Abstract
Working from home becomes the norm; this trend has put added pressure on urban logistics, as large volumes of goods and services are required for domestic use. Meanwhile, public transport operators face a big challenge and trade-off due to higher labour and frequent cleaning costs, with lower passenger revenue over a longer period. Considering the collaborative urban public transport services achieve a seamless movement for both passengers and goods, and could reduce the adverse effects of the existing urban public transport systems. Therefore, a mixed-integer linear programming model introducing the concept of capacity matching is proposed to assist this collaborative urban freight service network in minimising total freight transport time at station hubs and not affecting passenger transportation in this paper. Moreover, an efficient improved optimisation algorithm based on the Artificial Bee Colony Algorithm (ABC) is designed, and the numerical examples and real cases are illustrated to demonstrate the feasibility and effectiveness of the proposed model and algorithm. The performance evaluations suggest that the coordinated operating strategy of the collaborative freight transportation system supports increasing mobility demands for freight, resulting in declining congestion levels and reducing transport emissions, while no influence in passenger transport, notably in urban areas.
Acknowledgements
This work was partially supported by the Fundamental Research Funds for the Central Universities [grant number 2021JBM014], the National Natural Science Foundation of China [grant numbers 72101013, 71890972/71890970, 71621001], the Beijing Intelligent Logistics System Collaborative Innovation Centre [grant number BILSCIC-2019KF-11], and the 111 Project (No. B20071). In addition, we would appreciate the editor and reviewers for their helpful comments and suggestions, which greatly improved this paper.
Availability of data
Data available on request from the authors.
Disclosure statement
No potential conflict of interest was reported by the author(s).
Additional information
Funding
Notes on contributors
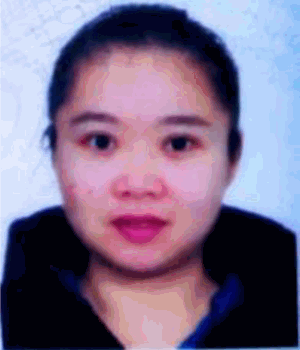
Feng Li
Feng Li received the B.S. degree in computer science and technology from Hunan Normal University in 2002, the M.S. degree in management science and engineering from Guilin University of Electronic Technology in 2007, and the Ph.D. degree in management science and engineering from University Of Science and Technology Beijing, Beijing, China, in 2011. She is currently dean and Associate Professor with the Department of Information management and e-commerce, School of Information, Beijing Wuzi University, Beijing, China. Her research interests include products design, job shop scheduling, and artificial intelligence.
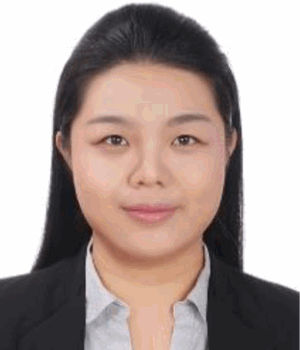
Xin Guo
Xin Guo received the Ph.D. degree in System Science from Beijing Jiaotong University, in 2018, China. Dr. Guo is currently a Lecturer with Key Laboratory of Transport Industry of Big Data Application Technologies for Comprehensive Transport, Beijing Jiaotong University. Her primary research interests include urban transit system optimisation, urban rail transit operation management and application, artificial intelligence.
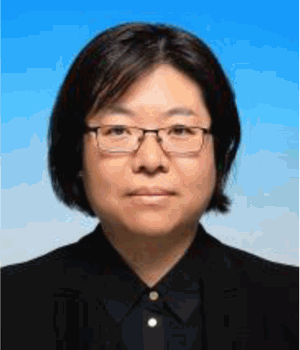
Li Zhou
Li Zhou received the Ph.D. degree in management from Beijing Institute of Technology,Beijing, China,in 2006. She is now a professor and Dean of the School of Information, Beijing Wuzi University. Director of Beijing Intelligent Logistics System Cooperative Innovation Centre. Her research interests include intelligent logistics systems, optimisation theory and methods, big data technology and applications.
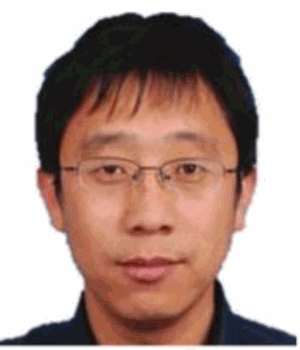
Jianjun Wu
Jianjun Wu received the Ph.D. degree in Transportation planning & management from Beijing Jiaotong University, in 2008, China. Dr. Wu joined in the State Key Laboratory of Rail Traffic Control and Safety at the Beijing Jiaotong University and was promoted to full Professor in 2015. He primary research interests include optimisation of the urban transit system, the complexity of transportation network, transport planning, big data in the transportation system. Currently, he is vice-director of the State Key Laboratory of Rail Traffic Control and Safety and the State Key Laboratory of Transport Industry of Big Data Application Technologies for Comprehensive Transport.
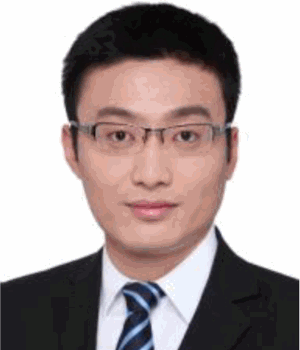
Tongfei Li
Tongfei Li received the Ph.D. degree in system science from Beijing Jiaotong University, in 2018, and the joint Ph.D. degree from the National University of Singapore, supported by the China Scholarship Council, in 2017. He is currently an associate professor with the College of Metropolitan Transportation, Beijing University of Technology, Beijing, China. His research interests include transportation and land use, ridesharing, and connected autonomous vehicle.