Abstract
Many companies, despite there being opportunities for automation in production and logistics (P&L) systems, still rely on human workers due to their cognitive and motor skills. Taking Human Factor (HF) aspects into consideration when making P&L system design and management decisions is therefore important, an ignorance of HF potentially resulting in operator fatigue, discomfort, subsequent injuries and negative consequences for operator performance and the P&L system. A review of the literature shows that the majority of studies that take HF into consideration focus either on designing the workplace or on operation planning activities. There is also still a gap in the literature. Little has been published on P&L systems that incorporate HF and that combine different levels of short-term operational policy decisions (e.g. job allocation) and long-term system characteristic decisions (e.g. layout design). Current state-of-the-art frameworks that support the design and management of P&L systems and that take HF into consideration rarely consider different decision levels. This study proposes a new framework that incorporates HF into P&L systems by combining different levels of decisions to improve performance, quality, and well-being.
1. Introduction
Production and Logistics (P&L) systems contain operation processes that play a crucial role in the maintenance of a company’s competitiveness (Sgarbossa et al. Citation2020). P&L systems consist of components such as equipment/machines and humans that work together as a sociotechnical system to carry out processes (Abdu, Peter, and Gupta Citation2016). Enhancing the performance of P&L systems requires improvements to all components jointly, as they are interlinked (Nardo, Forino, and Murino Citation2020; Boon-itt and Wong Citation2016; Bolatan et al. Citation2016; Neumann and Dul Citation2010; Neumann et al. Citation2021). A review of recent studies of operation processes shows a focus on only automating equipment/machines, human-related aspects being neglected (Lee and Seppelt Citation2009). Humans are critically involved in many operational processes. They are more flexible than equipment/machines and can adapt to rapid changes (Sgarbossa et al. Citation2020). Most companies also find retaining manual human work to be more affordable than automation (Kadir, Broberg, and Conceição Citation2019). Failing to consider human factor (HF) aspects in a P&L system may therefore result in a deviation from the performance enhancements that are expected to be achieved (Sgarbossa et al. Citation2020).
The International Ergonomics Association has defined HF as: ‘The scientific discipline concerned with the understanding of interactions among humans and other elements of a system, and the profession that applies theory, principles, data, and methods to design in order to optimize human well-being and overall system performance’. It is clear from this definition that there is a huge need to consider both social and business goals when designing and managing a system (De Nul, Breque, and Petridis Citation2021).
Sgarbossa et al. (Citation2020) showed that most P&L planning models developed for P&L system managerial decision support have neglected or underestimated HF considerations. This can lead to undesired deviations in system performance through increases in the time and cost of operations and reductions in productivity and quality of work (Sobhani, Wahab, and Neumann Citation2017). A lack of knowledge of HF issues can result in fatigue, discomfort, and subsequent injuries of operators, so reducing their performance and increasing errors (quality) (Kolus, Wells, and Neumann Citation2018).
Thus, to improve the performance of a P&L system, it is important to consider HF when making decisions based on the design and management of the system, for instance, improving the performance of order picking systems by evaluating the ergonomics of order pickers when picking products from different pallet picking storage design alternatives (Calzavara et al. Citation2016), and also improving the performance of order picking systems by considering the operational decisions, such as the scheduling of activities among operators based on their accumulated fatigue and recovery (Calzavara et al. Citation2019).
It is important, if we are to understand the cause of deviations between actual and expected performance, that we understand the impact of design and management decisions on the interaction between humans and system elements. This paper therefore considers two major decision levels: Design Level (DL), decisions at this level being based on long-term goals at the point in time the system is designed; and Operational Level (OL), decisions being based on short-term goals at the point in time the system is brought into operation.
Decisions at the design level primarily promote long-term duration (Schmidt and Wilhelm Citation2010). Practitioners, such as designers and high-level managers who are responsible for system design decisions, make design-level decisions before the system is brought into operation (Kolus, Wells, and Neumann Citation2018). Examples of decisions made at the design level include assembly layout design, warehouse layout design, and level of automation. These decisions are based on specific requirements and require investment and effort to implement. They therefore cannot be changed habitually and must be made in a very deliberate way, weighing many considerations (Elbanna Citation2006). Decisions at the design level are therefore usually made multi-annually (Schmidt and Wilhelm Citation2010).
Decisions made at the operational level primarily relate to the short-term duration. Practitioners such as industrial engineers and low-level managers are responsible, at this level, for making decisions on system use and how the system is to operate (Kolus, Wells, and Neumann Citation2018). Job scheduling, job sequencing, and routing are examples of operational-level decisions made on a short-term basis. These can be changed quickly, as they do not require high levels of investment and effort to implement, and can therefore be changed habitually (de Koster, le-Duc, and Roodbergen Citation2007). OL decisions are therefore usually made daily or weekly (Schmidt and Wilhelm Citation2010).
Figure shows the interaction between the design level and the operational level. Design decisions initially impact at the operational level, as represented by the grey arrow. An example of this is warehouse layout design, which includes the setting of the number of blocks, the number, width, and length of aisles in each block, and rack layout configurations. These are design-level decisions made for a long-term period. These are, however, also decisions that impact the ways operators work and therefore, in turn, HF aspects of the workplace (Altarazi and Ammouri Citation2018; Calzavara et al. Citation2016). Not taking HF into consideration at the operational level can therefore result in work-related health problems such as lower back pain, and general fatigue and so a negative deviation from expected performance (Grosse, Glock, Jaber, et al. Citation2015). The operational level also has an impact on the design level, decisions made at this level causing actual performance to deviate from expected. This, for example, can be due to work-related illness, absenteeism, and presenteeism. New and additional decisions must therefore be made at the design level to counter the deviation in performance, examples of this including increasing the number of operators (Sobhani, Wahab, and Neumann Citation2015a), as represented by the black arrow.
Figure 1. Interaction between design level and operational level.
The figure depicts the interaction between the design and operational levels, with examples for each level.
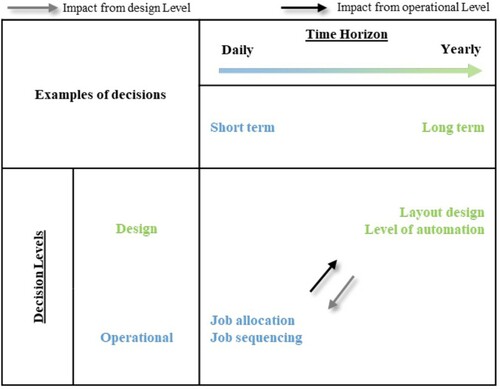
Decisions that aid P&L systems are the main aim and scope of the International Journal of Production Research (IJPR). This paper therefore contributes to the journal aim by investigating decision levels in P&L systems that take HF into consideration. A positive and important trend in IJPR is the evolution of models that include HF (Grosse, Glock, and Neumann Citation2017), the integration of HF not having been given much consideration in the production research and industrial engineering field. Studies currently focus on the design level or operational level, only one paper in IJPR examining frameworks that incorporate HF in P&L systems – Grosse et al. (Citation2015). The authors of this paper therefore discuss the inclusion of HF in order picking systems.
The above argument illustrates that there is a need for a new framework that can encourage and guide practitioners to take HF into consideration when designing and managing P&L systems. There are very few frameworks in the engineering literature that incorporate HF into P&L systems (Karltun et al. Citation2017). These have furthermore failed to emphasise the necessity of incorporating HF into P&L systems and mix the long-term and short-term decision time frame in the design and management of P&L systems (Sgarbossa et al. Citation2020). The new framework presented here therefore incorporates HF in P&L systems from both DL and OL perspectives. It also shows the interaction between the two based on defined attributes, variables, and their relationships. This can be used to reduce deviation from expected performance, so demonstrating practical relevance, scientific rigour being demonstrated through this being supported by previously published research.
Two research objectives (RO) have been defined to support the development of the new framework, which are as follows:
RO1: To develop a new framework that incorporates HF in P&L systems that combine different decision levels
State-of-the-art frameworks that incorporate HF in P&L systems were first understood, to support scientific rigour. The gap in the current frameworks was then analyzed. A new framework was then developed to fill the gap in the literature.
RO2: To assist practitioners in applying the developed framework in P&L systems
The process of using the framework should be explained to practitioners to support practical relevance. Practitioners include managers, designers, industrial engineers, HF professionals (ergonomists), and teams involved in the design and management of the system.
The remainder of this paper is organised as follows. Section 2 explains the methodology adopted in this paper. Section 3 explains state-of-the-art frameworks that incorporate HF in P&L systems. Section 4 explains the newly developed framework and provides examples of decision levels and variables. Section 5 explains how the developed framework is applied. Section 6 summarises the paper by highlighting key points.
2. Methodology
This paper has adopted a three-stage research approach to providing a solution to the above research objectives: analyze the state-of-the-art frameworks, develop a new framework, and explain how the framework can be applied. The research design is further elaborated below.
2.1. State-of-the-art frameworks
It is important that current state-of-the-art frameworks that incorporate HF into P&L systems are first understood. A literature review (LR) was therefore conducted to find state-of-the-art frameworks, the LR providing a critical assessment of literature on the conceptual framework (Hart Citation1998). A three-step LR was therefore conducted to determine the need for a new framework.
Step 1 Searching the papers: Frameworks were found by using the search terms ‘human factors’, ‘conceptual framework’, and ‘production and logistics systems’ and the synonyms listed in Table . A search string was created from these search terms using ‘AND’ and ‘OR’, so arranging keywords in sets to ensure all possible results are included. The search string was then used to search three main databases, SCOPUS, Web of Science, and Engineering Village. These databases were selected due to their broad access to scientific studies in the areas of HF, engineering, and management. The search yielded 307 papers, including peer-reviewed journals, conference papers, and reviews, so ensuring that all possible frameworks were included in the search result.
Table 1. Search terms used for step 2.1.
Step 2 Filtration: The 307 papers were filtered in two stages. The papers were, in the first stage, filtered based on the abstract to select out papers that addressed HF in P&L systems. This resulted in 104 papers. These 104 papers were, in the second stage, thoroughly screened to find frameworks that address HF in P&L systems. The filtration processes did not restrict papers based on time frame to ensure the inclusion of every reliable conceptual framework across all time frames. This resulted in 16 frameworks that illustrate HF in P&L systems.
Step 3 Data analysis and interpreting frameworks: The shortlisted frameworks were then analyzed and categorised based on four factors – scope of framework, decision level, HF aspects, and performance. These factors address which P&L system area the framework explores, the decision level that can be investigated using the framework, which HF aspects are explored through the framework, and the performance measured within the framework. These are further elaborated under Section 3. The purpose and limitation of each framework was then analyzed to understand the gaps in this set of frameworks, and to obtain an indication of the need for a new framework.
2.2. Developing a framework
The authors, after understanding the need for a new framework (the why) in P&L systems (the where), developed a new conceptual framework. Developing a framework requires variables at both DL and OL, because these variables are the framework characteristics that require study (Miles and Huberman Citation1994). Framework variables were selected to address four attributes – ‘When’, ‘What’, ‘How’, and ‘With’ – through which the author explains the variables and their relationships (Miles and Huberman Citation1994). ‘When’ defines the time horizon across which decisions are made, ‘what’ defines the type of process that needs to be carried out, ‘how’ defines the arrangements and functioning of operations, and ‘with’ addresses the facilities that assist operations. The variables then need to be positioned in a framework. The variables for the new framework were selected from state-of-the-art frameworks, the positioning of variables and their relationship then being refined through a recursive process. The framework developed after a number of iterations and was cross-checked using the feasibility of the arrangements and reviews from experts in the field of human factors in production and logistics systems. The new framework and its variables and relationships are further explained in SECTION 4.
2.3. Framework application
RO2 is addressed by a literature search, which was conducted to find examples and to show the application of the new framework in an assembly system and order picking (OP) system. A standard structure for applying the framework was then created. The assembly system, as an example of the production system, and the OP system, as an example of the logistics system, were selected because these systems still include extensive manual human work (Sgarbossa et al. Citation2020). The examples were supported by literature from the literature search. The literature search was carried out in SCOPUS using keyword combinations such as ‘human factors’ and ‘assembly system’ for the assembly system, and ‘human factors’ and ‘order picking system’ for the order picking system, and corresponding synonyms as listed in Table . The search results were then filtered to include only papers, the time frame being limited to between 2010 and 2020. This resulted in 102 papers for the assembly system and 93 for the order picking system. The subject area was limited to engineering, business, management, and accounting, to limit papers to P&L systems. The top 10 papers for the assembly system and OP system were then shortlisted by citations and most recent, papers are relevant to the scope of the framework through this being selected. This filtering procedure was used to provide examples that illustrate the variables of the new framework. The papers further analyzed based on decision level (DL and OL), HF aspects, and performance. Examples that support the framework are provided in Section 4.5 and 4.6.
Table 2. Search terms used for step 2.3.
The authors, after exhibiting examples of applying the framework, developed standard steps that help practitioners apply the framework.
3. State-of-the-art frameworks
State-of-the-art frameworks that incorporate HF in P&L logistics systems were, to address RO1, listed in this section. The shortlisted frameworks from Section 2.1 were arranged in chronological order, a summary of the 16 frameworks being presented in Table . This table provides a brief description of the purpose of the framework and classifies the state-of-the-art framework based on its purpose, variables, scope, decision levels, HF aspects, and performance. The scope was primarily classified into Production (P) and Logistic (L) systems, and decision levels were classified into design level (DL) and operational level (OL). Frameworks that have variables both at DL and OL are categorised as being DL/OL. HF aspects were categorised based on the Physical (Ph) aspects linked to the physical conditions of the operator performing the task. Mental (M) aspects were linked to the cognitive condition of the operator, Perceptual (Pe) aspects were linked to the perceiving ability of the operator and Psychosocial (Ps) aspects included non-physical work dimensions such as job satisfaction, job demand, job control, work pressure supervisory and feedback. Performance was finally classified by productivity, well-being, and quality.
Table 3. Summary of state-of-the-art frameworks that incorporated HF in P&L systems.
Generic frameworks have been developed by Reiman and Oedewald (Citation2007), Mosier and Fischer (Citation2010), and Karltun et al. (Citation2017). These frameworks do not contain variables that fall under any specific scope. The Reiman and Oedewald (Citation2007) framework depicts organisational and work-psychological factors that influence the safety effects of human performance. The Mosier and Fischer (Citation2010) framework, however, illustrates the layers of context that surround individual decision-makers in complex environments. These frameworks were used based on the support of examples in P&L systems. Karltun et al. (Citation2017), however, introduced a model that involves humans as a separate subsystem at the same level as technology and organisation, this approach being termed the HTO (Human, Technology & Organization) concept. Reiman and Oedewald (Citation2007) and Mosier and Fischer (Citation2010) included variables from both the design level and operational level. The Karltun et al. (Citation2017) framework explains at the design level. Mosier and Fischer (Citation2010) and Karltun et al. (Citation2017) do not mention any specific HF aspects in their framework. Reiman and Oedewald (Citation2007), however, include a variable that relates to the psychosocial aspect of operators. All three frameworks only mention well-being during the performance.
The variables used in Das (Citation1998) and Bruseberg (Citation2008), in a production system, have not been classified by design and operational level. Das (Citation1998) has created a comprehensive model for industrial work design. Bruseberg (Citation2008), however, represents the human view through considering HF and systems engineering. The framework of Neumann and Village (Citation2012) integrates human factors into the work system design process, the variables in this framework being classified into design level and operational level. This framework does not, however, consider attributes at different decision levels such as ‘What’, ‘How’, and ‘With’. There are also many frameworks (Baines et al. Citation2005; Zhou et al. Citation2009; Neumann and Dul Citation2010; Krugh and Mears Citation2018; Kolus, Wells, and Neumann Citation2018; Peruzzini, Grandi, and Pellicciari Citation2020; Kadir and Broberg Citation2021) that were designated as being under the design level, only Sobhani, Wahab, and Neumann (Citation2016) describing a framework under the operational level. The framework of Baines et al. (Citation2005) supports simulation modelling tools for designing a system, the principal factors taking HF aspects into consideration. Zhou et al. (Citation2009) showed the relationship between task, workspace, tool and material attributes, worker attributes, postures, motions, and work-related musculoskeletal disorder (MSD) risk factors. Neumann and Dul (Citation2010) studied the effects of HF on operations systems (OS), and Krugh and Mears (Citation2018) integrated the cyber human system (CHS) with cyber-physical systems to assist smart manufacturing system application. Kolus, Wells, and Neumann (Citation2018) depicted the design phase of operations systems and showed their impact on humans and systems. Peruzzini, Grandi, and Pellicciari (Citation2020) assisted in collecting data on worker’s actions, the objective being to improve the performance of a factory, and Kadir and Broberg (Citation2021) helped design the work system through combining HF in the transition towards Industry 4.0. The frameworks of (Das Citation1998; Zhou et al. Citation2009; Neumann and Dul Citation2010;, Sobhani, Wahab, and Neumann Citation2016; Kolus, Wells, and Neumann Citation2018; Peruzzini et al. 2020; Kadir and Broberg Citation2021) have primarily examined physical aspects, but have been backed by mental aspects (Neumann and Dul Citation2010; Krugh and Mears Citation2018; Peruzzini, Grandi, and Pellicciari Citation2020; Kadir and Broberg Citation2021). Less attention has, however, been given to psychosocial aspects (Das Citation1998; Baines et al. Citation2005; Sobhani, Wahab, and Neumann Citation2016; Kadir and Broberg Citation2021). The performance variables of all the frameworks do, however, emphasise the well-being of the operators. The productivity of the production system has been examined in the frameworks of (Baines et al. Citation2005; Zhou et al. Citation2009; Neumann and Dul Citation2010; Neumann and Village Citation2012; Sobhani, Wahab, and Neumann Citation2016; Kolus, Wells, and Neumann Citation2018; Peruzzini, Grandi, and Pellicciari Citation2020). Manufacturing quality has, however, received the least attention in frameworks that address production systems (Neumann and Dul Citation2010; Neumann and Village Citation2012; Kolus, Wells, and Neumann Citation2018).
There are remarkably few frameworks in logistics systems that look to include HF in P&L systems. Grosse, Glock, and Müller (Citation2015) include variables from both the design and system levels but have not classified them accordingly. This framework is, however, the only one that mentions all four HF aspects, i.e. physical, mental, perceptual, and psychosocial. Figueira, Machado, and Nunes (Citation2012) have also described their framework at the design level. This framework does not mention any HF features specifically in its variables. The performance variables of the framework only examined the well-being of the operators.
All these frameworks had incorporated HF in the production and logistics system. There were 10 frameworks with production system scope, only 2 with logistics systems scope. Nine frameworks addressed variables at the design level, just one framework considering both decision levels. This framework has, however, not distinguished its variables between the design level and operational level. There is therefore a need for a framework that incorporates HF in P&L systems and considers the different decision levels. It is important to show the impact of decisions on the performance of the system, and on the well-being of the operators at the different levels of analysis.
4. Framework
A new framework is, based on the previous section, proposed and introduced in this section. Examples based on assembly systems and order picking problems are provided to support the framework variables.
The variables at DL were firstly adopted and modified based on the framework of Das (Citation1998) and Karltun et al. (Citation2017). This is based on that these frameworks are explained at OL and include critical variables. They also provide an answer to which attributes should be included in the new framework, resulting in variables such as ‘Process’, ‘System settings’ and ‘Technology’. Secondly, the variables at OL were adopted and modified based on the framework of Zhou et al. (Citation2009). This framework is explained at OL and includes critical variables that answer which attributes should be included in the new framework. This results in variables such as ‘task’, ‘operational policies’ and ‘tools’. The variables are shown in Table .
Table 4. Attributes of variables for the framework.
The grey arrow in Figure , which shows the interaction between DL and OL, shows the impact of DL on OL. This is due to HF aspects of operators being affected by decisions made at DL. HF aspects are therefore adopted into this framework from Grosse, Glock, and Müller (Citation2015), resulting in ‘physical’, ‘mental’, ‘psychosocial’, and ‘perceptual’. The black arrow in Figure furthermore shows the impact of the operational level on the design level due to decisions made at the operational level influencing performance at the design level. Three performance indicators are therefore introduced into the framework from Grosse, Glock, and Müller (Citation2015): ‘productivity’, ‘well-being’, and ‘quality’. HF aspects and performance are taken from Grosse, Glock, and Müller (Citation2015), as this is the only framework that mentions all four HF aspects and the three performances.
The framework developed is shown in Figure , the green triangle highlighting DL. This has an impact on HF operator aspects and is represented by single arrows. The blue triangle represents OL, and the double-line arrows from OL represent the performance that depends on OL. Variables under the design level, HF aspects, operational level, and performance level are explained below. The application of the framework will be further explained in the next section.
Figure 2. Framework incorporating HF in production and logistics systems.
The figure represents a framework to incorporate human factors with the design and management of production and logistics systems.
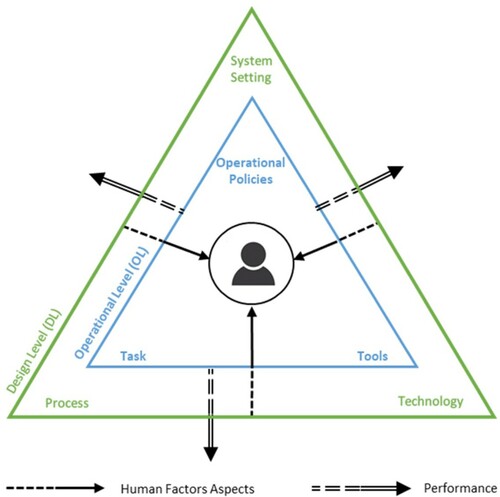
4.1. Design level (DL)
The variables of DL are process, system setting, and technology, and are interrelated.
Process: The process determines what kind of action and combination of tasks are needed to complete an activity. The processes in production systems determine the combination of tasks required to convert raw materials into finished products (Babu Citation2012), such as the assembly process and kitting process. Processes in a logistics system, however, determine the combination of tasks involved in the flow of products from the origin to the point of consumption to meet customer demand (Lummus, Krumwiede, and Vokurka Citation2001). For example, packaging, warehousing activities, cross-docking, and distribution.
System Setting: System setting determine the arrangement of tasks that need to be performed, to provide an optimised system. The system setting in the production system determines the type of production process engaged. This can for example be, in an assembly system, the system layout (line, u-shape, fixed position, etc.) and configurations (number of workstations) (Meller and Gau Citation1996). The system setting in logistics systems determine the designing and managing of tasks. For example, the layout design in the OP system, i.e. number of blocks and the number, length, and width of aisles in each block, and rack layout configurations (de Koster, le-Duc, and Roodbergen Citation2007).
Technology: The technology provides a mainstay for the entire system and ensures proper communication between each task in the system. New technologies have, with the advancement of technologies in the production system, assisted different processes. For example, collaborative robots sharing the workspace with humans in the assembly system (Michalos et al. Citation2015). The advancement of technologies in logistics systems can improve performance and reduce errors and costs. For example, in the OP system, deciding upon assistive technologies such as paperless picking, and AGV/AMR assisted picker to assist order pickers in picking the appropriate product from the storage locations (Jaghbeer, Hanson, and Johansson Citation2020).
4.2. HF aspects
The grey arrow in Figure shows the interaction of DL with OL, i.e. the impact of decisions at DL on the HF aspects of operators at OL. The four HF aspects physical, mental, perceptual, and psychosocial, are explained through this framework as mental, physical, perceptual, and psychosocial aspects.
Physical aspect: The physical aspect reflects physical ergonomics, risk, manual work tasks, fatigue, and posture condition of the operator in P&L systems. At DL, system settings and technologies can be designed to improve the physical aspects of the operator. For example, in an assembly system, taking into consideration the ergonomics of the operator in the design of the assembly system can deliver higher productivity through improved operator working conditions (Neumann and Medbo Citation2008). In an OP system, a different rack layout could have different ergonomic implications for operators, which can influence picking efficiency (Calzavara et al. Citation2016).
Mental aspects: The mental aspect reflects the cognitive, learning/forgetting behavioural and training condition of the operator in P&L systems. At DL, system settings and technologies can be designed to improve the cognitive workload of the operator. For example, in an assembly system, the parallel flow production line had longer ramp-up times and higher throughput capacity than serial flow. The learning effect upon the operator was, in parallel flow, not taken into consideration when setting up production flow (Neumann and Medbo Citation2017). In an OP system, order picker learning has an impact on OP efficiency. Warehouse layout therefore needs to be designed such that ambiguity for order pickers is reduced, so increasing efficiency (Grosse and Glock Citation2015).
Perceptual aspects: The perceptual aspect reflects the visual, auditory, or tactility demands on operators in P&L systems. At DL, system settings and technologies can be designed for the perceptual aspects of the operator. For example, in an assembly system, assistive technologies such as augmented reality can support the information perceiving skill of the operator, and so improve productivity (Michalos et al. Citation2015). Paperless picking solutions have reduced pick error in an OP system by assisting the perceptual aspect of order pickers (Battini et al. Citation2015).
Psychosocial aspects: The psychosocial aspect reflects the motivation, feedback, incentives, stress, boredom, work satisfaction, time pressure, and personal condition of the operator in P&L systems (Vandergrift et al. Citation2012). System settings and technologies can be designed at DL for the psychosocial aspects of the operator. For example, in an assembly system, designing the system without considering the psychosocial aspect of operators can lead to a reduction in productivity and can also impact operator health (Vandergrift et al. Citation2012; Di Pasquale et al. Citation2018). The introduction of paperless picking design in an OP process has been shown to not only reduced picking error but also improved worker satisfaction (Goomas and Yeow Citation2010).
4.3. Operational Level (OL)
The variables of OL are tasks, operational policies, and tools, and are interrelated.
Task: Task defines the action the operator must carry out within the defined space of work. For example, the tasks in an assembly process are the actions the operator must carry out at his workstation to assemble the parts. This could involve tasks such as locate the part, pick, locate the position and assemble the parts (Pimminger, et al. Citation2020). In an OP system, tasks are the actions the operator must carry out within his physical zone to fulfil the customer orders, tasks for example including setup, travel, search, and pick (de Koster, le-Duc, and Roodbergen Citation2007).
Operational Policies: Operational policies control the work environment in which the defined task is performed. The working environment determines the ergonomic factor of the task. For example, job allocation and job rotation among operators in an assembly process improve the working conditions of the operator (Battini, Delorm, et al. Citation2016). In an OP process, policies relating to batching, zoning, routing, and storage assignments are operational policies that can improve or degrade the working conditions of order pickers (de Koster, le-Duc, and Roodbergen Citation2007).
Tools: An operator engaged in a task requires tools to perform the task. For example, the use of VR/MR (Virtual Reality/Mixed Reality) in an assembly process assists the operator in the assembly of the product by providing step-by-step instructions (Wolfartsberger, Haslwanter, and Lindorfer Citation2019). In an OP process, the tools required to pick-by-voice include a headset, microphone, and a barcode scanner to crosscheck the item (Jaghbeer, Hanson, and Johansson Citation2020).
4.4. Performance
The three performances are explained through this framework. These primarily are performance, quality, and well-being.
Productivity: The productivity of the system determines the time and cost required to complete an activity. For example, improper operator working conditions in an assembly system can have a negative effect on the financial performance of the system (Sobhani, Wahab, and Neumann Citation2015b). In an OP system, an order picker with less experience of the storage assignment can have a negative effect on the performance of the system, due to the increased time required to search for and identify the product (Grosse and Glock Citation2013).
Quality: Quality is determined by the rate of error during the activity. For example, human error in assembly systems is due to aspects such as non-compliant products, injuries, machine slowdown or failure, near misses, and latent errors (Pasquale et al. Citation2016). In an OP system, pick errors are caused by the wrong product or item being picked from the wrong storage location due to complex storage methods (Burinskiene Citation2010).
Well-being: Health encompasses overall human well-being and is influenced by the entire system. Ill-health in workers can lead to substantial production and financial losses due to decreases in on-the-job performance and longer recovery. For example, the well-being of the operator in assembly systems depends on working conditions, examples including the task repetitiveness, handling of heavy loads, and types of tools used for assembly (Sobhani, Wahab, and Neumann Citation2017). In an OP system, the well-being of the order picker depends on the shape, size, weight, location, and position of items to be picked, and pick frequency (Grosse Glock, Jaber, et al. Citation2015).
4.5. Examples of the decision levels and variables based on assembly system
Examples of assembly systems are provided in Table and illustrate the application of the proposed framework in a production system. These examples are supported by previous literature, a significant number of these papers being from IJPR. The methodology used gives the most cited and relevant literature for the scope of the framework. These examples are further listed below (Table 6).
Table 5. Examples regarding variables of the framework based on assembly system.
Table 6. Examples of variables of the framework based on order picking system.
4.5.1. Design level
Five examples from the literature on the variables of DL in an assembly system are provided below.
Battini et al. (Citation2016c) investigated system settings based on assembly line balancing. Operator’s physical aspects were examined using their energy expenditure, performance being measured by the productivity and well-being of the operators. The results from the study show that different assembly line balancing solutions have an impact on the productivity of the system and the well-being of the operators.
Neumann and Medbo (Citation2017) compared two different system settings, serial flow and parallel assembly lines. The mental aspect of operators was then investigated using their learning rate. Performance is measured by the productivity and well-being of the operators. The results of the study show that the benefits of parallel flow exceed those of conventional serial flow, despite a reduced learning effect due to the longer cycle time (and hence reduced learning opportunities).
Finco, Battini, et al. (Citation2020) investigated system settings based on assembly line balancing. Operator physical aspects were examined using their energy expenditure, performance being measured by the productivity and well-being of the operators. The study introduced a new mathematical model to improve the assembly process performance, both the performance and well-being of the operator being taken into consideration.
Mattsson et al. (Citation2020) investigated technology based on the cognitive automation strategy associated with complex assembly systems. The mental and psychosocial aspects of operators were investigated using operator learning, stress, and job satisfaction, the performance of the system being evaluated by the productivity and well-being of the operator. The results of the study suggest a three-step strategy for the design of technology that takes into consideration cognitive automation outcomes.
Hietanen et al. (Citation2020) investigated technology aspects through the design of human-robot collaboration that uses augmented reality (AR). Operator physical aspects were studied using their workload, system performance being measured by productivity, well-being, and quality. The results from the study show that projector-based AR human-robot collaboration yields better performance than the baseline without AR and HoloLens-based AR human-robot collaborations.
Most of the studies at DL in these assembly system examples that support framework variables have explored system settings in an assembly system through layout. It is also clear, from the examples, that operator physical aspects are the primary aspect examined under HF aspects, most of the studies including the improvement of operator ergonomics in the assembly layout. The studies therefore show that involving HF aspects in the design of assembly layout has a positive impact on performance through, for example, improved productivity and well-being of the operator and reductions in errors (quality).
4.5.2. Operational level
Five examples from the literature on the variables of OL in an assembly system are provided below.
Lee, Chang, and Choe (Citation2017) have studied technology, using moving robots and automated storage and retrieval systems (AS/RS) in co-work OP systems. The order picker physical aspects examined under HF aspects relate to operator working posture. Performance was measured by the productivity and well-being of the operators, the results showing that AS/RS resulted in lower ergonomic risk than moving robot-type OP systems.
Kim, Nussbaum, and Gabbard (Citation2019) have studied tools using head-worn displays (HWD). The operator perceptual and psychosocial aspects investigated under HF aspects were visual discomfort and job performance, performance being measured by well-being and quality. The results show that graphics-based information on the HWD increased performance and decreased the perceived workload of operators.
Finco, Calzavara, et al. (Citation2020) have studied operational policies using job sequencing, and the physical conditions of the operator. Operator physical aspects were investigated using the energy expenditure of the operators, performance being measured by the productivity and well-being of operators. The results show that the age of operators has an impact on system performance, when measured in terms of operator productivity and well-being.
Savino, Riccio, and Menanno (Citation2020) studied operational policies using workforce scheduling to investigate the influence of ergonomics. Operator physical aspects were investigated using the posture of the operators, performance being measured by the productivity and well-being of the operators. The results show that ergonomics has an impact on workforce scheduling through maximising production capacity and reducing physical loads on operators.
Bänziger, Kunz, and Wegener (Citation2020) have studied operational policies using task allocation in human-robot systems. Operator physical aspect was investigated under HF aspects using operator workload, performance being measured by the productivity and well-being of operators. The results show a new method for task allocation in a human-robot system which takes into consideration that there is an operator and robot in a shared workspace.
Most of the studies at OL, in these examples of the assembly system that support the variables of the framework, have explored the management of operational policy using job rotation. It can be seen from the examples that operator physical aspects are the most examined, as physical fatigue can be quantified for each operator and optimal job rotation can then be introduced. The examples therefore show that job rotation based on the operator physical aspect has improved performance based on time (productivity) and reduced ergonomic risks (well-being).
4.6. Examples of decision levels and variables based on the order picking system
Examples of OP systems are provided to illustrate the application of the developed framework in a logistics system. The examples are supported by the literature, a significant number of papers being from IJPR. The methodology gives the most cited and relevant literature for the scope of the framework. These examples are further listed below.
4.6.1. Design level
Five examples from the literature on the variables of DL in OP systems are provided below.
Reif et al. (Citation2010) studied technology aspects by comparing pick by light with conventional picking list approaches. The perceptual and psychosocial HF aspects of order pickers were examined using reading ability and motivation, performance being measured by productivity and quality. The results show that pick by vision outperformed the conventional picking list in terms of picking time and error rate.
Yeow and Goomas (Citation2014) studied technology design by comparing the conventional picking list with a wireless handheld scanning system. The perceptual and psychosocial HF aspects of order pickers were examined using visual/audio feedback and order picker satisfaction, performance being measured by productivity, well-being, and quality. The results show that the new technology improved productivity, worker satisfaction, and work quality.
Calzavara et al. (Citation2016) analyzed different operational policies using different storage layouts including pallets, half-pallets, and half-pallets equipped with the pull-out system. Order picker physical aspect was examined under HF aspects using the energy expenditure rate of the order picker. Performance was measured by productivity and well-being. The results show that replacing the conventional pallet storage system with half-pallets on the upper and with half-pallets with a pull system on the lower rack improves economic and ergonomic performance.
Kreutzfeldt, Renker, and Rinkenauer (Citation2018) studied the technology design of smart devices through the attentional perspective of users. The mental and perceptual aspects were examined under HF aspects using cognitive workload and information processing capabilities. Performance was measured by productivity, well-being, and quality. The results show that smart glasses are, in an order picking system, more suitable for more complex tasks, and that headsets are more suitable for simple tasks.
Nookea and Vanichchinchai (Citation2020) have studied operational policies using different storage layouts that assign products to different heights. Physical aspects were examined under HF aspects using the body posture of order pickers, performance being measured by the productivity and well-being of order pickers. The results show that products arranged based on the demand for the products improved picking efficiency and reduced the physical stress on order pickers.
Most of the studies at DL in these OP system examples that support the variables of the framework have explored the design of technologies in an OP system primarily by examining technologies that assist operators in picking tasks, such as pick by voice, pick by light, and HMD. Order picker perceptual aspects were primarily examined in the examples, as these are the technologies that are used to assist order pickers in picking the right product from the right storage location. Performance in the studies shows improvements in worker satisfaction (well-being) and reductions in errors (quality).
4.6.2. Operational level
Five examples from the literature on variables at the OL in OP systems are provided below.
Battini, Calzavara, et al. (Citation2016) studied operational policy using human availability in two situations (directly employed and indirectly employed order pickers). The order picker physical aspect was examined under HF aspects using the ergonomic conditions, the performance of the system being measured by productivity and well-being. Battini et al. therefore proposed new procedures that could assist in the estimation of ergonomic effort in an OP system, through using human availability and rest allowance. The results show that these procedures could evaluate future interventions based on cost and ergonomics.
Battini, Glock, et al. (Citation2016) have studied operational policy using storage assignments policies, order picker energy expenditure rate, and order processing time. System performance was measured by productivity and well-being. Order picker physical aspects were those examined under HF aspects. The authors developed a mathematical model that estimates the trade-offs between energy expenditure rate and time, for different storage assignment solutions.
Elbert et al. (Citation2017) have studied operational policy using routing policies and human behaviour. Order picker mental aspect was examined under HF using behavioural factors. The performance of the system was measured by productivity and well-being. The results show that order pickers tend to deviate from the defined route if they find it to be impractical, so reducing OP efficiency.
Diefenbach and Glock (Citation2019) studied operational policies using storage assignments in a U-shaped order picking zone. Order picker physical workload was examined under HF aspects using walking distance and ergonomic strain. The performance of the system was measured by productivity and well-being. The authors developed a mathematical model that reduces the travel distance and ergonomic strain on order pickers through optimal storage assignments.
Motmans, Debaets, and Chrispeels (Citation2018) have studied tools by examining the use of order picker exoskeletons in the OP system. Order picker physical workload was examined under HF aspects using the working posture of the back and shoulders of order pickers. The performance of the system was measured by the well-being of order pickers. The results show that the exoskeleton positively influenced physical load, reduced fatigue, and improved the safety of order pickers.
Most of the studies at OL in the examples of the OP system that support the variables of the framework, explore the management of operational policy primarily through storage assignment policies. The physical and mental aspects of order pickers were examined in the examples through storage assignment policies, as incorrectly placed products can impact the energy expenditure and learning effect of order pickers. Storage assignment policies that take into consideration the physical and mental aspects of order pickers can therefore improve performance through the improved picking time (productivity) and better working conditions (well-being) of order pickers. The new framework therefore, through this, successfully accommodates the understanding and inter-relation between the framework variables.
5. How to apply the framework?
Standard steps to help practitioners apply the framework when designing a new system or improving an existing system have been developed based on the above examples that show the application of the developed framework in P&L systems. Practitioners, which includes industrial engineers and operational managers, must be conscious of the implications of designing and managing P&L systems. The standard steps for applying this framework were developed according to Sgarbossa et al. (Citation2020) and begin with system design, then the analysis of HF aspects, managing OL, and finally evaluation of performance. The framework can, through this, aid decision-makers at different levels in collaborating. These steps are further explained in detail below. This is followed by an example that supports a real-life scenario in an assembly system and order picking process that illustrates how industrial engineers can apply the framework to improve the overall performance of the system (Figure ).
Figure 3. Steps for applying the framework.
The figure represents a framework to incorporate human factors with the design and management of production and logistics systems.
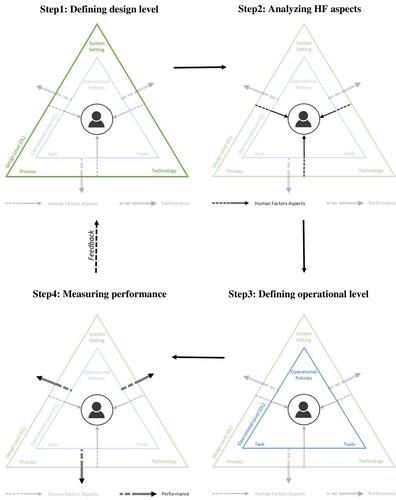
Step 1: Defining design level. Practitioners who have the authority to make decisions on system design should, when designing a new system, consider all three variables from DL (process, system settings, and technology). Practitioners need, in an assembly system, an understanding of the assembly configurations and level of automation that is required to assist the assembly system. This includes the choice of collaborative robots and instructive assistive technologies. (Peron, Sgarbossa, and Strandhagen Citation2020). Warehouse layout is, in an OP system, based on the picker-to-parts or parts-to-picker method and on the technologies that support the OP system. This includes automatic storage and retrieval systems (AS/RS), mini load, vertical lift module (VLM), carousel, or paperless picking solutions in picker-to-parts systems (de Koster, le-Duc, and Roodbergen Citation2007). Practitioners, when improving an existing system, can analyze the existing system based on the three variables, and then select the area that needs to be improved.
Step 2: Analyzing HF aspects. HF aspects are, in this stage, evaluated using the physical, mental, psychosocial, and perceptual aspects of operators. Analyzing these HF aspects can help practitioners design and manage OL (Neumann et al. Citation2021). Practitioners, when designing a new system, must analyze HF aspects through conducting an experimental study, for example analyzing the operator physical aspect in an assembly system using operator workplace ergonomic conditions and work-related ill health (WIH) (Neumann and Medbo Citation2008). The perceptual aspect was evaluated in an OP system by conducting an experimental study of the information processing ability of the order picker (Weaver et al. Citation2010). Practitioners, when improving an existing system, can analyze operator HF aspects by collecting data through using subjective studies (Stanton et al. Citation2004). For example, NASA TLX (Task Load Index) could be used to evaluate the physical, mental, and psychosocial aspects of the operator (Hart and Staveland Citation1988). The subjective workload assessment technique (SWAT) can furthermore be used to evaluate mental and psychosocial aspects using the three dimensions of time load, mental load, and psychological stress load (Reid and Nygren Citation1988).
Step 3: Defining operational level. Practitioners who have the authority to operate the system need to, based on the analysis of HF aspects, manage OL in a way that improves the HF aspect of operators. Practitioners must, when managing a new OL, consider all three variables (task, operational policies, and tools). The task, design of the workstation, and the selection of particular tools are decided in an assembly system based on the evaluation of HF aspects. For example, the physical aspect is found to be the most challenging in step 2. Practitioners therefore need to consider this HF aspect when managing the task, assembly workstation, and the tools that assist the operator in the assembly of a product (Romero et al. Citation2015). Practitioners should also do this in OP systems if the analysis of step 2 shows that the mental aspects of order pickers cause order pickers to deviate from their prescribed routes. Practitioners could therefore manage the operational level using more reliable routing policies and storage assignments, which would reduce the mental workload on the operators (Elbert et al. Citation2017). Practitioners could evaluate the HF aspects of the operator when improving an existing OL through the using of subjective and objective tools, then analyze the current variables in OL and then choose the area that needs to be improved.
Step 4: Measuring performance. Performance at OL is measured using three factors, such as productivity, well-being, and quality. The performance at OL is measured across a short-term duration, and the performance of two scenarios can therefore be measured when designing a new system. The performance at the design level is then evaluated for a long-term duration by analyzing the performance at the operation level. Practitioners could then analyze each performance element, returning to step 1 when there is a need for improvement. Practitioners can therefore, using this framework, achieve an iterative process for improving the entire P&L system by incorporating HF.
Finally, one example for each assembly and OP system is described to provide the reader with a better understanding of how to apply the framework.
Industrial engineers could, for example in an assembly system, define that decisions on paced and un-paced lines, number of operators, number of workstations, and the workstation’s configuration are DL decisions. These decisions have an impact on operator HF aspects, aspects which can be analyzed using different tools (Takala et al. Citation2010; Perez and Neumann Citation2015). Engineers could, for example, also partially measure the mental and psychosocial aspects of operators using OCRA (Occhipinti Citation1998), which measures the physical workload on the upper limb. This can be supported by subjective methods such as NASA TLX (Hart and Staveland Citation1988) and SWAT (Reid and Nygren Citation1988). Physical operator HF aspects can also be analyzed using an HR monitoring device (Smart watches), which assists in the evaluation of the energy expenditure required to perform the assembly task at each workstation (Battini, Finco, and Sgarbossa Citation2020). Engineers can, based on decisions at DL and on resulting HF aspects, introduce dynamic scheduling (job allocation) at OL by taking into consideration the physical fatigue level of the operators. Operators could therefore be scheduled such that the less fatigued operators are assigned to workstations with heavier tasks and more fatigued operators to workstations with lighter tasks (Finco, Calzavara, et al. Citation2020). Performance will ultimately show that this not only improves performance measured by productivity but also worker well-being (Battini, Finco, and Sgarbossa Citation2020).
Engineers could, in an OP system, define the decision to introduce a pick and transport robot (PTR) to assist the operator with picking tasks, as being made at DL (Lee and Murray Citation2019). Order picker HF aspects, when picking products from different rack layouts, can then be evaluated. Picking heavy products and picking products from the lower shelf can increase fatigue and the possible risk of MSD and injuries to order pickers (Calzavara et al. Citation2016). Engineers can then, from the decision made at DL and from analyzing HF aspects, make decisions on the allocation of orders (job allocation) to PTR and order pickers, which is made at OL. Tasks will be allocated in such a way that heavy products and products on the lower shelf are picked by PTR and order pickers pick the lightweight and complex products which cannot be picked by PTR. Performance will then show an improvement in the productivity of the OP system and in the well-being of the order pickers (Azadeh, de Koster, and Roy Citation2019).
6. Conclusion
The review of previous literature on state-of-the-art frameworks reveals that most frameworks are for production systems, very few being for logistics systems. No framework has, furthermore, categorised its variables using decision levels and through incorporating all the four operator HF aspects (physical, mental, perceptual, and psychosocial) and the three performance aspects (productivity, well-being, and quality) in both production and logistics systems. There is therefore a need for a new framework that incorporates human factors into P&L systems, both from a long-term design and a short-term operation perspective. This study presents a new framework by introducing the design level and operational level, through which practitioners can design and manage P&L systems. The new framework can assist practitioners in their understanding of how decisions at DL can affect operators, and then model OL around operators through using HF aspects, integrating OL into DL, and therefore improving the overall performance of P&L systems. For example, layout design in an OP system is a decision at DL and routing is a decision at OL. If these decisions are not properly modelled based on HF aspects of operators, then they can result in a substantial decrease in overall performance due to a decrease in on-the-job performance and longer recovery. Examples based on the assembly system and OP system are provided to support the new framework.
One limitation of this paper is the methodology used to validate the framework. A literature review, based on the assembly system and OP system, was provided to support the framework, the framework representing an attempt to classify into two decision levels using variables that answer ‘what’, ‘how’, and ‘with’. Future versions of the framework could consider other attributes. The work reported in this paper could also be extended to an empirical study to validate the new framework. For example, conducting multiple case studies in different companies to provide an understanding of their systems and development process based on DL and OL, so measuring performance for different DL and OL arrangements. Simulations could also be conducted, multiple case studies taking a great deal of time.
Disclosure statement
No potential conflict of interest was reported by the author(s).
Additional information
Notes on contributors

Vivek Vijayakumar
Vivek Vijayakumar is a Ph.D. candidate at the Department of Mechanical and Industrial Engineering (MTP) at NTNU (Norway). He has a master's degree in Production Development and Management from Jönköping University. His research interests include warehousing, supply chain management, human factors engineering, and material handling.

Fabio Sgarbossa
Fabio Sgarbossa has been a Full Professor of Industrial Logistics at the Department of Mechanical and Industrial Engineering (MTP) at NTNU (Norway) since October 2018. He was an Associate Professor at the University of Padova (Italy), where he also received his Ph.D. in Industrial Engineering in 2010. He is the leader of the Production Management Group at MTP, and he is responsible for the logistics 4.0 Lab at NTNU. He has been involved in several European and National Projects. He is the author and co-author of about 120 publications in relevant international journals, about industrial logistics, material handling, materials management, supply chain. He is a member of Organising and Scientific Committees of several International Conferences, and he is a member of editorial boards and associate editor in relevant International Journals.

W. Patrick Neumann
W. Patrick Neumann is a Full Professor in the Department of Mechanical and Industrial Engineering of Ryerson University, Canada. Current research, conducted at the Human Factors Engineering Lab, focuses on the design of work systems that are both effective and sustainable from human and technical perspectives. He is a Design Scientist and certified human factors professional (Eur. Erg.).

Ahmad Sobhani
Ahmad Sobhani is an Assistant Professor of Decision & Information Sciences at Oakland University's School of Business. His research focuses on the application of operations research techniques and advanced data analytics to problems in human factors modelling, manufacturing, healthcare systems, and supply chain management.
References
- Abdu, Y. A., O. Peter, and U. K. Gupta. 2016. “Lean Concepts and Methods: 3P.” International Journal of Scientific Research in Computer Science 1 (2): 20–24.
- Altarazi, S., and M. Ammouri. 2018. “Concurrent Manual-Order-Picking Warehouse Design: A Simulation-Based Design of Experiments Approach.” International Journal of Production Research 56 (23): 7103–7121.
- Azadeh, K., R. de Koster, and D. Roy. 2019. “Robotized and Automated Warehouse Systems: Review and Recent Developments.” Transportation Science 53: 917–945.
- Babu, V. R. 2012. Concepts of Production and Productivity. Industrial Engineering in Apparel Production, 1–13.
- Baines, T. S., R. Asch, L. Hadfield, J. P. Mason, S. Fletcher, and J. M. Kay. 2005. “Towards a Theoretical Framework for Human Performance Modelling Within Manufacturing Systems Design.” Simulation Modelling Practice and Theory 13 (6): 486–504.
- Bänziger, T., A. Kunz, and K. Wegener. 2020. “Optimizing Human–Robot Task Allocation Using a Simulation Tool Based on Standardized Work Descriptions.” Journal of Intelligent Manufacturing 31 (7): 1635–1648.
- Battini, D., M. Calzavara, A. Persona, and F. Sgarbossa. 2015. “A Comparative Analysis of Different Paperless Picking Systems.” Industrial Management & Data Systems 115 (3): 483–503.
- Battini, D., M. Calzavara, A. Persona, and F. Sgarbossa. 2016. “Additional Effort Estimation due to Ergonomic Conditions in Order Picking Systems.” International Journal of Production Research 55 (10): 2764–2774.
- Battini, D., X. Delorme, A. Dolgui, A. Persona, and F. Sgarbossa. 2016c. “Ergonomics in Assembly Line Balancing Based on Energy Expenditure: A Multi-Objective Model.” International Journal of Production Research 54: 824–845.
- Battini, D., S. Finco, and F. Sgarbossa. 2020. Human-Oriented Assembly Line Balancing and Sequencing Model in the Industry 4.0 Era. Scheduling in Industry 4.0 and Cloud Manufacturing. International Series in Operations Research & Management Science, vol. 289. Springer, Cham, 141–165.
- Battini, D., C. H. Glock, E. H. Grosse, A. Persona, and F. Sgarbossa. 2016. “Human Energy Expenditure in Order Picking Storage Assignment: A bi-Objective Method.” Computers & Industrial Engineering 94: 147–157.
- Bolatan, G. I., S. Gozlu, L. Alpkan, and S. Zaim. 2016. “The Impact of Technology Transfer Performance on Total Quality Management and Quality Performance.” Procedia - Social and Behavioral Sciences 235: 746–755.
- Boon-itt, S., and C. Wong. 2016. “Empirical Investigation of Alternate Cumulative Capability Models: A Multi-Method Approach.” Production Planning & Control 27 (4): 299–311.
- Bruseberg, A. 2008. “Human Views for MODAF as a Bridge Between Human Factors Integration and Systems Engineering.” Journal of Cognitive Engineering and Decision Making 2 (3): 220–248.
- Burinskiene, A. 2010. “Order Picking Process at Warehouses.” International Journal of Logistics Systems and Management 6 (2): 162–178.
- Calzavara, M., C. H. Glock, E. H. Grosse, A. Persona, and F. Sgarbossa. 2016. “Analysis of Economic and Ergonomic Performance Measures of Different Rack Layouts in an Order Picking Warehouse.” Computers & Industrial Engineering 111: 527–536.
- Calzavara, M., A. Persona, F. Sgarbossa, and V. Visentin. 2019. “A Model for Rest Allowance Estimation to Improve Tasks Assignment to Operators.” Internation Journal of Production Research 57 (3): 948–962.
- Das, B. 1998. “Development of a Comprehensive Industrial Work Design Model.” Human Factors and Ergonomics in Manufacturing & Service Industries 9 (4): 393–411.
- de Koster, R., T. le-Duc, and K. Roodbergen. 2007. “Design and Control of Warehouse Order Picking: A Literature Review.” European Journal of Operational Research 182 (2): 481–501. Elsevier
- De Nul, L., M. Breque, and A. Petridis. 2021. Industry 5.0. Retrieved from Industry 5.0: Towards more sustainable, resilient and human-centric industry: https://ec.europa.eu/info/news/industry-50-towards-more-sustainable-resilient-and-human-centric-industry-2021-jan-07_en.
- Diefenbach, H., and C. H. Glock. 2019. “Ergonomic and Economic Optimization of Layout and Item Assignment of a U-Shaped Order Picking Zone.” Computers & Industrial Engineering 138: 106094.
- Di Pasquale, V., S. Miranda, W. Neumann, and A. Setayesh. 2018. “Human Reliability in Manual Assembly Systems: A Systematic Literature Review.” IFAC-PapersOnLine 51 (11): 675–680.
- Elbanna, S. 2006. “Strategic Decisionmaking: Process.” International Journal of Management Reviews 8 (1): 1–20.
- Elbert, R. M., T. Franzke, C. H. Glock, and E. H. Grosse. 2017. “The Effects of Human Behavior on the Efficiency of Routing Policies in Order Picking: The Case of Route Deviations.” Computers & Industrial Engineering 111: 537–551.
- Figueira, S., V. C. Machado, and I. L. Nunes. 2012. “Integration of Human Factors Principles in LARG Organizations–a Conceptual Model.” Work 41 (Suppl 1): 1712–1719.
- Finco, S., D. Battini, X. Delorme, A. Persona, and F. Sgarbossa. 2020. “Workers’ Rest Allowance and Smoothing of the Workload in Assembly Lines.” International Journal of Production Research 58 (4): 1255–1270.
- Finco, S., M. Calzavara, F. Sgarbossa, and I. Zennaro. 2020. “Including Rest Allowance in Mixed-Model Assembly Lines.” International Journal of Production Research, 1–23.
- Goomas, D. T., and P. H. Yeow. 2010. “Ergonomics Improvement in a Harsh Environment Using an Audio Feedback System.” International Journal of Industrial Ergonomics 40 (6): 767–774.
- Grosse, E. H., and C. H. Glock. 2013. “An Experimental Investigation of Learning Effects in Order Picking Systems.” Journal of Manufacturing Technology Management 24 (6): 850–872.
- Grosse, E. H., and C. H. Glock. 2015. “The Effect of Worker Learning on Manual Order Picking Processes.” International Journal of Production Economics 170: 882–890.
- Grosse, E. H., C. H. Glock, M. Y. Jaber, and P. W. Neumann. 2015. “Incorporating Human Factors in Order Picking Planning Models: Framework and Research Opportunities.” International Journal of Production Research 53 (3): 695–717.
- Grosse, E. H., C. H. Glock, and S. Müller. 2015. “Production Economics and the Learning Curve: A Meta-Analysis.” International Journal of Production Economics 170: 401–412.
- Grosse, E. H., C. H. Glock, and W. P. Neumann. 2017. “Human Factors in Order Picking: A Content Analysis.” International Journal of Production Research 55 (5): 1260–1276.
- Hart, C. 1998. Doing a Literature Review: Releasing the Research Imagination. London: Sage.
- Hart, S. G., and L. E. Staveland. 1988. “Development of NASA-TLX (Task Load Index): Results of Empirical and Theoretical Research.” Advances in Psychology 52: 139–183.
- Hietanen, A., R. Pieters, M. Lanz, J. Latokartano, and J. K. Kämäräinen. 2020. “AR-based Interaction for Human-Robot Collaborative Manufacturing.” Robotics and Computer-Integrated Manufacturing 63: 101891.
- Jaghbeer, Y., R. Hanson, and M. I. Johansson. 2020. “Automated Order Picking Systems and the Links Between Design and Performance: A Systematic Literature Review.” International Journal of Production Research 58 (15): 4489–4505.
- Kadir, B. A., and O. Broberg. 2021. “Human-centered Design of Work Systems in the Transition to Industry 4.0.” Applied Ergonomics 92: 103334.
- Kadir, B. A., O. Broberg, and C. d. Conceição. 2019. “Current Research and Future Perspectives on Human Factors and Ergonomics in Industry 4.0.” Computers & Industrial Engineering 137: 106004.
- Karltun, A., J. Karltun, M. Berglund, and J. Eklund. 2017. “HTO – a Complementary Ergonomics Approach.” Applied Ergonomics 59 (A): 182–190.
- Kim, S., M. A. Nussbaum, and J. L. Gabbard. 2019. “Influences of Augmented Reality Head-Worn Display Type and User Interface Design on Performance and Usability in Simulated Warehouse Order Picking.” Applied Ergonomics 74: 186–193.
- Kolus, A., R. Wells, and P. Neumann. 2018. “Production Quality and Human Factors Engineering: A Systematic Review and Theoretical Framework.” Applied Ergonomics 73: 55–89.
- Kreutzfeldt, M., J. Renker, and G. Rinkenauer. 2018, July. The Attentional Perspective on Smart Devices: Empirical Evidence for Device-specific Cognitive Ergonomics. In International Conference on Applied Human Factors and Ergonomics (pp. 3-13). Springer, Cham.
- Krugh, M., and L. Mears. 2018. “A Complementary Cyber-Human Systems Framework for Industry 4.0 Cyber-Physical Systems.” Manufacturing Letters 15: 89–92.
- Lee, J. A., Y. S. Chang, and Y. H. Choe. 2017, July. Assessment and Comparison of Human-robot Co-work Order Picking Systems Focused on Ergonomic Factors. In International Conference on Applied Human Factors and Ergonomics (pp. 516-523). Springer, Cham.
- Lee, H.-Y., and C. Murray. 2019. “Robotics in Order Picking: Evaluating Warehouse Layouts for Pick, Place, and Transport Vehicle Routing Systems.” International Journal of Production Research 57 (18): 5821–5841.
- Lee, J. D., and B. D. Seppelt. 2009. Human Factors in Automation Design, edited by S. Nof. Berlin: Springer Handbook of Automation.
- Lummus, R. R., D. W. Krumwiede, and R. J. Vokurka. 2001. “The Relationship of Logistics to Supply Chain Management: Developing a Common Industry Definition.” Industrial Management & Data Systems 101 (8): 426–432.
- Mattsson, S., Å Fast-Berglund, D. Li, and P. Thorvald. 2020. “Forming a Cognitive Automation Strategy for Operator 4.0 in Complex Assembly.” Computers & Industrial Engineering 139: 105360.
- Meller, R. D., and K.-Y. Gau. 1996. “The Facility Layout Problem: Recent and Emerging Trends and Perspectives.” Journal of Manufacturing Systems 15 (5): 351–366.
- Michalos, G., S. Makris, P. Tsarouchi, T. Guasch, D. Kontovrakis, and G. Chryssolouris. 2015. “Design Considerations for Safe Human-Robot Collaborative Workplace.” Procedia CIRP 37: 248–253.
- Miles, M. B., and A. M. Huberman. 1994. Qualitative Data Analysis: An Expanded Sourcebook. California: Sage.
- Mosier, K. L., and U. M. Fischer.. 2010. “Judgment and Decision Making by Individuals and Teams: Issues, Models, and Applications.” Reviews of Human Factors and Ergonomics 6 (1): 198–256.
- Motmans, R., T. Debaets, and S. Chrispeels. 2018, August. Effect of a Passive Exoskeleton on Muscle Activity and Posture During Order Picking. In Congress of the International Ergonomics Association (pp. 338-346). Springer, Cham.
- Nardo, M., D. Forino, and T. Murino. 2020. “The Evolution of Man–Machine Interaction: The Role of Human in Industry 4.0 Paradigm.” Production & Manufacturing Research 8: 20–34.
- Neumann, W. P., and J. Dul. 2010. “Human Factors: Spanning the Gap Between OM and HRM.” International Journal of Operations & Production Management 30 (9): 923–950.
- Neumann, W. P., and P. Medbo. 2008. “Integrating Human Factors Into Discrete Event Simulations of Parallel Flow Strategies.” Production Planning and Control 20: 3–16.
- Neumann, P. W., and P. Medbo. 2017. “Simulating Operator Learning During Production Ramp-up in Parallel vs. Serial Flow Production.” International Journal of Production Research 55 (3): 845–857.
- Neumann, W. P., and J. Village. 2012. “Ergonomics Action Research II: A Framework for Integrating HF Into Work System Design.” Ergonomics 55 (10): 1140–1156.
- Neumann, W., S. Winkelhaus, E. Grosse, and C. Glock. 2021. “Industry 4.0 and the Human Factor – a Systems Framework and Analysis Methodology for Successful Development.” International Journal of Production Economics 233: 107992.
- Nookea, W., and A. Vanichchinchai. 2020, April. An Ergonomics-based Storage Bin Allocation for Picking Efficiency Improvement. In 2020 IEEE 7th International Conference on Industrial Engineering and Applications (ICIEA) (pp. 307-310). IEEE.
- Occhipinti, E. 1998. “OCRA: A Concise Index for the Assessment of Exposure to Repetitive Movements of the Upper Limbs.” Ergonomics 41 (9): 1290–1311.
- Padula, R. S., M. L. C. Comper, E. H. Sparer, and J. T. Dennerlein. 2017. “Job Rotation Designed to Prevent Musculoskeletal Disorders and Control Risk in Manufacturing Industries: A Systematic Review.” Applied Ergonomics 58: 386–397.
- Pasquale, V. D., C. Franciosi, A. Lambiase, and S. Miranda. 2016. “Methodology for the Analysis and Quantification of Human Error Probability in Manufacturing Systems.” IEEE Student Conference on Research and Development (SCOReD), 1–5.
- Perez, J., and W. P. Neumann. 2015. “Ergonomists’ and Engineers’ Views on the Utility of Virtual Human Factors Tools.” Human Factors and Ergonomics in Manufacturing & Service Industries 25 (3): 279–293.
- Peron, M., F. Sgarbossa, and J. O. Strandhagen. 2020. “Decision Support Model for Implementing Assistive Technologies in Assembly Activities: A Case Study.” International Journal of Production Research 1–27.
- Peruzzini, M., F. Grandi, and M. Pellicciari. 2020. “Exploring the Potential of Operator 4.0 Interface and Monitoring.” Computers & Industrial Engineering 139: 105600.
- Pimminger, S., W. Kurschl, L. Panholzer, T. Neumayr, M. Augstein, J. Altmann, and J. Heinzelreiter. 2020. “Assembly Task Analysis Using the General Assembly Task Model (GATM) on the Shop Floor.” Procedia CIRP 93: 1109–1114.
- Reid, G. B., and T. E. Nygren. 1988. “The Subjective Workload Assessment Technique: A Scaling Procedure for Measuring Mental Workload.” Advances in Psychology 52: 185–218.
- Reif, R., W. A. Günthner, B. Schwerdtfeger, and G. Klinker. 2010. “Evaluation of an Augmented Reality Supported Picking System Under Practical Conditions.” Computer Graphics Forum 29 (1): 2–12.
- Reiman, T., and P. Oedewald. 2007. “Assessment of Complex Sociotechnical Systems – Theoretical Issues Concerning the Use of Organizational Culture and Organizational Core Task Concepts.” Safety Science 45 (7): 745–768.
- Romero, D., O. Noran, J. Stahre, P. Bernus, and Å Fast-Berglund. 2015. Towards a Human-Centred Reference Architecture for Next Generation Balanced Automation Systems: Human-Automation Symbiosis. IFIP International Conference on Advances in Production Management Systems (pp. 556-566). Springer, Cham.
- Savino, M. M., C. Riccio, and M. Menanno. 2020. “Empirical Study to Explore the Impact of Ergonomics on Workforce Scheduling.” International Journal of Production Research 58 (2): 415–433.
- Schmidt, G., and W. E. Wilhelm. 2010. “Strategic, Tactical and Operational Decisions in Multi-National Logistics Networks: A Review and Discussion of Modelling Issues.” International Journal of Production Research 38 (7): 1501–1523.
- Sgarbossa, F., E. H. Grosse, W. P. Neumann, and D. Battini. 2020. “Human Factors in Production and Logistics Systems of the Future.” Annual Reviews in Control 49: 295–305.
- Sobhani, A., M. I. Wahab, and P. W. Neumann. 2015a. “An Innovative Modeling Method to Evaluate Human Factor Effects on the Performance of Manufacturing Systems.” IEEE International Conference on Industrial Engineering and Engineering Management, 130–134.
- Sobhani, A., M. I. Wahab, and W. P. Neumann. 2015b. “Investigating Work-Related ill Health Effects in Optimizing the Performance of Manufacturing Systems.” European Journal of Operational Research 241: 708–718.
- Sobhani, A., M. I. M. Wahab, and P. W. Neumann. 2016. “Integrating Ergonomics Aspects Into Operations Management Performance Optimization Models: A Modeling Framework.” IIE Transactions on Occupational Ergonomics and Human Factors 4 (1): 19–37.
- Sobhani, A., M. I. Wahab, and P. W. Neumann. 2017. “Incorporating Human Factors-Related Performance Variation in Optimizing a Serial System.” European Journal of Operational Research 257 (1): 69–83.
- Stanton, N., A. Hedge, K. Brookhuis, S. Eduardo, and H. Hendrick. 2004. Handbook of Human Factors and Ergonomics Methods. London: CRC Press.
- Takala, E.-P., I. Pehkonen, M. Forsman, G-Å Hansson, S. E. Mathiassen, W. P. Neumann, … J. Winkel. 2010. “Systematic Evaluation of Observational Methods Assessing Biomechanical Exposures at Work.” Scandinavian Journal of Work, Environment & Health 36 (1): 3–24.
- Vandergrift, J. L., J. E. Gold, A. Hanlon, and L. Punnett. 2012. “Physical and Psychosocial Ergonomic Risk Factors for low Back Pain in Automobile Manufacturing Workers.” Occupational and Environmental Medicine 69 (1): 29–34.
- Weaver, K. A., H. Baumann, T. Starner, H. Iben, and M. Lawo. 2010. “An Empirical Task Analysis of Warehouse Order Picking Using Head-Mounted Displays.” In Proceedings of the SIGCHI Conference on Human Factors in Computing System, 1695–1704.
- Wolfartsberger, J., J. H. Haslwanter, and R. Lindorfer. 2019. Perspectives on Assistive Systems for Manual. Technologies 7 (1): 12.
- Yeow, P. H., and D. T. Goomas. 2014. “Ergonomics Improvement in Order Selectionin a Refrigerated Environment.” Human Factors and Ergonomics in Manufacturing & Service Industries 24 (3): 262–274.
- Zhou, W., T. J. Armstrong, M. P. Reed, S. G. Hoffman, and D. M. Wegner. 2009. Simulating Complex Automotive Assembly Tasks using the HUMOSIM Framework. SAE Technical Paper.