ABSTRACT
Industry 4.0 (I4.0) is a fast-evolving area of research, bringing together knowledge from multiple academic fields into creative solutions for manufacturing innovation. Despite the growing amount of published work covering a wide range of I4.0 areas, there has been relatively little research devoted to the organisational side of implementing I4.0. To address this gap, this systematic review paper used quantitative analysis by text-mining 97 articles from 2015 to 2021. The analysis identified eleven research streams, which were grouped into five levels, namely industry and firm, smart factory, data, human resources and supply chain. The research streams were then comprehensively reviewed and presented. For each stream, the paper presents a number of sub-themes and highlights important findings and areas that may require further development. We discuss three important research avenues in the organisational management literature: (1) the impact of the pandemic on the implementation of I4.0, (2) the tension between value creation and value protection during the implementation of 4.0 and (3) the relevance of a contingency approach during the implementation of I4.0.
1. Introduction
Industry 4.0 (I4.0) represents an ongoing transformational phase for manufacturing organisations aiming to fully interlink their business functions and production systems with data from the entire lifecycle or End-to-End Digital Integration (Liao et al. Citation2017; Castelo-Branco, Cruz-Jesus, and Oliveira Citation2019). The implementation of I4.0 has been conceptualised not only in terms of the technological dimension of the factory of the future, but also in terms of the broader organisational dimensions, such as strategy, people, and culture (Bibby and Dehe Citation2018). The 3rd industrial revolution or Digital Transformation proposed a ‘digital business ecosystem’ (Hanelt et al. Citation2020) supported by the firm’s existing IT capabilities (Wessel et al. Citation2021) and analytics ecosystem (Pappas et al. Citation2018). In contrast, I4.0 and its implementation extend far beyond connectivity, the boundary of a single firm or a limited set of information technologies, leveraging the convergence of autonomous and data-driven technology ecosystems with the human element (Schuh et al. Citation2017). I4.0 aims to increase the interconnection and exchange of real-time production data between and among people, processes, services, smart products, and production equipment (Prause Citation2015; Frank et al. Citation2019). I4.0 technologies could potentially be applied even beyond manufacturing, such as in construction supply chains (Dallasega, Rauch, and Linder Citation2018), humanitarian supply chains (Kumar and Singh Citation2021), and in fighting the current pandemic (Lepore et al. Citation2021).
Successful implementation of I4.0 potentially enables a higher level of self-configuration, automation, informatisation and decentralised decision-making (Stock and Seliger Citation2016). Such significant transformation is enabled by cyber-physical production systems, which sense and act upon their immediate surroundings. These systems are enhanced by machine learning and the Industrial Internet of Things (IIoT) to create a digital twin of the manufacturing environment (Negri, Fumagalli, and Macchi Citation2017). As application fields widen Liao et al. (2017), the impact of implementing I4.0 is not just limited to the factory floor level (smart factory), but also within the internal support functions of an organisation (R&D, human resources, marketing etc.) and across supply chain partners (Ivanov, Dolgui, and Sokolov Citation2019). In this context, the implementation stage follows the readiness assessment and planning stage in the path towards the adoption of I4.0 (Himang et al. Citation2020). The implementation strategy or method represents a crucial stage and to a large degree influences the outcome of implementing any digital transformation strategy (Correani et al. Citation2020). Embarking on the path towards I4.0 implementation could potentially improve production performance (Tortorella et al. Citation2019; Lorenz et al. Citation2020). Yet other firms use I4.0 to enhance environmental (Müller, Kiel, and Voigt Citation2018; Ghobakhloo Citation2020a) and social sustainability (Pappas et al. Citation2018; Margherita and Braccini Citation2020). At the same time, implementation represents a major challenge for SMEs and multinationals that aim to take advantage of I4.0 in order to underpin new business models and innovative human-machine work structures.
Given the technical underpinning of I4.0, it is not surprising that initially the organisational dimension of implementing I4.0 remained overlooked and understudied. Therefore, previous literature reviews have approached implementation from a technology-centric viewpoint. For instance, I4.0 technologies have been classified (Oztemel and Gursev Citation2020) and application fields have been reviewed in the context of manufacturing (Zheng et al. Citation2020) and remanufacturing (Kerin and Pham Citation2019). Others reviewed the data-driven paradigm of I4.0 technologies (Klingenberg, Borges, and Antunes Jr Citation2019) and technology lifecycle (Núñez-Merino et al. Citation2020). Similarly, literature review papers have examined I4.0 technology-centric design principles, including interoperability and predictability (Butt Citation2020) to understand maintenance transformation (Silvestri et al. Citation2020), the predictive maintenance capabilities of I4.0 (Zonta et al. Citation2020) and additive manufacturing (Hernandez Korner et al. Citation2020). Other reviews have also investigated specific operational aspects. For instance, Xu, Xu, and Li (Citation2018) examined both the technological and operational perspectives of implementation, focusing on the smart factory environment.
As reviews like those mentioned above have covered the technology in detail and touched upon operational plant or factory aspects, reviewing I4.0 implementation from the organisational perspective remains an important research gap. Undertaking such a review in a more granular manner can inform our understanding of implementation beyond the purely technological aspects of the ongoing industrial transformation. There is a pressing need to review the literature from such a vantage point, due to the rapidly increasing number of empirical publications related to the management of implementing I4.0 (Schneider Citation2018; Piccarozzi, Aquilani, and Gatti Citation2018). Also, routine disruptions (e.g. market volatility) and the recent pandemic have created a context of uncertainty regarding organisational value creation with a pressing need for shifting operations towards digitisation and automation (McKinsey & Company et al. Citation2020; Papagiannidis, Harris, and Morton Citation2020; Seifert and Markoff Citation2021).
Scholars have recently started to review various management aspects of I4.0. For example, reviews have investigated I4.0 maturity and readiness models (Hizam-Hanafiah, Soomro, and Abdullah Citation2020; Hajoary Citation2021) or more commonly the implications for sustainability and the process integration of I4.0 (Kamble, Gunasekaran, and Gawankar Citation2018). In the same vein, Sony and Naik (Citation2020) systematically reviewed the design mechanism for implementing I4.0 from a socio-technical perspective, acknowledging the role of the human element in I4.0.
Extant reviews have to some degree investigated I4.0, regarding specific yet narrow aspects related to different types of business model and work design (Wagire, Rathore, and Jain Citation2019), and increasingly lean practices (Erro-Garcés Citation2019; Bittencourt, Alves, and Leão Citation2021). Most notably, Schneider (Citation2018) provided a comprehensive review of the relevant management literature from 2010 to 2016, recognising the role of the manager and the importance of management strategy for change and leadership, yet not elaborating specifically on I4.0 implementation. Similarly Piccarozzi, Aquilani, and Gatti (Citation2018) have reviewed studies published from 2014 to 2018 and defined the managerial working definition of I4.0, aiming to understand developments at the single firm level with limited elaboration on I4.0 implementation resources, stages, and outcomes. Although some light has been shed on specific organisational aspects, the full picture of what implementation encompasses remains fragmented. Implementation of I4.0 solutions is an evolving process dependent on the implementation style (Geels et al. Citation2021). However, the areas of use for I4.0 (i.e. factory, project, product, process, etc.) have so far not been effectively linked to the organisation.
Given the above and the gaps in the I4.0 reviews, the purpose of this paper is to address the following two research questions: (a) What are the antecedents and outcomes of I4.0 implementation for organisations? (b) What is the research agenda on the implementation of I4.0 from an organisational perspective and how can we synthesise and address the limitations? Viewing I4.0 from an organisational perspective amalgamates the operational (Xu, Xu, and Li Citation2018), management (Piccarozzi, Aquilani, and Gatti Citation2018) and the relatively well-studied technical considerations. Such an approach helps delineate the stages of implementation (Stornelli, Ozcan, and Simms Citation2021) and the wider complexities of implementation at multiple levels. We start by presenting the reason for the review methodology adopted for this systematic review in Section 2 and the results of the text-mining analysis in Section 3. The identified research streams were grouped within five levels and critically reviewed (Section 4). Lastly, we present and discuss potential avenues for future research on implementing I4.0 from an organisational perspective.
2. Methodology
The current review employed a methodology based on Tranfield, Denyer, and Smart (Citation2003) and guided by Denyer and Tranfield (Citation2009), following the process shown in Figure . During the preparation stage, the guidelines for the review were set out and the research focus and goals were defined. These shaped the research questions and direction of the study.
Figure 1. Methodology.
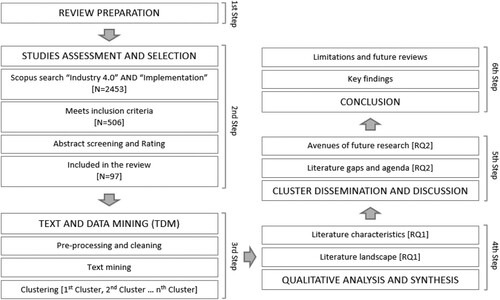
Following the first step, the articles were filtered, evaluated, and rated to validate the relevance to the research objectives. The third stage included the text-mining operation (Davlembayeva, Papagiannidis, and Alamanos Citation2019; Pavlidou, Papagiannidis, and Tsui Citation2020), followed by the qualitative review. Each step is discussed in more detail below.
2.1. Review preparation
The preparation stage included the planning and formulation of the review protocols based on the gaps identified within the literature. A preliminary examination of the literature was conducted to facilitate the identification and clarification of the research gaps within the literature from multiple angles, supporting the formulation of the research objectives. An initial review of extant studies on implementing I4.0 supported the need to undertake the review.
2.2. Selection and assessment
To capture the full spectrum of data contained in the pool of papers on I4.0 all the articles with the keywords ‘Industry 4.0’ and ‘Implementation’ mentioned in the title, keywords or abstract of the paper were selected from the Scopus database, as of February 2021. Scopus is user-friendly, includes a wide range of journals (approx. 20% more coverage compared to WoS) and is more consistent than other databases like Google Scholar (Falagas et al. Citation2008). Articles available in full text and in English were included in the initial search criteria. No limits were set on the publication year. Subject areas were filtered to exclude highly technical domains (e.g. mathematics, chemical engineering, medicine) while including subject areas relating to or within the boundaries of management, which reduced the number of articles to 506. The articles were then subjected to an independent screening by all the authors, who reviewed the meta information (title, abstract, keywords). At this final screening stage, all papers were rated from zero to two, based on the relevance to the review’s research objectives.
After rating, 52 papers were given an average rating of 2, indicating a direct link to implementation and 45 papers were rated between 1.5 and 2, indicating a high link to implementation. Another 375 papers were rated below 1.5, showing indirect or only partial relation to implementation. In total, 97 articles rated above 1.5 were included in the review, while the remaining 375 papers were excluded due to limited elaboration on actual implementation or partial data. The pool of 97 articles were predominantly published in the period from 2018 to 2021 (see Figure (a)) as ‘I4.0 implementation’ has only recently surged in popularity. Articles originated from a diverse background, from both developing and developed nations (see Figure (b)). Studies predominantly represent the business domains. Multidisciplinary studies often overlapped with engineering and computer science subject areas, which were also represented (see Figure (c)). From a methodological perspective, most studies (84%) were empirical, including 39 surveys and questionnaires and 23 case studies. The remainder of the papers (16%) was conceptual (see Figure (d)). Lastly, the studies have researched I4.0 at different units-of-analysis, including several at the individual and asset level, but mostly at the organisation level and rarely at the industry, plant, or group level (see Figure (e)).
Figure 2. (a) Cases by year of publication, (b) cases by country of origin, (c) cases by subject area, (d) cases by methodology, (e) units-of-analysis.
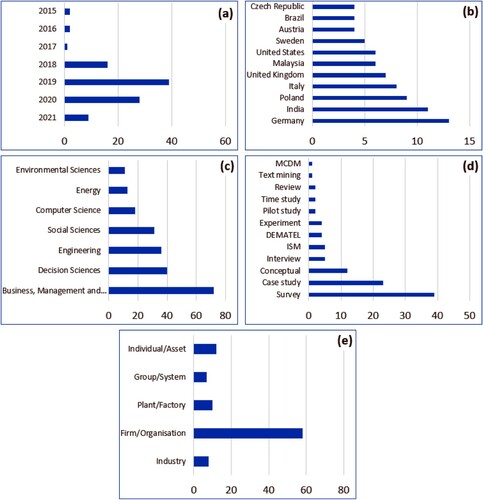
2.3. Text mining
The data extraction stage included the text-mining operation, consisting of three sub-stages of pre-processing, the text-mining operation, and clustering of themes. The pre-processing stage aimed to clean the data presented in the articles to reveal the relevant textual data for the next stage of the text-mining process. For the purposes of this research, figures and images as well as the data contained within brackets ‘()’ and braces ‘’ were removed, as this presented irrelevant information like references. Also, due to the range of similar terms with the same meaning throughout the corpus, terms were thematically substituted and represented by single keywords (e.g. firm and company). Similarly, a number of plural and singular versions, as well as acronyms, were standardised and replaced with single word terms to avoid duplicate results. In addition, to simplify the synthesis process, potentially redundant or duplicated segments scattered across the documents (e.g. references or journal details) were excluded from the synthesis.
Following the pre-processing stage, the processed text was imported into Wordstat (QDA miner add-on software) for quantitative full-text analysis. In order to clarify the existing themes within the corpus, the keyword frequency analysis (limited to 100 results) and phrase frequency was reviewed to illustrate the most frequent terms and phrases within the corpus. This was accomplished by examining the keyword-in-context for every term, which exposed the list of case segments (article sections) from which the term had originally been extracted. In addition, the term frequency-inverse document frequency (TF*IDF) of every keyword was reviewed to distinguish the keywords with high frequency, yet low case occurrence. Topic extraction and keyword levelling revealed the relation of similar terms that had the same meaning or are used within the same context, characterising the content of the corpus (Ananiadou et al. Citation2009). Building a topic model for identifying levels based on keywords and sentence coherence across the full spectrum of the topics discussed within the corpus allowed for a more accurate interpretation of the management complexities.
2.4. Qualitative analysis and synthesis
The qualitative review of individual cases was divided into two sequential steps. Initially, the abstracts of the papers were reviewed to clarify the diverse spectrum of studies and the main topics discussed. This was followed by a systematic and critical review to determine the aim of the study, clarify the methodology, and assess the findings of the paper by carefully reading through the body of the text. Synthesis remains a complex process in the review as it is necessary to describe the set of the research identified, assess the reliability of the research outcomes, combining similar findings into groups (Ananiadou et al. Citation2009).
2.5. Reporting and dissemination
Multi-document summarisation aims to extract and condense the most salient information collected during the text mining and review stage (Okazaki, Matsuo, and Ishizuka Citation2005). A summary of the most predominant levels represented by the principal research streams is presented. For the purpose of this research, particular attention has been paid to following a coherent article structure, choosing the right balance between breadth and depth, and focusing on concepts (concept-centric) by thematically structuring the review section as opposed to a chronological or alphabetical structure of the extant publications (Fisch and Block Citation2018).
3. Results
According to Wordstat collection statistics, a total of 21,046 sentences, 216,870-word forms (tokens), and 926-word types have been analysed. The keyword frequency results (see Table ) show the keywords based on the highest frequency representing the importance of a word within the context of the larger corpus. The higher the TF*IDF the scarcer a term is within the corpus, yet it does not denote an importance level for any specific term.
Table 1. Top 50 term results based on frequency.
To further clarify the relation of the keywords beyond the frequency list, a dendrogram analysis was undertaken. Removing the word levels that consist of fewer than nine words reveals the groups of terms highly related throughout the corpus. Levels within the dendrogram results show three predominant research levels. The top-level linked management principles like lean production and lean supply chains with technologies. The second level highlighted the interconnection of the industry level impact of I4.0 and the performance outcome at the factory level. In comparison, the third level grouped I4.0 technologies, but only those on the factory floor (Figure ).
Figure 3. Dendrogram.
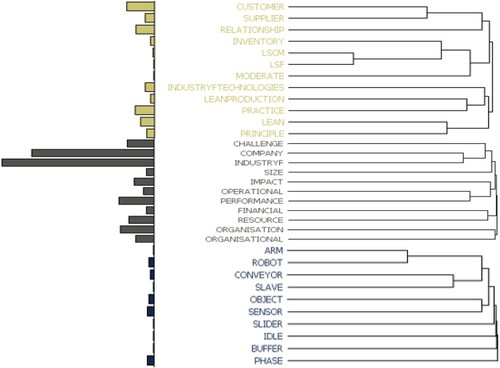
The keyword frequency analysis and the dendrogram analysis illustrate the complex and widespread group of studies and emerging research areas of I4.0. For instance, the top level is formed by twelve similar terms on lean and other management aspects. Similarly, the second level is formed by terms related to the impact of I4.0, followed by the third level, which highlights some technologies. Therefore, to expand beyond the qualitative and single level view of I4.0 implementation it was necessary to have a more in-depth interpretation of the dominant research levels.
3.1. Clustering
For the clustering, a Non-negative Matrix Factorisation (NMF) approach was conducted. A total of twenty research clusters was generated by the text mining based on the coherence of the dominant keywords within each cluster, which were then further grouped together (see Table ). The coherence, a measure of the logical interconnections between the keywords that shape the cluster, and both the frequency and case occurrence, show a high representation of the articles in the corpus, while more clearly distinguishing between streams of research at each level.
Table 2. Clustering of I4.0 literature streams.
4. Literature review
Based on the text-mining analysis, eleven distinct streams of research that are related to the implementation of I4.0 have been identified. The results directly address the first research question: What are the antecedents and outcomes of I4.0 implementation for organisations?
The literature landscape on ‘industry 4.0 implementation’ is represented by five broad levels, namely industry and firm, smart factory, data, human resource and supply chain. The following section presents the insights on the main themes covered by each stream within each of the levels related to the organisation. In addition, indicative references are presented for each sub-theme. The Appendix shows the full list of references attributed to each stream.
4.1. Level 1: industry and firm level
In this section, we summarise and contextualise the drivers of implementing I4.0 at the industry level (Table ) and the organisational enablers at the firm level (Tables and ). Solutions to barriers, as well as management topics, have been increasingly studied in relation to a different aspect of implementation.
Table 3. Industry drivers and barriers.
4.1.1. Stream 1: industry drivers and barriers
Drivers for adopting I4.0 vary due to different government policies and regulations across different regions and countries. The literature distinguishes the relative significance of adopting I4.0 within regional (Pessot et al. Citation2020) and country-specific contexts (Singhal Citation2020) at the industry level. Overcoming unique regional and county-specific barriers inherent in emerging and developed economies requires a context-specific and tailored approach (Alekseev et al. Citation2018; Ślusarczyk Citation2018). For example, government support as a proactive stakeholder (Kumar, Vrat, and Shankar Citation2021) and e-government services (Ślusarczyk, Haseeb, and Hussain Citation2019) can differentiate regional approaches. Low interest rates (Kohnová, Papula, and Salajová Citation2019), and industry specific subsidies (Lin et al. Citation2018) can boost investment in implementing I4.0 (Grenčíková, Kordoš, and Sokol Citation2019). In contrast, boundaries to implementation in the form of overregulation impede technology implementation and manufacturing innovation (Herceg et al. Citation2020; Yu and Schweisfurth Citation2020; Kumar, Vrat, and Shankar Citation2021). Also, universities and other centres of research can be a source of knowledge and cooperation (Szabo et al. Citation2020; Vrchota et al. Citation2021). Within regional markets, SMEs require a different approach compared to large firms (Müller, Kiel, and Voigt Citation2018; Pech and Vrchota Citation2020). Smaller firms within networks were found to be indirectly pressured by partners along the supply chain (Ghobakhloo and Ching Citation2019; Himang et al. Citation2020). SMEs were also found to be pressurised by competitors (Bosman, Hartman, and Sutherland Citation2019; Himang et al. Citation2020) and customers in B2B contexts (Szabo et al. Citation2020) to digitise and implement I4.0. In parallel, technology selection and integration challenge SMEs (Yu and Schweisfurth Citation2020). In addition, empirical studies on regional factories implementing I4.0 to a varying degree were found to adopt a passive stance, despite showing a high willingness to implement the necessary technologies (Ingaldi and Ulewicz Citation2020; Pessot et al. Citation2020; Vrchota et al. Citation2021). This is due to hidden risks, which may endanger current capabilities and jeopardise competitiveness in the market (Bag et al. Citation2021; Ramírez-Durán et al. Citation2021). Lastly, a few studies explored the socio-economic effects of I4.0 across multiple countries. They identified a lack of industry standards and I4.0 strategy as major barriers, partly overcome by lean practices (Tortorella and Fettermann Citation2018; Tortorella et al. Citation2019; Raj et al. Citation2020). Globally, unclear benefits (Ślusarczyk Citation2018; Alekseev et al. Citation2018) hamper implementation while regionally organisations vary in their approach. Eastern European studies found the need for technology competence and the shortage of skilled human resource as challenges (Kohnová, Papula, and Salajová Citation2019; Szabo et al. Citation2020). In comparison, western European regions had a lack of clear digital vision (Pessot et al. Citation2020). SMEs were widely studied in other European countries (Chiarini, Belvedere, and Grando Citation2020), Asian (Ghobakhloo and Ching Citation2019) and African regions (Salimon et al. Citation2019).
4.1.2. Stream 2: organisational enablers of I4.0
The review of organisational enablers (Table ) considered a spectrum of industry and firm challenges inherent in the implementation of I4.0. For instance, studies attribute flattening organisational structures (Jerman, Pejić Bach, and Aleksić Citation2020) and therefore managerial hierarchy (Veile et al. Citation2019) to widening the span of control (Cimini et al. Citation2020). This has raised the need for leadership and experience with digital change (Johansson et al. Citation2019; Vrchota et al. Citation2021). Also, ever-increasing decentralisation of structures may require organisations to spin-off business units (Veile et al. Citation2019) to improve agility across departments (Butt Citation2020). However, although structural and cultural changes have been considered as key organisational enablers for the implementation of I.4.0, there is as yet a lack of understanding about whether new I4.0 teams or new departments should be prioritised. Our analysis found a few studies on value creation but none on value protection. Beyond traditional corporate communication methods such as internal social media (Hauer, Harte, and Kacemi Citation2018), alignment is contingent on the level of access to information (Salimon et al. Citation2019). High-level information sharing can display performance improvement data (Robert et al. Citation2020) and accelerate the sharing of ‘soft resources’ like documents and software (Wagire et al. Citation2021). Understandably, resource sharing needs to be regulated so as to reduce cybersecurity risks (Raj et al. 2020).
Table 4. Organisational enablers of I4.0.
In addition to structure, studies attribute organisational enablers to the culture of the firm. Promoting and fostering a culture of innovation (Barata, Rupino Cunha, and Coyle Citation2019) improves internal culture (Wagire et al. Citation2021; Bag et al. 2021) and makes it possible for firms to overcome regional social-cultural barriers (Kumar, Vrat, and Shankar Citation2021). For instance, openness and willingness to embrace change enables information sharing (Pfeiffer, Lee, and Held Citation2019) and affects the knowledge development process (Kohnová, Papula, and Salajová Citation2019). Successfully introducing new I4.0 communication technologies hinges on the initial reconfiguration of both organisational culture and structures (Ślusarczyk, Haseeb, and Hussain Citation2019; Cimini et al. Citation2020).
Alternatively, to overcome rigid organisational structures and culture and a lack of ICT technology, absorptive capacity and open innovation have been increasingly studied as organisational enablers. For example, Yu and Schweisfurth (Citation2020) pointed to absorptive capacity as an enabler for organisational capability to sense, evaluate and learn from external sources of information in ICT implementation. In addition, open innovation can encourage new resource acquisition by involving the end-user and customers at the manufacturing stage (Gerlitz Citation2015; Prause Citation2015; Pfeiffer, Lee, and Held Citation2019; Himang et al. Citation2020), especially for SMEs (Prause Citation2015). However, there have been no studies on the balance of openness (Himang et al. Citation2020) and the degree of indigenous R&D (Kumar, Vrat, and Shankar Citation2021) as implementation unfolds. In the same vein, the effective implementation of sharing technologies (Chiarini, Belvedere, and Grando Citation2020) enables active resource sharing and facilitates the exploration of new services and streams of revenue (Calabresee et al. Citation2021). Resource sharing is commonly followed by the exploitation of value propositions based on parallel innovation of both technology and management. The reconfiguration of tangible and intangible resources under different business conditions is an important aspect of I4.0, especially in the understudied Socio Technical Systems (Sony and Naik Citation2019; Pollak et al. Citation2020). In this context, there was only one study on dynamic capabilities as a strategy for resource reconfiguration scenarios (Bag et al. 2021).
4.1.3. Stream 3: organisational readiness and maturity
At the firm level, the next stream reviews studies that have examined the organisational readiness and maturity for implementing I4.0, as summarised in Table .
Table 5. Organisational readiness and maturity.
Regardless of organisational and industry enablers, firms are urged to assess the readiness for implementing I4.0 by quantifying the current maturity of the organisation. The literature differentiated between readiness assessments and maturity models for I4.0, assisting managers and practitioners in accordance with legal, ethical and contractual requirements (Saengchai and Jermsittiparsert Citation2019; Kumar, Vrat, and Shankar Citation2021). Assessing the preparedness of a firm aiming to implement I4.0 has been characterised as an important tool to ‘test the readiness for initialisation of the digitalization process’ according to Herceg et al. (2020). Yet there were few empirical studies in this area. For example, Črešnar et al. (Citation2020) surveyed and presented empirical evidence about the importance of traditional management tools like balanced scoreboards and customer segmentation, as important readiness factors. Other studies examined the preparations for implementation based on system thinking (Simetinger and Zhang Citation2020).
I4.0 maturity models (MM) in comparison received more attention, due to the increasing contribution to practice as a more applicable guideline and tool for managers in developing frameworks and roadmaps for change (Liebrecht et al. Citation2021). Comparison of I4.0 maturity models defined the logical dependencies which have to be overcome (or ‘breaking point’) in order to reach a higher level of I4.0 maturity (Simetinger and Zhang Citation2020).
Our analysis found studies broadening the maturity indicators beyond the smart factory and I4.0 technologies by including organisational factors, people, culture, and strategy measures (Bibby and Dehe Citation2018; Wagire et al. Citation2021; Herceg et al. 2020). In contrast, the levels of implementation have been measured less frequently based on management indicators like decision-making, resource allocation, strategy, and policy formulation (Himang et al. Citation2020).
Inevitably the increasing maturity of manufacturers implementing I4.0 changes how I4.0 is measured and how the business model evolves. For example, the degree of implementation and the actual delivery system/methods were found to be highly interdependent (Butt Citation2020). This raised the need for standardised implementation protocols and alignment and governance to better determine performance (KPI), risk (KRI), and process (PPI) indicators. KPIs and strategic impact factors for implementation were based on cost, time, quality, employees, and flexibility (Liebrecht et al. 2021). They were defined at, and were limited to, single business units (Pessot et al. Citation2020). Implementation, however, was not only linked to process management, but many other factors, such as production planning and control, logistics, supply chain management, cybersecurity and customer support (Narula et al. Citation2020).
To this end, the implementation of pilot projects was deemed advantageous in terms of overcoming maturity model breaking points (Veile et al. Citation2019; Pollak et al. Citation2020; Kumar, Vrat, and Shankar Citation2021) as a reversible test environment, minimising disruptions to operations (Butt Citation2020). For instance, the implementation of I4.0 has been linked to an increased level of R&D (Lin, Wu, and Song Citation2019), which if localised to pilot projects can help identify project-specific budget and resource requirements (Prause Citation2015; Veile et al. Citation2019). The early stages of technology implementation were found to benefit from guided ‘exploration projects’ and pilot programmes, and limited investment to allow integration and challenges to drive innovation and the scalability of technology (Ghobakhloo Citation2020b).
4.2. Level 2: smart factory
4.2.1. Stream 4: industry 4.0 technologies
Enabling technologies can significantly drive the implementation of I4.0. In this section we briefly (as this is not the main focal point of this review) consider the technologies underpinning I4.0 at the smart factory level, dividing them into two groups, namely information and digital technologies (IDT) and manufacturing technologies, as shown in Table .
Table 6. I4.0 ICT and manufacturing technologies.
Studies in the first group compared different implementation techniques for IDTs. For instance, the Internet of Things (IoT) and Big Data Analysis (BDA) amplify the firm’s data capabilities by ‘developing the [communication] protocols for the heterogeneous devices’ (Rajput and Singh Citation2019b) and ‘improving predictions of customer needs’ for technology and manufacturing companies (Oncioiu et al. Citation2019). Similarly, Artificial Intelligence (AI), neural networks and machine learning have often been linked to I4.0 implementation. They have been widely regarded as a critical tool to improve the planning and logistics of the factory (Rakyta et al. Citation2016; Ellefsen et al. Citation2019). For instance, neural-pseudo networks have been found to improve production planning (Fertsch Citation2020), augmenting current IT systems, while machine learning has introduced a higher level of predictability and self-configuration in production planning and control by predicting and maintaining, or adjusting to, optimal production conditions (Ellefsen et al. Citation2019; Rauch, Dallasega, and Unterhofer Citation2019; Konur et al. Citation2021). Data-intensive technologies are augmented by high-performance computing (Ghobakhloo and Ching Citation2019; Calabrese et al. Citation2021) and further augment simulation capabilities (Urban et al. Citation2020), like ‘virtual testing’ (Narula et al. 2020) and discrete event simulation (Yazdi, Azizi, and Hashemipour Citation2019). Also, virtualisation is facilitated by virtual and augmented reality (AR/VR) to provide services for both users and clients (Pech and Vrchota Citation2020; Ramírez-Durán et al. 2021).
Increasing mobility and the decentralisation of technologies have been found to intensify cyber-attacks. Security risks can be mitigated through data protection measures (Stentoft and Rajkumar Citation2020) by read-only access to production control data (Konur et al. 2021) and Blockchain technology (Bibby and Dehe Citation2018; Wagire et al. Citation2021). In fact, cyber security is regarded as a prerequisite to other I4.0 IDT technologies (Yu and Schweisfurth Citation2020; Calabrese et al. Citation2021). Existing technologies, such as the Manufacturing Execution System and enterprise resource planning, are found to be a reliable foundation for implementing more advanced technologies (Bibby and Dehe Citation2018; Sader, Husti, and Daróczi Citation2019; Ghobakhloo and Ching Citation2019). Also, cloud computing capabilities are interlinked with big data analytics, in turn improving real-time connectivity and traceability across the supply chains (Bibby and Dehe Citation2018), facilitating cloud manufacturing (Calabrese et al. 2021) as well as enhancing cybersecurity by developing a cloud policy (Pessot et al. Citation2020).
The growing maturity, accessibility and affordability of present manufacturing technologies also enable the implementation of I4.0. For example, advanced sensors are widely used to collect data (Magalhaes, Lugli, and Pimenta Citation2020; Pech and Vrchota Citation2020) leveraging sensor efficiency and capability (Sanghavi, Parikh, and Raj Citation2019). Also, autonomous robots are used in product development and production (Stentoft and Rajkumar Citation2020; Yu and Schweisfurth Citation2020), implemented after changing the plant layout (Chiarini, Belvedere, and Grando Citation2020). In addition, controllers (e.g. PLC, DCS, SCADA) monitor the production and display critical massages (Rakyta et al. Citation2016; Ghobakhloo and Ching Citation2019), making necessary corrective adjustments (Konur et al. 2021). Similarly, sliders (Yazdi, Azizi, and Hashemipour Citation2018) and automated guided vehicles automate shop-floor transportation and speed up material handling (Rakyta et al. Citation2016; Sanghavi, Parikh, and Raj Citation2019). In contrast to subtractive manufacturing, additive manufacturing (e.g. 3D printing) has been increasingly studied in relation to reducing the inventory (Turner et al. Citation2019), enabling rapid prototyping (Wagire et al. Citation2021; Pech and Vrchota Citation2020), and customisation (Devi et al. Citation2021). Lastly, computer-aided design (CAD) tools and computer numerical control (CNC) machines are used as base technologies to simplify the flow of technological documentation during production (Urban et al. 2020), but also for visualising products and the production process (Ramirez-Duran et al. 2021).
Although the literature has yielded rich descriptive insights about the broad range of technologies that could be used during the implementation of I4.0, yet there is a lack of understanding about the decision-making processes of either buying these technologies from suppliers or developing some of them in-house to ensure the protection of value and minimise the risks of knowledge misappropriation. In addition, the literature does not elaborate on the value protection strategies that are applied during the implementation of I4.0. These questions are important from an organisational perspective (Teece Citation2018). We differentiate the enablers of I4.0 at the organisation level, which we have reviewed in Stream 2, from the enablers at the level of the smart factory, expounded below and summarised in Table .
Table 7. Smart factory enablers to I4.0.
As a general low-tech solution, lean practices such as lean six sigma (Sony Citation2020) have been widely discussed when it comes to implementing the smart factory (Sjödin et al. Citation2018). In fact, lean and agile production have been discussed as enablers of I4.0 implementation (Chiarini, Belvedere, and Grando Citation2020) regardless of the socio-economic conditions or firm size (Tortorella and Fettermann Citation2018; Tortorella et al. Citation2019). Even within developed nations lean operation and thinking has been characterised as ‘a solid behavioural and processes foundation on which technologies can foster another layer of improvements’ (Tortorella et al. Citation2019). Lean and agile strategies are a necessity for larger firms (Zangiacomi et al. Citation2020) and to simplify implementation and the trade-off between organisational efficiency and effectiveness for SMEs (Cimini et al. Citation2020). In this context, I4.0 implementation has not only been enabled by lean practices with customers and suppliers (Hotrawaisaya, Pakvichai, and Sriyakul Citation2019), but also through lean manufacturing (LM) or lean production (LP). For instance, several elements of Lean Manufacturing, like Just-In-Time (e.g. pull system), Jidoka, and heijunka can improve process optimisation (Sanders, Elangeswaran, and Wulfsberg Citation2016) and promote I4.0 technology use (Rosin et al. Citation2019; Ghobakhloo and Fathi Citation2019). However, lean manufacturing has also been advantageous in reaching a specific readiness level for implementation (Črešnar et al. Citation2020). Equally, Lean Production emphasises the technological aspects of being lean, including Human Computer Interaction (HCI), production optimisation and reconfigurability (Jiwangkura and Sophatsathit Citation2020). Lean culture has been found to be advantageous in organisational restructuring, necessitated by new technology adoption (Cimini et al. Citation2020). In addition, lean and agile thinking ultimately define the degree of continuous improvement of factory operations across departmental silos (Saabye et al. Citation2020; Raj et al. 2020) aiming to improve productivity (Robert et al. 2020), implement Total Quality Management and ultimately enhance customer satisfaction (Sader, Husti, and Daróczi Citation2019; Črešnar et al. Citation2020).
We further found standardisation (e.g. device, process, communication) and the appropriateness of infrastructure (Rajput and Singh Citation2019b; Birkel et al. Citation2019; Pfeiffer, Lee, and Held Citation2019) to be smart factory enablers. Standardisation improves competitiveness (Müller, Kiel, and Voigt Citation2018), cybersecurity (Singh and Bhanot Citation2020), interoperability (Sanghavi, Parikh, and Raj Citation2019; Konur et al. 2021) and ultimately vertical integration (Simetinger and Zhang Citation2020).
Technology competency and integration also act as important enablers of the smart factory, especially for SMEs integrating I4.0 technologies within their core competencies (Yu and Schweisfurth Citation2020). For instance, IDT knowledge competency can speed up smart manufacturing information and the adoption of digital technologies (SMIDT) and I4.0 implementation (Ghobakhloo and Ching Citation2019). Retrofitting is also considered highly complex (Müller, Kiel, and Voigt Citation2018), yet valuable as a tool, when it comes to reducing the cost of implementation (Birkel et al. Citation2019). To this end, there were studies on competencies which define the firm’s factory resource reconfiguration by means of Dynamic Capability (Bag et al. 2021). Yet there were only two studies on transforming legacy systems in a traditional factory setting (Ramírez-Durán et al. 2021; Konur et al. 2021).
Lastly, there were a few studies on harmonising the factory with its logistical component. In this context, the integration of I4.0 technologies has been found to ‘bridge the gap between logistic enterprises’ performance and shared knowledge and communication’ (Ślusarczyk, Haseeb, and Hussain Citation2019) for both inbound and outbound logistics (Herceg et al. 2020). Other studies investigated AI for logistics (Ellefsen et al. Citation2019), automated guided vehicles (Rakyta et al. Citation2016) and autonomous mobile robots (Chiarini, Belvedere, and Grando Citation2020).
4.2.2. Stream 5: effect of industry 4.0 on factory performance
As summarised below in Table , the successful implementation of I4.0 is attributed to several beneficial outcomes related to improved performance and productivity.
Table 8. Effect on factory performance.
Our analysis uncovered several productivity-related outcomes of implementation. Paperless manufacturing and order visualisation were found to reduce production costs (Liebrecht et al. 2021), while e-value chains aim to reduce lead times and therefore minimise inventory costs (Bibby and Dehe Citation2018). Similarly, improving real-time analysis can increase production performance by 10% (Saaybe et al. 2020). Also, cloud operated hybrid supply chain models could potentially save up to 30% of spending, due to the transparent and lean ordering and delivery of supplies (Sundarakani et al. Citation2019).
Programmable manufacturing advisors can inform managers on issues related to bottlenecks, settling time and lead time (Alavian et al. Citation2020; Ramírez-Durán et al. 2021). Semi-autonomous systems based on monitoring, virtualising and visualising factory operations address throughput losses (20–30% in manufacturing), which commonly result in overtime (Alavian et al. Citation2020). Rapid prototyping has been found to improve the agility of production (Rauch, Dallasega, and Unterhofer Citation2019) and contribute to workers’ creativity, due to more broad design possibilities (Črešnar et al. Citation2020). In addition, integration with customer and supplier processes can improve the sharing of information and best practices (Wagire et al. Citation2021; Himang et al. Citation2020). Involving stakeholders is further linked to performance gains, such as lead and delivery time, product quality and logistics costs (Chiarini, Belvedere, and Grando Citation2020). The use of sensors, cloud, and BDA can improve product quality and consistency, by reducing often risky manual decision-making (Konur et al. 2021). In this context, the virtualisation of one production line predicted the best conditions for other lines and future facilities (Nguyen and Luu Citation2020). Similarly, implementing highly interconnected, yet energy inefficient ‘IoT ecosystems’ can improve manufacturing performance (Ślusarczyk, Haseeb, and Hussain Citation2019; Singh and Bhanot Citation2020).
Other experimental time studies (observations made over a time period) identify Overall Equipment Efficiency (OEE) as an example of a standard for evaluating manufacturing productivity (Yazdi, Azizi, and Hashemipour Citation2018; Yazdi, Azizi, and Hashemipour Citation2019). For example, linking the availability, performance and OEE of individual resources of a system resulted in a 10% increase in OEE due to I4.0 implementation (Pessot et al. Citation2020). Preventative maintenance is another increasingly important example of internal service provision related to the maintenance that needs to be provided as a service prior to failure, based on data analysis (Alavian et al. Citation2020; Singhal Citation2020; Konur et al. 2021). This reduces machine down time due to failure, adding to the number of active machines and improving capacity utilisation (Rakyta et al. Citation2016).
The benefits of implementing I4.0 have similarly been associated with agility. Specifically, the feedback loop was found to be reduced by 25% and product development and production cycle times were reduced by 30% to improve the flow of data through the implementation of VR, CAD, CNC (Urban et al. 2020). Improved cycle times, in turn, reduce production time by 10–90% and time-to-market by 30–90%. Flexibility has also been found to be improved through the implementation of ‘lean-digitized manufacturing’ (Ghobakhloo and Fathi Citation2019), linked to improved product mix (Alavian et al. Citation2020). In general, greater enhancement of performance is expected for SMEs due to simpler business structures, high leverage, agility, and competitiveness (Rauch, Dallasega, and Unterhofer Citation2019). In contrast, larger companies have a greater probability of success, due to a more diverse set of resources (Lin, Wu, and Song Citation2019) and strategic log-term oriented business models (Müller, Kiel, and Voigt Citation2018). For example, implementation for large companies was linked to mass customisation of multiple individualised products simultaneously (Pessot et al. Citation2020; Devi et al. Citation2021). While the above studies have shown the direct effect of I4.0 on performance, there are also many factors at various levels (i.e. firm and factory) that may moderate the strength of this relationship. The role of such moderators at the firm and factory levels is not well-understood.
4.2.3. Stream 6: effect of industry 4.0 on factory sustainability
Beyond production performance gains, implementing I4.0 has been linked to greater sustainability gains (Table ). For example, experimental studies identified sustainable enterprise resource planning as a tool to harmonise implementation steps (initiation, plan, execution, monitoring/control, closure) at multiple levels (Chofreh et al. Citation2020). To this end, sustainable enterprise resource planning (ERP) has been linked to reducing energy consumption and carbon emissions by up to 40%. Likewise, implementation has been linked to reduced land and water usage (Yazdi, Azizi, and Hashemipour Citation2018; Yazdi, Azizi, and Hashemipour Citation2019), lower energy consumption (Urban et al. 2020; Konur et al. 2021), higher potential to use renewable sources of energy (Vrchota et al. Citation2021; Pessot et al. Citation2020), and reduced air emissions (Rajput and Singh Citation2019a; Narula et al. 2020). Yet, for many firms, flexibility and automation have been prioritised over sustainability (Pessot et al. Citation2020). The release of emissions and the factories’ carbon footprint was referred to, but not empirically backed (Gerlitz Citation2015; Rajput and Singh Citation2019a; Birkel et al. Citation2019). In contrast, there was only one study on reducing waste leakage and loss, reducing the cost for the operation of technical equipment and machines and improved thermal energy usage (Vrchota et al. Citation2021).
Table 9. Effect on factory sustainability.
4.3. Level 3: data
4.3.1. Stream 7: big data analytics
The data level highlighted studies on the data management capabilities and requirements, collected at various stages, yet in different formats and quality. As shown in Table , the implementation of I4.0 is fuelled by data from across the lifecycle. Managing the flow of data (acquisition, transfer, storage, analysis) creates new activities such as data-driven customer services (Narula et al. 2020; Ramírez-Durán et al. 2021), internal services in the form of predictive maintenance (Narula et al. 2020) and automated services like machine-to-machine communication (Müller Citation2019; Sanghavi, Parikh, and Raj Citation2019).
Table 10. Big data analytics.
In terms of data collection, embedded devices can ‘interact with the surrounding environment and have the capability to store and share data on their status and their use during their entire lifecycle’ (Arcidiacono et al. Citation2019). However, this requires suitable information bridging technology like IoT data infrastructure (Tarifa-Fernández, Sánchez-Pérez, and Cruz-Rambaud Citation2019; Rajput and Singh Citation2019b) to reduce irrelevant information flow (Sjödin et al. Citation2018). We found that this also improves data consistency (Jiwangkura and Sophatsathit Citation2020) and in some cases maintains a post-usage repository of data with the product information (Rajput and Singh Citation2019b). Few firms have a dedicated data management department, while most of the data is either not analysed, examined ad hoc by employees, or analysed by embedded software functions (Pessot et al. Citation2020). Interestingly, data flow within digital communication networks was relatively high (67–74% respectively) in upstream and downstream value chains, with a similar level of digitisation across firms (Pessot et al. Citation2020). Equally, intra-firm communication has benefitted from setting up web communities and internal social media platforms (Veile et al. Citation2019). Increased availability of mobile technology, agent-based systems, and the augmented capabilities of internal wireless networks improves decentralised data collection within production lines (Barata, Rupino Cunha, and Coyle Citation2019). In this context, the industrial Internet of Things (IIoT) enhanced human-machine and machine-to-machine (M2M) communication (Wilkesmann and Wilkesmann Citation2018), enabled by advanced sensors, and fast, but not real-time, 5G networks among other technologies (Ellefsen et al. Citation2019; Ghobakhloo and Fathi Citation2019).
IoT and BDA were a prerequisite for large scale data processing and interpretation, enabling more advanced computational and analytical capabilities, such as unstructured data gathering, data formatting, pattern recognition and predictive analytics (Rajput and Singh Citation2019b). IoT ecosystems enable the service-oriented architecture (SOA), in which vendors and I4.0 providers can offer data-centric logistics and maintenance services via the Internet of Services (IoS) (Wang and Wang Citation2018; Ślusarczyk and Haque Citation2019). Other studies emphasised the need to address cyber security and investment issues, but they largely associate BDA with the optimisation of inventory and asset productivity in addition to faster response times and greater integration along the supply chain (Oncioiu et al. Citation2019).
Real-time capability in the context of data management is integral to implementation yet empirically understudied. Most notably, the lack of real-time data capability is due to low maturity and weak integration among technologies (Wagire et al. Citation2021), such as for cloud computing (Birkel et al. Citation2019). Still, real-time technologies, which can be implemented by third-party partners (Pessot et al. Citation2020), have been deemed insufficient. To this end, full utilisation of real-time capability requires ‘second-order problem-solving abilities’ and a supportive learning environment (Saaybe et al. 2020).
Closed-loop supply chains could potentially introduce and expand the digital twin (DT) concept beyond the digital copy of the smart factory per se and implement a shared network of manufacturing resources (Rajput and Singh Citation2019b). DT is an increasingly important tool for visualising (through BDA and simulation) and controlling operations across multiple complex stages. DT is characterised as ‘software representations of assets and processes that contribute to the prediction and optimisation of manufacturing performance’ (Ghobakhloo Citation2020b). In addition, experimental (Wang and Wang Citation2018) and case studies (Gu et al. Citation2019) of electrical and electronics equipment (EEE) reiterate the significance of a universal DT and the integration of lifecycle data from cradle-to-grave. Nonetheless, universal DT propositions remain understudied within other high-tech sectors, while no DT solution for low-tech sectors and SMEs was found. This was partially due to sensitive production stages, which remained concealed and isolated, due to information asymmetry and undefined data sharing boundaries to protect intellectual property (IP) (Wang and Wang Citation2018).
4.3.2. Stream 8: cloud
Cloud computing (CC) is an alternative to rigid internal infrastructure for data management, and recent papers have started to examine its importance in the context of I4.0 (Table ).
Table 11. Cloud.
Cloud technology has been widely considered as a viable option for ‘on-demand network access to a shared pool of configurable resources’ (Bibby and Dehe Citation2018; Sanghavi, Parikh, and Raj Citation2019; Butt Citation2020; Konur et al. 2021). In this context, cloud networks support real-time decision-making for internal services (Bag et al. 2021; Konur et al. 2021). Also, cloud networks provide new customer services (Ramírez-Durán et al. 2021), for instance in Cyber Industrial Networks (Saniuk and Saniuk Citation2018). Specifically, cloud computing is an effective tool to facilitate the implementation of the Industrial Internet of Things by integrating soft resources (Urban et al. 2020; Wagire et al. Citation2021; Calabrese et al. 2021). Cloud computing can reduce data cluttering (Singh and Bhanot Citation2020), in effect enhancing simulation capability (Simetinger and Zhang Citation2020) and enterprise resource planning (Ghobakhloo and Fathi Citation2019). Yet only between 8% (Ingaldi and Ulewicz Citation2020), 20% (Yu and Schweisfurth Citation2020), and 54% of SMEs were found to store data in the cloud, in contrast to 92% for large firms. This is due to a lack of expertise and thrust (Pech and Vrchota Citation2020) and understudied security issues (Bibby and Dehe Citation2018; Singh and Bhanot Citation2020; Birkel et al. Citation2019).
Cloud brokers within hybrid cloud networks connect different departments and functions (Veile et al. Citation2019; Jiwangkura and Sophatsathit Citation2020) for private internal users and external customers (the public) (Wagire et al. Citation2021). For example, the cloud enables the servitisation of platforms to align software and processes with customers, yet not as a tool for performance measurement (Chiarini, Belvedere, and Grando Citation2020). Hybrid cloud platforms based on software sharing across users (‘multitenancy’) and service offering (e.g. Infrastructure as a service or Iaas) enhanced monitoring and the servitisation of complex supply chain networks (Sundarakani et al. Citation2019). Lastly, the cloud enables Just-In-Time (Rosin et al. Citation2019) and DT across the product lifecycle (Wang and Wang Citation2018).
4.4. Level 4: human resources
4.4.1. Stream 9: job profiles and competencies
The human element of implementing I4.0 is increasingly studied in the context of I4.0 implementation as shown in Table .
Table 12. Job profiles and competencies.
The implementation of I4.0 is expected to impact on workers and many aspects of the work environment (Basir, Lian, and Shaharin Citation2019; Grenčíková, Kordoš, and Sokol Citation2019) given the ever more defined competency requirements for Implementation (Barata, Rupino Cunha, and Coyle Citation2019; Marnewick and Marnewick Citation2019; Sony and Naik Citation2019). For instance, I4.0 technologies foster the emergence of innovative new job profiles with more autonomy by ‘combining technical and non-technical competencies’ (Cimini et al. Citation2020). Remote or tele-work is changing working time models (Müller, Kiel, and Voigt Citation2018) while increasing monitoring and automation is shifting working conditions and patterns (Sanghavi, Parikh, and Raj Citation2019; Robert et al. 2020) and therefore workplace design (Veile et al. Citation2019). Nonetheless, automation is often synonymous with job losses and a cause of employee resistance to change, which remains an important implementation issue (Zangiacomi et al. Citation2020; Raj et al. 2020). Resistance slows the learning of new competencies (Ingaldi and Ulewicz Citation2020) and hinders the acceptance of new technology like VR and can reduce the decision-making and problem-solving competencies of shop-floor workers (Chiarini, Belvedere, and Grando Citation2020). In contrast, Cobots (collaborative robots) are more widely accepted. Nevertheless, due to increasing throughput, labour-intensive work down the line also increases (Neumann et al. Citation2021). Resistance is less significant for middle managers, who attribute workforce challenges to a lack of training, but also a lack of management competencies (Herceg et al. 2020). Furthermore, guiding skilled and new members of the workforce throughout I4.0 implementation requires harmonising the human resource strategy (Veile et al. Citation2019). For instance, the collaborative training of the workforce is related to ‘flexibility to adapt to new roles and work environments’ (Kazancoglu and Ozkan-Ozen Citation2018). This in turn is deemed beneficial for evolving the entire workforce of the smart factory (Sjödin et al. Citation2018).
There were other studies addressing the changing role of the worker from the competence perspective. For example, team fluidity across production levels (Pfeiffer, Lee, and Held Citation2019) is linked to promoting the early involvement of employees (Arcidiacono et al. Citation2019; Pfeiffer, Lee, and Held Citation2019) from across industry sectors (Škrinjarić and Domadenik Citation2019; Robert et al. 2020). The formation of such ‘teams of performers’ specifically for high-tech projects (Matyushenko et al. Citation2019) necessitates project management specific competencies. In particular, having a ‘servant-leadership’ style as opposed to an outdated ‘command and control’ strategy simplifies implementation (Marnewick and Marnewick Citation2019; Sony and Naik Citation2019; Herceg et al. 2020; Vrchota et al. Citation2021). In this context, generic competencies more transferable to different roles and departments (Škrinjarić and Domadenik Citation2019) were deemed beneficial. More specifically, soft skills in the form of digital know-how (e.g. software usage, analytics etc.) have been widely related to successful implementation (Raj et al. 2020; Kumar, Vrat, and Shankar Citation2021). Increasing demand for technical skills related to mechatronics, the maintenance of smart systems, process analysis, and bionics increases factory productivity (Jerman, Pejić Bach, and Aleksić Citation2020) and the capability to handle traditional analogue production systems (Ingaldi and Ulewicz Citation2020). At the early stages of implementation, technical competencies are prioritised over personal or methodological competencies, e.g. problem-solving and risk management (Cimini et al. Citation2020).
In addition to the above, companies need to incentivise and retain their skilled workforce such as programmers (Birkel et al. Citation2019) and look for internal talent prior to recruiting externally (Zangiacomi et al. Citation2020). Internal and external training of the workforce has been widely discussed in the literature, regarded as an indispensable part of the I4.0 delivery (Devi et al. Citation2021; Bag et al. 2021). Training should go beyond simple ICT competencies and include interdisciplinary knowledge gained through e-learning and scenario-based as well as traditional on-the-job training and workshops (Veile et al. Citation2019; Škrinjarić and Domadenik Citation2019). Learning formalises the work routine (Saabye et al. Citation2020) during the implementation of I4.0 and requires both technical training (Arcidiacono et al. Citation2019) and soft skills, partly delivered by software (Pech and Vrchota Citation2020). Competencies related to analytics, teamwork, and self-management (Jerman, Pejić Bach, and Aleksić Citation2020; Pessot et al. Citation2020) improve procedural understanding, abstraction abilities and fault and error recovery skills (Kazancoglu and Ozkan-Ozen Citation2018).
Lastly, there were a few studies on workers’ safety. As an example, Human-Machine-Interaction (HMI) requires comprehensive consideration of work safety beyond legal regulations (Veile et al. Citation2019). Likewise, considering human wellbeing in job design can reduce occupational health and physical safety issues (e.g. fatigue, musculoskeletal disorders). We found this to be true for psychological health (e.g. fairness, stress, motivation) and other secondary effects on humans, which system designers and I4.0 implementation teams need to consider (Neumann et al. Citation2021).
4.5. Level 5: supply chain level
4.5.1. Stream 10: horizontal integration
As presented in Table , the implementation of I4.0 is associated with many supply chain outcomes. For example, there were a few studies on modelling tools for supply chain integration, such as the supply chain operations reference model (Gu et al. Citation2019). A number of studies found that implementation improves supply chain agility (Oncioiu et al. Citation2019; Chiarini, Belvedere, and Grando Citation2020) and to a lesser degree supply chain resilience (Saengchai and Jermsittiparsert Citation2019).
Table 13. Supply chain horizontal integration.
Integration of the supply chain ultimately results in the potential relocation of facilities near the customer to shorten the supply chain and mitigate the adverse impact on the environment (Wang and Wang Citation2018; Rajput and Singh Citation2019b). Other studies focused on the customer lifecycle. For instance, the two phases of discovering and shopping, as well as the use and service phase of the customer lifecycle, have been investigated in an SME through the services provided by a client application, integrated with the manufacturing process (Ramírez-Durán et al. 2021). This can enhance the rate and accuracy of continuous and agile customer feedback on product quality (Ghobakhloo and Fathi Citation2019) and therefore better define customer requirements (Barata, Rupino Cunha, and Coyle Citation2019). The level of integration among factories, as well as suppliers and customers, could potentially create digital and interdependent industrial networks (Saniuk and Saniuk Citation2018; Veile et al. Citation2019; Sundarakani et al. Citation2019). However, SMEs in particular are more reluctant to share data within the supply chains, due to the fear of losing bargaining power and data security issues (Arcidiacono et al. Citation2019; Birkel et al. Citation2019).
4.5.2. Stream 11: recycling and remanufacturing
The reuse and recycling of resources enabled by I4.0 technologies have also been considered within the context of sustainability and continuous efforts towards attaining more advanced I4.0 capabilities. For example, some companies provide recycling as after-sales services (Pessot et al. Citation2020). Still, I4.0 enablers have been found to positively moderate the implementation of sustainable manufacturing capabilities (Bag et al. 2021). This is due to product modularity reducing the cost of disassembly (Gu et al. Citation2019) and DT storing data on the remanufactured products (Wang and Wang Citation2018), simplifying the refurbishment and disposal process, and therefore remanufacturing.
A larger view of the efficient utilisation and recirculation of I4.0 material resources is the Circular economy (CE). In this context, implementation ‘resources will remain in the closed loop; thus, the life of the resources will increase’ (Bag et al. 2021). This prolongs the value of the materials, products, and components (Rajput and Singh Citation2019a) especially for facilities near customers (Turner et al. Citation2019) (Table ).
Table 14. Recycling and remanufacturing.
5. Discussion
In the previous sections we analysed the relevant literature using text mining (Section 3), before reviewing the identified research streams. The review also made it possible to identify a number of research gaps, effectively addressing this paper’s second research question. Table organises the studies considered by level and stream to showcase visually the areas potentially requiring further attention.
Table 15. I4.0 implementation literature.
The results indicate a growing interest in the implementation of I4.0 for organisations. Still, there are important levels (such as the Data and Supply chain ones) in which the literature remains relatively underdeveloped. Also, many performance metrics and only a few enablers have been identified at the industry and firm level and smart factory level. Similarly, the Data, HR, and Supply chain levels as well as the streams on readiness and maturity and sustainability show relatively fewer empirical studies. Given the uneven maturity and pace of development across levels, it is not surprising that management theories are infrequently applied in the context of I4.0.
This impedes the inductive and deductive theory building and testing cycle (Eisenhardt and Graebner Citation2007; Yaniv Citation2011). Adopting appropriate theory for future I4.0 research facilitates the accurate abstraction of empirical findings into robust and clear concepts (Suddaby Citation2010) to advance the research agenda on if, when and to what extent I4.0 should be implemented in an organisation. Over time, as the I4.0 implementation literature matures, management and non-management theories have cross-fertilised (Robert et al. 2020) and merged into new, more applicable theories, to support I4.0 due to the high-level abstraction of constructs (Yaniv Citation2011). Given the above strengths and limitations of the literature, it has become apparent that several niche fields could be explored by future research to clarify the implementation issues from an organisational perspective. We identify three avenues for future research below.
5.1. First research avenue: the impact of the pandemic on the implementation of I4.0
The recent Covid 19 pandemic has impacted on operations, disrupted supply chains, and changed working patterns in manufacturing. Recent reports suggest that the pandemic has accelerated the rate of the implementation of I4.0, as digitisation and automation have become a necessity (UBS et al. Citation2020; McKinsey & Company et al. Citation2020) due to factories having to operate with fewer people on site. While some manufacturing sectors experienced lower demand, other manufacturing sectors had to address a surge in demand for personalised products and services. Implementing I4.0 to address the challenges of the pandemic could offer a very insightful avenue for future research.
5.1.1. HR during the Covid-19 pandemic and post-pandemic
As a case, remote working (stream 9) can offer many insights and learning experiences on how to improve productivity during exceptional circumstances such as those experienced during the pandemic. It is also expected that there will be a shift to more flexible and remote working arrangements post-pandemic and the future of work will be transformed more quickly (McKinsey Global Institute Citation2021). However, the I4.0 literature has little to offer on the effect of the pandemic on some of the core technologies of I4.0, the manufacturing workforce and shifting HR strategy. The digital twin technology market, which is one of the core technologies of I4.0, is expected to grow from $3.1 billion in 2020 to $48.2 billion by 2026 (Markets and Markets Citation2020) as more companies are adopting digital twin solutions to increase resilience and optimise resource management.
As digital twin technologies can mimic and replicate real-life spaces, situations and processes in the factory, it allows more flexibility to have fewer workers on the factory shop floor and to manage some of the routine tasks remotely. For companies which have delocalised their production to other countries, there is a higher necessity to visualise the operations and also to enable virtual integration across departments as the challenges of frequent business trips and physical presence on site may persist in the post-pandemic period. Future studies could focus on how the adoption of the digital twin technologies can help companies to develop new work habits and patterns for their workforce, while maintaining production and preserving the workers’ health and safety. Future studies could investigate visualisation and Virtual Reality as a platform to connect with the employees. In the same vein, studies could explore training and learning opportunities using information technologies regardless of the workers’ location. As factories are likely to change the production processes to adapt to the pandemic, re-training of the workforce will be required. Virtual reality can be used as an effective tool and becomes a substitute to traditional training as it has become more difficult to have physical mass training on site.
5.1.2. Supply networks during Covid-19
It is relatively well established that industry I4.0 enhances supply chain integration, as briefly discussed in connection with the supply chain level. Supply chain performance can be improved with I4.0 technologies such as digital twins and IoT to capture, analyse and in almost real-time visualise disruptions along the supply chain (Fatorachian and Kazemi Citation2021). This reduces operation costs and improves flexibility due to higher predictability, giving early adopters of I4.0 technologies an edge in fighting disruptions caused by the pandemic (McKinsey & Company et al. Citation2020). These solutions require large investment and the collaboration of network partners, however. Also, in terms of the logistics of the firm, many questions remain unanswered. For example, future studies could investigate if alternative more cost-effective, yet less mature, solutions such as blockchains, could facilitate the management of digital networks in fast-changing markets. In addition, studies could survey if SMEs and other firms inherently reluctant to share information are more willing to implement IoT ecosystems or other I4.0 technology due to Covid 19. Due to increasing data collection across partner firms, B2B services enabled by I4.0 visualisation and Artificial intelligence could also be investigated. For instance, future case studies could research how decision-making is evolving due to the increasing adoption of data-driven methods (Pappas et al. Citation2018), especially for SMEs.
5.2. Second research avenue: creating and protecting value during the implementation of I4.0
5.2.1. Value creation and value protection
During the implementation of I4.0, value is created at different levels. Value capture mechanisms can be in the form of well-studied cost and productivity gains (stream 5), less studied environmental sustainability gains (stream 6) and even from the rarely studied social sustainability gains. In fact, I4.0 enabled flexible manufacturing (Margherita and Braccini Citation2020) could improve social sustainability by leveraging human capital resources in the organisation (Shet and Pereira Citation2021). However, few studies compare sustainability gains and the trade-off or balance between economic, social, and environmental sustainability in the form of I4.0 value created. Future longitudinal studies could quantify organisations’ or single factories’ sustainable value creation over time during their transformational path. Likewise, other studies could focus on the definability and enforceability of I4.0 environmental sustainability measures for actors along the value chain.
The literature suggests the importance of structural changes to support the implementation of I4.0 (stream 2). Although the importance of structural change has been highlighted in Stream 3, there is a lack of understanding of how either dedicated I4.0 teams or centralised units can support the implementation of I4.0 both in terms of value creation and value protection strategies. Future research could explore further if those conscious adopters of I4.0, having implemented structural and cultural changes within the companies, are in a better position to create and protect value, as opposed to those companies that have implemented industry 4.0 in an ad-hoc way by adopting only a few I4.0 technologies in a fragmented way.
Given the growing number of studies showing that I4.0 is associated with value creation, the question remains unaddressed on the protection of the value and risks of knowledge misappropriation that can emerge during the implementation of I4.0. Previous research in the innovation management literature has highlighted how value creation and value protection are complementary strategies (Teece Citation2018). To this end, researchers could investigate the relational governance between plants or firms and their I4.0 technology providers (stream 10). Studies could identify the difference in supplier performance compared to regular suppliers of raw goods to clarifying the legal framework and dynamics of the relationship over time (Zhang et al. Citation2020) once I4.0 technology is implemented.
For instance, one of the technological areas where value protection is important is the digital twin technologies. Firms have two main options when implementing digital twin technologies. They can either rely on external vendors or develop the technologies internally. There is a lack of understanding in the implementation literature of I4.0 about how each of these different strategies (outsourcing to external suppliers or internal development) impact on value creation and protection. One of the potential risks of over-relying on external providers of digital twins is leakage of data and know-how regarding the internal production processes that form part of the core competencies of the firm. The digital twin literature has elaborated well on the technical development of digital twins (Schleich et al. Citation2017), but from an organisational perspective, there is still a lack of knowledge about the benefits and risks of these alternative strategies to implement digital twins. As there is an uptake of digital twin solutions within companies due to the pandemic, future qualitative research could explore the extent to which these two alternative strategies to implementing digital twins impact on both value creation and protection. In addition, studies could investigate how overprotection of value can hinder the firm from taking risks and embracing new alliances.
5.2.2. Value creation and open innovation
An interesting niche field in this context is the ways in which value can be created in the context of I4.0 and the balance between the indigenous R&D of I4.0 (Kumar, Vrat, and Shankar Citation2021) and outsourcing of I4.0 technologies. As we discussed in the previous section, there is a tension between value creation and value protection in some key areas of I4.0, such as the development of digital twins. This tension and the risks of over-relying on vendors of digital twin solutions lead some companies to build their digital twin solution in-house. However, to speed up the development process and to fill the knowledge gaps, the internal development of digital twins will also require the inputs of external resources from the network. Open innovation has only recently been regarded as an enabling strategy to target and acquire the necessary resources for implementing I4.0 (Himang et al. Citation2020).
Open innovation can help balance the risk of implementation among partners and collaborators to minimise resource waste, but in house or indigenous R&D (Himang et al. Citation2020) prevents overreliance and provides more control over the value created. As already shown in the results section, SMEs are reluctant to share information with partners (stream 10) and large firms due to data protection concerns (stream 4). Closed innovation also increases the firms’ deep understanding of patentable value, which can be licenced by I4.0 technology providers. The open innovation literature has highlighted how many firms have created dedicated open innovation teams to support innovation at the firm level (Mortara and Minshall Citation2011) and the adoption of open innovation practices has become well established in developed countries (Chesbrough and Brunswicker Citation2013). Future research could expand on how open innovation and I4.0 teams can collaborate together for the searching and acquisition of know-how for the implementation of the core technologies of I.4.0. Future research could add to our knowledge when firms transition from an inhouse focus on a specific project development to an open model of innovation. To this end, future studies could investigate the appropriate timing of this switch, which has so far been studied with regard to radical innovation projects (Bahemia, Sillince, and Vanhaverbeke Citation2018). Also, does openness matter if the innovation is gradual or following a brownfield approach, as most I4.0 cases do? Future studies could investigate other factors in the same setting, such as urgency, and external factors necessitating the firm to change the way in which the value is being created (internally or outsourcing).
5.3. Third research avenue: a contingency approach to the implementation of I4.0
Another promising theoretical avenue to explore and further refine the implementation of I4.0 from an organisational perspective is the contingency lens (Sousa and Voss Citation2008). Contingency theory holds that organisations adapt their designs, responses, structures and strategies to maintain a fit with dynamic and changing contextual factors so as to maximise the performance level (Woodward Citation1958; Lawrence and Lorsch Citation1967; Thompson Citation1967; Donaldson Citation2001). From the contingency point of view, there are many ways of implementing I4.0 and a one-I4.0 solution-fits-all does not exist for all firms (Ghobakhloo Citation2020b). In this context, implementation decisions depend on the context and environment. For instance, firm size has been widely discussed throughout the literature as a contingency factor, often accepted as a control variable (Lorenz et al. Citation2020). Other I4.0 contingency factors that have been studied include exportability (global reach), and country context (Szász et al. Citation2020). However, there are other key contingency variables that can influence the relationship between I4.0 and performance level.
5.3.1. Organisational strategy
Strategy has rarely been discussed in the literature and, as a result, many avenues for future research exist on this front. The literature briefly and mostly conceptually discussed readiness and maturity models, typically applicable for short-term pilot-project strategies (stream 3). Nonetheless, long term-term implementation requires planning based on the firm’s business model, which is lacking empirical support in the literature. In this context, business model innovation could be an organisational enabler of I4.0. Specifically, future research could expand on how business model innovation can be an enabler and not just an outcome (Foss and Saebi Citation2017) of implementing I4.0. Future studies could define the concepts of I4.0 for users and providers (as defined by Müller Citation2019) to investigate specific ‘user-centric business models’ for SMEs and ‘provider-centric business models’ for OEMs and multinationals. Empirical studies could further investigate to what degree SMEs benefit from business model innovation as a result of the implementation of I4.0. Other studies could investigate how business models build consensus around a digital strategy among internal stakeholders and external stakeholders such as government and other non-for-profit organisations. Business model innovation for I4.0 shows many benefits that need to be empirically investigated.
5.3.2. Contingency factors at the project/factory level
Most of the studies that have considered the benefits of I4.0 at the firm and factory levels (Streams 5 and 6) have not shed light on the extent to which the implementation of I4.0 in relation to the investment in different technologies can differ across different factories within the same firm. For instance, the types of product being manufactured, and the complexity of production processes are all relevant for the implementation of I4.0, in that they constitute important contingency factors that influence the levels of investment required for I4.0 and its effectiveness. To advance our knowledge on the implementation of I4.0, future research could explore the contingency factors that influence a low level or high level of investment in I4.0 technologies. We argue that there is potentially a high level of variance in terms of the investment of I4.0 technologies across different factories in the same firm due to contextual factors at the factory level and it is likely that a low or moderate level of investment in I4.0 will fit some contexts and factories more than others. Therefore, future research could provide a more granular view of the different ways to implement I4.0 (low, moderate or high levels) by moving from a firm to a project level of analysis so as to capture the contingency factors that influence how I4.0 is implemented in different ways (i.e. level of investment in I4.0 technologies) in different factories.
5.3.3. Leadership
Apart from the factors outlined above that could potentially moderate the relationship between I4.0 and performance level, there are also other project factors such as leadership that can moderate this relationship. Lack of leadership competency has been acknowledged as a cause of not implementing I4.0 (stream 2). Still, studies have not elaborated on how leadership enables implementation (except for Marnewick and Marnewick Citation2019). Leadership style can play a moderating role in the success of technology projects (Thite Citation2000). Future research on leadership could expand on different implementation approaches across departments and distinguish leadership styles and methods specific to particular business functions. Similarly, different firms require different levels of I4.0 technology integration. However, it is not known if different factories operating under one company require custom-made approaches to the leadership of implementation projects. What are the leadership decision-making factors that determine the appropriate I4.0 leadership style for one factory compared to another? Future studies could survey the significance of these factors in balancing exploitative and explorative leadership strategies as implementation matures. Leadership at the project level could also benefit from more research. Future studies could empirically investigate the intellectual stimulation of team members and a ‘digital mindset’ to enable a greater understanding of the lower-level effects (individual and group unit-of-analysis), during and after the enabling of technology integration. For example, it is mostly unknown how Artificial intelligence and manufacturing advisors change leadership styles over time as managers become ever more reliant on technology. Also, it would be interesting to investigate the relationships among project leaders at different hierarchical levels and workers during I4.0 pilot projects and initial stages of implementation to forecast leadership change. In addition, studies could investigate the underdeveloped area of individual leadership style given the increasing flattening of managerial hierarchy and the mobility of the workforce. Allocating greater responsibility to individual employees could make some management roles redundant. Which management roles are at greatest risk and how leadership styles seamlessly transition during implementation remain to be investigated by future studies. In other words, the transition from a leadership style encouraging exploration, in which the leader senses new opportunities, and an exploitative leadership style (value creation) in implementation can be further understood.
6. Conclusion
I4.0 is an increasingly important topic, bringing together knowledge from multiple academic fields into creative solutions for manufacturing innovation. Our review and findings have contributed to the extant literature by offering insights into the organisational aspects of the implementation of I4.0. To this end, we used text mining to analyse 97 academic articles. This was followed by a comprehensive qualitative review of the streams across five levels related to I4.0. The review showed that SMEs approach implementation differently and there is an increasing interest in I4.0 using traditional low-cost methods such as lean management. Several performance indicators, such as productivity and sustainability, were identified. Also, enablers at the factory and firm level were elaborated on. In contrast, we found little empirical evidence across the data and supply chain levels, which remain rather atheoretical. Lastly, the results indicated a critical lack of studies on the organisational issues of I4.0, necessitating further studies across three avenues for future research. Our review aims not only to offer state-of-the art coverage of the relevant literature in the area but also to inform future research projects. The review has provided a comprehensive picture of the I4.0 implementation literature for organisations that helps bridge the gap between theory and practice, by highlighting the main findings related to practice and identifying potential areas for further work. To this end, the review makes a tangible contribution by identifying three overarching areas that future research could tackle. The review also contributes to clarifying I4.0 implementation at different levels such as the organisational, single plant and individual levels of analysis. It suggests that more are needed at the factory, project, and production process levels, since these are the main areas in which I4.0 is experienced from a practitioner’s perspective. Given the very nature of the topic and its implications for practice, managers may find this review of value to prepare and plan for the implementation process. The analysis helps bring closer the academic and practitioner perspectives on I4.0 at five organisational levels. The review concisely presents the key findings related to implementation and offers insights as to what constitutes good practice and how the challenges may be overcome.
When it comes to the limitations of this review, extending the search criteria would have resulted in a larger pool of papers to include. Similarly, a lower inclusion score could have extended the scope of the review and could have potentially offered insights as to the peripheral literature. Still, although analysing more papers using text mining would not have been a significant overhead, qualitative reviewing more papers would have made it a challenging endeavour. Finally, future review papers could focus more specifically on the wider social sustainability and the different stages of the implementation and weight findings depending on the relative impact they have on adopting and operationalising I4.0 technologies and the relative advantage they have.
Disclosure statement
No potential conflict of interest was reported by the author(s).
Data availability statement
The data supporting the findings of this review are available from the corresponding author (Nayernia, H.), upon reasonable request.
Additional information
Notes on contributors
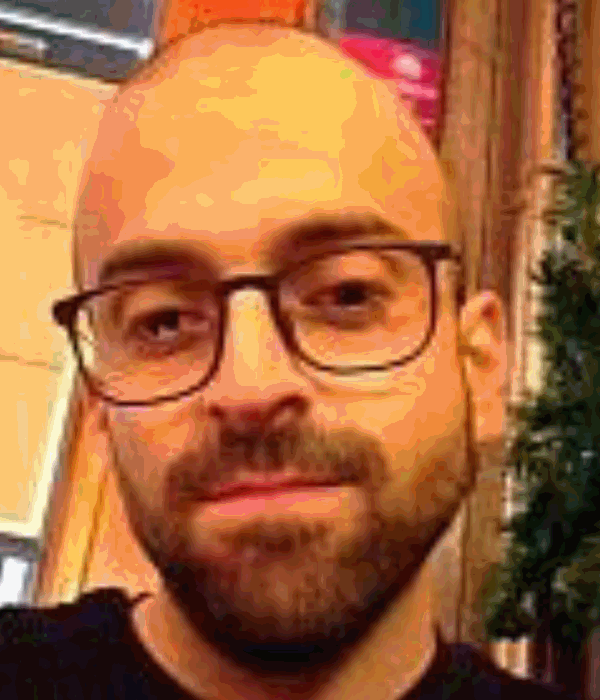
Hamed Nayernia
Hamed Nayernia is a PhD researcher at Newcastle business school with a master’s in engineering management focusing on emerging space technologies and an undergraduate degree in aeronautical engineering. Research interests include implementation of industry 4.0 and digital transformation strategy as well as operations management. He is currently collaborating with industry 4.0 practitioners and academics to empirically measure the causes and enablers of the ongoing industrial transformation and smart manufacturing as well as implications for factory operations and the larger impact on the business, society, and the environment.
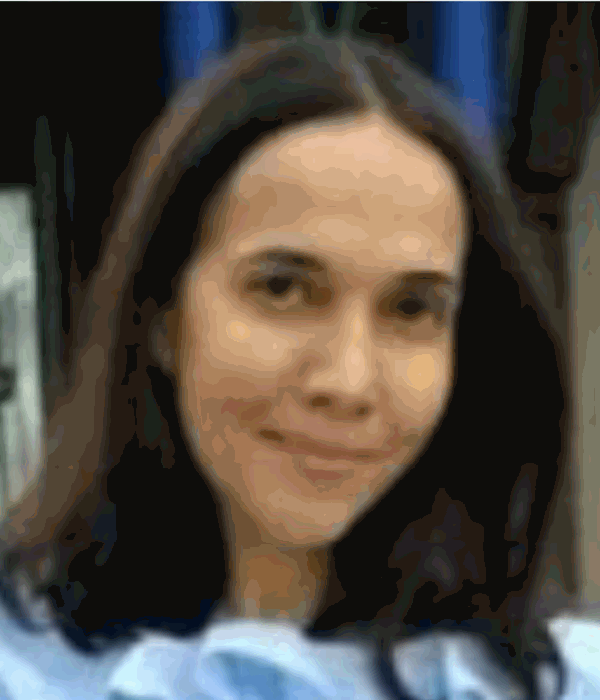
Hanna Bahemia
Hanna Bahemia is a lecturer in Innovation and Technology Management at Newcastle University Business School (UK). She holds a PhD in Innovation Management and MSc in Operations Management from the University of Manchester (UK). Her research links the domain of strategic management, technology and innovation management and manufacturing strategy with a particular focus on open innovation, business model innovation, and industry 4.0. She is currently collaborating with several multinational companies in the UK and Europe, and she is conducting multiple case studies on the challenges of implementing open innovation and industry 4.0 in the automotive, home appliances and publishing industries.
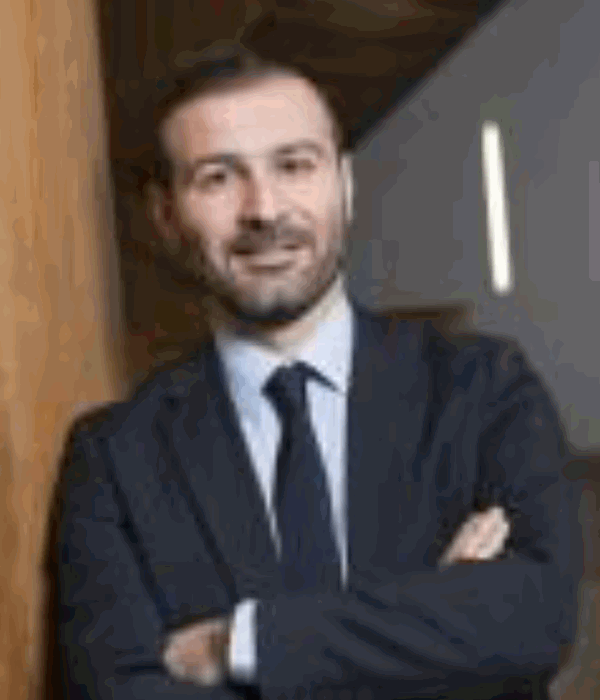
Savvas Papagiannidis
Prof Savvas Papagiannidis is the David Goldman Professor of Innovation and Enterprise at Newcastle University Business School. His research interests revolve around electronic business and its various sub-domains and how digital technologies can transform organisations and societies alike. More specifically, his research aims to inform our understanding of how e-business technologies affect the social and business environment, organisational strategies and business models, and how these are implemented in terms of functional innovations (especially in e-marketing and e-commerce). His work puts a strong emphasis on innovation, new value creation and exploitation of entrepreneurial opportunities, within the context of different industries. Apart from the impact that the Internet and related technologies can have on businesses, he is also very much interested in the impact such technologies can have on individual users.
References
- McKinsey & Company, Agrawal, M., Eloot, K., Mancini, M., & Patel, A. 2020. “Industry 4.0: Reimagining Manufacturing Operations after COVID-19.” https://www.mckinsey.com/business-functions/operations/our-insights/industry-40-reimagining-manufacturing-operations-after-covid-19.
- Alavian, P., Y. Eun, S. M. Meerkov, and L. Zhang. 2020. “Smart Production Systems: Automating Decision-Making in Manufacturing Environment.” International Journal of Production Research 58 (3): 828–845. doi:https://doi.org/10.1080/00207543.2019.1600765.
- Alekseev, A. N., S. Y. Evdokimov, A. Y. Tarasova, K. S. Khachaturyan, and A. A. Khachaturyan. 2018. “Financial Strategy of Development of Industry 4.0 in the Countries with Developing Economy.” Espacios 39: 12. https://www.scopus.com/inward/record.uri?eid=2-s2.085044348944&partnerID=40&md5=3ff52304862308341b0189d07ce43ab2.
- Ananiadou, S., B. Rea, N. Okazaki, R. Procter, and J. Thomas. 2009. “Supporting Systematic Reviews Using Text Mining.” Social Science Computer Review 27 (4): 509–523.
- Arcidiacono, F., A. Ancarani, C. D. Mauro, and F. Schupp. 2019. “Where the Rubber Meets the Road. Industry 4.0 among SMEs in the Automotive Sector.” IEEE Engineering Management Review 47 (4): 86–93. doi:https://doi.org/10.1109/EMR.2019.2932965.
- Bag, S., S. Gupta, and S. Kumar. 2021. “Industry 4.0 Adoption and 10R Advance Manufacturing Capabilities for Sustainable Development.” International Journal of Production Economics 231: 107844. doi:https://doi.org/10.1016/j.ijpe.2020.107844.
- Bahemia, H., J. Sillince, and W. Vanhaverbeke. 2018. “The Timing of Openness in a Radical Innovation Project, a Temporal and Loose Coupling Perspective.” Research Policy 47 (10): 2066–2076.
- Barata, J., P. Rupino Cunha, and S. Coyle. 2019. “Evolving Manufacturing Mobility in Industry 4.0: The Case of Process Industries.” Journal of Manufacturing Technology Management 31 (1): 52–71. doi:https://doi.org/10.1108/JMTM-10-2018-0361.
- Basir, N., O. C. Lian, and H. Shaharin. 2019. “Assessment of Outcome-Based Integrated Design Project.” JOTSE 9 (1): 77–84.
- Bibby, L., and B. Dehe. 2018. “Defining and Assessing Industry 4.0 Maturity Levels–Case of the Defence Sector.” Production Planning and Control 29 (12): 1030–1043. doi:https://doi.org/10.1080/09537287.2018.1503355.
- Birkel, H. S., J. W. Veile, J. M. Müller, E. Hartmann, and K. I. Voigt. 2019. “Development of a Risk Framework for Industry 4.0 in the Context of Sustainability for Established Manufacturers.” Sustainability (Switzerland 11: 2. doi:https://doi.org/10.3390/su11020384.
- Bittencourt, V. L., A. C. Alves, and C. P. Leão. 2021. “Industry 4.0 Triggered by Lean Thinking: Insights from a Systematic Literature Review.” International Journal of Production Research 59 (5): 1496–1510.
- Bosman, L., N. Hartman, and J. Sutherland. 2019. “How Manufacturing Firm Characteristics Can Influence Decision Making for Investing in Industry 4.0 Technologies.” Journal of Manufacturing Technology Management, doi:https://doi.org/10.1108/JMTM-09-2018-0283.
- Butt, J. 2020. “A Conceptual Framework to Support Digital Transformation in Manufacturing Using an Integrated Business Process Management Approach.” Designs 4 (3): 17.
- Calabrese, A., N. Levialdi Ghiron, and L. Tiburzi. 2021. “‘Evolutions’ and ‘Revolutions’ in Manufacturers’ Implementation of Industry 4.0: A Literature Review, a Multiple Case Study, and a Conceptual Framework.” Production Planning & Control 32 (3): 213–227. doi:https://doi.org/10.1080/09537287.2020.1719715.
- Castelo-Branco, I., F. Cruz-Jesus, and T. Oliveira. 2019. “Assessing Industry 4.0 Readiness in Manufacturing: Evidence for the European Union.” Computers in Industry 107: 22–32.
- Chesbrough, H., and S. Brunswicker. 2013. “Managing Open Innovation in Large Firms.” Garwood Center for Corporate Innovation at California University, Berkeley in US & Fraunhofer Society in Germany.
- Chiarini, A., V. Belvedere, and A. Grando. 2020. “Industry 4.0 Strategies and Technological Developments. An Exploratory Research from Italian Manufacturing Companies.” Production Planning & Control 31 (16): 1385–1398.
- Chofreh, A. G., F. A. Goni, J. J. Klemeš, M. N. Malik, and H. H. Khan. 2020. “Development of Guidelines for the Implementation of Sustainable Enterprise Resource Planning Systems.” Journal of Cleaner Production 244. doi:https://doi.org/10.1016/j.jclepro.2019.118655.
- Cimini, C., A. Boffelli, A. Lagorio, M. Kalchschmidt, and R. Pinto. 2020. “How do Industry 4.0 Technologies Influence Organisational Change? An Empirical Analysis of Italian SMEs.” Journal of Manufacturing Technology Management 32 (3): 695–721. doi:https://doi.org/10.1108/JMTM-04-2019-0135.
- Correani, A., A. De Massis, F. Frattini, A. M. Petruzzelli, and A. Natalicchio. 2020. “Implementing a Digital Strategy: Learning from the Experience of Three Digital Transformation Projects.” California Management Review 62 (4): 37–56.
- Črešnar, R., V. Potočan, and Z. Nedelko. 2020. “Speeding Up the Implementation of Industry 4.0 with Management Tools: Empirical Investigations in Manufacturing Organizations.” Sensors 20 (12): 3469. doi:https://doi.org/10.3390/s20123469.
- Dallasega, P., E. Rauch, and C. Linder. 2018. “Industry 4.0 as an Enabler of Proximity for Construction Supply Chains: A Systematic Literature Review.” Computers in Industry 99: 205–225.
- Davlembayeva, D., S. Papagiannidis, and E. Alamanos. 2019. “Mapping the Economics, Social and Technological Attributes of the Sharing Economy.” Information Technology & People 33 (3): 841–872. doi:https://doi.org/10.1108/ITP-02-2018-0085.
- Denyer, D., and D. Tranfield. 2009. The SAGE Handbook of Organizational Research Methods, 671–689. Los Angeles, CA: Sage Publications Ltd.
- Devi K., Sivananda, K. P. Paranitharan, and I. Agniveesh. 2021. “Interpretive Framework by Analysing the Enablers for Implementation of Industry 4.0: An ISM Approach.” Total Quality Management & Business Excellence 32 (13–14): 1494–1514. doi:https://doi.org/10.1080/14783363.2020.1735933.
- Donaldson, L. 2001. The Contingency Theory of Organizations. Thousand Oaks, CA: SAGE Publications, Inc. doi:https://doi.org/10.4135/9781452229249.
- Eisenhardt, K. M., and M. E. Graebner. 2007. “Theory Building from Cases: Opportunities and Challenges.” Academy of Management Journal 50 (1): 25–32.
- Ellefsen, A. P. T., J. Oleśków-Szłapka, G. Pawłowski, and A. Toboła. 2019. “Striving for Excellence in AI Implementation: AI Maturity Model Framework and Preliminary Research Results.” Logforum 15 (3): 363–376. doi:https://doi.org/10.17270/J.LOG.2019.354.
- Erro-Garcés, A. 2019. “Industry 4.0: Defining the Research Agenda.” Benchmarking: An International Journal 28 (5): 1858–1882. doi:https://doi.org/10.1108/BIJ-12-2018-0444.
- Falagas, M. E., E. I. Pitsouni, G. A. Malietzis, and G. Pappas. 2008. “Comparison of PubMed, Scopus, web of Science, and Google Scholar: Strengths and Weaknesses.” The FASEB Journal 22 (2): 338–342.
- Fatorachian, H., and H. Kazemi. 2021. “Impact of Industry 4.0 on Supply Chain Performance.” Production Planning & Control 32 (1): 63–81.
- Fertsch, M. 2020. “Artificial Neural Pseudo-Network for Production Control Purposes.” Logforum 16 (1): 7–13. doi:https://doi.org/10.17270/J.LOG.2020.382.
- Fisch, C., and J. Block. 2018. “Six Tips for Your (Systematic) Literature Review in Business and Management Research.” Management Review Quarterly 68 (2): 103–106. doi:https://doi.org/10.1007/s11301-018-0142-x.
- Foss, N. J., and T. Saebi. 2017. “Fifteen Years of Research on Business Model Innovation: How Far Have we Come, and Where Should we go?” Journal of Management 43 (1): 200–227.
- Frank, A. G., G. H. Mendes, N. F. Ayala, and A. Ghezzi. 2019. “Servitization and Industry 4.0 Convergence in the Digital Transformation of Product Firms: A Business Model Innovation Perspective.” Technological Forecasting and Social Change 141: 341–351.
- Geels, F. W., S. Sareen, A. Hook, and B. K. Sovacool. 2021. “Navigating Implementation Dilemmas in Technology-Forcing Policies: A Comparative Analysis of Accelerated Smart Meter Diffusion in the Netherlands, UK, Norway, and Portugal (2000–2019).” Research Policy 50 (7): 104272.
- Gerlitz, L. 2015. “Design for Product and Service Innovation in Industry 4.0 and Emerging Smart Society.” Journal of Security and Sustainability Issues 5 (2): 181–198. doi:https://doi.org/10.9770/jssi.2015.5.2(5).
- Ghobakhloo, M. 2020a. “Industry 4.0, Digitization, and Opportunities for Sustainability.” Journal of Cleaner Production 252: 119869.
- Ghobakhloo, M. 2020b. “Determinants of Information and Digital Technology Implementation for Smart Manufacturing.” International Journal of Production Research, doi:https://doi.org/10.1080/00207543.2019.1630775.
- Ghobakhloo, M., and N. T. Ching. 2019. “Adoption of Digital Technologies of Smart Manufacturing in SMEs.” Journal of Industrial Information Integration 16), doi:https://doi.org/10.1016/j.jii.2019.100107.
- Ghobakhloo, M., and M. Fathi. 2019. “Corporate Survival in Industry 4.0 Era: The Enabling Role of Lean-Digitized Manufacturing.” Journal of Manufacturing Technology Management 31 (1): 1–30. doi:https://doi.org/10.1108/JMTM-11-2018-0417.
- Grenčíková, A., M. Kordoš, and J. Sokol. 2019. “The Approach to Industry 4.0 Within the Slovak Business Environment.” Social Sciences 8: 4. doi:https://doi.org/10.3390/socsci8040104.
- Gu, F., J. Guo, P. Hall, and X. Gu. 2019. “An Integrated Architecture for Implementing Extended Producer Responsibility in the Context of Industry 4.0.” International Journal of Production Research 57 (5): 1458–1477. doi:https://doi.org/10.1080/00207543.2018.1489161.
- Hajoary, P. K. 2021. “Industry 4.0 Maturity and Readiness Models: A Systematic Literature Review and Future Framework.” International Journal of Innovation and Technology Management 17 (7). doi:https://doi.org/10.1142/S0219877020300050.
- Hanelt, A., R. Bohnsack, D. Marz, and C. Antunes Marante. 2020. “A Systematic Review of the Literature on Digital Transformation: Insights and Implications for Strategy and Organizational Change.” Journal of Management Studies 58 (5): 1159–1197. doi:https://doi.org/10.1111/joms.12639.
- Haseeb, M., H. I. Hussain, B. Ślusarczyk, and K. Jermsittiparsert. 2019. “Industry 4.0: A Solution Towards Technology Challenges of Sustainable Business Performance.” Social Sciences 8: 5. doi:https://doi.org/10.3390/socsci8050154.
- Hauer, G., P. Harte, and J. Kacemi. 2018. “An Exploration of the Impact of Industry 4.0 Approach on Corporate Communication in the German Manufacturing Industry.” International Journal of Supply Chain Management 7 (4): 125–131.
- Herceg, I. V., V. Kuč, V. M. Mijušković, and T. Herceg. 2020. “Challenges and Driving Forces for Industry 4.0 Implementation.” Sustainability 12 (10): 4208. doi:https://doi.org/10.3390/su12104208.
- Hernandez Korner, M. E., M. P. Lambán, J. A. Albajez, J. Santolaria, L. D. C. Ng Corrales, and J. Royo. 2020. “Systematic Literature Review: Integration of Additive Manufacturing and Industry 4.0.” Metals 10 (8): 1061.
- Himang, C., L. Ocampo, J. J. Obiso, M. Bongo, S. A. Caballes, D. P. Abellana, C. Caballes, and R. Ancheta. 2020. “Defining Stages of the Industry 4.0 Adoption via Indicator Sets.” Engineering Management in Production and Services 12 (2): 32–55.
- Hizam-Hanafiah, M., M. A. Soomro, and N. L. Abdullah. 2020. “Industry 4.0 Readiness Models: A Systematic Literature Review of Model Dimensions.” Information 11 (7): 364.
- Hotrawaisaya, C., V. Pakvichai, and T. Sriyakul. 2019. “Lean Production Determinants and Performance Consequences of Implementation of Industry 4.0 in Thailand: Evidence from Manufacturing Sector.” International Journal of Supply Chain Management 8 (5): 559–571. https://www.scopus.com/inward/record.uri?eid=2-s2.0-85074515952&partnerID=40&md5=94c3a607360e577d674eea13fed543ce.
- Ingaldi, M., and R. Ulewicz. 2020. “Problems with the Implementation of Industry 4.0 in Enterprises from the SME Sector.” Sustainability 12 (1): 217.
- Ivanov, D., A. Dolgui, and B. Sokolov. 2019. “The Impact of Digital Technology and Industry 4.0 on the Ripple Effect and Supply Chain Risk Analytics.” International Journal of Production Research 57 (3): 829–846.
- Jerman, A., M. Pejić Bach, and A. Aleksić. 2020. “Transformation Towards Smart Factory System: Examining new job Profiles and Competencies.” Systems Research and Behavioral Science 37 (2): 388–402. doi:https://doi.org/10.1002/sres.2657.
- Jiwangkura, S., and P. Sophatsathit. 2020. “Industrial Internet of Things Implementation Strategies with HCI for Adoption.” International Journal of Automation and Smart Technology 10 (1): 153–168. doi:https://doi.org/10.5875/ausmt.v10i1.2108.
- Johansson, P. E. C., L. Malmsköld, Å Fast-Berglund, and L. Moestam. 2019. “Challenges of Handling Assembly Information in Global Manufacturing Companies.” Journal of Manufacturing Technology Management, doi:https://doi.org/10.1108/JMTM-05-2018-0137.
- Kamble, S. S., A. Gunasekaran, and S. A. Gawankar. 2018. “Sustainable Industry 4.0 Framework: A Systematic Literature Review Identifying the Current Trends and Future Perspectives.” Process Safety and Environmental Protection 117: 408–425.
- Kazancoglu, Y., and Y. D. Ozkan-Ozen. 2018. “Analyzing Workforce 4.0 in the Fourth Industrial Revolution and Proposing a Road map from Operations Management Perspective with Fuzzy DEMATEL.” Journal of Enterprise Information Management 31 (6): 891–907. doi:https://doi.org/10.1108/JEIM-01-2017-0015.
- Kerin, M., and D. T. Pham. 2019. “A Review of Emerging Industry 4.0 Technologies in Remanufacturing.” Journal of Cleaner Production 237: 117805.
- Klingenberg, C. O., M. A. V. Borges, and J. A. V. Antunes Jr. 2019. “Industry 4.0 as a Data-Driven Paradigm: A Systematic Literature Review on Technologies.” Journal of Manufacturing Technology Management 32 (3): 570–592. doi:https://doi.org/10.1108/JMTM-09-2018-0325.
- Kohnová, L., J. Papula, and N. Salajová. 2019. “Internal Factors Supporting Business and Technological Transformation in the Context of Industry 4.0.” Business: Theory and Practice 20: 137–145. doi:https://doi.org/10.3846/btp.2019.13.
- Konur, S., Y. Lan, D. Thakker, G. Morkyani, N. Polovina, and J. Sharp. 2021. “Towards Design and Implementation of Industry 4.0 for Food Manufacturing.” Neural Computing and Applications 14: 778. doi:https://doi.org/10.1007/s00521-021-05726-z.
- Kumar, P., and R. K. Singh. 2021. “Application of Industry 4.0 Technologies for Effective Coordination in Humanitarian Supply Chains: A Strategic Approach.” Annals of Operations Research January: 1–33. doi:https://doi.org/10.1007/s10479-020-03898-w.
- Kumar, V., P. Vrat, and R. Shankar. 2021. “Prioritization of Strategies to Overcome the Barriers in Industry 4.0: A Hybrid MCDM Approach.” Opsearch 58: 711–750. doi:https://doi.org/10.1007/s12597-020-00505-1.
- Lawrence, P. R., and J. W. Lorsch. 1967. “Differentiation and Integration in Complex Organizations.” Administrative Science Quarterly 12 (1): 1–47. doi:https://doi.org/10.2307/2391211.
- Lepore, D., A. Micozzi, and F. Spigarelli. 2021. “Industry 4.0 Accelerating Sustainable Manufacturing in the COVID-19 Era: Assessing the Readiness and Responsiveness of Italian Regions.” Sustainability 13 (5): 2670. doi:https://doi.org/10.3390/su13052670.
- Liao, Y., F. Deschamps, E. D. F. R. Loures, and L. F. P. Ramos. 2017. “Past, Present and Future of Industry 4.0-a Systematic Literature Review and Research Agenda Proposal.” International Journal of Production Research 55 (12): 3609–3629. doi:https://doi.org/10.1080/00207543.2017.1308576.
- Liebrecht, C., M. Kandler, M. Lang, S. Schaumann, N. Stricker, T. Wuest, and G. Lanza. 2021. “Decision Support for the Implementation of Industry 4.0 Methods: Toolbox, Assessment and Implementation Sequences for Industry 4.0.” Journal of Manufacturing Systems 58: 412–430. doi:https://doi.org/10.1016/j.jmsy.2020.12.008.
- Lin, D., C. K. M. Lee, H. Lau, and Y. Yang. 2018. “Strategic Response to Industry 4.0: An Empirical Investigation on the Chinese Automotive Industry.” Industrial Management and Data Systems 118 (3): 589–605. doi:https://doi.org/10.1108/IMDS-09-2017-0403.
- Lin, B., W. Wu, and M. Song. 2019. “Industry 4.0: Driving Factors and Impacts on Firm’s Performance: An Empirical Study on China’s Manufacturing Industry.” Annals of Operations Research, doi:https://doi.org/10.1007/s10479-019-03433-6.
- Lorenz, R., C. Benninghaus, T. Friedli, and T. H. Netland. 2020. “Digitization of Manufacturing: The Role of External Search.” International Journal of Operations & Production Management 40 (7/8): 1129–1152. doi:https://doi.org/10.1108/IJOPM-06-2019-0498.
- Magalhaes, A. Z. C., A. B. Lugli, and T. C. Pimenta. 2020. “Study and Implementation of a Real Industrial Application Involving Concepts of Industry 4.0.” Health 11 (7). doi:https://doi.org/10.24507/icicelb.11.07.623.
- Margherita, E. G., and A. M. Braccini. 2020. “Industry 4.0 Technologies in Flexible Manufacturing for Sustainable Organizational Value: Reflections from a Multiple Case Study of Italian Manufacturers.” Information Systems Frontiers July: 1–22. doi:https://doi.org/10.1007/s10796-020-10047-y.
- Markets and Markets. 2020. “Digital Twin Market by Technology, Type (Product, Process, and System), Application (predictive maintenance, and others), Industry (Aerospace & Defense, Automotive & Transportation, Healthcare, and others), and Geography – Global Forecast to 2026.” https://www.marketsandmarkets.com/Market-Reports/digital-twin-market-225269522.html.
- Marnewick, A. L., and C. Marnewick. 2019. “The Ability of Project Managers to Implement Industry 4.0-Related Projects.” IEEE Access 8: 314–324.
- Marodin, G. A., G. L. Tortorella, A. G. Frank, and M. Godinho Filho. 2017. “The Moderating Effect of Lean Supply Chain Management on the Impact of Lean Shop Floor Practices on Quality and Inventory.” Supply Chain Management: An International Journal 22 (6): 473–485. doi:https://doi.org/10.1108/SCM-10-2016-0350.
- Matyushenko, I., M. Danova, O. Feoktystova, and R. Melnyk. 2019. “Formation of Teams of Performers of Projects at Innovative Enterprises Within the Framework of the Industry 4.0 Concept.” International Journal of Supply Chain Management 8 (4): 962–969. https://www.scopus.com/inward/record.uri?eid=2-s2.0-85071591516&partnerID=40&md5=aa95d74a2421dbf0da8ebaf35d21f0ba.
- McKinsey Global Institute. 2021. “The Future of Work after COVID-19 | McKinsey.” www.mckinsey.com. February 18, 2021.https://www.mckinsey.com/featured-insights/future-of-work/the-future-of-work-after-covid-19.
- Mortara, L., and T. Minshall. 2011. “How Do Large Multinational Companies Implement Open Innovation?” Technovation 31 (10–11): 586–597. doi:https://doi.org/10.1016/j.technovation.2011.05.002.
- Müller, J. M. 2019. “Business Model Innovation in Small-and Medium-Sized Enterprises: Strategies for Industry 4.0 Providers and Users.” Journal of Manufacturing Technology Management 30 (8): 1127–1142. doi:https://doi.org/10.1108/JMTM-01-2018-0008.
- Müller, J. M., D. Kiel, and K. I. Voigt. 2018. “What Drives the Implementation of Industry 4.0?” The Role of Opportunities and Challenges in the Context of Sustainability. Sustainability (Switzerland) 10: 1. doi:https://doi.org/10.3390/su10010247.
- Narula, S., S. Prakash, M. Dwivedy, V. Talwar, and S. P. Tiwari. 2020. “Industry 4.0 Adoption Key Factors: An Empirical Study on Manufacturing Industry.” Journal of Advances in Management Research 17 (5): 697–725. doi:https://doi.org/10.1108/JAMR-03-2020-0039.
- Negri, E., L. Fumagalli, and M. Macchi. 2017. “A Review of the Roles of Digital Twin in cps-Based Production Systems.” Procedia Manufacturing 11: 939–948.
- Neumann, W. P., S. Winkelhaus, E. H. Grosse, and C. H. Glock. 2021. “Industry 4.0 and the Human Factor – A Systems Framework and Analysis Methodology for Successful Development.” International Journal of Production Economics 233: 107992.
- Nguyen, X. T., and Q. K. Luu. 2020. “Factors Affecting Adoption of Industry 4.0 by Small-and Medium-Sized Enterprises: A Case in Ho Chi Minh City, Vietnam. The Journal of Asian Finance.” Economics, and Business 7 (6): 255–264.
- Núñez-Merino, M., J. M. Maqueira-Marín, J. Moyano-Fuentes, and P. J. Martínez-Jurado. 2020. “Information and Digital Technologies of Industry 4.0 and Lean Supply Chain Management: A Systematic Literature Review.” International Journal of Production Research 58 (16): 5034–5061.
- Okazaki, N., Y. Matsuo, and M. Ishizuka. 2005. “Improving Chronological Ordering of Sentences Extracted from Multiple Newspaper Articles.” ACM Transactions on Asian Language Information Processing (TALIP 4 (3): 321–339.
- Oncioiu, I., O. C. Bunget, M. C. Türkes, S. Capusneanu, D. I. Topor, A. S. Tamas, I. S. Rakos, et al. 2019. “The Impact of big Data Analytics on Company Performance in Supply Chain Management.” Sustainability Switzerland 11: 18. doi:https://doi.org/10.3390/su11184864.
- Oztemel, E., and S. Gursev. 2020. “Literature Review of Industry 4.0 and Related Technologies.” Journal of Intelligent Manufacturing 31 (1): 127–182.
- Papagiannidis, S., J. Harris, and D. Morton. 2020. “WHO led the Digital Transformation of Your Company? A Reflection of IT Related Challenges During the Pandemic.” International Journal of Information Management 55: 102166.
- Pappas, I. O., P. Mikalef, M. N. Giannakos, J. Krogstie, and G. Lekakos. 2018. “Big Data and Business Analytics Ecosystems: Paving the way Towards Digital Transformation and Sustainable Societies.” Information Systems and E-Business Management 16 (3): 479–491.
- Pavlidou, I., S. Papagiannidis, and E. Tsui. 2020. “Crowdsourcing: A Systematic Review of the Literature Using Text Mining.” Industrial Management & Data Systems 120 (11): 2041–2065. doi:https://doi.org/10.1108/IMDS-08-2020-0474.
- Pech, M., and J. Vrchota. 2020. “Classification of Small-and Medium-Sized Enterprises Based on the Level of Industry 4.0 Implementation.” Applied Sciences 10 (15): 5150.
- Pessot, E., A. Zangiacomi, C. Battistella, V. Rocchi, A. Sala, and M. Sacco. 2020. “What Matters in Implementing the Factory of the Future.” Journal of Manufacturing Technology Management 32 (3): 795–819. doi:https://doi.org/10.1108/JMTM-05-2019-0169.
- Pfeiffer, S., H. Lee, and M. Held. 2019. “Doing Industry 4.0 – Participatory Design on the Shop Floor in the View of Engineering Employees.” Cuadernos de Relaciones Laborales 37 (2): 293–311. doi:https://doi.org/10.5209/crla.66039.
- Piccarozzi, M., B. Aquilani, and C. Gatti. 2018. “Industry 4.0 in Management Studies: A Systematic Literature Review.” Sustainability (Switzerland) 10 (10): 3821.
- Pollak, A., A. Hilarowicz, M. Walczak, and D. Gąsiorek. 2020. “A Framework of Action for Implementation of Industry 4.0. An Empirically Based Research.” Sustainability 12 (14): 5789.
- Prause, G. 2015. “Sustainable Business Models and Structures for Industry 4.0.” Journal of Security and Sustainability Issues 5 (2): 159–169. doi:https://doi.org/10.9770/jssi.2015.5.2(3).
- Raj, A., G. Dwivedi, A. Sharma, A. B. Lopes de Sousa Jabbour, and S. Rajak. 2020. “Barriers to the Adoption of Industry 4.0 Technologies in the Manufacturing Sector: An Inter-Country Comparative Perspective.” International Journal of Production Economics 224: 107546. doi:https://doi.org/10.1016/j.ijpe.2019.107546.
- Rajput, S., and S. P. Singh. 2019a. “Industry 4.0 − Challenges to Implement Circular Economy.” Benchmarking 28 (5): 1717–1739. doi:https://doi.org/10.1108/BIJ-12-2018-0430.
- Rajput, S., and S. P. Singh. 2019b. “Identifying Industry 4.0 IoT Enablers by Integrated PCA-ISM-DEMATEL Approach.” Management Decision 57 (8): 1784–1817. doi:https://doi.org/10.1108/MD-04-2018-0378.
- Rakyta, M., M. Fusko, J. Herčko, L. Závodská, and N. Zrnić. 2016. “Proactive Approach to Smart Maintenance and Logistics as a Auxiliary and Service Processes in a Company.” Journal of Applied Engineering Science 14 (4): 433–442. doi:https://doi.org/10.5937/jaes14-11664.
- Ramírez-Durán, V. J., I. Berges, and A. Illarramendi. 2021. “Towards the Implementation of Industry 4.0: A Methodology-Based Approach Oriented to the Customer Life Cycle.” Computers in Industry 126: 103403. doi:https://doi.org/10.1016/j.compind.2021.103403.
- Rauch, E., P. Dallasega, and M. Unterhofer. 2019. “Requirements and Barriers for Introducing Smart Manufacturing in Small and Medium-Sized Enterprises.” IEEE Engineering Management Review 47 (3): 87–94. doi:https://doi.org/10.1109/EMR.2019.2931564.
- Robert, M., P. Giuliani, and C. Gurau. 2020. “Implementing Industry 4.0 Real-Time Performance Management Systems: The Case of Schneider Electric.” Production Planning & Control: 1–17. doi:https://doi.org/10.1080/09537287.2020.1810761.
- Rosin, F., P. Forget, S. Lamouri, and R. Pellerin. 2019. “Impacts of Industry 4.0 Technologies on Lean Principles.” International Journal of Production Research 58 (6): 1644–1661. doi:https://doi.org/10.1080/00207543.2019.1672902.
- Saabye, H., T. B. Kristensen, and B. V. Wæhrens. 2020. “Real-Time Data Utilization Barriers to Improving Production Performance: An In-depth Case Study Linking Lean Management and Industry 4.0 from a Learning Organization Perspective.” Sustainability 12 (21): 8757. doi:https://doi.org/10.3390/su12218757.
- Sader, S., I. Husti, and M. Daróczi. 2019. “Industry 4.0 as a Key Enabler Toward Successful Implementation of Total Quality Management Practices.” Periodica Polytechnica Social and Management Sciences 27 (2): 131–140. doi:https://doi.org/10.3311/PPso.12675.
- Saengchai, S., and K. Jermsittiparsert. 2019. “Coping Strategy to Counter the Challenges Towards Implementation of Industry 4.0 in Thailand: Role of Supply Chain Agility and Resilience.” International Journal of Supply Chain Management 8 (5): 733–744. https://www.scopus.com/inward/record.uri?eid=2-s2.0-85074509110&partnerID=40&md5=e4cef65cad333a93a0832d951e56cf2d.
- Salimon, M. G., H. H. Abdullah, A. H. Gorondutse, S. S. Bin Abdullah, A. Tafida, Y. I. Ango, and A. B. Abdul-Qadir. 2019. “Empirical Implementation of Industry 4.0 Model among Malaysian and Nigerian SMEs.” International Journal of Supply Chain Management 8 (3): 622–632. https://www.scopus.com/inward/record.uri?eid=2-s2.0-85068325051&partnerID=40&md5=4286a5f38fa4b490310f636f485fa1ed.
- Sanders, A., C. Elangeswaran, and J. Wulfsberg. 2016. “Industry 4.0 Implies Lean Manufacturing: Research Activities in Industry 4.0 Function as Enablers for Lean Manufacturing.” Journal of Industrial Engineering and Management 9 (3): 811–833. doi:https://doi.org/10.3926/jiem.1940.
- Sanghavi, D., S. Parikh, and S. A. Raj. 2019. “Industry 4.0: Tools and Implementation.” Management and Production Engineering Review 10 (3): 3–13. doi:https://doi.org/10.24425/mper.2019.129593.
- Saniuk, S., and A. Saniuk. 2018. “Challenges of Industry 4.0 for Production Enterprises Functioning Within Cyber Industry Networks.” Management Systems in Production Engineering 26 (4): 212–216. doi:https://doi.org/10.1515/mspe-2018-0034.
- Schleich, B., N. Anwer, L. Mathieu, and S. Wartzack. 2017. “Shaping the Digital Twin for Design and Production Engineering.” CIRP Annals 66 (1): 141–144.
- Schneider, P. 2018. “Managerial Challenges of Industry 4.0: An Empirically Backed Research Agenda for a Nascent Field.” Review of Managerial Science 12 (3): 803–848. doi:https://doi.org/10.1007/s11846-018-0283-2.
- Schuh, G., R. Anderl, J. Gausemeier, M. ten Hompel, and W. Wahlster. 2017. Industrie 4.0 Maturity Index. Managing the Digital Transformation of Companies. Munich: Herbert Utz.
- Seifert, R., and R. Markoff. 2021. “Industry 4.0 and COVID-19: Why the Pandemic Might Have Provided a Clearing in the Woods.” IMD. https://www.imd.org/research-knowledge/articles/the-pandemic-might-have-provided-a-clearing-in-the-woods-for-industry-4/.
- Shet, S. V., and V. Pereira. 2021. “Proposed Managerial Competencies for Industry 4.0 – Implications for Social Sustainability.” Technological Forecasting and Social Change 173: 121080. doi:https://doi.org/10.1016/j.techfore.2021.121080.
- Silvestri, L., A. Forcina, V. Introna, A. Santolamazza, and V. Cesarotti. 2020. “Maintenance Transformation Through Industry 4.0 Technologies: A Systematic Literature Review.” Computers in Industry 123: 103335.
- Simetinger, F., and Z. Zhang. 2020. “Deriving Secondary Traits of Industry 4.0: A Comparative Analysis of Significant Maturity Models.” Systems Research and Behavioral Science 37 (4): 663–678.
- Singh, R., and N. Bhanot. 2020. “An Integrated DEMATEL-MMDE-ISM Based Approach for Analysing the Barriers of IoT Implementation in the Manufacturing Industry.” International Journal of Production Research 58 (8): 2454–2476. doi:https://doi.org/10.1080/00207543.2019.1675915.
- Singhal, N. 2020. “An Empirical Investigation of Industry 4.0 Preparedness in India.” Vision, 0972262920950066.
- Sjödin, D. R., V. Parida, M. Leksell, and A. Petrovic. 2018. “Smart Factory Implementation and Process Innovation: A Preliminary Maturity Model for Leveraging Digitalization in Manufacturing Moving to Smart Factories Presents Specific Challenges That Can be Addressed Through a Structured Approach Focused on People, Processes, and Technologies.” Research-Technology Management 61 (5): 22–31.
- Škrinjarić, B., and P. Domadenik. 2019. “Examining the Role of key Competences in Firm Performance.” International Journal of Manpower. doi:https://doi.org/10.1108/IJM-10-2018-0349.
- Ślusarczyk, B. 2018. “Industry 4.0: Are we Ready?” Polish Journal of Management Studies 17.
- Ślusarczyk, B., and A. U. Haque. 2019. “Public Services for Business Environment: Challenges for Implementing Industry 4.0 in Polish and Canadian Logistic Enterprises.” Administratie si Management Public 2019 (33): 57–76. doi:https://doi.org/10.24818/amp/2019.33-04.
- Ślusarczyk, B., M. Haseeb, and H. I. Hussain. 2019. “Fourth Industrial Revolution: A way Forward to Attain Better Performance in the Textile Industry.” Engineering Management in Production and Services 11 (2): 52–69.
- Sony, M. 2020. “Design of Cyber Physical System Architecture for Industry 4.0 Through Lean six Sigma: Conceptual Foundations and Research Issues.” Production & Manufacturing Research 8 (1): 158–181.
- Sony, M., and S. S. Naik. 2019. “Ten Lessons for Managers While Implementing Industry 4.0.” IEEE Engineering Management Review 47 (2): 45–52. doi:https://doi.org/10.1109/EMR.2019.2913930.
- Sony, M., and S. Naik. 2020. “Industry 4.0 Integration with Socio-Technical Systems Theory: A Systematic Review and Proposed Theoretical Model.” Technology in Society 61: 101248.
- Sousa, R., and C. A. Voss. 2008. “Contingency Research in Operations Management Practices.” Journal of Operations Management 26 (6): 697–713.
- Stentoft, J., and C. Rajkumar. 2020. “The Relevance of Industry 4.0 and its Relationship with Moving Manufacturing out, Back and Staying at Home.” International Journal of Production Research, doi:https://doi.org/10.1080/00207543.2019.1660823.
- UBS, Stiehler, A., Gantori, S., & Scheiner, A. 2020. “Industry 4.0 and the IIoT: After COVID-19.” https://www.ubs.com/global/en/wealth-management/chief-investment-office/life-goals/executives-and-entrepreneurs/2020/industry-4-point-zero-after-covid-19/_jcr_content/mainpar/toplevelgrid_2005646792/col2/teaser/linklist/actionbutton.1629036945.file/bGluay9wYXRoPS9jb250ZW50L2RhbS9hc3NldHMvd20vZ2xvYmFsL2Npby9kb2MvaW5kdXN0cnktNC1wb2ludC16ZXJvLWFuZC10aGUtaWlvdC1hZnRlci1jb3ZpZC0xOS0xLnBkZg==/industry-4-point-zero-and-the-iiot-after-covid-19-1.pdf
- Stock, T., and G. Seliger. 2016. “Opportunities of Sustainable Manufacturing in Industry 4.0.” Procedia Cirp 40: 536–541.
- Stornelli, A., S. Ozcan, and C. Simms. 2021. “Advanced Manufacturing Technology Adoption and Innovation: A Systematic Literature Review on Barriers, Enablers, and Innovation Types.” Research Policy 50 (6): 104229.
- Suddaby, R. 2010. “Editor's Comments: Construct Clarity in Theories of Management and Organization.” Academy of Management Review 35 (3): 346–357. doi:https://doi.org/10.5465/amr.35.3.zok346.
- Sundarakani, B., R. Kamran, P. Maheshwari, and V. Jain. 2019. “Designing a Hybrid Cloud for a Supply Chain Network of Industry 4.0: A Theoretical Framework.” Benchmarking: An International Journal 28 (5): 1524–1542. doi:https://doi.org/10.1108/BIJ-04-2018-0109.
- Szabo, R. Zs., I. Vuksanović Herceg, R. Hanák, L. Hortovanyi, A. Romanová, M. Mocan, and D. Djuričin. 2020. “Industry 4.0 Implementation in B2B Companies: Cross-Country Empirical Evidence on Digital Transformation in the CEE Region.” Sustainability 12 (22): 9538. doi:https://doi.org/10.3390/su12229538.
- Szász, L., K. Demeter, B. G. Rácz, and D. Losonci. 2020. “Industry 4.0: A Review and Analysis of Contingency and Performance Effects.” Journal of Manufacturing Technology Management.
- Tarifa-Fernández, J., A. M. Sánchez-Pérez, and S. Cruz-Rambaud. 2019. “Internet of Things and Their Coming Perspectives: A Real Options Approach.” Sustainability (Switzerland) 11: 11. doi:https://doi.org/10.3390/su11113178.
- Teece, D. J. 2018. “Business Models and Dynamic Capabilities.” Long Range Planning 51 (1): 40–49.
- Thite, M. 2000. “Leadership Styles in Information Technology Projects.” International Journal of Project Management 18 (4): 235–241.
- Thompson, E. P. 1967. “Time, Work-Discipline, and Industrial Capitalism.” Past & Present 38 (1): 56–97. doi:https://doi.org/10.1093/past/38.1.56.
- Tortorella, G. L., and D. Fettermann. 2018. “Implementation of Industry 4.0 and Lean Production in Brazilian Manufacturing Companies.” International Journal of Production Research 56 (8): 2975–2987. doi:https://doi.org/10.1080/00207543.2017.1391420.
- Tortorella, G. L., M. Rossini, F. Costa, A. Portioli Staudacher, and R. Sawhney. 2019. “A Comparison on Industry 4.0 and Lean Production Between Manufacturers from Emerging and Developed Economies.” Total Quality Management and Business Excellence, doi:https://doi.org/10.1080/14783363.2019.1696184.
- Tranfield, D., D. Denyer, and P. Smart. 2003. “Towards a Methodology for Developing Evidence-Informed Management Knowledge by Means of Systematic Review.” British Journal of Management 14 (3): 207–222.
- Turner, C., M. Moreno, L. Mondini, K. Salonitis, F. Charnley, A. Tiwari, and W. Hutabarat. 2019. “Sustainable Production in a Circular Economy: A Business Model for re-Distributed Manufacturing.” Sustainability (Switzerland 11: 16. doi:https://doi.org/10.3390/su11164291.
- Urban, W., K. Łukaszewicz, and E. Krawczyk-Dembicka. 2020. “Application of Industry 4.0 to the Product Development Process in Project-Type Production.” Energies 13 (21): 5553. doi:https://doi.org/10.3390/en13215553.
- Veile, J. W., D. Kiel, J. M. Müller, and K. I. Voigt. 2019. “Lessons Learned from Industry 4.0 Implementation in the German Manufacturing Industry.” Journal of Manufacturing Technology Management, doi:https://doi.org/10.1108/JMTM-08-2018-0270.
- Vrchota, J., P. Řehoř, M. Maříková, and M. Pech. 2021. “Critical Success Factors of the Project Management in Relation to Industry 4.0 for Sustainability of Projects.” Sustainability 13 (1): 281. doi:https://doi.org/10.3390/su13010281.
- Wagire, A. A., R. Joshi, A. P. S. Rathore, and R. Jain. 2021. “Development of Maturity Model for Assessing the Implementation of Industry 4.0: Learning from Theory and Practice.” Production Planning & Control 32 (8): 603–622. doi:https://doi.org/10.1080/09537287.2020.1744763.
- Wagire, A. A., A. Rathore, and R. Jain. 2019. “Analysis and Synthesis of Industry 4.0 Research Landscape: Using Latent Semantic Analysis Approach.” Journal of Manufacturing Technology Management 31 (1): 31–51. doi:https://doi.org/10.1108/JMTM-10-2018-0349.
- Wang, X. V., and L. Wang. 2018. “Digital Twin-Based WEEE Recycling, Recovery and Remanufacturing in the Background of Industry 4.0.” International Journal of Production Research 57 (12): 3892–3902. doi:https://doi.org/10.1080/00207543.2018.1497819.
- Wessel, L., A. Baiyere, R. Ologeanu-Taddei, J. Cha, and T. Blegind Jensen. 2021. “Unpacking the Difference Between Digital Transformation and IT-Enabled Organizational Transformation.” Journal of the Association for Information Systems 22 (1): 6.
- Wilkesmann, M., and U. Wilkesmann. 2018. “Industry 4.0–Organizing Routines or Innovations?” VINE Journal of Information and Knowledge Management Systems 48 (2): 238–254.
- Woodward, J. 1958. Management and Technology. London: H.M.S.O.
- Xu, L. D., E. L. Xu, and L. Li. 2018. “Industry 4.0: State of the art and Future Trends.” International Journal of Production Research 56 (8): 2941–2962.
- Yaniv, E. 2011. “Construct Clarity in Theories of Management and Organization.” Academy of Management Review 36 (3): 590–592.
- Yazdi, P. G., A. Azizi, and M. Hashemipour. 2018. “An Empirical Investigation of the Relationship Between Overall Equipment Efficiency (OEE) and Manufacturing Sustainability in Industry 4.0 with Time Study Approach.” Sustainability (Switzerland) 10: 9. doi:https://doi.org/10.3390/su10093031.
- Yazdi, P. G., A. Azizi, and M. Hashemipour. 2019. “A Hybrid Methodology for Validation of Optimization Solutions Effects on Manufacturing Sustainability with Time Study and Simulation Approach for SMEs.” Sustainability (Switzerland) 11: 5. doi:https://doi.org/10.3390/su11051454.
- Yu, F., and T. Schweisfurth. 2020. “Industry 4.0 Technology Implementation in SMEs–A Survey in the Danish-German Border Region.” International Journal of Innovation Studies 4 (3): 76–84.
- Zangiacomi, A., E. Pessot, R. Fornasiero, M. Bertetti, and M. Sacco. 2020. “Moving Towards Digitalization: A Multiple Case Study in Manufacturing.” Production Planning and Control 31 (2-3): 143–157. doi:https://doi.org/10.1080/09537287.2019.1631468.
- Zhang, Q., J. L. Jin, and D. Yang. 2020. “How to Enhance Supplier Performance in China: Interplay of Contracts, Relational Governance and Legal Development.” International Journal of Operations & Production Management 40 (6): 777–808. doi:https://doi.org/10.1108/IJOPM-02-2020-0093.
- Zheng, T., M. Ardolino, A. Bacchetti, and M. Perona. 2020. “The Applications of Industry 4.0 Technologies in Manufacturing Context: A Systematic Literature Review.” International Journal of Production Research 59 (6): 1–33. doi:https://doi.org/10.1080/00207543.2020.1824085.
- Zonta, T., C. A. da Costa, R. da Rosa Righi, M. J. de Lima, E. S. da Trindade, and G. P. Li. 2020. “Predictive Maintenance in the Industry 4.0: A Systematic Literature Review.” Computers & Industrial Engineering 150: 106889. doi:https://doi.org/10.1016/j.cie.2020.106889.