Abstract
For manufacturing companies, quality management is a key feature for increasing the competitiveness, productivity, profitability, and sustainability of their systems. Quality improvement (QI) methods aim to achieve high-quality parts without reducing performance. The Industry 4.0 framework brought technological developments that cannot be used by traditional QI methods, such as Six Sigma, Lean, Lean Six, the Theory of Constraints, and Total Quality Management, which are widely used in manufacturing companies. The need for higher manufacturing sustainability and market requirements has led to the search for alternative QI methods with superior performance to traditional QI methods such as Zero-Defect Manufacturing (ZDM). The current paper is a position paper with a goal to present the ZDM approach and providing a clear definition about ZDM to align everyone in one common understanding of ZDM. Many researchers and manufactures are skeptical about ZDM, therefore, numerous argumentative questions have been created and answered, to convince them why they should migrate from traditional QI methods to ZDM. The migration to ZDM has already started, to support this statement numerous facts from the literature have been presented. Finally, several directions were identified, demonstrating that there is still plenty of room for research in several domains.
1. Introduction
Poor product quality can have an impact on many levels, from direct economic losses to the ecological impact of wasted resources. Quality management is therefore vital for manufacturing companies that want to maintain or increase their financial and operational performance (Kumar, Maiti, and Gunasekaran Citation2018). The effects of poor quality can also occur at the social level, where the image of the company is damaged by its poor-quality products and customer dissatisfaction (Jun, Chang, and Jun Citation2020). To achieve high-quality parts with minimum performance loss, manufacturers must implement at least one quality improvement (QI) method (Özcan, Akdoğan, and Durakbasa Citation2021). Although traditional QI methods, such as six sigma (SS), lean manufacturing (LM), theory of constraints (TOC), and total quality management (TQM), have been widely used by manufacturers for more than three decades, there is still much ground to cover. This is because such methods were created based on the manufacturing standards of the time without knowledge of the contemporary technological advancements under the framework of Industry 4.0 (Psarommatis et al. Citation2020) or in general data-driven approaches. A recent state-of-the-art paper presented traditional product QI policies, depicting their characteristics, enabling factors, and barriers, and concluding on the need for alternative QI methods, because of the several disadvantages of traditional QI methods that affect their performance and efficiency (Psarommatis et al. Citation2020). The contemporary technologies open a new horizon for manufacturers to achieve goals that in the past would have sounded like fiction. Such technologies include machine learning, deep learning, and artificial Intelligence (AI). Another set of factors that boost Industry 4.0 technologies even further is the tremendous increase in the computational capacity of computer systems, the advanced capabilities of data management systems, and the significant drop in the price of sensors (Mourtzis, Vlachou, and Milas Citation2016; Chien, Hsu, and Chen Citation2013; Kuo et al. Citation2013; Choi et al. Citation2012). All these factors and market requirements have brought to life a concept called Zero-Defect Manufacturing (ZDM). ZDM is not a new concept; it was first introduced in 1965 by the U.S. army during the Cold War (Quality and Reliability Assurance Handbook Citation1965). The concept of ZDM aims to go beyond the traditional QI approaches because it utilises the full potential of modern, advanced, data-driven technologies such as Industry 4.0 technologies (Psarommatis et al. Citation2020) or big data. One of the key aspects of ZDM’s increasing popularity is that manufacturers actually want to implement it because they see the potential benefits (Lindström et al. Citation2019). ZDM is not an approach created from scratch; it embraces numerous ideas and concepts from the traditional QI methods. For example, six sigma’s core concept is that 3.4 defects occur per 1 million products, or almost zero; the same idea is embraced by ZDM, but the difference is how it is achieved/computed and potentially the improvement of that (statistical vs. full inspection).
The necessity of evolving from traditional QI approaches comes from the change of lot sizes, from mass production to mass customisation and lot size one. The constant pressure to reduce manufacturing cycles for fast delivery to the customer creates factors that delay production and mainly inspection activities on the spot (Ferreira et al. Citation2020; Sousa, Silva, and Machado Citation2022). This creates the need to break data silos and achieve the full integration and interoperability of manufacturing systems, demonstrating that current quality-oriented approaches to manufacturing do not fulfil the Industry 4.0 vision. In that sense, the holistic approach of ZDM is key for manufacturing companies that wish to embrace Industry 4.0 and produce products with zero defects and waste efficiently.
1.1 Paper scope
The current paper is a multi-purpose position paper around the topic of ZDM. Since contemporary ZDM is a new topic there is a lot of confusion around the topic about what ZDM is and how it can be implemented. This creates a significant drag towards ZDM understanding and implementation. Therefore, the first goal of the paper is to provide a clear understanding of ZDM, explain all the aspects of ZDM and how it can be implemented. This will educate and align researchers and manufacturers to the ZDM approach which is critical for making manufacturing systems that are defect free and at the same time increase their sustainability. Furthermore, the definition of ZDM is presented as an outcome of a collaborative work from an ongoing CEN-CENELEC Workshop Agreement (CWA) in which the authors participate. The aim of the ZDM definition is to align everyone together and have a clear view of ZDM.
The vast majority of researchers and manufacturers are using traditional QI approaches for decades and therefore they are more skeptical about migrating from traditional QI to ZDM. In this context, the current paper aims to provide numerous arguments and literature facts in order to convince researchers and more importantly manufacturers why they should migrate from traditional QI to ZDM. Stressing the fact that the benefits and opportunities from ZDM implementation are numerous and that ZDM can offer higher manufacturing sustainability. Furthermore, another goal for the current position paper is to present a series of facts from literature, industry and academia that showing that the migration from traditional QI to ZDM has already begun. Finally, numerous topics that are critical for successful and efficient implementation are identified and are suggested as future research required for making ZDM approach more mature.
1.2 Paper methodological approach and structure
The current paper was produced based on specific methodological steps taken from the literature that are needed for position papers (Gaspar et al. Citation2012; Vargas et al. Citation2018; Pinto and Fernandes Citation2021; Allgöwer et al. Citation2019). The first step is to explain in detail the proposed approach, in the current case ZDM. The next step is to create some counter-argument questions that someone against the proposed approach would ask and provide detailed answers based on the literature. The next step is to provide facts that illustrates and support the use of the proposed approach. Finally, based on the research performed identify future steps and directions that will help on the improvement of the proposed approach.
The organisation of the article is as follows: In Section 2, the definition of ZDM is provided from an ongoing CEN-CENELEC Workshop Agreement (CWA) in which the authors participate. Furthermore, the framework of ZDM is presented as well to explain in detail the ZDM approach and put an end to the misalignments that exist in the research and industrial communities. Manufacturers tend to be skeptical and conservative in the sound of ZDM, therefore, in Section 3 some arguments why industries should adopt ZDM are presented. Section 4 presents some facts that illustrate that the migration from traditional QI to ZDM has started and some key figures are presented as well. Section 5 presents some of the identified fields of research that will allow the efficient and successful implementation of ZDM. The key areas that more research is required are presented with explanations and literature examples in order to support authors’ claim that those areas are crucial for ZDM. Section 6 summarises the scientific contribution of the paper and presents some concluding remarks.
2. Towards standardisation of ZDM
ZDM is a viable concept of sustainable manufacturing, but the research already conducted in the field has considered ZDM from a different perspective. The necessity to standardise the terminology in the ZDM field is acknowledged by field experts (Blind Citation2016). Therefore, the current paper provides a clear definition of ZDM to be used across the scientific community, thus eliminating any misunderstandings that exist.
‘ZDM is a holistic approach for ensuring both process and product quality by reducing defects through corrective, preventive, and predictive techniques, using mainly data-driven technologies and guaranteeing that no defective products leave the production site and reach the customer, aiming at higher manufacturing sustainability.’
This definition is a result of a CEN-CENELEC ZDM terminology standardisation process which will be further detailed in Section 4.2.
Manufacturing environments are characterised by high uncertainty, and therefore, defects are an unavoidable phenomenon. Defects ‘occur’ when non-conformance to product specifications is detected. The only possible action is to minimise defects as much as possible without compromising the performance of the system. The term ‘Zero-Defect’ refers to the number of defects at the end of the manufacturing process of a product, or the end of manufacturing life (EOML). To achieve this, any quality strategy must be applied to all the parts produced without sampling performed. Figure presents the four individual strategies that comprise ZDM, namely Detect, Predict, Repair, and Prevent, alongside the corresponding connections as defined by Psarommatis et al. (Citation2020). The four ZDM strategies are divided into two categories, namely triggering factors and actions. The triggering strategies are Detect and Predict, which are responsible for identifying a quality issue. Notably, the triggering ZDM strategies must be applied for every product that is produced. Detect can be divided into physical and virtual detection of a defect that has already occurred, whereas Predict is responsible for predicting when a defect will occur in the near future. Those two triggering strategies alone cannot increase the quality of the production. For that reason, there are the other two ZDM strategies of Repair and Prevent, which are the actions that can be taken in the event of input from the triggering ZDM strategies. This means that ZDM strategies must always be used in pairs, using one triggering strategy and one action strategy. By doing so, the following ZDM strategy pairs are formed: Detect–Repair, Detect–Prevent, and Predict–Prevent.
Figure 1. Generic ZDM framework (Psarommatis, May, et al. Citation2020).
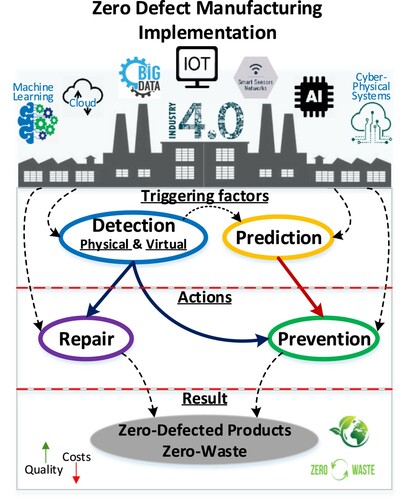
The first two ZDM pairs (Detect–Repair and Detect–Prevent) are not new, having existed in the manufacturing domain for decades; however, modern advanced data-driven technologies allow a more efficient implementation. Detect–Repair is referred to in the literature as a corrective approach, where something is already faulty and corrections are required. Detect–Prevent is referred to in the literature as a preventive approach, where data are used from the production to avoid future defects. On the other hand, Predict–Prevent is a fairly new concept that relies on advanced data-driven approaches for predicting when in the future a fault will be created, thus enabling it to be prevented before it occurs. This strategy follows the philosophy of ‘doing things right the first time,’ whereas the other two involve acting after the fault. In ZDM, a fault is called a defect, which is defined as ‘non-fulfilment of a requirement related to an intended or specified use’ (ISO 9000 Citation2005). ZDM follows two parallel approaches: the product-oriented one and the process-oriented one, which both lead to the same result of defect minimisation and zero defects at the end of the production line. Figure illustrates the two different approaches as presented by Psarommatis et al. (Citation2020). Each approach characterises the starting point of the analysis. In the product-oriented approach to ZDM, the starting point is product quality, and the measured quality is checked against specifications. If the product quality meets the standards, the corresponding machine’s status is deemed healthy; if it does not, then the corresponding machine needs either maintenance and/or (re)calibration or tuning based on the defect data. By contrast, in the process-oriented approach, the health status of the machine is monitored; if it is good, then the produced product will be of good quality. In both cases, the implementation of ZDM is a closed-loop process that has its feedback loop either on the product or on the process quality, leading to maintenance and/or machine (re)calibration and/or tuning in case of low quality. The selection between product and process-oriented ZDM is not an easy and straight forward process and therefore investigation is required for selecting the proper ZDM approach for a specific use case (Psarommatis and Kiritsis Citation2021b).
Figure 2. ZDM approaches (Psarommatis, May, et al. Citation2020).
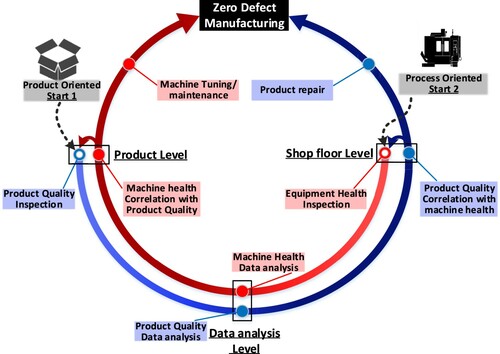
To conclude, ZDM is not composed of one method but rather a set of methods capable of dealing with any type of production system. The proper use of ZDM involves using more than one method – namely the ZDM pair strategy – at each stage to ensure a holistic view of the quality at a given manufacturing stage. As mentioned at the beginning of the chapter, defects are unavoidable events, and therefore, their corrective actions are required to mitigate them.
3. Current challenges to ZDM adoption
To emphasise and analyse the advantages of ZDM compared with traditional QI methodologies and to provide arguments that are necessary for guiding manufacturers in the adoption of a ZDM approach, this section provides several literature backed answers to common questions that may be asked about ZDM approach:
Is ZDM so different from current QI approaches in manufacturing? (Section 3.1).
Is there room for improvement for companies that already use six-sigma? (Section 3.3).
How complex is ZDM implementation - is the framework simple enough for it to be widespread? (Section 3.4).
Is poor performance expected by inspecting 100% of the produced parts? (Section 3.6).
What is the responsiveness of ZDM solutions? (Section 3.7).
How can such a large volume of data be processed in the company? (Section 3.8).
Can traceability in the supply chain be improved with ZDM? (Section 3.9).
3.1 Comparison of ZDM with current QI approaches in manufacturing
ZDM has evolved from current preventive and corrective actions based on data collected from past production and defects to the capacity to predict when defects will occur and to learn from them by analysing both the past and the present and attempt to avoid them before even they created. To perform the comparison authors have used the results from a recent (2020) review article that analyses all the traditional QI methods (Psarommatis et al. Citation2020). Traditional QI methods analyse only the past to improve the future, which in many cases is still a human-based activity. Therefore, there is a loss of potentially important information from the present. Not analysing the present creates an inertia between the occurrence of an event and the identification of an improvement linked to it. In this sense, ZDM provides an additional predictive layer to traditional approaches and looks for faster, more reliable, and adaptive methods of ensuring defect-free products by looking at data from both the process and the product (Yamaguchi and Yamashita Citation2021). In fact, LM, SS, Lean Six Sigma (L6S), the TOC, and TQM do not learn from defects but rather simply remove them, and neither do they utilise the full capacity of Industry 4.0 technologies.
Traditional quality approaches are suitable for mass production since they focus on the improvement of processes from standardisation. While standardisation from every perspective is essential for QI (and thus for ZDM), ZDM looks for benefits with diversity/variability. The key point is to ensure that no defective product reaches the customer while maintaining the ability to produce and inspect products using sustainable methods, even for highly customised products (Mourtzis, Angelopoulos, and Panopoulos Citation2021).
Contrary to traditional approaches, ZDM reaches for the causality link between defects and its cause to achieve multilevel-control closed loops using past and real-time data; moreover, it must be integrated into the production process right from the beginning as opposed to trying to address the issues at a later stage (Psarommatis et al. Citation2020).
Additionally, contrary to other approaches, ZDM is also conceptually simpler to implement, since the defined ZDM framework is easier to be understood by people who are not experts in ZDM (Figures and ). For more details see section 3.4.
ZDM is the evolution of other methods. It includes some of the core concepts of traditional QI methods but the implementation is changing. The standard SS methodology embraces ZDM as one of its core concepts, defining it as allowing a maximum of 3.4 defects per million products, since achieving zero defects in a real context is practically impossible (Guleria et al. Citation2021). To achieve this, the evolution of Industry 4.0-enabling, data-driven innovation leads to an easier and more efficient implementation of the ZDM concept, due to the availability of the required amount of data for techniques such as machine learning to work properly (Nazarenko et al. Citation2021). ZDM is also interested in continuous improvement (to reach zero defects) and can also make use of continuous (quality) improvement (CI) tools such as DMAIC, FMEA, and SPC. To summarise ZDM in a holistic approach the utilises the best practices of all the QI methods while it exploits the notion of defect prediction something that is not the case of the traditional QI.
3.2 Cost considerations when moving to ZDM
Traditional QI methods (i.e. SS, LM, L6S, the TOC, and TQM) were developed decades ago to serve the mass production paradigm. Currently, the manufacturing landscape has significantly changed and shifted from mass production to mass customisation or in some cases personalisation (Mourtzis et al. Citation2013; Mourtzis, Doukas, and Psarommatis Citation2015; Zhang et al. Citation2019). At the same time, the product life cycle has been reduced significantly to capture market needs (Psarommatis and Kiritsis Citation2018). In the era of mass customisation and personalisation, batch sizes have been significantly reduced, a consequence of which is an increase of defects during production as a result of less time being available for optimising the production and implementing traditional QI (Psarommatis, Gharaei, and Kiritsis Citation2020). The traditional QI methods were designed using the ‘corrective’ paradigm as driver, meaning that in order to be applied, there must first be a problem in order to solve it (Psarommatis et al. Citation2020). ZDM comes to fill this gap using the ‘prediction’ strategy, which identifies the problem before it happens, providing the time for reacting and preventing the problem from being created. ZDM, as mentioned in Section 2, is a set of methods for addressing quality issues in a holistic approach, meaning for example that if the ‘prediction’ of a defect is not successful and the defect cannot be prevented, then repair actions on the defective part will be applied to mitigate the defect. This concept of addressing quality issues at multiple levels has a significant positive impact on the measured KPIs. The improved KPIs are an outcome of multiple effects caused by ZDM approach implementation. Because all products are inspected and no defective products leave the factory, customers are satisfied and the likelihood of re-ordering is increased; furthermore, the image of the company is maintained or increased simultaneously, and these two factors constitute key factors in the profitability of the company (Zhang, Vonderembse, and Cao Citation2009; Piechota, Glas, and Essig Citation2021).
Furthermore, because of 100% inspection, no defective products will leave the factory, thus reducing the negative environmental impact of production is reduced while not increasing the production burden. More specifically, in the event that a defective product leaves the factory and reaches the customer, three possibilities can occur. First, the customer asks for a refund and does not order again, forcing him or her to order from another supplier, meaning that production and transportation costs are losses for the company in addition to the environmental impact of ‘useless’ transportation. Second, the customer asks for a new product, meaning the company must make a new one (extra production burden) and ship it again to the customer, at the same price but double the workload and environmental impact. The third possibility is similar to the second one with the difference being that the customer ships the product back to be repaired; the company will ship it back to the customer, which again entails extra production burden as well as tripled environmental impact and transportation costs.
Combining ZDM pair strategies is the solution to all of the aforementioned problems, which enables catching the defects as soon as possible to reduce the losses, negative environmental impact, and production burden. This is achieved because in ZDM, defect events are treated differently in that repairing is performed whenever possible; moreover, most importantly, prevention measures are implemented in light of defect prediction or detection to avoid future potential defects, thus ensuring things are ‘done right the first time.’ Getting things right at the first attempt is a key advantage of ZDM compared with the other QI methods. The implementation of ZDM starts one step before all the other QI methods, which is why it can achieve better results. In other words, the implementation starts before the defect is generated, and therefore, there are no losses compared with traditional QI methods, which start when a defect is observed. At this point, someone might ask the following question: ‘Could inspecting 100% of products have a negative impact on production performance?’ The answer is yes and no; the performance in some cases might be slightly worse but those losses would be significantly lower than those arising from a defective product at the customer end. To contradict this slight performance loss, Deloitte (Citation2015) reported that 36% of customers wish to purchase a customised product, with 50% stating they would accept a longer period of time for acquiring it (Deloitte Citation2015). Additionally, modern, advanced, data-driven technologies offer the capabilities for supporting the ZDM approach but also maintaining the performance of the system at acceptable levels (more details are provided in Section 3.6).
3.3 Why should manufacturers implement ZDM if they already have near-zero defects?
In general, near-zero-defect production is rare, and when achieved, it is for products that have been thoroughly studied, with must knowledge and time spent behind the scenes. To achieve this state using traditional QI requires years of observation and corrective actions from manufacturers, and most importantly that nothing is changed about the process or the product. For such products, ZDM might seem unnecessary, but what if the product changes? As explained in Section 3.2, the product life cycle has reduced significantly and customers require customised products; therefore, at some point, the process or product will change and all the knowledge and improvements acquired over the years will not be applicable to the new situation. This is due to the fact that traditional QI methods do not learn from defects; they simply remove them from the production, ignoring the valuable information that can be acquired (Psarommatis et al. Citation2020). The ZDM approach, on the other hand, uses advanced data-driven technologies and can thus learn from the defects or other types of failures and increase the resilience and flexibility of the production system (Lindström et al. Citation2019). This means that in light of a change, the quality of the manufacturing process will not be affected and significant time will be saved from not applying the traditional QI methods to the new manufacturing circumstances. To summarise, manufacturers that have production stages with near-zero defects can also benefit from the adoption of the ZDM approach. They will increase the resilience of their production, making it flexible to adapt to changes, thus reducing the time required to bring quality at the designed levels in the event of change.
3.4 Is ZDM easier to implement?
The ZDM framework, illustrated in Figure , as developed by Psarommatis et al. (Citation2020), is designed to be easy to understand and conceptualise. It does not require someone to be trained or to possess special knowledge to understand it. Despite its simplicity, the concepts described include all the required information for the successful implementation of ZDM. a critical failure factor of traditional QI methods is the lack of implementation framework as stated in the literature (Pinho and Mendes Citation2017; Pacheco et al. Citation2015; Psarommatis et al. Citation2020). The framework presented in Section 2 (Psarommatis et al. Citation2020), resolves the problem that exists in traditional QI methods. Another critical failure factor of traditional QI methods is that they are very complex, and moreover, whoever wants to use them must be an expert to understand them (Psarommatis et al. Citation2020). Based on the literature review conducted by Psarommatis et al., a large variety of tools exist for implementing traditional QI (e.g. DMAIC, VSM, 5S, JIT, and Kaizen); more specifically, 99 appeared the most, which is by itself a barrier for implementing the QI for those who are not specialists in the field. To overcome this barrier, practitioners must invest a considerable amount of time to learn and understand the available tools and how they can be applied to each QI method and then proceed with the implementation (Psarommatis et al. Citation2020). ZDM is implemented using advanced data-driven technologies that do not rely only on experts for implementing the QI. Additionally, the lack of top management commitment is another reason why traditional QI methods fail; specifically, due to the complexity of the methods, top management is unable to understand the needs for implementing a QI.
At this point, it should be clarified that implementing each pair of ZDM strategies is not an easy task and but ZDM requires significantly less resources compared to traditional QI methods in both time and economical aspects, which makes it more friendly for the top management of a company. Because of ZDM’s conceptual simplicity practitioners do not have to spend a considerable amount of time to understand the tools before they are ready to apply them as it happens to traditional QI methods (Psarommatis et al. Citation2020). This saves a significant amount of time in the implementation phase of the QI which can be also translated to cost saved in terms that the production will operate less time under ‘high’ defect rate. Additionally, traditional QI methods are implemented once there is a problem and they attempt to resolve it, which can be translated into a significant cost. In ZDM that cost does not exist because ZDM is implemented in order to avoid defects and assure the product quality. The most critical resource that ZDM requires are data, which in most cases industries have already have them, but not exploit them, or they can easily collect the required data, using the capabilities that modern technological achievements are offering (Farooqui et al. Citation2020). Furthermore, in ZDM the cost for inspecting all the parts is balanced from the significantly reduced or eliminated cost of poor quality which can be devastating for a company (Cheah, Amirul, and Taib Citation2011; Tannock and Saelem Citation2007).
There are three main pairs of ZDM strategies as stated in section 2, therefore, when designing the implementation of ZDM, it is clear what to do: if a defect is detected then it must be repaired if possible. In cases where repair is not possible, a preventative action should be taken to avoid future defects. The ultimate tool of ZDM is defect prediction: if a defect is predicted that will occur in the near future, then manufacturers can act before it has been generated and thus prevent it. Finally, it is recommended that manufacturers combine some of the three pairs to achieve higher efficiency and increase sustainability. To conclude, the ZDM approach as it is defined can be considered a user-friendly method because it relies on logical and understandable statements for planning the implementation of QI. Furthermore, since there is a clear framework there is the room for development of methodologies to properly design manufacturing systems for achieving ZDM, as presented by Psarommatis (Citation2021).
3.5 Is it realistic to claim that ZDM is feasible?
Real manufacturing environments are characterised by a high level of uncertainty, which makes it nearly impossible to reach actual zero defects, meaning that no defects occur during the manufacturing process. One popular argument for ZDM not being feasible is the fact that variability constantly threatens the production system. Incoming raw material, for example, has an intrinsic complexity that brings variability and a probability of a defect appearing and propagating through the production line. The goal of ZDM is to reduce defects as much as possible, and it is compulsory that all products that leave the factory are defect free. In other words, defects are kept in the factory, since it is virtually impossible to have incoming material, in-process material, and final products defect free every time. This will have a significant impact on the sustainability of the corresponding manufacturing system. This is due to the fact that if a defective part is sent to a customer, the customer will return the part and either ask for a new one or never order again, which will both impact the manufacturer. ZDM prevents this negative side effect by ensuring that no defective product leaves the factory (Fraile et al. Citation2019). The usage of digital platforms can help to democratise ZDM through the manufacturing domain and assist companies in the adoption of the ZDM approach.
Additionally, advanced data-driven technologies are emerging from R&D projects (see Section 3.1), revealing that implementations are reaching cost-effective values to be implemented in either SMEs or large enterprises.
3.6 Manufacturing system performance vs. 100% inspection
To determine whether a specific lot or another amount is acceptable, 100% inspection is performed in manufacturing as part of ‘acceptance inspection.’ It is more commonly deployed to satisfy legal or political requirements when the consequence of appearance of a defect is severe, either for safety reasons (e.g. the aviation industry) or contractual monetary penalties. Contrary to occasional misinterpretation, 100% does not refer to all quality characteristics of a part (or assembly) being inspected. Instead, it refers to a certain characteristic being 100% inspected on every manufactured part. Inspecting 100% of production is becoming key for smart factory to enable the early detection of defects and avoid its propagation by the proliferation of in-process inspection, also known as in-line inspection or quality control (Chiariotti et al. Citation2018).
The use of this level of inspection brings several disadvantages, such as a substantial cost increase, either by requiring additional personnel or measuring devices to perform the inspection or by consuming time. Furthermore, 100% inspection is unreliable to implement when destructive inspection methods must be used, such as when legal requirements are imposed.
However, the most significant disadvantage of 100% inspection is the fact that unless the inspection method is 100% effective, some defects will continue to appear since inspections errors can occur due to imperfect measurement systems (Feng and Kapur Citation2006). This is especially critical for human-based inspection (such as visual inspection), where the ability for defect detection can vary with tiredness, attention, laziness, or stress. This bias can be largely avoided with the use of faster, more accurate, and more reliable inspection systems, such as (but not exclusive to) machine vision and laser systems (Sousa et al. Citation2020).
Although several generic models for the cost of quality were already developed, it is still arguable if the optimum level for quality equals zero defects or not (Schiffauerova and Thomson Citation2006).
3.7 Responsiveness and accuracy of ZDM compared with traditional QI
Traditional QI methods utilise historical data in an attempt to improve the future without considering the present production status. This means that real-time data are not utilised, and therefore, critical information about a defect may be lost. This creates a significant amount of inertia between the time of the quality event detection and the time of assigning the mitigation action (Psarommatis et al. Citation2020). This has an effect of a delay in the response time in case of a quality issue as well as reduced accuracy of the QI method.
ZDM resolves these issues because it utilises both historical and real-time data, which are crucial for back-tracing the cause of a defect and learning from that event. ZDM can target the time of defect occurrence exactly and act immediately, whereas traditional QI cannot act immediately. Through utilising both historical and real-time data, ZDM achieves quick response times as well as more accurate solutions with fewer resources, thus increasing the sustainability of the production system.
Furthermore, because ZDM requires advanced data-driven approaches to implement, it takes advantage of another technology called a semantic framework. With the use of ontologies and other technologies, the semantic framework can enrich data with context and thus extract useful knowledge from existing data, which in other cases would remain unknown (El Kadiri and Kiritsis Citation2015; Efthymiou et al. Citation2013). This type of technology can boost the responsiveness and accuracy of ZDM even higher, something that traditional QI methods cannot take advantage of because they are not designed to be data-driven and utilise the Predict–Prevent pair to such an extent.
3.8 The role of big data to ZDM
Current QI approaches such as SS do not perform well in complex systems where large amounts of data must be analysed. In the manufacturing context, data may come from different sources and have different formats, semantics, and quality (Kuo and Kusiak Citation2019). The amount of data is also continuously increasing since processes are increasingly digital and information technology is evolving. Information and computer systems are increasingly integrated, and emerging technologies such as the Internet of Things, AI, big data, and cloud computing are being used to collect, store, process, and visualise manufacturing data (Mourtzis, Vlachou, and Milas Citation2016). ZDM uses data-driven technologies by applying sensors and inspection devices along the production line to enable process monitoring, visualisation, and control. Data can also be merged with additional datasets from different phases of the product life cycle, such as product design (e.g. dimensional, geometric, and surface characteristics), to enable advanced data-based techniques such as AI and big data to find hidden complex patterns, seemingly invisible to humans (Majeed et al. Citation2021). Although ZDM is mainly oriented toward predictive quality analysis, data-driven technologies are being implemented either for defect prevention or prediction (Tao et al. Citation2018), on top of product quality data such as surface roughness using machine vision applications. The aim is to detect, diagnose, and address product defects, not only by identifying the defect (and the defective product) itself but also by controlling or eliminating the factors that led to the defect. Data-driven technologies can also be used for the early detection of product defects and the identification of the root cause using big data; the classification of conditions that lead to a quality defect and the corresponding probability using machine learning; the identification of the most influential parameters for product quality using Bayesian inference; root cause identification and analysis of product failure using big data; monitoring of process quality; and final dimensional verification of components (Sousa et al. Citation2020).
The result of the use of data-driven technologies in manufacturing is the ability to effectively learn from each quality issue and address the root cause in a cost-effective manner, thus avoiding recurrence in the future.
3.9 The road to zero defects leads to an improvement in traceability
Suppliers and customers need to tighten the bonds between them in order to achieve end-to-end traceability of defects in a supply chain. Technologies like blockchain are being tested for this purpose and RFID is establishing itself as the most prominent mean (Cui, Hu, and Liu Citation2018). Products need to be traceable to applied manufacturing methods, conditions (machine parameters), and performed inspection activities. ZDM can provide the tools for both suppliers and customers to be able to trace back to the root cause of an undetected defect that was mistakenly delivered to the customer (Sousa et al. Citation2020); such as a construction company that requires multiple suppliers (e.g. concrete, steel tubes, and slabs for cladding) that may be tested before being applied at the construction site. A certain lot can be rejected based on nonconformity to a required standard and the construction company can then alert the supplier of a spotted defective lot and request another lot in return. Although the relationship could be somehow damaged, the supplier can now trace back the lot to manufacturing conditions that may have led to the defective product appearing. This traceability is enabled by data-driven technologies, relying on real-time collected data from the workshop and its storage. AI algorithms can be applied to analyse the root cause of the defect and enable the process to be tuned to prevent such defects appearing again, thus providing a closed control loop that goes beyond the factory walls and reaches the customer (Sousa et al. Citation2020). This will provide a different level of relationship between suppliers and clients, where the trust may increase considering all this ZDM potential.
4. The ZDM revolution has begun: some facts and figures
This section presents some of the facts that illustrate the interest and adoption of ZDM approach from industrial and academic communities. In short, much work and research has been conducted and even more is underway. Currently, the manufacturing domain is in a transitional state due to the capabilities that new production technologies and methodologies are offering (Onu and Mbohwa Citation2021; Enyoghasi and Badurdeen Citation2021). Driven by market needs and environmental circumstances, manufacturing companies must shift toward being more efficient and flexible with a smaller environmental footprint in production; in other words, they must become more sustainable (Krishna and Srikanth Citation2021). The urgent need for more sustainable manufacturing processes has been raised by numerous researchers (Gupta, Kumar, and Wasan Citation2021; Enyoghasi and Badurdeen Citation2021). To reach higher levels of sustainability, manufacturing companies must investigate the reasons behind waste creation and how to eliminate it. This process is performed using QI methods, it is evident that traditional QI methods cannot cope with the new requirement – namely higher sustainability – of the manufacturing domain, and that a replacement method is required. ZDM seems to be a viable replacement for the traditional QI methods (Psarommatis et al. Citation2020). The subsections that follow illustrate that a great interest exists in the ZDM approach, and also demonstrate a transition momentum toward ZDM because of an exponential increase in the interest in ZDM and because standardisation processes have already begun to bring ZDM to the industrial level. ZDM can increase the sustainability of a manufacturing system because reducing the number of defects and disruptions to production reduces the negative environmental impact of the production. On the other hand, because advanced data-driven technologies allow for more efficient quality assurance, the production process can become more economically sound (Majeed et al. Citation2021).
4.1 ZDM-oriented EU-funded projects
The European Commission’s promotion of zero-defect–oriented projects dates back to 2011, with several projects under the Seventh Framework Program for Research (FP7) within the field of Nanosciences, Nanotechnologies, Materials, and New Production Technologies (NMP), namely MIDEMMA, IFaCOM, MuProD, and MEGAFIT.
Nonetheless, a great impulse was given in 2016 under the H2020-EU.2.1.5.1. – Technologies for Factories of the Future program for zero-defect strategies at the system level for multi-stage manufacturing in production lines, resulting in projects such as GO0D MAN, Z-Fact0r, ForZDM, ZAero, STREAM-0D, Z-BRE4 K, DAT4.ZERO, and InterQ, forming the 4ZDM cluster that joins these nine projects under the same topic. The interest was reinforced in 2019 with the projects QU4LITY and ZDMP under the topic of Digital Manufacturing Platforms for Connected Smart Factories. In addition, skill enhancement programs have targeted zero defects since 2015, with projects such as MICROMAN and DIGIMAN4.0.
The European Commission’s commitment to improving process efficiency and product quality through ZDM initiatives has thus far resulted in an investment of almost 120 million euros. Table summarises the budgets and the timeframe of all the ZDM-oriented EU-funded projects. Table presents a short description of each project and also provides the links for the webpage of each project.
Table 1. European Commission-funded zero-defect-related projects: programs, budget, and timeline.
Table 2. EU-funded projects description.
4.2 CEN/CENELEC DIN standardisation process for ZDM
There are few or no definitions for the concepts associated with ZDM. The 4ZDM cluster has already prepared a glossary around ZDM that can be used, but standardised terminology will facilitate the exchange of information between different companies and drive further research around the topic. In this direction, a CEN-CENELEC Workshop is underway to establish a terminology standard, including all relevant definitions in the field of ZDM. The Workshop promotes the common understanding of a group of field specialists, including the authors of the current article, who are also cooperating in some of the Zero-Defect oriented projects.
A CWA is a normative document that is developed and approved in a CEN workshop. As it is a specification (as opposed to a standard), a CWA carries no obligation to be adopted at the national level. Since it can be used as the basis for the preparation of a complete standard, it can be the first step toward an ISO or EN standard. A CWA can be used to support a short time to market, promotes the dissemination of innovative solutions and research project results, and creates trust and market acceptance.
4.3 Literature momentum toward ZDM
A critical factor demonstrating the great interest in ZDM is the trend of the number of research papers published specifically on this topic. To identify and demonstrate the research activity on ZDM, a search was performed in the major engineering-oriented scientific databases. More specifically, a search was performed in the following databases: Scopus, IEEE, Compendex, Inspec, Web of Science, Google Scholar, and Science Direct. The search criterion was an exact match of the phrase ‘Zero-Defect Manufacturing’ or variations thereof, such as ‘Zero-Defect Manufacturing,’ in the title, abstract, or keywords. After removing duplicate and irrelevant papers, 198 papers remained for the analysis of the ZDM domain. Figure illustrates that a great momentum exists toward ZDM, which proves that there is great interest in ZDM. From 2008 to 2020, there was a clear exponential upward trend for ZDM, with research works published between 2018 and 2020 accounting for 46.97% of the total articles published directly on ZDM. At this point, it should be mentioned that for 2019 and 2020, the number of publications was lower compared with 2018, but this is a side effect of the COVID-19 pandemic, which slowed research activities.
4.4 Dedicated ZDM academic courses
For the manufacturing domain, an important step in migrating from traditional QI to ZDM is via academic institutions. Specifically, academic institutions must educate and prepare future engineers on the concept of ZDM. Students must learn the ZDM approach alongside the traditional QI methods to understand the differences and the potential of ZDM. Once future engineers possess the knowledge of ZDM acquired from the beginning of their studies, they will be ready to apply it in manufacturing companies and help in the global task of making the manufacturing domain more sustainable. To this extent, some academic institutions have already created dedicated courses to ZDM. In general, Scandinavian countries are well-known globally for being pioneers on sustainability issues. It is also proven that Norwegian and Finnish academic institutions have already introduced courses regarding ZDM. Table illustrates the only courses that currently exist related to ZDM. ZDM is at an early stage, and once there is a clearer understanding of what it is, more courses will be introduced. Furthermore, the standardisation process described in Section 4.2 will help in this direction.
Table 3. ZDM courses.
4.5 Other sources justifying the ZDM trend
This section is devoted to presenting some information outside of the academic environment to demonstrate that the ZDM topic is discussed by industrial companies and other communities as well. Not many resources discuss the ZDM matter publicly because it is still immature, no standards exist yet, and there is confusion over what ZDM is. Two cases illustrating the interest in ZDM in industrial environments and industrial communities are presented as follows:
In 2020, the National Institute of Standards and Technology (NIST) presented a case of a company based in Germany that was interested in achieving ZDM (‘A Company Laser-Focused on Zero Defects | NIST’ Citation2020). According to NIST article, the company Hilite had achieved Zero Defects using traditional QI approaches but embraced the core concept of ZDM as defined in the current paper (Psarommatis, Provoust, et al. Citation2020; Psarommatis, May, et al. Citation2020). Said concept is to ‘do things right the first time’ rather than spend time on reworking. Based on the information given in Section 3, ZDM can achieve the same outcomes while utilising fewer resources.
Efficient manufacturing (EM) is a web magazine of industry.com focused on promoting new technologies for achieving EM. In 2019, it published an article that provided an overview of ZDM. Specifically, the article described the concept of ZDM and presented some methods and applications of ZDM. The article also explained the different causes and types of defects, and stated that to achieve ZDM, 100% of the parts must be inspected and that immediate feedback is required in case of a defect (‘Zero Defect Manufacturing - Poka Yoke Overview’ Citation2019).
5. Future steps and directions for successful ZDM
The implementation of ZDM into a manufacturing system can have clearly beneficial effects on production, but it is not a straightforward procedure because of some implications that ZDM creates. For a successful ZDM implementation, the following points have been identified as counteractions for the implications generated by ZDM. As defined in Section 2, ZDM is implemented using the four ZDM strategies of Detect, Predict, Repair, and Prevent; in fact, most of the research done on ZDM is for those individual strategies (Psarommatis et al. Citation2020). However, for a successful and efficient implementation of ZDM, more studies are required to further the research on ZDM strategies. The list below presents the areas in which more research is required to successfully support the ZDM approach:
Scheduling: The implementation of ZDM affects the scheduling of production systems significantly. This is due to the logic behind ZDM, which is to act on every event in the production and schedule a mitigation action to counteract the implications of the event. A key factor here is that the number of events will be increased, and simultaneously, some of those events are considered time-sensitive, which means that the counteractions must be scheduled at a given point of time. This creates the need for rescheduling methods that are designed specifically for ZDM and for an increased number of events. Those rescheduling methods should be flexible enough to be able to also address traditional events such as new orders and maintenance actions. An initialisation of such an approach was performed by Psarommatis, Gharaei, and Kiritsis (Citation2020). Additionally, most existing scheduling tools do not consider product quality as a KPI. In fact, product quality was first introduced to production management systems by Colledani and Tolio in 2011 (Colledani and Tolio Citation2011). Furthermore, a literature review study regarding scheduling tools performed in 2016 revealed that the least considered factor in a scheduling tool is quality-related KPIs (Dios and Framinan Citation2016). Therefore, a need exists for scheduling tools that incorporate product and process quality as suggested by Psarommatis and Kiritsis (Citation2018). Moving forward, the management and optimisation of other aspects of production, such as inventory management or buffer optimisation (Psarommatis, Boujemaoui, and Kiritsis Citation2020), should be redefined, taking into consideration the implications that arising from the implementation of ZDM strategies. Currently, the manufacturing domain is shifting from mass production to mass customisation or even personalisation, which in addition to other implications is disrupting the product ordering patterns. There is a significantly higher number of orders with fewer products ordered, creating an extra burden for scheduling tools. Therefore, enhanced dynamic methods are required to rank orders dynamically, respecting ZDM principles for product quality (Psarommatis, Zheng, and Kiritsis Citation2021).
Decision Support Systems (DSS): DSS systems are vital for the implementation of the ZDM approach. They are responsible for implementing the ‘action’ ZDM strategies, repair and prevention. Once a quality event either has been detected or predicted a DSS system is required in order to decide the future of the event. A 2021 study presents a knowledge-based DSS system using ontologies in order to implement the repair ZDM strategy (Psarommatis and Kiritsis Citation2021a). Therefore, advanced data-driven DSS tools are critical for the efficient and successful implementation of ZDM.
Quality assurance design methods: In the literature, most research has been performed to develop methods and technologies for implementing ZDM strategies. For manufacturers to migrate to ZDM, they require tools and methods for investing in the most suitable quality-oriented equipment or re-purposing existing equipment for their specific case. A preliminary study in 2019 presented the direction of such an approach (Psarommatis and Kiritsis Citation2019). A more complete and elaborated method investigating all the ZDM pair strategies presented in Psarommatis (Citation2021). Such methods are crucial for efficiently implementing ZDM.
Data management systems: As ZDM is implemented using advanced data-driven technologies, the need exists for an enhanced and standardised solution for efficient data management. It is critical that specific common architectures are proposed for efficient data management, and more importantly for knowledge extraction, which is a key aspect of ZDM-oriented technologies, (Grevenitis et al. Citation2019) (Hildebrand et al. Citation2019).
Standardisation: Processes standardisation is required to avoid confusion caused by the numerous tools required for applying traditional QI methods. In addition to the terminology standardisation process described in Section 4.2, more standards are required to clearly define and standardise the emerging technologies and methods related to ZDM. Doing so will make ZDM more attractive to manufacturers and simultaneously ensure the results of the ZDM approach.
Redefine the relations: Defects must be traceable back to their root cause. The bond between supplier and customer must be strengthened by guaranteeing a complete traceable chain from the product to the deployed tools, machine parameters, and so on. Some promising results from the use of zApps from the ZDMP project (Table ) are showing promising impacts in the industry. Therefore, further is required towards this direction.
Academic courses: Academic institutes must re-adapt and create courses related to ZDM in order to teach the concept to future engineers and how this approach will help achieve more sustainable manufacturing systems while also satisfying the needs of the market.
6. Concluding remarks
The current paper was a position paper with scientific contributions on different levels around the topic of ZDM. The primary goal of the paper was to present the details of the ZDM approach in order to align researchers, engineers and industries in one common understanding about the ZDM approach, and thus eliminating any misunderstanding or misalignment among industrial and research communities (section 2). Furthermore, a thorough analysis of the state-of-the-art has been performed in order to compare ZDM with the traditional QI approaches and to present the benefits coming from the adoption of ZDM. This analysis is a key scientific contribution of the current paper because those that are skeptical of ZDM can be convinced to adopt ZDM and understand how much they can gain from the ZDM implementation (Section 3). The analysis of the state-of-the-art also revealed that there is a substantial momentum and interest towards ZDM and at the same time a lot of confusion on what ZDM is. Therefore, numerous facts that demonstrate that the migration to ZDM has already begun have been documented and analysed (Section 4). Using the results and information for Sections 2–4 numerous research areas have identified as key areas in ZDM and more research is needed on those areas in order for ZDM to be implemented efficiently and achieve true sustainable manufacturing (section 5).
More specifically section 2 presented the ZDM strategies, detect, predict, repair and prevent were presented alongside their interconnections. Furthermore, the two ZDM approaches, the product and process oriented were explained and their differences were analysed. A key contribution of the current paper was that a clear and comprehensive definition of what ZDM is was given (from ongoing standardisation process by CEN-CENELEC, in which authors participate). ZDM represents the natural evolutionary step for quality approaches in manufacturing, with the emergence of advanced data-driven technologies, below once more the definition of ZDM:
‘ZDM offers a holistic approach, aiming at greater manufacturing sustainability, which ensures both process and product quality by reducing product defects through the use of corrective, preventive, and predictive techniques made possible by data-based technologies, and guarantees that no defective products leave the production site and reach the customer’.
Section 3 was devoted to creating some counter-argument questions as they were going to be asked by skeptical people for ZDM. Also, the answers to those questions have been provided in detail in order to convince industries, researchers and engineers why they should migrate from traditional QI methods to ZDM. The findings from answering those counter- argument questions revealed that ZDM has a clear advantage over traditional QI and can lead to more sustainable manufacturing. Traditional QI methods have several disadvantages over ZDM, such as they are not exploiting the full potential of Industry 4.0 and in general data-driven technologies. Also, traditional QI are studying only the past to improve the future without considering the present as in ZDM. The goal of traditional QI approaches is to reduce the defects without learning anything from the defects. The lack of specific framework is one of the top 10 critical failure factors of traditional QI methods, something that is not the case in ZDM, manufacturers and researchers can implement ZDM using the framework described in section 2. Additionally, ZDM is easier to be implemented compared to traditional QI approaches because of the well-defined framework but also due to the reduced resources that are required for its implementation. Traditional QI approaches focus on the correction and prevention of defects without drawing real lessons from them. Although the percentage of defective products delivered may be low with current approaches, the complexity of applying these methods, the consequences of delivering a defective product, and the emergence of advanced data-based technologies are paving the way for the move to ZDM.
Section 4 presented some facts illustrating that there is an interest and momentum towards ZDM. Evidences suggest that the migration to ZDM is already underway, led by researchers from academia, notorious industrial partners, and standardisation experts. More specifically, numerous EU-funded ZDM-oriented projects were identified, which means that there is a significant interest in Europe about ZDM. Furthermore, because there is this increased interest in ZDM its standardisation that is undergoing from CEN-CENELEC is critical for becoming an industrial standard and be used across all industries. Additionally, the bibliometric study performed using as keyword the terms ‘Zero-Defect Manufacturing’ or ‘ZDM’ revealed that from 2008 there is an exponential increase for ZDM in the research community. From this search and a recent review article on ZDM (Psarommatis et al. Citation2020) it was clear that there is a confusion in the domain of ZDM, this gap in the literature was the key driver of the current paper. The scientific contribution of the current paper was to educate and align all communities to the ZDM concept and convince those that are skeptical on the adoption of ZDM.
Acknowledgments
The presented work presented supported partially by the following EU H2020 projects: Z-Fact0r (723906), QU4LITY (825030), ZDMP (825631) and Z-Break (768869), and Eur3ka (101016175). The paper reflects the authors’ views and the Commission is not responsible for any use that may be made of the information it contains.
Disclosure statement
No potential conflict of interest was reported by the author(s).
Data availability statement
Data available within the article. The authors confirm that the data supporting the findings of this study are available within the article.
Additional information
Funding
Notes on contributors
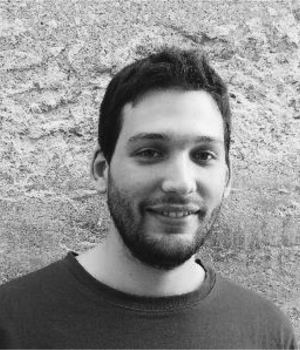
Foivos Psarommatis
Foivos Psarommatis is a passionate and active researcher in the area of quality improvement in manufacturing systems at École polytechnique fédérale de Lausanne – EPFL. More specifically is a pioneer in the area of Zero-Defect Manufacturing (ZDM), as is the first who modernised and set the foundation of modern ZDM. His scientific interests, motivation and vision are around Industry 4.0 and on how ZDM can be applied efficiently to production systems, focusing on the decision making, scheduling and design of a system or a product, with ultimate goal to achieve true sustainable manufacturing. He is actively involved in EU research programs in the area of Factories of the Future and Enabling ICT for Sustainable Manufacturing. He holds a BSc and an MSc in Mechanical engineering with specialisation on design and manufacturing engineering from the University of Patras. He has also an MSc from National University of Athens in Automation systems with specialisation on manufacturing and production systems. He did his PhD around the topic of Zero-Defect Manufacturing École polytechnique fédérale de Lausanne - EPFL. He is an active member on a CEN/CENELEC working group responsible for standardising ZDM and a member of IOF (Industrial Ontologies Foundry) where he is the chair of the ZDM working group.
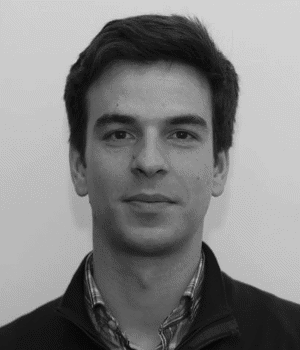
João Sousa
João Sousa holds an MSc degree in Mechanical Engineering from the University of Minho, Portugal, and is currently pursuing a PhD at the same university. He has been actively involved in several national and EU research programs in the areas of Factories of the Future, Information and Communication Technologies (ICT) and Connected Smart Factories with a close collaboration with industry. His motivation is to help manufacturing companies achieve sustainable manufacturing with Industry 4.0 technologies. The main research areas are information modelling, industrial metrology and IIoT/CPPS. He is currently a Centre of Technology and Systems (CTS) - UNINOVA researcher in Portugal.
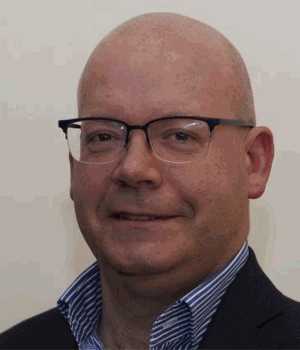
João Pedro Mendonça
João Pedro Mendonça is currently Assistant Professor in the Department of Mechanical Engineering, University of Minho. He received his M.Sc. in Mechanical Engineering and Ph.D. in Manufacturing Technologies from University of Minho. He regularly teaches ‘Technical Drawing and Computer Modelling’ and ‘Metrology’ at School of Engineering – University of Minho, where he is responsible for the Laboratory of Dimensional Metrology. He has been integrated member of MEtRICs, the research center unit of the Department of Mechanical Engineering for FCT. He is responsible and unit coordinator of a number of international and national projects, namely: H2020-FoF C2NET Cloud Collaborative Manufacturing Networks. He has been also scientific leader of national projects funded by P2020 and private companies, namely ‘PD4.0 Progressive Dies’, ‘IoT4Stone - IoT deployment of Stone CNC machinery’ or ‘NEXTGEN 3D Printer – Development of 3D SLS printing machine under industry 4.0 principles’. He is the author of a number of papers (ISI-Scopus) and peer-review conference proceedings papers. He is inventor of two international patents. Actually, he is member of Manufacturing Enterprise Interoperability Group of INTEROP-VLab european virtual LabS network. His main research interests include CPS Ciber-Physical Systems focused in CIM/Metrology, zero defects manufacturing, semantic interoperability of technical information, Internet of Things and FIWARE and open standards-based PLM frameworks.
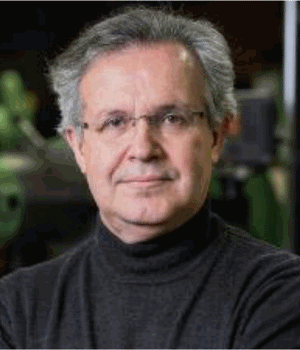
Dimitris Kiritsis
Dimitris Kiritsis is Faculty Member at the Institute of Mechanical Engineering of the School of Engineering of EPFL, Switzerland, where he is leading a research group on ICT for Sustainable Manufacturing. His research interests are Closed-Loop Lifecycle Management, Sustainable Manufacturing IIoT, Semantic Technologies, Industrial Ontologies and Data Analytics for Engineering Applications. He serves as Director of the doctoral Program of EPFL on Robotics, Control and Intelligent Systems (EDRS). He served as Guest Professor at the IMS Center of the University of Cincinnati, and Invited Professor at the University of Technology of Compiègne, the University of Technology of Belfort-Montbéliard and at ParisTech ENSAM Paris. He is actively involved in EU research programs in the area of Factories of the Future and Enabling ICT for Sustainable Manufacturing. He has more than 220 publications. From September 2013 to September 2019 Dimitris was the Chair of IFIP WG5.7 – Advanced Production Management Systems. From 2013 to 2017 he was member of the Advisory Group of the European Council on Leadership on Enabling Industrial Technologies – AG LEIT-NMBP. He is also founding fellow member of the International Society for Engineering Asset Management (ISEAM) and among the initiators and co-founders of the IOF (Industrial Ontologies Foundry). Since 2019 Dimitris is member of the WEF Global Future Council of Advanced Manufacturing and Production
References
- “A Company Laser-Focused on Zero Defects | NIST”. 2020. https://www.nist.gov/mep/successstories/2017/company-laser-focused-zero-defects.
- Allgöwer, Frank, João Borges de Sousa, James Kapinski, Pieter Mosterman, Jens Oehlerking, Patrick Panciatici, Maria Prandini, Akshay Rajhans, Paulo Tabuada, and Philipp Wenzelburger. 2019. “Position Paper on the Challenges Posed by Modern Applications to Cyber-Physical Systems Theory.” Nonlinear Analysis: Hybrid Systems 34 (November): 147–165 Elsevier. doi:https://doi.org/10.1016/J.NAHS.2019.05.007.
- Blind, Knut. 2016. “The Impact of Standardisation and Standards on Innovation.” In Handbook of Innovation Policy Impact, edited by Jakob Edler, Paul Cunningham, Abdullah Gök, and Philip Shapira, 423–449. Edward Elgar Publishing Ltd. doi:https://doi.org/10.4337/9781784711856.00021.
- Cheah, Soo Jin, Amirul Shah Amirul, and Fauziah Md Taib. 2011. “Tracking Hidden Quality Costs in a Manufacturing Company: An Action Research.” International Journal of Quality and Reliability Management 28 (4): 405–425. Emerald Group Publishing Limited: doi:https://doi.org/10.1108/02656711111121816.
- Chiariotti, Paolo, Matteo Fitti, Paolo Castellini, Saverio Zitti, Marco Zannini, and Nicola Paone. 2018. “Smart Quality Control Station for Non-Contact Measurement of Cylindrical Parts Based on a Confocal Chromatic Sensor.” IEEE Instrumentation and Measurement Magazine 21 (6): 22–28. Institute of Electrical and Electronics Engineers Inc. doi:https://doi.org/10.1109/MIM.2018.8573589.
- Chien, Chen Fu, Shao Chung Hsu, and Ying Jen Chen. 2013. “A System for Online Detection and Classification of Wafer Bin Map Defect Patterns for Manufacturing Intelligence.” International Journal of Production Research 51 (8): 2324–2338. doi:https://doi.org/10.1080/00207543.2012.737943.
- Choi, Gyunghyun, Sung Hee Kim, Chunghun Ha, and Suk Joo Bae. 2012. “Multi-Step ART1 Algorithm for Recognition of Defect Patterns on Semiconductor Wafers.” International Journal of Production Research 50 (12): 3274–3287. doi:https://doi.org/10.1080/00207543.2011.574502.
- Colledani, M., and T. Tolio. 2011. “Integrated Analysis of Quality and Production Logistics Performance in Manufacturing Lines.” International Journal of Production Research 49 (2): 485–518. Taylor & Francis Group. doi:https://doi.org/10.1080/00207540903443246.
- “Course - Zero Defect Manufacturing - TØL4009 - NTNU”. 2021. Accessed February 20. https://www.ntnu.edu/studies/courses/TØL4009#tab=omEmnet.
- Cui, Yao, Ming Hu, and Jingchen Liu. 2018. “Values of Traceability in Supply Chains.” SSRN Electronic Journal, December. Elsevier BV. doi:https://doi.org/10.2139/ssrn.3291661.
- Deloitte. 2015. “Made to Order: The Rise of Mass Personalisation.” https://www2.deloitte.com/ch/en/pages/consumer-business/articles/made-to-order-the-rise-of-mass-personalisation.html.
- Dios, Manuel, and Jose M. Framinan. 2016. “A Review and Classification of Computer-Based Manufacturing Scheduling Tools.” Computers and Industrial Engineering 99: 229–249. Elsevier Ltd. doi:https://doi.org/10.1016/j.cie.2016.07.020.
- Efthymiou, K., K. Sipsas, D. Mourtzis, and G. Chryssolouris. 2013. “On an Integrated Knowledge Based Framework for Manufacturing Systems Early Design Phase.” Procedia CIRP 9: 121–126. Elsevier B.V. doi:https://doi.org/10.1016/j.procir.2013.06.179.
- El Kadiri, Soumaya, and Dimitris Kiritsis. 2015. “Ontologies in the Context of Product Lifecycle Management: State of the Art Literature Review.” International Journal of Production Research 53 (18): 5657–5668. Taylor and Francis Ltd. doi:https://doi.org/10.1080/00207543.2015.1052155.
- Enyoghasi, Christian, and Fazleena Badurdeen. 2021. “Industry 4.0 for Sustainable Manufacturing: Opportunities at the Product, Process, and System Levels.” Resources, Conservation and Recycling 166 (March): 105362. Elsevier B.V. doi:https://doi.org/10.1016/j.resconrec.2020.105362.
- Farooqui, Ashfaq, Kristofer Bengtsson, Petter Falkman, and Martin Fabian. 2020. “Towards Data-Driven Approaches in Manufacturing: An Architecture to Collect Sequences of Operations.” International Journal of Production Research 4947–4963. doi:https://doi.org/10.1080/00207543.2020.1735660.
- Feng, Qianmei, and Kailash C. Kapur. 2006. “Economic Design of Specifications for 100% Inspection with Imperfect Measurement Systems.” Quality Technology & Quantitative Management 3 (2): 127–144. Informa UK Limited. doi:https://doi.org/10.1080/16843703.2006.11673105.
- Ferreira, José, Fábio Lopes, Guy Doumeingts, João Sousa, João P. Mendonça, Carlos Agostinho, and Ricardo Jardim-Goncalves. 2020. “Empowering SMEs with Cyber-Physical Production Systems: From Modelling a Polishing Process of Cutlery Production to CPPS Experimentation.” Studies in Computational Intelligence 864: 139–177. Springer. doi:https://doi.org/10.1007/978-3-030-38704-4_7.
- Fraile, Francisco, Raquel Sanchis, Raul Poler, and Angel Ortiz. 2019. “Reference Models for Digital Manufacturing Platforms.” Applied Sciences (Switzerland) 9 (20): 4433–4457. doi:https://doi.org/10.3390/app9204433.
- Gaspar, Rogério, Buket Aksu, Alain Cuine, Meindert Danhof, Milena Jadrijevic Mladar Takac, Hans H. Linden, Andreas Link, et al. 2012. “Towards a European Strategy for Medicines Research (2014–2020): The EUFEPS Position Paper on Horizon 2020.” European Journal of Pharmaceutical Sciences 47 (5): 979–987. Elsevier. doi:https://doi.org/10.1016/J.EJPS.2012.09.020.
- Grevenitis, K., F. Psarommatis, A. Reina, W. Xu, I. Tourkogiorgis, J. Milenkovic, J. Cassina, and D. Kiritsis. 2019. “A Hybrid Framework for Industrial Data Storage and Exploitation.” Procedia CIRP 81: 892–897. Elsevier B.V. doi:https://doi.org/10.1016/j.procir.2019.03.221.
- Guleria, Prateek, Abhilash Pathania, Himani Bhatti, Kuldeep Rojhe, and Dalgobind Mahto. 2021. “Leveraging Lean Six Sigma: Reducing Defects and Rejections in Filter Manufacturing Industry.” Materials Today: Proceedings 46 (17): 8532–8539. doi:https://doi.org/10.1016/j.matpr.2021.03.535.
- Gupta, Himanshu, Ashwani Kumar, and Pratibha Wasan. 2021, February. “Industry 4.0, Cleaner Production and Circular Economy: An Integrative Framework for Evaluating Ethical and Sustainable Business Performance of Manufacturing Organizations.” Journal of Cleaner Production 126253. Elsevier. doi:https://doi.org/10.1016/j.jclepro.2021.126253.
- Hildebrand, Marlène, Ioannis Tourkogiorgis, Foivos Psarommatis, Damiano Arena, and Dimitris Kiritsis. 2019. “A Method for Converting Current Data to RDF in the Era of Industry 4.0.” IFIP Advances in Information and Communication Technology 566: 307–314. Springer New York LLC. doi:https://doi.org/10.1007/978-3-030-30000-5_39.
- ISO 9000. 2005. “ISO - ISO 9000:2005 - Quality Management Systems — Fundamentals and Vocabulary.” https://www.iso.org/standard/42180.html.
- Jun, Ji-hye, Tai-Woo Chang, and Sungbum Jun. 2020. “Quality Prediction and Yield Improvement in Process Manufacturing Based on Data Analytics.” Processes 8 (9): 1068–1085. doi:https://doi.org/10.3390/pr8091068.
- Krishna, L. Siva Rama, and P. J. Srikanth. 2021. “Evaluation of Environmental Impact of Additive and Subtractive Manufacturing Processes for Sustainable Manufacturing.” Materials Today: Proceedings 166: 3054–3060. doi:https://doi.org/10.1016/j.matpr.2020.12.060.
- Kumar, Pankaj, J. Maiti, and Angappa Gunasekaran. 2018. “Impact of Quality Management Systems on Firm Performance.” International Journal of Quality and Reliability Management 35 (5): 1034–1059. doi;https://doi.org/10.1108/IJQRM-02-2017-0030.
- Kuo, Chung Feng, Chien Tung Max Hsu, Chih Heng Fang, Shin Min Chao, and Yu De Lin. 2013. “Automatic Defect Inspection System of Colour Filters Using Taguchi-Based Neural Network.” International Journal of Production Research 51 (5): 1464–1476. doi:https://doi.org/10.1080/00207543.2012.695877.
- Kuo, Yong Hong, and Andrew Kusiak. 2019. “From Data to Big Data in Production Research: The Past and Future Trends.” International Journal of Production Research 57 (15-16): 4828–4853. doi:https://doi.org/10.1080/00207543.2018.1443230.
- Lindström, John, Erik Lejon, Petter Kyösti, Massimo Mecella, Dominic Heutelbeck, Matthias Hemmje, Mikael Sjödahl, Wolfgang Birk, and Bengt Gunnarsson. 2019. “Towards Intelligent and Sustainable Production Systems with a Zero-Defect Manufacturing Approach in an Industry4.0 Context.” Procedia CIRP 81: 880–885. Elsevier B.V. doi:https://doi.org/10.1016/j.procir.2019.03.218.
- Majeed, Arfan, Yingfeng Zhang, Shan Ren, Jingxiang Lv, Tao Peng, Saad Waqar, and Enhuai Yin. 2021. “A Big Data-Driven Framework for Sustainable and Smart Additive Manufacturing.” Robotics and Computer-Integrated Manufacturing 67 (February): 102026. Elsevier Ltd: doi:https://doi.org/10.1016/j.rcim.2020.102026.
- “Master’s Programme in Manufacturing (EIT Manufacturing Master School) - Zero Defect Manufacture for a Circular Economy | Aalto University.” 2021. Accessed February 20. https://www.aalto.fi/en/study-options/masters-programme-in-manufacturing-eit-manufacturing-master-school-zero-defect.
- Mourtzis, Dimitris, John Angelopoulos, and Nikos Panopoulos. 2021. “Equipment Design Optimization Based on Digital Twin Under the Framework of Zero-Defect Manufacturing.” Procedia Computer Science 180: 525–533. Elsevier B.V. doi:https://doi.org/10.1016/j.procs.2021.01.271.
- Mourtzis, Dimitris, Michalis Doukas, and Foivos Psarommatis. 2015. “A Toolbox for the Design, Planning and Operation of Manufacturing Networks in a Mass Customisation Environment.” Journal of Manufacturing Systems 36: 274–286. The Society of Manufacturing Engineers: doi:https://doi.org/10.1016/j.jmsy.2014.06.004.
- Mourtzis, D., M. Doukas, F. Psarommatis, and N. Panopoulos. 2013. “Design of Multi-Stage Manufacturing Networks for Personalized Products Using Metaheuristics.” Lecture Notes in Mechanical Engineering 7: 1263–1276. doi:https://doi.org/10.1007/978-3-319-00557-7_103.
- Mourtzis, D., E. Vlachou, and N. Milas. 2016. “Industrial Big Data as a Result of IoT Adoption in Manufacturing.” Procedia CIRP 55: 290–295. Elsevier B.V. doi:https://doi.org/10.1016/j.procir.2016.07.038.
- Nazarenko, Artem A., Joao Sarraipa, Luis M. Camarinha-Matos, Christian Grunewald, Marc Dorchain, and Ricardo Jardim-Goncalves. 2021. “Analysis of Relevant Standards for Industrial Systems to Support Zero Defects Manufacturing Process.” Journal of Industrial Information Integration, Elsevier B.V. doi:https://doi.org/10.1016/j.jii.2021.100214.
- Onu, Peter, and Charles Mbohwa. 2021. “Industry 4.0 Opportunities in Manufacturing SMEs: Sustainability Outlook.” Materials Today: Proceedings 44: 1925–1930. doi:https://doi.org/10.1016/j.matpr.2020.12.095.
- Özcan, Ali Mert, Anıl Akdoğan, and Numan M. Durakbasa. 2021. “Improvements in Manufacturing Processes by Measurement and Evaluation Studies According to the Quality Management System Standard in Automotive Industry.” Lecture Notes in Mechanical Engineering, 483–492. Springer Science and Business Media Deutschland GmbH. doi:https://doi.org/10.1007/978-3-030-62784-3_41.
- Pacheco, Diego, Isaac Pergher, Guilherme Luís Roehe Vaccaro, Carlos Fernando Jung, and Carla Ten Caten. 2015. “18 Comparative Aspects Between Lean and Six Sigma: Complementarity and Implications.” International Journal of Lean Six Sigma 6 (2): 161–175. Emerald Group Publishing Ltd. doi:https://doi.org/10.1108/IJLSS-05-2014-0012.
- Piechota, Steffen, Andreas Glas, and Michael Essig. 2021. “Questioning the Relevance of Supplier Satisfaction for Preferred Customer Treatment: Antecedent Effects of Comparative Alternatives and Multi-Dimensionality.” Journal of Purchasing and Supply Management 27 (1): 100672. doi:https://doi.org/10.1016/j.pursup.2021.100672.
- Pinho, Cláudia, and Luis Mendes. 2017. “IT in Lean-Based Manufacturing Industries: Systematic Literature Review and Research Issues.” International Journal of Production Research 55 (24): 7524–7540. doi:https://doi.org/10.1080/00207543.2017.1384585.
- Pinto, Eduardo B., and Gabriela Fernandes. 2021. “Collaborative R&D the Key Cooperation Domain for University-Industry Partnerships Sustainability – Position Paper.” Procedia Computer Science 181 (January): 102–109. Elsevier. doi:https://doi.org/10.1016/J.PROCS.2021.01.109.
- Psarommatis, Foivos. 2021. “A Generic Methodology and a Digital Twin for Zero Defect Manufacturing (ZDM) Performance Mapping Towards Design for ZDM.” Journal of Manufacturing Systems 59 (April): 507–521. Elsevier. doi:https://doi.org/10.1016/j.jmsy.2021.03.021.
- Psarommatis, Foivos, Ali Boujemaoui, and Dimitris Kiritsis. 2020. “A Computational Method for Identifying the Optimum Buffer Size in the Era of Zero Defect Manufacturing.” IFIP Advances in Information and Communication Technology 592: 443–450. IFIP Springer. doi:https://doi.org/10.1007/978-3-030-57997-5_51.
- Psarommatis, Foivos, Ali Gharaei, and Dimitris Kiritsis. 2020. “Identification of the Critical Reaction Times for Re-Scheduling Flexible Job Shops for Different Types of Unexpected Events.” Procedia CIRP 93 (January): 903–908. Elsevier. doi:https://doi.org/10.1016/j.procir.2020.03.038.
- Psarommatis, Foivos, and Dimitris Kiritsis. 2018. “A Scheduling Tool for Achieving Zero Defect Manufacturing (ZDM): A Conceptual Framework.” IFIP Advances in Information and Communication Technology 536: 271–278. Springer New York LLC. doi:https://doi.org/10.1007/978-3-319-99707-0_34.
- Psarommatis, Foivos, and Dimitris Kiritsis. 2019. “Identification of the Inspection Specifications for Achieving Zero Defect Manufacturing.” IFIP Advances in Information and Communication Technology 566: 267–273. Springer New York LLC. doi:https://doi.org/10.1007/978-3-030-30000-5_34.
- Psarommatis, Foivos, and Dimitris Kiritsis. 2021a. “A Hybrid Decision Support System for Automating Decision Making in the Event of Defects in the Era of Zero Defect Manufacturing.” Journal of Industrial Information Integration 100263. Elsevier Inc. doi:https://doi.org/10.1016/j.jii.2021.100263.
- Psarommatis, Foivos, and Dimitris Kiritsis. 2021b. “Comparison Between Product and Process Oriented Zero-Defect Manufacturing (ZDM) Approaches.” IFIP Advances in Information and Communication Technology, 630: 105–112. Springer, Cham. doi:https://doi.org/10.1007/978-3-030-85874-2_11.
- Psarommatis, Foivos, Gökan May, Paul-Arthur Dreyfus, and Dimitris Kiritsis. 2020. “Zero Defect Manufacturing: State-of-the-Art Review, Shortcomings and Future Directions in Research.” International Journal of Production Research 58: 1–17. doi:https://doi.org/10.1080/00207543.2019.1605228.
- Psarommatis, Foivos, Sylvain Prouvost, Gökan May, and Dimitris Kiritsis. 2020. “Product Quality Improvement Policies in Industry 4.0: Characteristics, Enabling Factors, Barriers, and Evolution Toward Zero Defect Manufacturing.” Frontiers in Computer Science 2 (August): 1–15. doi:https://doi.org/10.3389/fcomp.2020.00026.
- Psarommatis, Foivos, Xiaochen Zheng, and Dimitris Kiritsis. 2021. “A Two-Layer Criteria Evaluation Approach for Re-Scheduling Efficiently Semi-Automated Assembly Lines with High Number of Rush Orders.” Procedia CIRP 97 (January): 172–177. Elsevier. doi:https://doi.org/10.1016/j.procir.2020.05.221.
- Quality and Reliability Assurance Handbook. 1965. “Assistant Secretary of Defense (Manpower Installations and Logistics) Washington DC: Guide To Zero Defects.”
- Schiffauerova, Andrea, and Vince Thomson. 2006. “A Review of Research on Cost of Quality Models and Best Practices.” International Journal of Quality and Reliability Management 23 (6): 647–669. doi:https://doi.org/10.1108/02656710610672470.
- Sousa, João, Ferreira José, Lopes Carlos, João Sarraipa, and João Silva. 2020. “Enhancing the Steel Tube Manufacturing Process With a Zero Defects Approach.” In ASME 2020 International Mechanical Engineering Congress and Exposition, Volume 2B: Advanced Manufacturing. November 16–19, Online. doi:https://doi.org/https://doi.org/10.1115/IMECE2020-24678.
- Sousa, João, João Silva, and José Machado. 2022. Metrology Information in Cyber-Physical Systems, 285–292. Springer, Cham. doi:https://doi.org/10.1007/978-3-030-79168-1_26.
- Tannock, James, and Sittichai Saelem. 2007. “Manufacturing Disruption Costs Due to Quality Loss.” International Journal of Quality and Reliability Management 24 (3): 263–278. Emerald Group Publishing Limited. doi:https://doi.org/10.1108/02656710710730861.
- Tao, Fei, Qinglin Qi, Ang Liu, and Andrew Kusiak. 2018. “Data-Driven Smart Manufacturing.” Journal of Manufacturing Systems 48 (July): 157–169. Elsevier B.V. doi:https://doi.org/10.1016/j.jmsy.2018.01.006.
- Vargas, Cyntia, Jens Bürger, Fabien Viertel, Birgit Vogel-Heuser, and Jan Jürjens. 2018. “System Evolution Through Semi-Automatic Elicitation of Security Requirements: A Position Paper.” IFAC-PapersOnLine 51 (10): 64–69. Elsevier. doi:https://doi.org/10.1016/J.IFACOL.2018.06.238.
- Yamaguchi, Takafumi, and Yoshiyuki Yamashita. 2021. “Quality Prediction for Multi-Grade Batch Process Using Sparse Flexible Clustered Multi-Task Learning.” Computers and Chemical Engineering 150 (July): 107320. Elsevier Ltd: doi:https://doi.org/10.1016/j.compchemeng.2021.107320.
- “Zero Defect Manufacturing - Poka Yoke Overview.” 2019. https://www.industr.com/en/zero-defect-manufacturing-poka-yoke-overview-2371462.
- Zhang, Cheng, Daindi Chen, Fei Tao, and Ang Liu. 2019. “Data Driven Smart Customization.” Procedia CIRP 81: 564–569. Elsevier B.V. doi:https://doi.org/10.1016/j.procir.2019.03.156.
- Zhang, Qingyu, Mark A. Vonderembse, and Mei Cao. 2009. “Product Concept and Prototype Flexibility in Manufacturing: Implications for Customer Satisfaction.” European Journal of Operational Research 194 (1): 143–154. North-Holland. doi:https://doi.org/10.1016/j.ejor.2007.12.013.