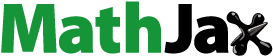
Abstract
Successful inter-organisational new product development (NPD) is a key source of competitive advantage, yet many NPD projects are still failing. To generate new insights on the phenomenon, we examine the performance implications of knowledge inputs among collaborating organisations, both in terms of external input ratio and input concentration. We test a set of hypotheses using multi-industry primary and secondary data on 210 inter-organisational NPD projects. Results support our hypotheses about the negative effect of input concentration on design quality, which in turn is positively associated with product market performance. Although we surprisingly find a negative linear effect of external input ratio on design quality, our post-hoc analysis confirms the relationship to be an inverse U-shaped relationship. We also find support for the roles of technology interdependence in moderating the performance implications of external input ratio and input concentration. By clarifying how knowledge inputs affect project outcomes under different levels of technology interdependence, the study provides managerial implications regarding how to select innovation partners in order to create a knowledge portfolio that best improves NPD project performance.
1. Introduction
Successful new product development (NPD) projects, as a form of firm innovation, can generate and sustain competitive advantage across all industries (Ettlie and Pavlou Citation2006; Sivadas and Dwyer Citation2000). As a first and crucial step in a firm’s production system, the NPD process constitutes the fuzzy front end of a manufacturer’s value creation process (Schoenherr and Wagner Citation2016). During the NPD process, a firm needs to make important product and supply chain design decisions, which significantly affect the performance of firm operations in the marketing and production stages (Swink and Calantone Citation2004). A high quality product design that meets customer requirements, a main outcome resulting from a successful NPD process, could save manufacturers millions of dollars in the mass production stage, while ensuring sufficient market revenue to compensate for the high R&D investment (Frankort Citation2016; Choo, Chandrasekaran, and Chinaprayoon Citation2020). Therefore, successful NPD projects are a key driver for firm performance (Rubera, Chandrasekaran, and Ordanini Citation2016; Hagedoorn, Lokshin, and Zobel Citation2018).
To bring new products more quickly to the market in an efficient manner, firms often need to collaborate with customers, suppliers and other types of organisations in NPD projects and tap into specific market knowledge and technological resources to lower market and development risks (Jayaram Citation2008). The Artemis project of the car manufacturer Audi, for example, underlines the criticality of bringing together knowledge from various partners for NPD. The Volkswagen Group, including Audi, has worked on many individual projects related to autonomous driving over more than 15 years and extended its internal R&D capabilities in this area with knowledge from external partners (Volkswagen Citation2017). As early as 2005, Volkswagen collaborated with specialists from Stanford University with the outcome of a rudimentary autonomously driving ‘robot’ vehicle. As the former head of research states: ‘Cooperation and collaboration are core concepts for Volkswagen Research’ (Volkswagen 2017). Collaborative inter-organisational NPD projects could potentially improve design quality and market performance of a new product, if external knowledge inputs are effectively integrated with internal knowledge to fully realise their synergy. However, the McKinsey Global Institute estimates that between 25% and 45% of new products never make it to market or fail at market entry (De Jong, Marston, and Roth Citation2015), showing that collaborative inter-organisational NPD projects remain a risky endeavour. Therefore, it is still important to gain more insights on the contingencies that help predict project performance.
Since market and technological knowledge is an important resource for developing new products, the knowledge portfolio available at the beginning of a NPD project should play a critical role for NPD success (Chesbrough Citation2003; Lawson et al. Citation2009; Kahn et al. Citation2012). A few strategic management and innovation studies have suggested the importance of resource portfolios on a firm level (e.g. Grand et al. Citation2004; York and Venkataraman Citation2010; Bolumole et al. Citation2015). However, the effects of the knowledge portfolio at an inter-organisational NPD project level has received little academic attention. The knowledge portfolio is composed of the knowledge inputs from both the focal firm and all types of external project partnering organisations (Wagner Citation2012; von Briel, Schneider, and Lowry Citation2018). In the earlier example, Volkswagen Group has collaborated with different customers, suppliers and other organisations over the years. These external knowledge inputs were bundled with Volkswagen’s internal knowledge at Audi’s Autonomous Intelligent Driving unit, today the Artemis project, to produce multiple technology partnerships. For instance, in 2018, Audi collaborated with Luminar Technologies, a provider of laser imaging, detection, and ranging sensors and software for autonomous driving (Phelan Citation2018). Internal knowledge inputs came from some of the 14 associated Volkswagen brands. The NPD literature has also shown that the proportion of knowledge inputs from internal and external sources could vary significantly from projects to projects (Taylor and Poltz Citation2020). Together, this evidence shows the importance of studying characteristics of knowledge inputs to see how they affect inter-organisational NPD outcomes. Specifically focusing on inputs and not on the knowledge generated during the development process, we study the effects of two knowledge input characteristics on product design and ultimately on project performance: (1) external input ratio and (2) input concentration.
The NPD literature has also widely shown that the success of inter-organisational NPD projects is contingent on product and project factors (Wowak et al. Citation2016; Yan and Azadegan Citation2017). One group of contingencies is related to the characteristics of the product and the adopted technology (Chen, Reilly, and Lynn Citation2005; Swink and Calantone Citation2004). However, research has focused on a limited set of characteristics, such as product novelty and complexity, which are on a strategic level and not directly under the discretion of the focal firm (Schoenherr and Wagner Citation2016; Carlo, Lyytinen, and Rose Citation2012). Instead, product technological architecture is an important operational level, engineering design factor that has not received enough attention from the NPD literature but at the same time could influence the types and amounts of knowledge inputs needed to facilitate successful NPD (Bstieler Citation2005; Yan and Dooley Citation2013). Thus, we introduce to our model such a factor: technology interdependence of a product, defined as the degree of interdependence among the product and process technologies to be developed (Swink and Calantone Citation2004). In the Artemis project, core technologies include the global positioning system, detection and ranging system, automated steering, electronic engine control, and software that used data input from all systems to make decision about the car’s speed and direction (Taylor and Poltz Citation2020). These technologies are highly dependent on each other for the car to function well. As technology interdependence is likely to affect the absorption and integration of internal and external knowledge inputs, we examine the moderating roles of technology interdependence.
In sum, we study the effects of knowledge inputs for NPD project success under different levels of technology interdependence. Specifically, we examine how external input ratio and input concentration affect design quality and subsequent product market performance. To test our hypotheses, we use primary and secondary data on 210 NPD projects in multiple industries. Results support our hypotheses about the negative effect of input concentration on design quality, which in turn is positively associated with product market performance. Interestingly, our post-hoc analysis shows an inverse U-shaped relationship between external input ratio and design quality, although the main effect of external input ratio on design quality is negative. We also find support for the moderating role of technology interdependence in affecting performance implications of both external input ratio and input concentration.
The study contributes to the literature in three ways. First, we contribute to the inter-organisational NPD literature by showing that the characteristics of knowledge inputs are a yet overlooked but influential factor to the success of inter-organisational NPD projects. Second, we reveal the moderating roles of technology interdependence for understanding how knowledge inputs affect design quality in different architectures of technological bundles. Our findings also support the general notion that product characteristics could affect knowledge needs, which provides insights about aligning engineering design decisions with project partner selection. Understanding both positive and negative performance implications of knowledge inputs, NPD managers could better select NPD partners by creating a knowledge portfolio that best fits the level of technology interdependence.
2. Related literature
2.1. Inter-organisational new product development
Inter-organisational NPD projects involve the active engagement of suppliers, customers and other non-supply chain partners, such as governmental institutions, consulting agencies, R&D institutes and universities (Petersen, Handfield, and Ragatz Citation2005; Najafi Tavani et al. Citation2013). While empirical evidence suggests that inter-organisational NPD strategies could lead to better product design quality and product market performance (Petersen, Handfield, and Ragatz Citation2005; Primo and Amundson Citation2002), these projects are not always successful (Yan and Dooley Citation2013). Exploring the factors and mechanisms for successful NPD yields valuable insight for scholars and practitioners.
Research has formed three distinct literature streams on inter-organisational NPD project success. The first group of studies takes on a relational view, assuming that the quality of the inherent inter-organisational relationships determines NPD project success (Cao and Zhang Citation2011; Mishra and Shah Citation2009). A second group specifically studies how, when and for how long to involve external organisations in order to improve NPD outcomes (Schoenherr and Wagner Citation2016; Wagner Citation2012). The third group investigates the effects of project organisation on the success of inter-organisational NPD projects (Kach, Azadegan, and Dooley Citation2012; Yan and Nair Citation2016). However, the existing literature has not given enough attention to knowledge management in inter-organisational NPD projects. As firms reach beyond organisational boundaries, especially to satisfy the increasing knowledge needs in NPD projects, the focal firm’s knowledge management capability might be a key success factor (Cassiman and Veugelers Citation2006; Nonaka Citation1994; Pathak et al. Citation2019).
2.2. Knowledge management in inter-organisational new product development
From a knowledge management view, the inter-organisational NPD literature has shown the importance of selecting and managing different knowledge sources, i.e. the source’s position in the value chain, which affect the types of knowledge contributed and the ‘stickiness’ of that knowledge (Schoenherr and Wagner Citation2016; Wowak et al. Citation2016). First, prior studies have identified customers, suppliers, consultants, and universities as key knowledge sources for product innovation activities (Chesbrough and Brunswicker Citation2014; Laursen and Salter Citation2006). Researchers have compared performance implications of different knowledge sources for product novelty, design and financial performance of a new product. However, no conclusive findings have been identified (Leiponen and Helfat Citation2011; Wang, Modi, and Schoenherr Citation2020; Yu, Duan, and Fan Citation2020). Second, the literature has also called attention to consider different types of knowledge needs, i.e. knowledge about the technical product and process, or market knowledge (Modi and Mabert Citation2007). Third, NPD performance could also be affected by the characteristics of the knowledge (Kogut and Zander Citation1992; Madhavan and Grover Citation1998; Pathak et al. Citation2019). For example, experience-based know-how for NPD is notoriously hard to access if a focal firm does not go through the learning process to acquire the know-how itself (Ngai, Jin, and Liang Citation2008; Szulanski Citation1996). In short, this knowledge management branch of inter-organisational NPD literature highlights the importance of understanding different types and sources of knowledge for successfully managing projects.
Viewing knowledge as a critical innovation resource, we also need to build upon the resource allocation/distribution literature in inter-organisational innovation in order to study the influences of knowledge distribution on project performance (Garcia, Calantone, and Levine Citation2003; Grand et al. Citation2004; Meier Citation2011; York and Venkataraman Citation2010). On the firm level, Klingebiel and Rammer (Citation2014) find that a firm’s innovation performance is influenced by their choice of resource allocation strategy. They recommend a broad resource allocation strategy, understood as investing in a wide range of NPD projects, to increase revenue. Vasudeva and Anand (Citation2011) examined how firms can access and acquire knowledge from shared alliance innovation portfolios. They derive different strategies to optimise knowledge utilisation. On a project level, resource contribution, allocation and distribution has received insufficient attention, with some notable exceptions (Choo, Chandrasekaran, and Chinaprayoon Citation2020; Fang Citation2011; Frankort Citation2016; Rothaermel and Deeds Citation2004). Mudambi and Tallman (Citation2010), for instance, studied innovation projects and characterise knowledge management outsourcing as ‘make-or-ally decision’. They provide a framework for how to govern the resulting project partnerships in the context of sensitive knowledge assets. Capaldo and Petruzzelli (Citation2014) focused on contributions and knowledge creations in project partnerships and performance implications. Although these studies all allude to the importance of studying knowledge distribution in inter-organisational NPD projects, this area is still lacking academic attention.
In addition, multiple studies show the effects of product characteristics on the success of the NPD project (Bstieler Citation2005; Tatikonda and Rosenthal Citation2000b). The engineering/technology-SCM interfacing literature has shown the importance to consider the alignment between product architecture and inter-organisational strategies when predicting innovation outcomes (Fine Citation2000; Boutellier and Wagner Citation2003; Ülkü and Schmidt Citation2011; Nepal, Monplaisir, and Famuyiwa Citation2012; Pashaei and Olhager Citation2015). However, no study has examined how technological interdependence, a critical product architecture design decision, moderate the influences of knowledge inputs from various types of organisations on NPD project outcomes. Technology interdependence refers to the degree of interdependence among the product and process technologies to be developed (Sosa, Eppinger, and Rowles Citation2004; Tatikonda and Rosenthal Citation2000b). For example, Yan and Wagner (Citation2017) study the role of conflicts on NPD project success. They find that product novelty and technology interdependence affect project conflicts and subsequently value creation and appropriation. Similarly, we argue that the technology interdependence of a product influences how different knowledge inputs drive NPD project performance. It is conceivable that, for example, the knowledge portfolio needed to develop a product with low technology interdependence and thus higher modularity is different from the one necessary to successfully design an integral new product. Therefore, our study fills this gap in the literature by exploring technology interdependence as a contingency.
Building upon the above inter-organisational innovation studies and knowledge management, this study attempts to fill two gaps in the literature: (1) a lack of attention to the performance implications of knowledge distribution, and (2) the moderating effects of technology interdependence on performance implications of knowledge distribution. Building upon this stream of work, we will explain below how effects of external input ratio and input concentration vary with different levels of technology interdependence.
3. Theory development
In this study, we examine the effects of knowledge inputs to a NPD project on product design quality. Specifically, we look at the external ratio and concentration of these inputs. In addition, we explore technology interdependence as a contingency to our proposed relationships. Our constructs are formally defined in Table .
Table 1. Description of the constructs used.
3.1. Knowledge inputs and knowledge integration
In this study, we see knowledge inputs to a NPD project as intangible resources (i.e. knowledge) in various forms. The knowledge inputs can come from the focal firm or any other partnering organisation, including suppliers, customers and other organisations. We specifically focus on the knowledge inputs, and not the knowledge that is created throughout the NPD process. To examine the effects of knowledge inputs on NPD performance, we specifically look at two main characteristics of the input flows: (1) the external input ratio and (2) the input concentration. External input ratio refers to the ratio of knowledge contributed by external types of organisations to the knowledge inputs by external types of organisations plus the focal firm. Input concentration indicates the extent to which all the knowledge inputs come from a few types of organisations, including both the focal firm and external organisation types.
The knowledge portfolio that is available at the beginning of a NPD process is understood as the sum of all knowledge inputs by different partnering organisations. We propose a two-step process of how the knowledge can be used within the project: (1) understand and then (2) integrate the knowledge. First, knowledge has to be understood. The focal firm has a specific domain of internal knowledge that relates to the firm’s product and service domain (Lettice, Roth, and Forstenlechner Citation2006). A mismatch or distance between knowledge domains of the firm and the knowledge inputs poses the challenge of adequately understanding the knowledge (Grant and Baden Fuller Citation2004; Yan and Azadegan Citation2017). Understanding depends on sufficient overlap between knowledge domains. Second, once knowledge is understood, it has to be integrated. While we acknowledge that during the NPD process, knowledge is always transferred among partnering organisations, different sources of knowledge have to be integrated before it can be utilised for meeting NPD project needs (Becker and Zirpoli Citation2003; Grant and Baden Fuller Citation2004). Characteristics of knowledge, such as contents and tangibility, could affect the challenges for the focal firm to realise synergies from integrating knowledge inputs. For instance, some tacit, hard-to-codify knowledge, such as practical skill or expertise that allows one to do something smoothly and efficient, could be very challenging to be transferred to a new context to integrate with other knowledge inputs (von Hippel Citation1988). The literature has shown that integrating tacit knowledge for NPD is notoriously hard to achieve, problematic and costly (Ngai, Jin, and Liang Citation2008; Szulanski Citation1996).
3.2. Knowledge inputs and product design quality
To gain insights into the effects of knowledge inputs on product design quality, we develop a set of hypotheses related to the main characteristics of the knowledge inputs: (1) external input ratio, and (2) input concentration.
External input ratio. We propose that a high external input ratio increases the chance for a focal firm to integrate novel knowledge from external partners successfully, positively contributing to product design quality. When the knowledge inputs mainly originate from external types of organisations, the knowledge portfolio potentially contains a higher degree of new-to-the-firm technological or market knowledge (Cassiman and Veugelers Citation2006). In contrast, when most knowledge inputs comes from the focal firm, the knowledge portfolio will overlap largely with what the focal firm has already known, which means little is an opportunity for the focal firm to integrate new knowledge and potentially improve the NPD outcome.
When a focal firm can integrate novel knowledge from external types of organisations, product design quality potentially improves. The novel knowledge from types of external organisations could be either market-related, such as consumer demand, competitor moves, etc. or technology-related, such as new patents, and know-how (Yli-Renko, Autio, and Sapienza Citation2001; Yan and Azadegan Citation2017). This means that the focal firm has more options when searching for a best product design solution (Laursen and Salter Citation2006; Subramaniam and Venkatraman Citation2001). For instance, if the focal firm has knowledge about specific unmet customer demands, the new product could be designed to satisfy customers better. Alternatively, if a patented technology that could improve the technical performance of the product is available to the focal firm, product design could also improve.
In practice, multiple firms have dedicated specific resources to establishing knowledge exchange relationships with key suppliers, in order to foster innovation outcomes (Potter and Paulraj Citation2020; Wagner Citation2012). For example, Procter & Gamble was among the first to radically open up their R&D department to engage with suppliers and other business partners. The firm’s strategic shift to actively encouraging external knowledge inputs has improved R&D productivity by 60%, contributed to 50% of the new products on the market, and generated billions of dollars in sales (Ampe-N'DA et al. Citation2020). In a recent interview, Tim Cook stresses the importance of working with suppliers for their knowledge (Murayama and Regalado Citation2019), as their supplier Seiko Advance was responsible for developing colour schemas for different Apple product lines. Therefore, we hypothesise:
Hypothesis 1a. External input ratio is positively associated with product design quality.
Input concentration. A lower level of input concentration increases diversity of the available knowledge portfolio. From the inter-organisational NPD and knowledge management literature, we know that different types of organisations tend to differ in the types of relationships with the focal firm (Argote Citation2012; Yan and Azadegan Citation2017). Specifically, customers and suppliers, due to their strong ties with a focal firm, tend to have more common knowledge shared with the focal firm. As a result, the focal firm could more efficiently integrate knowledge, which however tends to be less novel, from customers and suppliers, as compared with knowledge inputs from other weakly connected organisations, such as consulting firms, competitors, universities, governments, and others. Again, customers and supplier, due to their different positions along a value chain, also differ in the types of knowledge they offer to a focal firm’s NPD project (Chien and Chen Citation2010). Customers tend to provide more market-related knowledge, such as unmet consumer needs, while suppliers tend to provide more supply-related knowledge, such as a new cost-cutting production technology (Feng, Sun, and Zhang Citation2010).
A lower level of input concentration suggests that knowledge is equally distributed across all participating organisations, instead of coming from a particular type of organisations. The resulting more diverse knowledge portfolio elevates the chance for the focal firm to integrate novel market or technology knowledge, which should contribute to improved product design quality (Reagans and McEvily Citation2003; Salomo, Keinschmidt, and De Brentani Citation2010). In practice, firms actively try to diversify their supplier pool. For example, Google has launched several initiatives over the years, such as the Google Small Business Supplier Diversity programme, with the goal to actively engage with unique small suppliers and acquire diverse knowledge inputs in order to improve its product and service offerings (Samaniego et al. Citation2017). Therefore, we hypothesise:
Hypothesis 1b. Input concentration is negatively associated with product design quality.
3.3. The moderating effects of technology interdependence
Technology interdependence refers to the degree of interdependence among the product and process technologies to be developed (Sosa, Eppinger, and Rowles Citation2004; Tatikonda and Rosenthal Citation2000b). A Higher levels of technology interdependence indicates that design changes in one part of the development task, i.e. one product module, could affect activities in another part of the development task, i.e. design of another module or the manufacturing process of the same module (Tatikonda and Rosenthal Citation2000b). Thus, high technology interdependence reflects a more integral design, increasing the complexity of the development task. For example, personal computers originally were introduced as all-in-one packages, where components were tightly integrated with a high level of technological interdependence (such as Intel’s MCS-4, the Kenback-1, the Apple II or the commodore PET) (Schilling Citation2000). However, a high level of technological interdependence increases coordination challenges during the development process and limits flexibility in end configurations. Therefore, modern computers are designed in a more modular way to reduce interdependence among modules or different stages of the development process.
The associated complexity in the product technological architecture reduces the focal firm’s ability to rely solely on its own knowledge base to figure out the best way to improve design quality. As a result, the need to integrate more external knowledge into the knowledge portfolio of a NPD project increases. A higher external input ratio could increase the novelty of the total knowledge portfolio because knowledge possessed by external partners are usually different from those possessed by the focal firm. This is helpful for the focal firm to challenge the status quo and facilitate ‘out-of-box’ thinking in order to find the optimal path in a tightly coupled, complex technology system for improving design quality. While integrating a large amount of external knowledge can be very costly, the benefits of the external knowledge are likely to outweigh the cost. Therefore, the positive effect of the external inputs ratio on design quality should be even greater when technology interdependence is high.
In contrast, when technology interdependence is low, the development task is modular and thus simpler, which reduces the overall need for novel knowledge from external types of organisations. For example, modular buildings generally consist of universal parts that are designed and manufactured in different factories and then shipped to a building site to be assembled into a variety of arrangements. In this case, technological interdependence of modular buildings is very low, which makes it less crucial for factories to integrate external knowledge to ensure design integrity. Understanding and integrating external knowledge in innovation projects with limited resources and high technological uncertainty is especially costly (Grant Citation1996). Given the limited benefits associated with external knowledge when technology interdependence is low, we could see fewer benefits, in terms of improving design quality, from a knowledge portfolio that is primarily composed of external knowledge. Therefore, we hypothesise that:
Hypothesis 2a. External input ratio is more positively associated with product design quality when technology interdependence is high.
In addition, we argue that technology interdependence should make more concentrated knowledge inputs less challenging for design quality. With a high level of input concentration, the knowledge portfolio is composed of knowledge concentrated within a few types of organisations, instead of equally dispersed among a large number of diverse types of organisations. Given that the nature and content of knowledge inputs usually vary among customers, suppliers, and other non-supply chain members of a focal firm, a high input concentration indicates a lower level of knowledge diversity. A less diverse knowledge portfolio is less costly for the focal firm to integrate (Grant and Baden Fuller Citation2004). This lower knowledge integration cost is especially crucial for allowing the focal firm to efficiently find the optimal path towards a better design in a technologically interdependent system (Singh Citation2008; Santoro, Bresciani, and Papa Citation2020). In other words, technology interdependence increases the efficiency-boosting effect of high input concentration to counteract its novelty-reducing effect. Therefore, low input concentration should be less damaging to design quality in a technologically interdependent NPD project.
In contrast, when technology interdependence is low, the path to the optimal design will usually be easier to find due to a lower level of design complexity (Danese and Filippini Citation2010). Consequently, the focal firm will have more slack resources left to integrate a diverse set of knowledge for improving design quality. Therefore, knowledge inputs that are more concentrated will hurt design quality more when technology interdependence is low (Wang, Chen, and Fang Citation2018; Fredrich, Bouncken, and Kraus Citation2019). Therefore, we hypothesise that:
Hypothesis 2b. Input concentration is less negatively associated with product design quality when technology interdependence is high.
3.4. Product design quality and product market performance
High product design quality is one driver of the market performance of the new product (Biedenbach and Müller Citation2012; Lawson, Krause, and Potter Citation2015). Effectively understanding and integrating external and internal knowledge, improves product design quality, characterised by good dimensional integrity, durability, functionality, manufacturability, and fits with customer needs, should be expected (Yan and Dooley Citation2013). Good product design has been shown to help the product gain market share, produce profits, and generate high return-over-investment, for the focal firm (Yan and Azadegan Citation2017). Therefore, we hypothesise:
Hypothesis 3. Product design quality is positively associated with product market performance.
In sum, we examine the effects of knowledge inputs on NPD project performance. In this section, we have developed arguments as to why and how knowledge inputs affect product design quality, which ultimately drives product market performance. Specifically, we look at the external input ratio and input concentration of these knowledge inputs. In addition, we explore technology interdependence as a contingency to our proposed relationships. The resulting research model is depicted in Figure .
4. Method
The objective of our study was to examine the effects of knowledge inputs on product design quality, which in turn affects product market performance. We also investigate potential moderation effects of technology interdependence on the relationships between external input ratio and input concentration, and product design quality. To test our hypotheses, we estimated a set of linear regressions using survey data of 210 NPD projects in Italy.
4.1. Data collection and sample description
In our sample selection, we specifically focused on the manufacturing industry while also sampling closely related industries, such as services, transportation, and trade. However, we excluded the general services and software industries, due to their distinctly different innovation processes and outcomes (von Hippel and von Krogh Citation2003). Accordingly, an initial list of potential respondents was retrieved from the AIDA – Bureau Van Dijk database, which holds records on public and private firms in Italy. Italy presents an interesting case to study European inter-organisational NPD activities (De Martino and Magnotti Citation2018; Hofstede Citation1984; Nakata and Sivakumar Citation1996). Highly customised products and knowledge-intensive innovation processes, characterise the Italian economy. Specifically, highly innovative clusters, such as the fashion industry in Northern Italy, drive NPD efforts by maintaining close relationships with suppliers and customers (Maltz Citation2012; Nassimbeni Citation2003).
The initial set included 994 potential respondents. The survey was hosted on the Qualtrics platform and participants were contacted via email. The online questionnaire introduced the context and objective of our research project. Participants later received the anonymised study results for benchmarking purposes. The questionnaire was carefully pre-tested (Ambulkar, Blackhurst, and Grawe Citation2015). The main objective of our pretest was to assure substantive validity of our measures and the overall survey design (Anderson and Gerbing Citation1991). We further made sure that the questions could be correctly understood and easily answered by the survey respondents. The questionnaire was translated into Italian and then back-translated into English (Brislin Citation1986). A group of supply chain managers from three large Italian firms was involved to increase clarity and avoid misinterpretations. Afterwards, a panel of three academics scholars from the operations management area reviewed the final draft once more and were asked to consider each item’s and measure’s relevance to the study objective.
Out of the 994 managers who were willing to participate, and after three follow-ups made via emails and phone calls, 251 respondents completed the survey by the end of 2014 (25.3% response rate). To ensure knowledgeable respondents, we selected managers with job titles and positions related to the NPD project, such as engineering, manufacturing, product development, project management, or purchasing. Participants also had to demonstrate adequate and up-to-date NPD experience by indicating that they have been actively involved in an inter-organisational NPD project during the last three years. Our sample covers a wide range of projects, ranging from foundational R&D initiatives to more specific product development and improvement. Foundational research is conducted to explore materials and systems to reduce waste and improve efficiency, such as creating new material compositions to improve vehicle safety. Other projects are concerned with developing antennas to improve mobile network coverage, testing filtration systems to reduce the pollution levels of cruise ships, and improving industrial air heat exchangers to limit energy consumption.
Additionally, we asked respondents to ‘ … consider a recent NPD project, finished within three years, where external organizations were involved in the development process’ when answering all the NPD-project related questions, to reduce the potential bias towards reporting on successful NPD projects only. We dropped 30 responses due to inadequate experience with the research topic; and another 11 due to extensive missing data. The final sample contained responses regarding 210 NPD projects. To reduce common method bias, we complemented the survey responses with secondary data (Craighead et al. Citation2011). Specifically, we utilise firm data on sales revenue, R&D intensity, and industry SIC codes, using both the AIDA – Bureau Van Dijk database and Thomson Reuters DataStream. We also calculate our main independent variables, rather than using latent constructs, which significantly reduces common method concerns (Wooldridge Citation2010).
In our final sample, the most common industries were Manufacturing (50.0%), such as machinery or appliances, closely related Services (16.67%) and Retail & Trade (10.5%). Our sample consists of both SMEs and larger firms. Our sample is comprised of 54% SMEs with less than 250 employees and 46% with more than 250. Annual sales revenue, for example, ranged from $0.6 million to $33.7 billion in 2016, with an average of $66.5 million. Taking Italian accounting practices into account, which tend to limit the percentage of firms reporting numerical R&D information in financial reports, we now also include other firm documents and notes to the firm balance sheet to provide a more representative measure of R&D intensity (Koh and Reeb Citation2015). The average R&D intensity in our sample is 2.4%. See Table for more detailed sample demographics. This wide range of industries, firm and project sizes ensures high levels of generalisability and reliability.
Table 2. Sample demographics.
4.2. Variables and measures
To test the relationships proposed in our conceptual model, we employ several measures using calculated measures from survey response scores, latent variables and archival firm data. Table shows our measures, including factor loadings, average variance extracted (AVE), construct reliability, and Cronbach’s Alphas for all latent variables.
Table 3. Variables and measures.
4.2.1. Independent variables
We calculate knowledge inputs based on four questions. Each question assesses the level of knowledge inputs from different types of potentially involved organisations: the focal firm, customers, suppliers, and other types of organisations. The item prompts the respondent to evaluate the inputs of several types of resources on a 7-point Likert scales, with ‘1’ indicating ‘low inputs’ and ‘7’ indicating ‘high inputs’. We specifically calculate our measures of knowledge inputs on one item. Calculating different measures from similar items is commonly done in the context of value creation (Scheer, Kumar, and Steenkamp Citation2003; Wagner, Eggert, and Lindemann Citation2010).
Specifically, we calculated external input ratio and input concentration according to their definitions in Table . External input ratio was operationalised as the fraction of knowledge inputs from all external types of organisations to the sum of external and internal (i.e. by the focal firm) knowledge inputs to the project. We calculated input concentration among all involved organisations to a NPD project as the standard deviation of knowledge input over the mean. If a type of organisation was not involved in a NPD project, knowledge inputs from that type of organisations were set to zero, to account for potentially different number of participants. As we are dealing with calculated measures, we also control for the overall level of knowledge inputs (see Section 4.2.2). See Table for the exact calculations.
4.2.2. Moderator and dependent variables
For our constructs technology interdependence, product design quality, and product market performance, we use established multi-item constructs utilising 7-point Likert scales, with ‘1’ indicating strong disagreement and ‘7’ indicating strong agreement. Technology interdependence, our moderator, refers to ‘the degree of interdependence between and among the product and process technologies to be developed’ (Tatikonda and Rosenthal Citation2000b). Technology interdependence is measured by a three-item scale used in Tatikonda and Rosenthal (Citation2000a). The three items measure interdependence among modules, stages and technologies by asking the extent to which a design change in one could influence that in another area.
In addition, we operationalised product design quality adopting a 3-item scale from Swink and Calantone (Citation2004). Following Yan and Azadegan (Citation2017), we measured product market performance as a latent construct using three items, which assesses the product’s influence on firm sales, return on investment, and profitability in comparison to competing products.
4.2.3. Control variables
To ensure validity and reliability of our results, we included a diverse set of control variables at the industry, firm and project level. We used archival firm data from Thompson Reuters to measure these variables. The industry context influences a firm’s ability to create and appropriate value from inter-organisational NPD practices (Lawson and Potter Citation2012; Yan and Wagner Citation2017). Different industries show varying degrees of innovation intensity, which could influence the NPD process (Subramaniam and Youndt Citation2005). We controlled for industry effects using two-digit SIC codes. We select Manufacturing as the base category, since it is the largest industry group (Wooldridge Citation2010).
At the firm level, we controlled for firm size and R&D intensity. The size of a firm could indicate its capabilities and resources available to manage knowledge in general (Leonard-Barton Citation1992). Larger firms are also more likely to possess superior project management and inter-organisational collaboration capabilities and experience. Suppliers might show more commitment, when working with a large firm, due to greater perceived power of bigger firms (Maloni and Benton Citation2000; Tang and Tomlin Citation2008). Large firms have additional means to market a product and facilitate its commercial success (Ketchen and Hult Citation2007). We operationalised firm size as the natural logarithm of the firm’s revenues from sales and services in US Dollar. We use US Dollar to ensure comparability with related studies. Other currencies were converted to US Dollar using the exchange rate of the reporting date. In addition, a firm’s innovation resources, indicated by R&D intensity, could affect the overall NPD process. Therefore, we also included the firm R&D intensity, defined as the ratio of expenditures on research and development to firm sales, as an additional control variable (Singh Citation2008).
At the project-level, as we evaluate calculated measures of knowledge, we also controlled for the average level of knowledge inputs to the NPD project, operationalised as the mean contribution by the focal firm, customers, suppliers, and other types of organisations. In addition, we controlled for inter-organisational innovation breadth, measured as the number of unique types of participants. A more diverse team, indicated by a large number of different types of organisations, such as suppliers, customers, universities, and consultants, might affect the knowledge management in an NPD context (Laursen and Salter Citation2006). The novelty of a product could also affect the types of inter-organisational interactions in the process (Ragatz, Handfield, and Scannell Citation1997). Product novelty is the degree to which a product fundamentally differs from a firm’s existing product offerings (Olson, Walker Jr, and Ruekert Citation1995). We operationalised product novelty as a three-item latent construct adapted from Biedenbach and Müller (Citation2012) and Subramaniam and Youndt (Citation2005), who measure the ‘newness’ of a product from the focal firm’s point of view.
4.3. Measurement assessment and data analysis
To ensure methodological rigour of our study, we tested for discriminant validity, content validity and construct reliability of our multi-item latent constructs. To test for overall construct validity, we first conducted a chi-square discriminant validity test (Bagozzi, Yi, and Phillips Citation1991; Garver and Mentzer Citation1999). We run a series of pairwise chi-square difference tests for all latent constructs, comparing an unconstrained CFA model with all latent constructs and one model with a fixed correlation between the two tested constructs. All construct pairs showed significant chi-squares, indicating discriminant validity. Since we conducted repeated comparisons, we used the Bonferroni-corrected p-values (Kroes and Ghosh Citation2010). Second, the square root of the AVE for each factor was also greater than the correlation of the factor with any other constructs (see Tables and ), which further supports the assumption of discriminant validity (Anderson and Gerbing Citation1988; Fornell and Larcker Citation1981). The AVE values of our constructs are greater than 0.6, indicating adequate convergent validity. To assert content validity, we relied on scales that are widely established in the operations and supply chain management literature.
We utilised AVE, construct reliability score, and Cronbach’s Alpha as our measures of construct reliability (Garver and Mentzer Citation1999). All latent constructs demonstrated Cronbach’s Alpha above 0.60, as recommended by Nunnally and Bernstein (Citation1994). AVE and construct reliability scores were higher than 0.70 and 0.50 respectively, indicating adequate construct reliability (Garver and Mentzer Citation1999). Table shows Cronbach’s Alpha, AVE, and construct reliability score as well as factor loadings for all our latent constructs.
To test for non-response and selection bias in our study, we used two different test procedures (Armstrong and Overton Citation1977; Wagner and Kemmerling Citation2010). First, we compared the first and last 30 survey responses. Results show no significant mean differences for our model items and variables (at p > 0.05). Second, 100 randomly selected sample firms were compared to 100 randomly selected non-responding firms. We conducted t-tests on sales revenue and R&D intensity and did not find significant differences (at p > 0.05). We concluded that non-response and selection bias was not a concern in our study.
To reduce potential common method bias, we use archival firm data, whenever possible, to complement survey responses. For instance, firm size, R&D intensity and industry are measured using archival firm data. Following Lindell and Whitney (Citation2001), we examined the correlation matrix. All variable correlations were low, except our two calculated measures external input ratio and input concentration, which is reasonable. Correlations suggest that common methods bias was not a major concern in our study (Bagozzi, Yi, and Phillips Citation1991; Hair et al. Citation2010). Table provides variable means, standard deviations, and correlations. Mean and standard deviation were calculated prior to scaling, correlations are on scaled variables as used in our regression models.
Table 4. Descriptive statistics and correlations.
We tested our hypotheses utilising linear regression analysis due to its statistical power in testing complex statistical models, with a relatively small sample (Cohen et al. Citation2013; De Beuckelaer and Wagner Citation2012). We apply confirmatory factor analysis to our measurement model of latent variables and use the resulting factor scores as the variables in our regression model (Rönkkö et al. Citation2016; Shah and Ward Citation2003). We assessed the Variance Inflation Factors (VIF) and found that all VIFs were well below three, as suggested by Hair et al. (Citation2010), which alleviates potential concerns about multi-collinearity biasing our results. The low VIFs also ease concerns regarding the correlation between our calculated IVs. Further, we did scale our variables to reduce potential collinearity and minimise the bias from different data sources and measurement scales. We estimated ordinary least squares (OLS) regression models.
5. Results
5.1. Regression results
The results for the structural relationships and hypotheses tests regarding the influence of knowledge inputs on product design quality and product market performance are summarised in Table and illustrated in Figure .
Table 5. Linear regression results.
First, we examine the effects of external input ratio and input concentration within the NPD project on product design quality. Model 1 in Table is the control model on product design quality. We find that product novelty negatively affects product design quality . It is conceivable that highly novel products are characterised by more uncertainties, which present more complex challenges, and are thus are inherently more difficult to design. Innovation breadth seems to foster product design quality, as more external project partners indicate a more diverse knowledge portfolio
. The overall level of knowledge contribution to the NPD project fosters design quality, as expected
. We also find that technology interdependence positively influences product design quality
.
Hypotheses H1a suggest a direct positive effects of external input ratio on product design quality. However, Model 2 in Table indicates a significant negative relationship . While we find the opposite effect to be significant, we have to reject our original Hypothesis H1a. We will explore this finding in our post-hoc analysis. In H1b, we propose a negative relationship, as high input concentration within a NPD project might affect knowledge processes and limit product design quality. Model 2 in Table confirms our Hypothesis H1b
.
Second, we investigate the moderation effects of technology interdependence. In Hypotheses H2a and H2b, we argue that technology interdependence attenuates the relationships between both external input ratio and input concentration, and product design quality. Model 3 in Table shows the interaction effects. We find that the effects of input ratio are positively moderated by technology interdependence, supporting H2a . Figure illustrates the interaction. To gain more nuanced insights on the moderation effect, we conduct a simple slopes analysis (Preacher, Curran, and Bauer Citation2003). Results suggest that for products characterised by low technology interdependence, higher external input ratio hurts the product design quality. Having access to widely dispersed and diverse knowledge does not significantly affect product design quality in cases of high technology interdependence.
Figure 3. The moderation effect of technology interdependence on external input ratio (Coefficients for slopes at ±1 SD in parentheses).
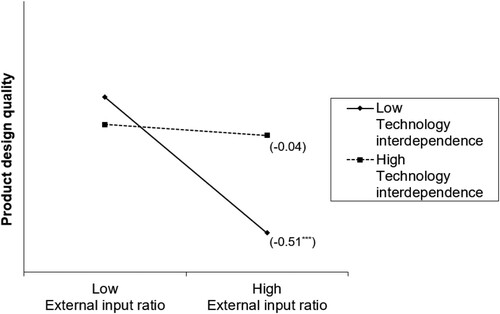
In addition, we also find support for H2b. Model 3 in Table suggests a positive moderation effect on input concentration . Figure illustrates the slopes for the interaction. High interdependence products are characterised by high complexity, which might impose additional challenges for firms to integrate knowledge from distinct sources. Thus, accessing concentrated knowledge might create less costly overhead for applying the knowledge to improve product design quality. However, the effect for high interdependent products is not significant. Design quality for products characterised by low technology interdependence is hurt significantly by higher input concentration, as lower complexity suggest a more seamless integration from a variety of knowledge sources.
Figure 4. The moderation effect of technology interdependence on input concentration (Coefficients for slopes at ±1 SD in parentheses).
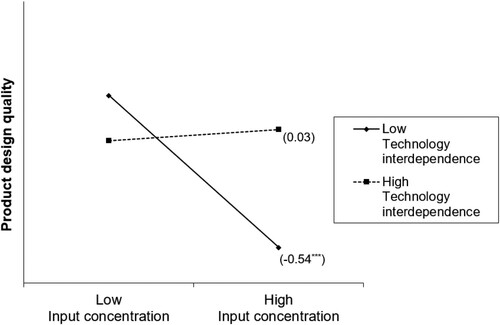
Third, in Hypothesis H3, we argue for a direct positive association of product design quality with product market performance. Model 4 in Table provides support for H3. This indicates that a firm’s capability to acquire valuable technical and market knowledge enables the firm to not only design higher quality products but also perform better in the market.
5.2. Post-hoc analysis
In Hypothesis H1a we argue for a positive effect of external input ratio on product design quality. However, results suggest a significant negative relationship. We conduct additional post hoc tests to bring further nuance to this finding and derive more detailed insights.
While we assume linear relationships, the literature suggests that knowledge integration processes are inherently complex and thus, these relationships might not necessarily be linear (Argote Citation2012; Yan and Azadegan Citation2017). Consequently, we further explore how our independent variables, external input ratio and input concentration, affect product design quality by testing for curvilinear relationships (Figure ).
Figure 5. Curvilinear effects of external input ratio on product design quality (unstandardised dependent and independent variables for visualisation purposes; we use average values for control variables).
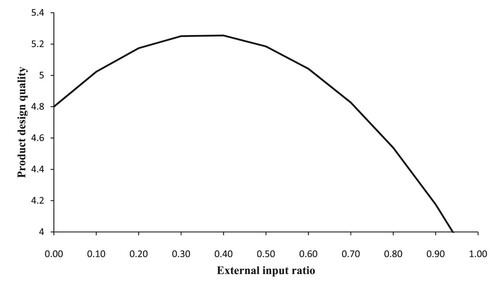
We run regression models adding quadratic terms for our independent variables and consider unstandardised coefficients to facilitate interpretation. We find indication of an inverse U-shaped relationship between external input ratio and design quality . The resulting inverse U-shape is illustrated in Figure . Intuitively, results suggest a ‘sweet spot’ around a 40% to 50% external input ratio, where accessing external knowledge can lead to significant improvements in product design quality. In contrast, we observe no significant curvilinear effect of input concentration on product design quality. Results are available upon request.
As a critical test procedure for the existence of an inverse U-shaped relationship between external input ratio and product design quality, we follow Lind and Mehlum (Citation2010). They argue that statistical significance of the quadratic term is not a sufficient test for curvilinear relationships and propose a more rigorous approach. The procedure is based on three criteria conditions: (1) significant quadratic terms, (2) the 90% confidence interval for the extreme point has to be within the range of the sample data, and (3) significant positive and negative slopes at the minimum and maximum of the sample data. Table presents the curvilinear analysis results following Lind and Mehlum (Citation2010). Again, we observe a significant quadratic term. The extremum point is estimated at 0.48 and we derive a Fieller 90% confidence interval (Fieller Citation1954), which is [0.313, 0.563] and sits well within our data range [0.125, 1.000], thus meeting the second criterion. The third criterion requires that the slope of the relationship at the lower bound of the sample data interval is significantly positive and the slope at the higher bound is significantly negative, creating the inverse U-shaped case. As seen in Table , both conditions are satisfied. Hence, there is a significant inverse U-shaped (hump-shaped) relationship over the full range of our sample data .
Table 6. Test for an inverse U-shaped relationship between external input ratio and product quality management.
5.3. Robustness checks
We include two robustness checks: (1) alternative variable measurements and (2) a multi-group structural equation modelling approach to retest the moderation effects of technology interdependence. Our robustness checks substantiate reliability and validity of our findings.
First, we check for robustness regarding our latent variables. Initially, we use the factor scores of our latent variables derived by maximum likelihood factor analysis in our regression models. However, there are other construct scoring approaches potentially leading to substantially better results in terms of reliability (Hair et al. Citation2010; Rönkkö et al. Citation2016). As alternatives, we consider average and sum item scores. Both sets of regression models confirm our previous results and indicate robustness of our study. Detailed estimates are available upon request.
Depending on its operationalisation, the measure for input concentration might not adequately account for the number of project partners. In our main analysis, we set the contribution of non-involved parties to zero. Alternatively, we calculate the standard deviation only using the involved partners and adding the inverse number of project partners. After including this measure of input concentration in the regression model, we are able to confirm all the hypothesis-testing findings. In addition, we also test alternative operationalisation of the non-latent variables. For example, we use the number of firm employees as a proxy for firm size and control for sales growth, as younger and expanding firms might have different innovation processes than bigger incumbent players. All our results are confirmed.
Second, we run structural equation models (SEM) with maximum likelihood estimation. We estimate our SEM using Stata 15. SEM is a second generation multivariate method allowing for the specification of a measurement model including multiple indicators for latent unobserved variables as well as a structural model defining relationships between multiple observed and latent variables (Bagozzi and Yi Citation1988; Fornell and Larcker Citation1981). As SEM can create issues when examining multiple interaction terms (Sardeshmukh and Vandenberg Citation2017), we use regression models in our main analysis. However, as a robustness check, we split our sample at the median level of technology interdependence, to test the moderation effects (Hair et al. Citation2010). Our results are robust to the multi-group SEM. Detailed results are available in Table in the Appendix.
6. Discussion and conclusion
In this study, we examine how the knowledge input characteristics among collaborating types of external organisations affects inter-organisational NPD project outcomes. Interestingly, we find that a high external input ratio has a negative effect on product design quality, an effect that is attenuated by technology interdependence of the new product. An interesting inverse U-shaped relationship between external input ratio on design quality is also found in our post-hoc analysis. We also find that high knowledge concentration could hurt product design quality, especially in a product setting with low technology interdependence. Overall, our results are indicative of the key role of knowledge inputs in explaining the product design outcomes in inter-organisational NPD projects. We discuss the theoretical and managerial implications of these findings and our post-hoc analysis below.
6.1. Theoretical implications
Theoretically, we contribute to the inter-organisational NPD literature by studying the performance implications of a rarely examined influencer of project performance: the characteristics of knowledge inputs. The strategic alliance and NPD literature have widely recognised the importance of understanding knowledge flows among partnering organisation types in a joint NPD project (Grant and Baden Fuller Citation2004; Lawson and Potter Citation2012; Sarin and McDermott Citation2003). Most often, the literature generally focuses on inter-organisational governing structures and team processes that encourage knowledge acquisition, integration and utilisation (Bstieler Citation2006; Yan et al. Citation2020). However, very rarely has the literature examined the knowledge inputs by different NPD project partners. To contribute to this stream of work, this study discovered significant influences of two knowledge input characteristics: external input ratio and input concentration.
Specifically, we show that when the knowledge portfolio is largely composed of external knowledge, product design quality also could be negatively affected. We see one main explanation for our findings. Although a higher external input ratio might help by creating greater access to external knowledge, the focal firm could also face challenges of integrating a higher percentage of external knowledge for improving the final product design (Grant Citation1996; von Briel, Schneider, and Lowry Citation2018). Knowledge integration will be more challenging when the knowledge portfolio is mainly made up of externally originated knowledge. Compared to internally created knowledge, externally accessed knowledge usually overlaps less with a focal firm’s business domain and product design needs. As a result, it is more challenging to integrate external knowledge with internal knowledge to facilitate NPD project success (Easterby Smith, Lyles, and Tsang Citation2008; Modi and Mabert Citation2007). In contrast, when the knowledge portfolio is primarily composed of a focal firm’s own knowledge, it is easier to integrate the knowledge, and improve the product design due to a shorter distance between the knowledge portfolio and the product development needs.
To better understand this counterintuitive negative influence of external input ratio on design quality, we conducted post hoc analysis and identified an inverted U-shape relationship between external input ratio and design quality. Our findings provide evidence for a ‘too much of a good thing’ effect (Busse, Mahlendorf, and Bode Citation2016). In other words, an intermediary level of external input ratio seems to work best for the focal firm to improve design quality. When the knowledge portfolio is composed of a balanced set of internal and external knowledge, the focal firm could benefit from accessing novel external knowledge without being burdened too much in integrating them with internal knowledge. A significant level of internal knowledge gives the focal firm better control on how the total knowledge portfolio contributes to the improvement of design quality. Therefore, although the overall effect of the external input ratio on design quality is negative, we are able to show that a low to medium level of external input ratio could still help. Overall, these interesting findings encourage future research to examine the interaction between internal and external knowledge in affecting NPD outcomes.
We also add to the literature by examining the effects of another overlooked predictor of NPD project outcomes, input concentration. As expected, we found that input concentration affects product design quality negatively. While a highly dispersed knowledge portfolio creates significant knowledge integration challenges, it provides the focal firm with a more diverse set of knowledge. Knowledge concentrated within few types of organisations also results in a higher level of focal firm dependency on that partner, creating additional challenges for knowledge integration due to the asymmetric power between the main knowledge contributor and the focal firm. These interesting findings should encourage future studies to investigate whether a higher level of concentration of other types of NPD project resources (i.e. financial, human, physical) also negatively affect team processes and project outcomes.
Further, this study provides important theoretical insights to the inter-organisational innovation literature by identifying the moderating role of technology interdependence in a knowledge intensive task environment. Technology interdependence is a product characteristic that introduces uncertainty and complexity to a NPD project. In NPD projects where technology interdependence is low, we found that both external input ratio and input concentration hurt design quality significantly more. Products with high technology interdependence often require more complex design solutions as well as more design-manufacturing interactions. A high external input ratio indicates a higher chance for the focal firm to access distant, external knowledge possessed by partnering organisation types. These distant knowledge, though challenging to be integrated, should be crucial for the focal firm to overcome the design challenges created by a complex, interdependent product structure (Argote Citation2012; Grant and Baden Fuller Citation2004; Lawson and Potter Citation2012). This finding sets up a stage for future studies about product architectures, modular vs integral, to take a knowledge acquisition and integration view and examine the knowledge management implications posed by product architecture.
We further show that the negative effect of input concentration on design quality is accentuated when technology interdependence is low. The significant design challenges associated with technology interdependence seem to pose enough coordination challenges within a NPD project to the extent that any further coordination need is hard to be met, which could make a less concentrated knowledge portfolio challenging to manage. As the literature suggests, close relationships are easier to maintain with fewer contributing partners, allowing for flexibility and agility when applying knowledge to develop the best design solution (Choo, Chandrasekaran, and Chinaprayoon Citation2020; Slot, Wuyts, and Geyskens Citation2020). Therefore, low knowledge concentration increases the challenge to keep close relationships with external partners, thus increasing knowledge integration challenges, especially when there are a lot of moving parts in the product design due to a high level of technology interdependence. The moderation effect of technology interdependence on the negative effect of input concentration on design quality provides an indication for a potential dark side of a highly dispersed knowledge portfolio in inter-organisational NPD projects. In general, we add to the literature by showing the importance of understanding product technological architectures for managing knowledge flows in inter-organisational NPD projects by establishing technology interdependence as a contingency factor that moderates effects of knowledge input characteristics on design quality. Future studies should explore other contingencies on performance implications the knowledge flows in inter-organisational NPD project.
Overall, our findings advance the inter-organisational collaboration literature that examines performance implications of resource distributions among partners by providing project-level evidence about the crucial roles of knowledge inputs. A few strategic management studies have provided evidence about the influences of resource diversification and concentration on firm-level or business-unit level innovation performance (Chen and Chu Citation2012; Cui and O'Connor Citation2012; Bos, Faems, and Noseleit Citation2017). Since knowledge is a critical type of innovation resources, the innovation literature has also shown the importance of considering diversity in knowledge, indicated by partner type diversity, innovation objective diversity, etc. could affect firm innovation performance (Leiponen and Helfat Citation2011; Wuyts and Dutta Citation2014; Hagedoorn, Lokshin, and Zobel Citation2018; Zhang, Yuan, and Wang Citation2019). The supply chain management literature has also shown that the concentration of sourcing resources among a few suppliers could benefit the absorption of external knowledge in certain situations (Moeen, Somaya, and Mahoney Citation2013). The literature suggests the nature of the total knowledge available to a focal firm in its inter-organisational network, i.e. diversity, distance, etc. could affect the extent to which the focal firm could effective absorb and integrate the knowledge (Vasudeva and Anand Citation2011). Adding to these firm-level insights, our inter-organisational project-level empirical analyses show that characteristics of knowledge inputs could also affect product design quality, an effect that is contingent on the level of technological interdependence. By showing the contrasting effects of external inputs ratio and total inputs concentration on design quality, our study opens up a new field for future studies to examine project-level performance implications of other types of resources inputs, i.e. tangible assets, financial investments, and others.
6.2. Managerial implications
Successful NPD projects are a key means for firm innovation. However, NPD managers are facing a plethora of complex decisions, especially when external organisation types are involved. Managers are responsible for managing knowledge flows among the collaborating types of organisation in order to facilitate successful NPD projects (Im, Rai, and Lambert Citation2019; Lawson and Potter Citation2012). Managers often struggle with creating, acquiring and internalising technical and market knowledge from outside partners, such as suppliers, R&D institutes or universities, in a most efficient and effective way. The findings in our study mainly concern the underlying knowledge input tradeoffs. While our study is set on the project level, our results still provide insights to guide higher-level managerial decision-making, e.g. partner selection, in the NPD context.
Specifically, our results provide insights to managers concerned with NPD partner selection decisions and managing knowledge inputs among collaborating organisations. When following an open innovation strategy and opening the NPD process to external types of organisations, the focal firm needs to select those partners with complementary and valuable innovation resources, among which intangible knowledge is an important one. Instead of sticking to a ‘more is better’ mindset when soliciting knowledge inputs from each partner type, NPD managers of the focal firm should consider the different performance implications of external input ratio and input concentration.
Based on our findings, we make three practical recommendations. First, even though a knowledge portfolio that is largely composed of external knowledge creates many learning opportunities, the challenge of accessing and integrating the external knowledge might limit or even cancel out the learning benefits. Based on our post-hoc analysis, we recommend an intermediate level of external input ratio, which seems to most improve the focal firm’s NPD outcomes. Achieving the appropriate level of external knowledge involves prospective supplier selection. Remembering the introductory example, Volkswagen Group’s Artemis project successfully balanced knowledge inputs by internal and external project partners based on current knowledge needs. We advise managers to examine carefully what potential suppliers can add to a project. Building long-term supplier relationships can also help with ensuring consistent knowledge inputs. We further suggest managing and maintaining a solid internal knowledge base, enabling a firm to balance out internal and external knowledge in a NPD project.
Second, given the complex tradeoffs in the context of external knowledge inputs, we emphasise the importance of building and maintaining a solid internal knowledge base. For example, without a solid internal knowledge base, the focal firm might be overwhelmed by the amount of external knowledge, i.e. the dark side of a high external input ratio, which could cause knowledge integration to be costly or even unfeasible. We advise an open innovating firm to develop its own core knowledge base before opening up the innovation process to outside partners.
Third, when selecting external partners, the focal firm should also manage the concentration of knowledge inputs among few types of organisations. For example, a less concentrated (more dispersed) knowledge portfolio creates significant knowledge integration challenges, as the added diversity may complicate the knowledge management processes. Only when technology interdependence is low, which suggests a lower information processing need, managers could consider sourcing knowledge more broadly from external partners. In contrast, for products with high technology interdependence, stronger ties with a few types of external organisations (i.e. high knowledge concentration) could be crucial for the focal firm to discover the best design.
Finally, we also advise NPD managers to consider product architecture when making project partner selection decisions. In many firms, engineering is concerned with designing product architectures. Our results suggest that these architecture decisions affect the types of knowledge needed and determine the optimal configuration for knowledge inputs of a NPD project. During the Artemis project, Audi always had to select technology partners to complement the knowledge within the complex technology architecture. However, partner selection is often a (strategic) management decision. Communication and collaboration between management and the project engineering team might be insufficient or non-existent, a problem widely discussed in project management practice (Laufer et al. Citation2015; Pedersen and Ritter Citation2017). We advise managers to engage with the engineering team and understand specific project needs and characteristics to make more informed decisions on inter-organisational NPD project partner selection.
6.3. Limitations and conclusion
This study has some limitations that need to be taken into account when interpreting its findings and conducting future research. First, we only focus on product design quality as our primary project outcome. Despite the fact that product design quality is a highly important and relevant outcome from a knowledge acquisition and integration view in an inter-organisational NPD project, there exist other important outcomes, such as time-to-market performance. We encourage future studies to extend our framework to other project outcome indicators. Second, the study is based on data from Italian firms. Italy presents an interesting case to study inter-organisational NPD activities, given its highly innovative clusters characterised by close relationships with suppliers and customers. While this premise is likely to have provided valuable insights, it also limits the generalisability of the study. Therefore, future research should also study the effects of knowledge inputs in different cultural contexts, including emerging markets. Third, knowledge is inherently difficult to measure. We chose to employ perceptual scales for the knowledge inputs. Specifically, we derive calculated variables based on these perceptual scales. While we assume that our calculated variables are still valid even based on perceptual measures, future research is encouraged to employ alternative measures of knowledge inputs. Fourth, we focus on design quality as the primary project performance measure for several reasons. Product design quality is directly related to the absorption and integration of various streams of knowledge inputs in the NPD process and is also tightly connected with technology interdependence. Given our focus on understanding how knowledge inputs affect NPD project performance, we believe that our variable is appropriate. However, we encourage future studies to explore the effects of knowledge inputs on alternative project performance measures, such as development process efficiency, product market performance, and others. Finally, when investigating the effects of knowledge contributions, we did not consider the implications of different types of organisations, i.e. those with more distant knowledge vs those with closer knowledge to the knowledge base of the focal firm. While Yan and Azadegan (Citation2017) show that there is no difference between the supply chain members and non-supply-chain sources’ influences on product novelty, it is still quite possible that knowledge distance between the focal firm and innovation partners might affect NPD outcomes. Therefore, we encourage future studies to take the type of organisation into account or even directly measure knowledge distance when studying inter-organisational NPD projects. Finally, we decided to examine project-level factors, as fewer studies explore inter-organisational knowledge flows on the project level. However, this focus could limit our attention to other important firm-level outcomes that are potentially affected by the focal NPD project. These outcomes and effects include inter-project knowledge flows, knowledge partner relationships and selection processes. We encourage future research to take on different perspectives on the role of knowledge inputs in NPD projects.
In conclusion, this study investigates a rarely examined influencer of inter-organisational NPD project performance: the characteristics of knowledge inputs. We focus on the ratio between external and internal knowledge inputs and the concentration of knowledge inputs between all types of collaborating organisations. Our results show how the characteristics of knowledge inflows affect NPD project success. Understanding and realising the performance benefits associated with different knowledge input characteristics, NPD managers could make more informed decisions when select innovation partners to create a knowledge portfolio that matches the specific project goals.
Disclosure statement
No potential conflict of interest was reported by the author(s).
Additional information
Notes on contributors
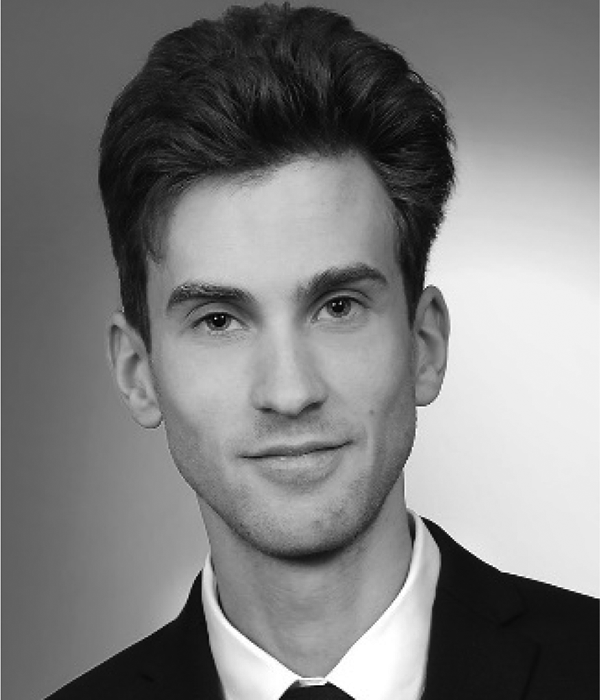
Christoph G. Schmidt
Christoph G. Schmidt is a postdoctoral researcher at the Chair of Logistics Management at the Swiss Federal Institute of Technology Zurich (ETH Zurich), Switzerland. His research interests in operations and supply chain management include supply chain risks and disruptions, sustainable practices and performance along the supply chain, and the intersection of social media technologies and supply chain management. His work has appeared in the Journal of Operations Management, Journal of Supply Chain Management, Journal of Business Logistics, Journal of Purchasing & Supply Management, and other journals.
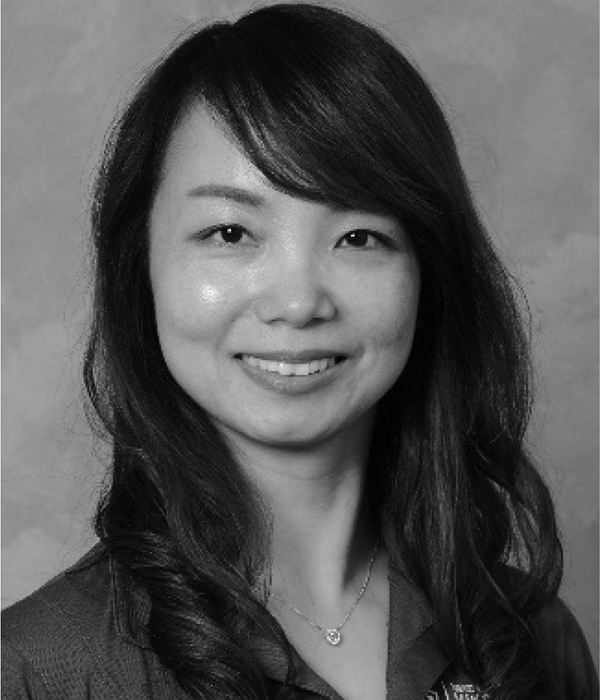
Tingting Yan
Tingting Yan is Professor, holds Charles H. Gershenson Distinguished Faculty Fellow at Wayne State University, and is a Co-Editor-in-Chief for Journal of Supply Chain Management. Current research interests include supply network, supply innovation, new product development, servitisation and behavioural operations management. Her work has been published in supply chain management journals, such as Journal of Operations Management, Decision Sciences, Journal of Supply Chain Management.
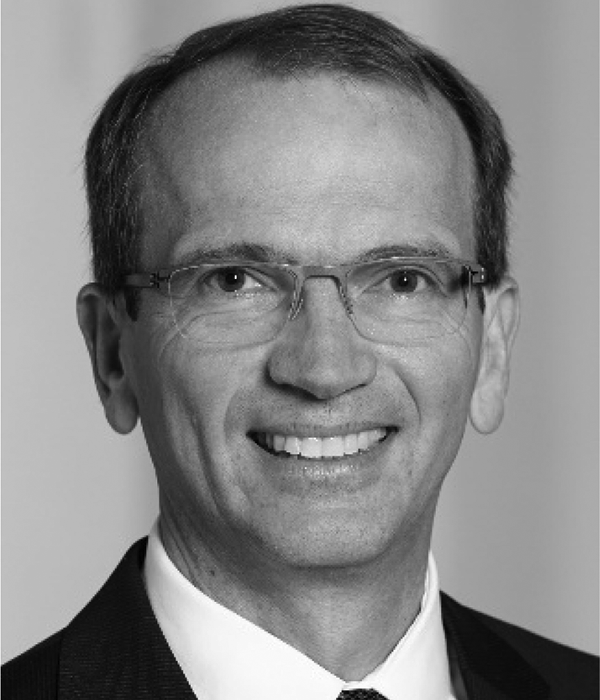
Stephan M. Wagner
Stephan M. Wagner is Professor, holds the Chair of Logistics Management, and is Faculty Director of the HumOSCM Lab at the Swiss Federal Institute of Technology Zurich (ETH Zurich). Current research interests include the management of startups as suppliers, supplier innovation, digitalisation, supply chain sustainability, and humanitarian operations and supply chain management. His work has been published in management journals, such as the Academy of Management Journal, Journal of Management, or Journal of Business Research, as well as operations management journals, such as Journal of Operations Management, Decision Sciences, Journal of Supply Chain Management, or Journal of Business Logistics.
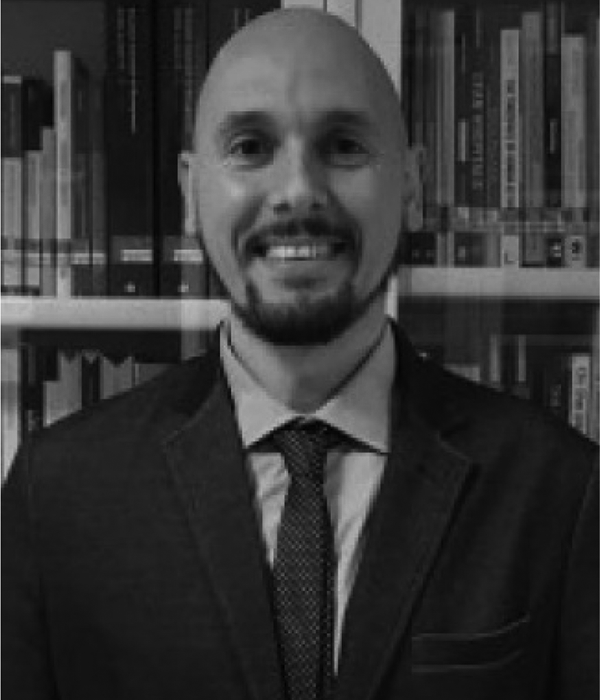
Lorenzo Lucianetti
Lorenzo Lucianetti is Professor and the Director of Undergraduate Studies in Economics and Management at the University of Chieti and Pescara. His research interests include performance measurement systems, organisational behaviour, supply chain management, corporate social responsibilities and ethical decision-making. His research has appeared in management journals, such as the Academy of Management Journal, Journal of Applied Psychology, or Journal of Management Studies, as well as operations management journals, such as Journal of Operations Management, Decision Sciences, or International Journal of Operations and Production Management.
References
- Ambulkar, S., J. Blackhurst, and S. Grawe. 2015. “Firm’s Resilience to Supply Chain Disruptions: Scale Development and Empirical Examination.” Journal of Operations Management 33–34 (2): 111–122.
- Ampe-N'DA, L. D., B. A. Payne, R. I. Spake, S. Sharpe, and A. Arora. 2020. “Buyer-Supplier Relationships: Role of Collaboration, Sustainability, and Technology.” In Sustainable Innovation, edited by A. S. Arora, S. Bacouel-Jentjens, M. Sepehri, and A. Arora, 47–58. Cham: Palgrave Pivot.
- Anderson, J. C., and D. W. Gerbing. 1988. “Structural Equation Modeling in Practice: A Review and Recommended Two-Step Approach.” Psychological Bulletin 103 (3): 411–429.
- Anderson, J. C., and D. W. Gerbing. 1991. “Predicting the Performance of Measures in a Confirmatory Factor Analysis with a Pretest Assessment of Their Substantive Validities.” Journal of Applied Psychology 76 (5): 732–740.
- Argote, L. 2012. Organizational Learning: Creating, Retaining and Transferring Knowledge. New York: Springer.
- Armstrong, J. S., and T. S. Overton. 1977. “Estimating Nonresponse Bias in Mail Surveys.” Journal of Marketing Research 14 (3): 396–402.
- Bagozzi, R. P., and Y. Yi. 1988. “On the Evaluation of Structural Equation Models.” Journal of the Academy of Marketing Science 16 (1): 74–94.
- Bagozzi, R. P., Y. Yi, and L. W. Phillips. 1991. “Assessing Construct Validity in Organizational Research.” Administrative Science Quarterly 36 (3): 421–458.
- Becker, M. C., and F. Zirpoli. 2003. “Organizing new Product Development: Knowledge Hollowing-Out and Knowledge Integration – The FIAT Auto Case.” International Journal of Operations & Production Management 23 (9): 1033–1061.
- Biedenbach, T., and R. Müller. 2012. “Absorptive, Innovative and Adaptive Capabilities and Their Impact on Project and Project Portfolio Performance.” International Journal of Project Management 30 (5): 621–635.
- Bolumole, Y. A., R. J. Calantone, C. A. Di Benedetto, and S. A. Melnyk. 2015. “New Product Development in New Ventures: The Quest for Resources.” International Journal of Production Research 53 (8): 2506–2523.
- Bos, B., D. Faems, and F. Noseleit. 2017. “Alliance Concentration in Multinational Companies: Examining Alliance Portfolios, Firm Structure, and Firm Performance.” Strategic Management Journal 38 (11): 2298–2309.
- Boutellier, R., and S. M. Wagner. 2003. “Sourcing Concepts: Matching Product Architecture, Task Interface, Supplier Competence and Supplier Relationship.” In Business Engineering, edited by H. Österle and R. Winter, 223–248. Berlin: Springer.
- Brislin, R. W. 1986. “The Wording and Translation of Research Instruments.” In Field Methods in Cross-Cultural Research, edited by W. J. Lonner and J. W. Berry, 137–164. Beverley Hills, CA: Sage.
- Bstieler, L. 2005. “The Moderating Effect of Environmental Uncertainty on New Product Development and Time Efficiency.” Journal of Product Innovation Management 22 (3): 267–284.
- Bstieler, L. 2006. “Trust Formation in Collaborative New Product Development.” Journal of Product Innovation Management 23 (1): 56–72.
- Busse, C., M. D. Mahlendorf, and C. Bode. 2016. “The ABC for Studying the Too-Much-of-a-Good-Thing Effect: A Competitive Mediation Framework Linking Antecedents, Benefits, and Costs.” Organizational Research Methods 19 (1): 131–153.
- Cao, M., and Q. Zhang. 2011. “Supply Chain Collaboration: Impact on Collaborative Advantage and Firm Performance.” Journal of Operations Management 29 (3): 163–180.
- Capaldo, A., and A. M. Petruzzelli. 2014. “Partner Geographic and Organizational Proximity and the Innovative Performance of Knowledge-Creating Alliances.” European Management Review 11 (1): 63–84.
- Carlo, J. L., K. Lyytinen, and G. M. Rose. 2012. “A Knowledge-Based Model of Radical Innovation in Small Software Firms.” MIS Quarterly 36 (3): 865–895.
- Cassiman, B., and R. Veugelers. 2006. “In Search of Complementarity in Innovation Strategy: Internal R&D and External Knowledge Acquisition.” Management Science 52 (1): 68–82.
- Chen, C.-N., and W. Chu. 2012. “Diversification, Resource Concentration, and Business Group Performance: Evidence from Taiwan.” Asia Pacific Journal of Management 29 (4): 1045–1061.
- Chen, J., R. R. Reilly, and G. S. Lynn. 2005. “The Impacts of Speed-to-Market on New Product Success: The Moderating Effects of Uncertainty.” IEEE Transactions on Engineering Management 52 (2): 199–212.
- Chesbrough, H. W. 2003. “A Better Way to Innovate.” Harvard Business Review 81 (7): 3–11.
- Chesbrough, H., and S. Brunswicker. 2014. “A Fad or a Phenomenon? The Adoption of Open Innovation Practices in Large Firms.” Research-Technology Management 57 (2): 16–25.
- Chien, S.-H., and J. Chen. 2010. “Supplier Involvement and Customer Involvement Effect on New Product Development Success in the Financial Service Industry.” Service Industries Journal 30 (2): 185–201.
- Choo, A. S., A. Chandrasekaran, and C. Chinaprayoon. 2020. “The Role of Domestic and Foreign Knowledge Inflows on the Relationship Between R&D Portfolio Mix and Innovation Outcomes: An Empirical Study of Manufacturing Firms in an Emerging Economy.” Decision Sciences 51 (2): 349–394.
- Cohen, J., P. Cohen, S. G. West, and L. S. Aiken. 2013. Applied Multiple Regression/Correlation Analysis for the Behavioral Sciences. Hillsdale, NJ: Routledge.
- Craighead, C. W., D. J. Ketchen, K. S. Dunn, and G. T. M. Hult. 2011. “Addressing Common Method Variance: Guidelines for Survey Research on Information Technology, Operations, and Supply Chain Management.” IEEE Transactions on Engineering Management 58 (3): 578–588.
- Cui, A. S., and G. O'Connor. 2012. “Alliance Portfolio Resource Diversity and Firm Innovation.” Journal of Marketing 76 (4): 24–43.
- Danese, P., and R. Filippini. 2010. “Modularity and the Impact on New Product Development Time Performance.” International Journal of Operations & Production Management 30 (11): 1191–1209.
- De Beuckelaer, A., and S. M. Wagner. 2012. “Small Sample Surveys: Increasing Rigor in Supply Chain Management Research.” International Journal of Physical Distribution & Logistics Management 42 (7): 615–639.
- De Jong, M., N. Marston, and E. Roth. 2015. “The Eight Essentials of Innovation.” McKinsey Quarterly 2: 1–12.
- De Martino, M., and F. Magnotti. 2018. “The Innovation Capacity of Small Food Firms in Italy.” European Journal of Innovation Management 21 (3): 362–383.
- Easterby Smith, M., M. A. Lyles, and E. W. Tsang. 2008. “Inter-Organizational Knowledge Transfer: Current Themes and Future Prospects.” Journal of Management Studies 45 (4): 677–690.
- Ettlie, J. E., and P. A. Pavlou. 2006. “Technology-Based New Product Development Partnerships.” Decision Sciences 37 (2): 117–147.
- Fang, E. 2011. “The Effect of Strategic Alliance Knowledge Complementarity on New Product Innovativeness in China.” Organization Science 22 (1): 158–172.
- Feng, T., L. Sun, and Y. Zhang. 2010. “The Effects of Customer and Supplier Involvement on Competitive Advantage: An Empirical Study in China.” Industrial Marketing Management 39 (8): 1384–1394.
- Fieller, E. C. 1954. “Some Problems in Interval Estimation.” Journal of the Royal Statistical Society: Series B (Methodological) 16 (2): 175–185.
- Fine, C. H. 2000. “Clockspeed-based Strategies for Supply Chain Design.” Production and Operations Management 9 (3): 213–221.
- Fornell, C., and D. F. Larcker. 1981. “Evaluating Structural Equation Models with Unobservable Variables and Measurement Error.” Journal of Marketing Research 18 (1): 39–50.
- Frankort, H. T. 2016. “When Does Knowledge Acquisition in R&D Alliances Increase New Product Development? The Moderating Roles of Technological Relatedness and Product-Market Competition.” Research Policy 45 (1): 291–302.
- Fredrich, V., R. B. Bouncken, and S. Kraus. 2019. “The Race Is on: Configurations of Absorptive Capacity, Interdependence and Slack Resources for Interorganizational Learning in Coopetition Alliances.” Journal of Business Research 101: 862–868.
- Garcia, R., R. Calantone, and R. Levine. 2003. “The Role of Knowledge in Resource Allocation to Exploration Versus Exploitation in Technologically Oriented Organizations.” Decision Sciences 34 (2): 323–349.
- Garver, M. S., and J. T. Mentzer. 1999. “Logistics Research Methods: Employing Structural Equation Modeling to Test for Construct Validity.” Journal of Business Logistics 20 (1): 33–57.
- Grand, S., G. Von Krogh, D. Leonard, and W. Swap. 2004. “Resource Allocation Beyond Firm Boundaries: A Multi-Level Model for Open Source Innovation.” Long Range Planning 37 (6): 591–610.
- Grant, R. M. 1996. “Toward a Knowledge-Based Theory of the Firm.” Strategic Management Journal 17 (2): 109–122.
- Grant, R. M., and C. Baden Fuller. 2004. “A Knowledge Accessing Theory of Strategic Alliances.” Journal of Management Studies 41 (1): 61–84.
- Hagedoorn, J., B. Lokshin, and A. K. Zobel. 2018. “Partner Type Diversity in Alliance Portfolios: Multiple Dimensions, Boundary Conditions and Firm Innovation Performance.” Journal of Management Studies 55 (5): 809–836.
- Hair, J. F., W. C. Black, B. J. Babin, R. E. Anderson, and R. L. Tatham. 2010. Multivariate Data Analysis. Upper Saddle River, NJ: Pearson Prentice Hall.
- Hofstede, G. 1984. Culture’s Consequences: International Differences in Work-Related Values. Newbury Park, CA: Sage Publications.
- Im, G., A. Rai, and L. S. Lambert. 2019. “Governance and Resource-Sharing Ambidexterity for Generating Relationship Benefits in Supply Chain Collaborations.” Decision Sciences 50 (4): 656–693.
- Jayaram, J. 2008. “Supplier Involvement in New Product Development Projects: Dimensionality and Contingency Effects.” International Journal of Production Research 46 (13): 3717–3735.
- Kach, A., A. Azadegan, and K. J. Dooley. 2012. “Analyzing the Successful Development of a High-Novelty Innovation Project Under a Time-Pressured Schedule.” R&D Management 42 (5): 377–400.
- Kahn, K. B., G. Barczak, J. Nicholas, A. Ledwith, and H. Perks. 2012. “An Examination of New Product Development Best Practice.” Journal of Product Innovation Management 29 (2): 180–192.
- Ketchen, Jr., D. J., and G. T. M. Hult. 2007. “Bridging Organization Theory and Supply Chain Management: The Case of Best Value Supply Chains.” Journal of Operations Management 25 (2): 573–580.
- Klingebiel, R., and C. Rammer. 2014. “Resource Allocation Strategy for Innovation Portfolio Management.” Strategic Management Journal 35 (2): 246–268.
- Kogut, B., and U. Zander. 1992. “Knowledge of the Firm, Combinative Capabilities, and the Replication of Technology.” Organization Science 3 (3): 383–397.
- Koh, P. S., and D. M. Reeb. 2015. “Missing R&D.” Journal of Accounting and Economics 60 (1): 73–94.
- Kroes, J. R., and S. Ghosh. 2010. “Outsourcing Congruence with Competitive Priorities: Impact on Supply Chain and Firm Performance.” Journal of Operations Management 28 (2): 124–143.
- Laufer, A., E. J. Hoffman, J. S. Russell, and W. S. Cameron. 2015. “What Successful Project Managers Do.” MIT Sloan Management Review 56 (3): 43–55.
- Laursen, K., and A. Salter. 2006. “Open for Innovation: The Role of Openness in Explaining Innovation Performance among UK Manufacturing Firms.” Strategic Management Journal 27 (2): 131–150.
- Lawson, B., D. Krause, and A. Potter. 2015. “Improving Supplier New Product Development Performance: The Role of Supplier Development.” Journal of Product Innovation Management 32 (5): 777–792.
- Lawson, B., K. J. Petersen, P. D. Cousins, and R. B. Handfield. 2009. “Knowledge Sharing in Interorganizational Product Development Teams: The Effect of Formal and Informal Socialization Mechanisms.” Journal of Product Innovation Management 26 (2): 156–172.
- Lawson, B., and A. Potter. 2012. “Determinants of Knowledge Transfer in Inter-Firm New Product Development Projects.” International Journal of Operations & Production Management 32 (10): 1228–1247.
- Leiponen, A., and C. E. Helfat. 2011. “Location, Decentralization, and Knowledge Sources for Innovation.” Organization Science 22 (3): 641–658.
- Leonard-Barton, D. 1992. “Core Capabilities and Core Rigidities: A Paradox in Managing New Product Development.” Strategic Management Journal 13 (1): 111–125.
- Lettice, F., N. Roth, and I. Forstenlechner. 2006. “Measuring Knowledge in the New Product Development Process.” International Journal of Productivity and Performance Management 55 (3): 217–241.
- Lind, J. T., and H. Mehlum. 2010. “With or Without U? The Appropriate Test for a U-Shaped Relationship.” Oxford Bulletin of Economics and Statistics 72 (1): 109–118.
- Lindell, M. K., and D. J. Whitney. 2001. “Accounting for Common Method Variance in Cross-Sectional Research Designs.” Journal of Applied Psychology 86 (1): 114–121.
- Madhavan, R., and R. Grover. 1998. “From Embedded Knowledge to Embodied Knowledge: New Product Development as Knowledge Management.” Journal of Marketing 62 (4): 1–12.
- Maloni, M., and W. C. Benton. 2000. “Power Influences in the Supply Chain.” Journal of Business Logistics 21 (1): 49–74.
- Maltz, A. 2012. “Global Supply Chains: Other Voices.” Journal of Supply Chain Management 48 (3): 3–19.
- Meier, M. 2011. “Knowledge Management in Strategic Alliances: A Review of Empirical Evidence.” International Journal of Management Reviews 13 (1): 1–23.
- Mishra, A. A., and R. Shah. 2009. “In Union Lies Strength: Collaborative Competence in New Product Development and Its Performance Effects.” Journal of Operations Management 27 (4): 324–338.
- Modi, S. B., and V. A. Mabert. 2007. “Supplier Development: Improving Supplier Performance Through Knowledge Transfer.” Journal of Operations Management 25 (1): 42–64.
- Moeen, M., D. Somaya, and J. T. Mahoney. 2013. “Supply Portfolio Concentration in Outsourced Knowledge-Based Services.” Organization Science 24 (1): 262–279.
- Mudambi, S. M., and S. Tallman. 2010. “Make, Buy or Ally? Theoretical Perspectives on Knowledge Process Outsourcing Through Alliances.” Journal of Management Studies 47 (8): 1434–1456.
- Murayama, K., and F. Regalado. 2019. “Apple CEO Looks at ‘All Countries’ to Pick Best Suppliers.” NIKKEI Asian Review. https://asia.nikkei.com/Editor-s-Picks/Interview/Apple-CEO-looks-at-all-countries-to-pick-best-suppliers.
- Najafi Tavani, S., H. Sharifi, S. Soleimanof, and M. Najmi. 2013. “An Empirical Study of Firm’s Absorptive Capacity Dimensions, Supplier Involvement and New Product Development Performance.” International Journal of Production Research 51 (11): 3385–3403.
- Nakata, C., and K. Sivakumar. 1996. “National Culture and New Product Development: An Integrative Review.” Journal of Marketing 60 (1): 61–72.
- Nassimbeni, G. 2003. “Local Manufacturing Systems and Global Economy: Are They Compatible? The Case of the Italian Eyewear District.” Journal of Operations Management 21 (2): 151–171.
- Nepal, B., L. Monplaisir, and O. Famuyiwa. 2012. “Matching Product Architecture with Supply Chain Design.” European Journal of Operational Research 216 (2): 312–325.
- Ngai, E. W., C. Jin, and T. Liang. 2008. “A Qualitative Study of Inter-Organizational Knowledge Management in Complex Products and Systems Development.” R&D Management 38 (4): 421–440.
- Nonaka, I. 1994. “A Dynamic Theory of Organizational Knowledge Creation.” Organization Science 5 (1): 14–37.
- Nunnally, J. C., and I. H. Bernstein. 1994. Psychological Theory. New York: McGraw-Hill.
- Olson, E. M., O. C. Walker Jr, and R. W. Ruekert. 1995. “Organizing for Effective New Product Development: The Moderating Role of Product Innovativeness.” Journal of Marketing 59 (1): 48–62.
- Pashaei, S., and J. Olhager. 2015. “Product Architecture and Supply Chain Design: A Systematic Review and Research Agenda.” Supply Chain Management: An International Journal 20 (1): 98–112.
- Pathak, S., P. K. Sen, J. Jayaram, and J. M. Miller. 2019. “‘Like Poles Repel While Unlike Poles Attract’: Contextual Performance Effects of Supply Base R&D, Focal Firm R&D, and Commercialization.” Decision Sciences 50 (5): 985–1030.
- Pedersen, C. L., and T. Ritter. 2017. “The 4 Types of Project Manager.” Harvard Business Review Digital Articles, July 27, 2–8.
- Petersen, K. J., R. B. Handfield, and G. L. Ragatz. 2005. “Supplier Integration into New Product Development: Coordinating Product, Process and Supply Chain Design.” Journal of Operations Management 23 (4): 371–388.
- Phelan, N. 2018. “Audi’s Autonomous Intelligent Driving Teams Up with Luminar.” Business Insider. https://www.businesswire.com/news/home/20181218005209/en/Audis-Autonomous-Intelligent-Driving-Teams-Up-With-Luminar.
- Potter, A., and A. Paulraj. 2020. “Building Supplier Innovation Triads: The Effects of Leadership Relationships and Alliance Partner Diversity.” International Journal of Operations & Production Management 40 (2): 144–172.
- Preacher, K. J., P. J. Curran, and D. J. Bauer. 2003. “Simple Intercepts, Simple Slopes, and Regions of Significance in MLR 2-Way Interactions.” Quantpsy 24.
- Primo, M. A., and S. D. Amundson. 2002. “An Exploratory Study of the Effects of Supplier Relationships on New Product Development Outcomes.” Journal of Operations Management 20 (1): 33–52.
- Ragatz, G. L., R. B. Handfield, and T. V. Scannell. 1997. “Success Factors for Integrating Suppliers into New Product Development.” Journal of Product Innovation Management 14 (3): 190–202.
- Reagans, R., and B. McEvily. 2003. “Network Structure and Knowledge Transfer: The Effects of Cohesion and Range.” Administrative Science Quarterly 48 (2): 240–267.
- Rönkkö, M., C. N. McIntosh, J. Antonakis, and J. R. Edwards. 2016. “Partial Least Squares Path Modeling: Time for Some Serious Second Thoughts.” Journal of Operations Management 48: 9–27.
- Rothaermel, F. T., and D. L. Deeds. 2004. “Exploration and Exploitation Alliances in Biotechnology: A System of New Product Development.” Strategic Management Journal 25 (3): 201–221.
- Rubera, G., D. Chandrasekaran, and A. Ordanini. 2016. “Open Innovation, Product Portfolio Innovativeness and Firm Performance: The Dual Role of New Product Development Capabilities.” Journal of the Academy of Marketing Science 44 (2): 166–184.
- Salomo, S., E. J. Keinschmidt, and U. De Brentani. 2010. “Managing New Product Development Teams in a Globally Dispersed NPD Program.” Journal of Product Innovation Management 27 (7): 955–971.
- Samaniego, A., A. Gardner, C. Genteel, and L. Greenhalgh. 2017. “How They Did It: Google’s Innovative Approach to Supplier Diversity.” Supply Chain Management Review. https://www.scmr.com/article/how_they_did_it_googles_innovative_approach_to_supplier_diversity.
- Santoro, G., S. Bresciani, and A. Papa. 2020. “Collaborative Modes with Cultural and Creative Industries and Innovation Performance: The Moderating Role of Heterogeneous Sources of Knowledge and Absorptive Capacity.” Technovation 92: 102040.
- Sardeshmukh, S. R., and R. J. Vandenberg. 2017. “Integrating Moderation and Mediation: A Structural Equation Modeling Approach.” Organizational Research Methods 20 (4): 721–745.
- Sarin, S., and C. McDermott. 2003. “The Effect of Team Leader Characteristics on Learning, Knowledge Application, and Performance of Cross-Functional New Product Development Teams.” Decision Sciences 34 (4): 707–739.
- Scheer, L. K., N. Kumar, and J.-B. E. Steenkamp. 2003. “Reactions to Perceived Inequity in US and Dutch Interorganizational Relationships.” Academy of Management Journal 46 (3): 303–316.
- Schilling, M. A. 2000. “Toward a General Modular Systems Theory and Its Application to Interfirm Product Modularity.” Academy of Management Review 25 (2): 312–334.
- Schoenherr, T., and S. M. Wagner. 2016. “Supplier Involvement in the Fuzzy Front End of New Product Development: An Investigation of Homophily, Benevolence and Market Turbulence.” International Journal of Production Economics 180: 101–113.
- Shah, R., and P. T. Ward. 2003. “Lean Manufacturing: Context, Practice Bundles, and Performance.” Journal of Operations Management 21 (2): 129–149.
- Singh, J. 2008. “Distributed R&D, Cross-Regional Knowledge Integration and Quality of Innovative Output.” Research Policy 37 (1): 77–96.
- Sivadas, E., and F. R. Dwyer. 2000. “An Examination of Organizational Factors Influencing New Product Success in Internal and Alliance-Based Processes.” Journal of Marketing 64 (1): 31–49.
- Slot, J. H., S. Wuyts, and I. Geyskens. 2020. “Buyer Participation in Outsourced New Product Development Projects: The Role of Relationship Multiplexity.” Journal of Operations Management 66 (5): 578–612.
- Sosa, M. E., S. D. Eppinger, and C. M. Rowles. 2004. “The Misalignment of Product Architecture and Organizational Structure in Complex Product Development.” Management Science 50 (12): 1674–1689.
- Subramaniam, M., and N. Venkatraman. 2001. “Determinants of Transnational New Product Development Capability: Testing the Influence of Transferring and Deploying Tacit Overseas Knowledge.” Strategic Management Journal 22 (4): 359–378.
- Subramaniam, M., and M. A. Youndt. 2005. “The Influence of Intellectual Capital on the Types of Innovative Capabilities.” Academy of Management Journal 48 (3): 450–463.
- Swink, M. L., and R. Calantone. 2004. “Design-Manufacturing Integration as a Mediator of Antecedents to New Product Design Quality.” IEEE Transactions on Engineering Management 51 (4): 472–482.
- Szulanski, G. 1996. “Exploring Internal Stickiness: Impediments to the Transfer of Best Practice Within the Firm.” Strategic Management Journal 17 (2): 27–43.
- Tang, C., and B. Tomlin. 2008. “The Power of Flexibility for Mitigating Supply Chain Risks.” International Journal of Production Economics 116 (1): 12–27.
- Tatikonda, M. V., and S. R. Rosenthal. 2000a. “Successful Execution of Product Development Projects: Balancing Firmness and Flexibility in the Innovation Process.” Journal of Operations Management 18 (4): 401–425.
- Tatikonda, M. V., and S. R. Rosenthal. 2000b. “Technology Novelty, Project Complexity, and Product Development Project Execution Success: A Deeper Look at Task Uncertainty in Product Innovation.” IEEE Transactions on Engineering Management 47 (1): 74–87.
- Taylor, E., and J. Poltz. 2020. “Audi Says Computing is the New Horsepower.” Reuters News. https://www.reuters.com/article/us-audi-ceo-strategy-idUSKCN24V2F0.
- Ülkü, S., and G. M. Schmidt. 2011. “Matching Product Architecture and Supply Chain Configuration.” Production and Operations Management 20 (1): 16–31.
- Vasudeva, G., and J. Anand. 2011. “Unpacking Absorptive Capacity: A Study of Knowledge Utilization from Alliance Portfolios.” Academy of Management Journal 54 (3): 611–623.
- Volkswagen. 2017. The Future is Created Through Research-Volkswagen Group Research is Designing Tomorrow's World. Volkswagen Group Press Release. https://www.volkswagennewsroom.com/en/press-releases/the-future-is-created-through-research-volkswagen-group-research-is-designing-tomorrows-world-997.
- von Briel, F., C. Schneider, and P. B. Lowry. 2018. “Absorbing Knowledge from and with External Partners: The Role of Social Integration Mechanisms.” Decision Sciences 50 (1): 1–39.
- von Hippel, E. 1988. The Sources of Innovation. Cambridge: MIT Press.
- von Hippel, E., and G. von Krogh. 2003. “Open Source Software and the “Private-Collective” Innovation Model: Issues for Organization Science.” Organization Science 14 (2): 209–223.
- Wagner, S. M. 2012. “Tapping Supplier Innovation.” Journal of Supply Chain Management 48 (2): 37–52.
- Wagner, S. M., A. Eggert, and E. Lindemann. 2010. “Creating and Appropriating Value in Collaborative Relationships.” Journal of Business Research 63 (8): 840–848.
- Wagner, S. M., and R. Kemmerling. 2010. “Handling Nonresponse in Logistics Research.” Journal of Business Logistics 31 (2): 357–381.
- Wang, M.-C., P.-C. Chen, and S.-C. Fang. 2018. “A Critical View of Knowledge Networks and Innovation Performance: The Mediation Role of Firms’ Knowledge Integration Capability.” Journal of Business Research 88: 222–233.
- Wang, Y., S. B. Modi, and T. Schoenherr. 2020. “Leveraging Sustainable Design Practices Through Supplier Involvement in New Product Development: The Role of the Supplier's Environmental Management Capability.” International Journal of Production Economics 232: 107919.
- Wooldridge, J. M. 2010. Econometric Analysis of Cross Section and Panel Data. Cambridge: MIT Press.
- Wowak, K. D., C. W. Craighead, D. J. Ketchen, and G. T. M. Hult. 2016. “Toward a ‘Theoretical Toolbox’ for the Supplier-Enabled Fuzzy Front end of the New Product Development Process.” Journal of Supply Chain Management 52 (1): 66–81.
- Wuyts, S., and S. Dutta. 2014. “Benefiting from Alliance Portfolio Diversity: The Role of Past Internal Knowledge Creation Strategy.” Journal of Management 40 (6): 1653–1674.
- Yan, T., and A. Azadegan. 2017. “Comparing Inter-Organizational New Product Development Strategies: Buy or Ally; Supply-Chain or Non-Supply-Chain Partners?” International Journal of Production Economics 183: 21–38.
- Yan, T., and K. J. Dooley. 2013. “Communication Intensity, Goal Congruence, and Uncertainty in Buyer-Supplier New Product Development.” Journal of Operations Management 31 (7–8): 523–542.
- Yan, T., and A. Nair. 2016. “Structuring Supplier Involvement in New Product Development: A China–U.S. Study.” Decision Sciences 47 (4): 589–627.
- Yan, T., and S. M. Wagner. 2017. “Do What and with Whom? Value Creation and Appropriation in Inter-Organizational New Product Development Projects.” International Journal of Production Economics 191: 1–14.
- Yan, T., Y. Yang, K. Dooley, and S. Chae. 2020. “Trading-Off Innovation Novelty and Information Protection in Supplier Selection for a New Product Development Project: Supplier Ties as Signals.” Journal of Operations Management 66 (7–8): 933–957.
- Yli-Renko, H., E. Autio, and H. J. Sapienza. 2001. “Social Capital, Knowledge Acquisition, and Knowledge Exploitation in Young Technology-Based Firms.” Strategic Management Journal 22 (7): 587–613.
- York, J. G., and S. Venkataraman. 2010. “The Entrepreneur-Environment Nexus: Uncertainty, Innovation, and Allocation.” Journal of Business Venturing 25 (5): 449–463.
- Yu, L., Y. Duan, and T. Fan. 2020. “Innovation Performance of New Products in China's High-Technology Industry.” International Journal of Production Economics 219: 204–215.
- Zhang, S., C. Yuan, and Y. Wang. 2019. “The Impact of Industry–University–Research Alliance Portfolio Diversity on Firm Innovation: Evidence from Chinese Manufacturing Firms.” Sustainability 11 (8): 2321.
Appendix
Table A1. Multi-group SEM results.