Abstract
Actionable Cognitive Twins are the next generation Digital Twins enhanced with cognitive capabilities through a knowledge graph and artificial intelligence models that provide insights and decision-making options to the users. The knowledge graph describes the domain-specific knowledge regarding entities and interrelationships related to a manufacturing setting. It also contains information on possible decision-making options that can assist decision-makers, such as planners or logisticians. This paper proposes a knowledge graph modelling approach to construct actionable cognitive twins for capturing specific knowledge related to production planning and demand forecasting in a manufacturing plant. The knowledge graph provides semantic descriptions and contextualisation of the production lines and processes, including data identification and simulation or artificial intelligence algorithms and forecasts used to support them. Such semantics provide ground for inferencing, relating different knowledge types: creative, deductive, definitional, and inductive. To develop the knowledge graph models for describing the use case thoroughly, systems thinking approach is proposed to design and verify the ontology, develop a knowledge graph and build an actionable cognitive twin. Finally, we evaluate our approach in two use cases developed for a European original equipment manufacturer related to the automotive industry as part of the European Horizon 2020 project FACTLOG.
Data availability statement
The authors confirm that the data supporting the findings of this study are available within the article.
Disclosure statement
No potential conflict of interest was reported by the author(s).
Notes
1 The ontology was published and is available online (Rožanec and Lu Citation2021).
2 https://neo4j.com/
Additional information
Funding
Notes on contributors
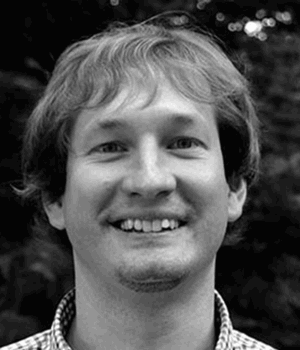
Jože M. Rožanec
Jože Martin Rožanec is a Ph.D. candidate in Information and Communication Technologies at Jožef Stefan International Postgraduate School. He is a Researcher at the Artificial Intelligence Laboratory (Jožef Stefan Institute), a machine learning engineer at Qlector d.o.o. (developing intelligent solutions for smart factories). He collaborates with the American Slovenian Education Foundation, where he leads multiple activities for Fellows and Alumni. Over more than ten years he worked for several companies (e.g. Mercado Libre, Navent, Globant) in software engineering and machine learning-related roles. His research interests include machine learning methods for recommendations, fraud detection, demand forecasting, active learning, and explainable artificial intelligence (XAI).
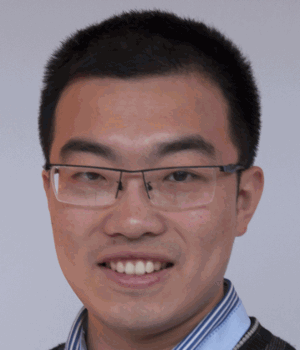
Jinzhi Lu
Jinzhi Lu, CSEP, is a research scientist at EPFL. He got his ph.d degree at KTH Royal Institute of Technology, Mechatronics Division in 2019. His research interest is MBSE tool-chain design and MBSE enterprise transitioning. He is senior member of China Council on Systems Engineering (CCOSE), China Council on Systems Engineering.
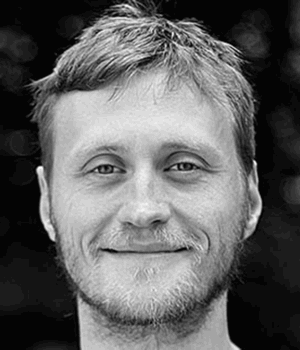
Jan Rupnik
Jan Rupnik (PhD) [M] is employed as a lead research scientist at QLECTOR d.o.o. and part time as a researcher at Artificial Intelligence Laboratory, Jožef Stefan Institute. In QLECTOR he is responsible for development of machine learning models that address uncertainty of several parts in the logistic chain: production (machine performance, organisational and technical stoppages, resource availability), supply (supplier lead times) and demand (customer behaviour variability, global market demand variability).
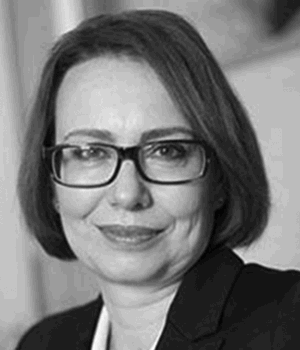
Maja Škrjanc
Maja Škrjanc has over 20 years of experience in the AI domain, in which she intertwines industry and research in various business domains such as finance, telco, insurance, retail, manufacturing, energy. Among Industry related experiences, she established and lead the Data Science unit in Telekom Slovenija. As Head of analytics at SAS Institute Adriatic region, she was responsible for AI solutions for large companies. In 2000 she joined AI Lab at Jožef Stefan Institute, as a researcher and was responsible for more than 20 national and EU projects. Her research interests include text and web mining, semantic technologies, social media analysis and reasoning.
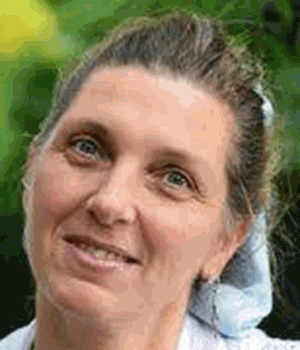
Dunja Mladenić
Dunja Mladenić 〈http://ailab.ijs.si/dunja_mladenic/〉 works as a researcher and a project leader at Jožef Stefan Institute, Slovenia, leading Artificial Intelligence Department and teaching at Jožef Stefan International Postgraduate School, University of Ljubljana and the University of Zagreb. She has extensive research experience in the study and development of Machine Learning, Big Data/Text Mining, the Internet of Things, Data Science, Semantic Technology techniques, and their application to real-world problems. She has published papers in refereed journals and conferences, co-edited several books, served on program committees of international conferences, and organised international events. She serves as a project evaluator of project proposals for the European Commission and USA National Science Foundation. From 2013–2017 she served on the Institute's Scientific Council as a vice president (2015–2017). She serves on the Executive Board of Slovenian Artificial Intelligence Society SLAIS (as a president of SLAIS (2010–2014)) and on the Advisory board of ACM Slovenija.
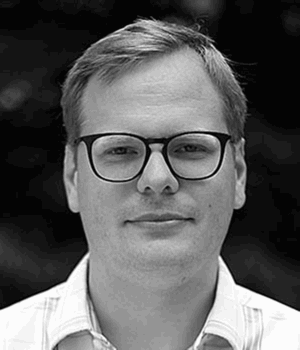
Blaž Fortuna
Blaž Fortuna is the managing director at Qlector, a startup developing artificial intelligence-based solutions for the manufacturing, logistics, finance, and senior researcher at Jožef Stefan Institute. He is the initiator and primary contributor to QMiner, the open-source data analytics platform for processing large-scale real-time streams containing structured and unstructured data and co-contributor to Event Registry. He did his Ph.D. at Jožef Stefan Institute. He was a research consultant for Bloomberg L.P., a Marie Curie Fellow at Stanford University, a postdoc at IBCN (Ghent University, Belgium), and the project manager for the XLike project.
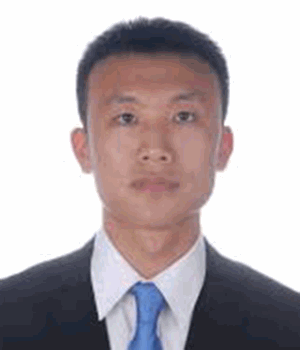
Xiaochen Zheng
Xiaochen Zheng, Ph.D, received his doctoral degree from Universidad Politécnica de Madrid. Before that he studied in Shandong University in Mechanical Engineering and obtained his bachelor and master degree. He is now working at École Polytechnique Fédérale de Lausanne as a postdoctoral scientist. His research interests include Internet of Things, Machine learning, Wearable technology, Distributed ledger technology and their applications in industry and healthcare etc.
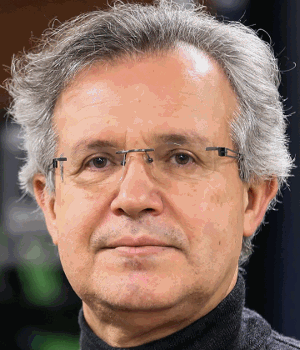
Dimitris Kiritsis
Dimitris Kiritsis is Faculty Member at the Institute of Mechanical Engineering of the School of Engineering of EPFL, Switzerland, where he is leading a research group on ICT for Sustainable Manufacturing. He serves also as Director of the doctoral Program of EPFL on Robotics, Control and Intelligent Systems (EDRS). His research interests are Closed Loop Life cycle Management, IoT, Semantic Technologies and Data Analytics for Engineering Applications.