoga Springs, NY: IEEE. doi:https://doi.org/10.1109/ASMC.2012.6212913.
- Rolnick, David, Andreas Veit, Serge Belongie, and Nir Shavit. 2017. “Deep Learning is Robust to Massive Label Noise.” Preprint. https://arxiv.org/pdf/1705.10694.pdf.
- Schirru, Andrea, Simone Pampuri, Cristina De Luca, and Giuseppe De Nicolao. 2011. “Multilevel Kernel Methods for Virtual Metrology in Semiconductor Manufacturing.” IFAC Proceedings Volumes 44 (1): 11614–11621. doi:https://doi.org/10.3182/20110828-6-IT-1002.01339.
- Shui, Huanyi, Xiaoning Jin, and Jun Ni. 2019. “Twofold Variation Propagation Modeling and Analysis for Roll-to-Roll Manufacturing Systems.” IEEE Transactions on Automation Science and Engineering 16 (2): 599–612. doi:https://doi.org/10.1109/TASE.2018.2844168.
- Su, Yu Chuan, Wen Huang Tsai, Fan Tien Cheng, and Wei Ming Wu. 2008. “Development of a Dual-Stage Virtual Metrology Architecture for TFT-LCD Manufacturing.” In 2008 IEEE International Conference on Robotics and Automation, Pasadena, May 19–23, 2008, 3630–3635. Pasadena, CA: IEEE. doi:https://doi.org/10.1109/ROBOT.2008.4543767.
- Susto, Gian Antonio. 2017. “A Dynamic Sampling Strategy Based on Confidence Level of Virtual Metrology Predictions.” In 2017 28th Annual SEMI Advanced Semiconductor Manufacturing Conference (ASMC), Saratoga Springs, May 15–18, 2017, 78–83. Saratoga Springs, NY: IEEE. doi:https://doi.org/10.1109/asmc.2017.7969203.
- Susto, Gian Antonio, Simone Pampuri, Andrea Schirru, Alessandro Beghi, and Giuseppe De Nicolao. 2015. “Multi-Step Virtual Metrology for Semiconductor Manufacturing: A Multilevel and Regularization Methods-Based Approach.” Computers and Operations Research, doi:https://doi.org/10.1016/j.cor.2014.05.008.
- Tang Hsuan, O., Min Hsiung Hung, Yu Chuan Lin, and Chao Chun Chen. 2020. “Construction of Virtual Metrology Cloud Platform with Machine Learning Tools for Providing Factory-Wide Manufacturing Service.” In Intelligent Information and Database Systems: ACIIDS 2020, edited by Pawel Sitek, Marcin Pietranik, Marek Krótkiewicz, and Chutimet Srinilta, 47–59. Singapore: Springer.
- Tannock, James, and Sittichai Saelem. 2007. “Manufacturing Disruption Costs Due to Quality Loss.” International Journal of Quality and Reliability Management 24 (3): 263–278. doi:https://doi.org/10.1108/02656710710730861.
- Tieng, Hao, Chun Fang Chen, Fan Tien Cheng, and Haw Ching Yang. 2017. “Automatic Virtual Metrology and Target Value Adjustment for Mass Customization.” IEEE Robotics and Automation Letters 2 (2): 546–553. doi:https://doi.org/10.1109/LRA.2016.2645507.
- Tieng, Hao, Tsung Han Tsai, Chun Fang Chen, Haw Ching Yang, Jhih Wei Huang, and Fan Tien Cheng. 2018. “Automatic Virtual Metrology and Deformation Fusion Scheme for Engine-Case Manufacturing.” IEEE Robotics and Automation Letters 3 (2): 934–941. doi:https://doi.org/10.1109/LRA.2018.2792690.
- Tilouche, Shaima, Samuel Bassetto, and Vahid Partovi Nia. 2014. “Classification Algorithms for Virtual Metrology.” In 2014 IEEE International Conference on Management of Innovation and Technology, Singapore, September 23–25, 2014, 495–499. Singapore: IEEE. doi:https://doi.org/10.1109/ICMIT.2014.6942477.
- Tsutsui, Takuro, and Takahito Matsuzawa. 2019. “Virtual Metrology Model Robustness Against Chamber Condition Variation Using Deep Learning.” IEEE Transactions on Semiconductor Manufacturing 32 (4): 428–433. doi:https://doi.org/10.1109/TSM.2019.2931328.
- Ul Haq, Asad Arsalan, and Dragan Djurdjanovic. 2019. “Dynamics-Inspired Feature Extraction in Semiconductor Manufacturing Processes.” Journal of Industrial Information Integration 13: 22–31. doi:https://doi.org/10.1016/j.jii.2018.12.001.
- Urhan, Aysun, and Burak Alakent. 2020. “Integrating Adaptive Moving Window and Just-in-Time Learning Paradigms for Soft-Sensor Design.” Neurocomputing 392: 23–37. doi:https://doi.org/10.1016/j.neucom.2020.01.083.
- Vallejo, Marcela, Carolina De La Espriella, Juliana Gómez-Santamaría, Andrés Felipe Ramírez-Barrera, and Edilson Delgado-Trejos. 2019. “Soft Metrology Based on Machine Learning: A Review.” Measurement Science and Technology 31 (3): 032001. doi:https://doi.org/10.1088/1361-6501/ab4b39.
- Vaswani, Ashish, Noam Shazeer, Niki Parmar, Jakob Uszkoreit, Llion Jones, Aidan N. Gomez, Łukasz Kaiser, and Illia Polosukhin. 2017. “Attention is All You Need.” Preprint. https://arxiv.org/pdf/1706.03762.pdf.
- Wadewale, K., and S. Desai. 2015. “Survey on Method of Drift Detection and Classification for Time Varying Data Set.” International Research Journal of Engineering and Technology 2 (9): 709–713. https://www.academia.edu/34972323/Survey_on_Method_of_Drift_Detection_and_Classification_for_time_varying_data_set.
- Wan, Jian, Bahman Honari, and Sean McLoone. 2013. “A Dynamic Sampling Methodology for Plasma Etch Processes Using Gaussian Process Regression.” 2013 XXIV International Conference on Information, Communication and Automation Technologies (ICAT), Saravejo, October 30-November 1, 2013, 1–6. Saravejo: IEEE. doi:https://doi.org/10.1109/ICAT.2013.6684080.
- Widmer, Gerhard, and Miroslav Kubat. 1996. “Learning in the Presence of Concept Drift and Hidden Contexts.” Machine Learning 23 (1): 69–101. doi:https://doi.org/10.1007/bf00116900.
- Wu, Xiaofei, Junghui Chen, Lei Xie, Lester Lik Teck Chan, and Chun I. Chen. 2020. “Development of Convolutional Neural Network Based Gaussian Process Regression to Construct a Novel Probabilistic Virtual Metrology in Multi-Stage Semiconductor Processes.” Control Engineering Practice 96: 104262. doi:https://doi.org/10.1016/j.conengprac.2019.104262.
- Wu, Wei Ming, Fan Tien Cheng, and Fan Wei Kong. 2012. “Dynamic-Moving-Window Scheme for Virtual-Metrology Model Refreshing.” IEEE Transactions on Semiconductor Manufacturing 25 (2): 238–246. doi:https://doi.org/10.1109/TSM.2012.2183398.
- Yang, Haw-Ching, Muhammad Adnan, Chih-Hung Huang, Fan-Tien Cheng, Yu-Lung Lo, and Chih-Hua Hsu. 2019. “An Intelligent Metrology Architecture with AVM for Metal Additive Manufacturing.” IEEE Robotics and Automation Letters 4 (3): 2886–2893. doi:https://doi.org/10.1109/LRA.2019.2921927.
- Yang, Wei Ting, Jakey Blue, Agnès Roussy, Jacques Pinaton, and Marco S. Reis. 2020. “A Physics-Informed Run-to-Run Control Framework for Semiconductor Manufacturing.” Expert Systems with Applications 155: 113424. doi:https://doi.org/10.1016/j.eswa.2020.113424.
- Yang, Haw Ching, Hao Tieng, and Fan Tien Cheng. 2016. “Total Precision Inspection of Machine Tools with Virtual Metrology.” Journal of the Chinese Institute of Engineers 39 (2): 221–235. doi:https://doi.org/10.1080/02533839.2015.1091279.
- Zeng, Dekong, and Costas J. Spanos. 2009. “Virtual Metrology Modeling for Plasma Etch Operations.” IEEE Transactions on Semiconductor Manufacturing 22 (4): 419–431. doi:https://doi.org/10.1109/TSM.2009.2031750.
- Zhou, Liangchuan, Surendra M. Gupta, Yuki Kinoshita, and Tetsuo Yamada. 2017. “Pricing Decision Models for Remanufactured Short-Life Cycle Technology Products with Generation Consideration.” Procedia CIRP 61: 195–200. doi:https://doi.org/10.1016/j.procir.2016.11.208.