Abstract
Digital transformation driven by artificial intelligence (AI) allows Industry 4.0 and the internet of things (IIoT) to make significant advancements in automating, controlling, and improving the quality of numerous manufacturing processes. Three-dimensional (3D) surface topography of manufactured products holds important information about the quality of manufacturing processes. Surface topography consists of unique properties, which makes the current monitoring approaches ineffective in identifying local and spatial surface faults. In this paper, we develop a generalised spatially weighted autocorrelation approach based on AI for monitoring changes in products based on their 3D topographic surfaces. We propose two effective algorithms to identify and assign spatial weights to the topographic regions with suspicious characteristics. The normal surface hard thresholding algorithm initially enhances the representation of surface characteristics through binarization, followed by the normal surface connected-component labelling algorithm, which utilises the obtained binary results to identify and assign spatial weights to the suspicious regions. We then introduce a generalised spatially weighted Moran index, which exploits the assigned weights to locally characterise and monitor changes in the spatial autocorrelation structure of identified regions. After an anomaly surface is detected, we extract different fault diagnostic information. The proposed approach proves its robustness and efficiency in characterising, monitoring, and diagnosing different patterns of faults in 3D topographic surfaces.
Acknowledgments
The authors acknowledge the valuable comments and suggestions made by the Associate Editor and three anonymous reviewers.
Disclosure statement
No potential conflict of interest was reported by the author(s).
Data availability statement
The data that support the findings of this study are available from the corresponding author, Myong K. Jeong, upon reasonable request.
Additional information
Notes on contributors
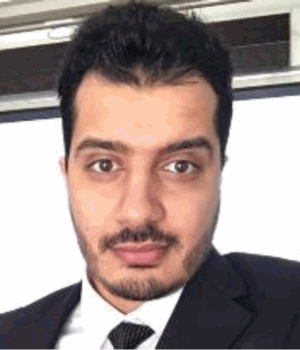
Mejdal A. Alqahtani
Dr. Mejdal A. Alqahtani is an Assistant Professor at the Department of Industrial Engineering, King Saud University, Saudi Arabia. He received his B.S. degree in Industrial Engineering from King Saud University, Saudi Arabia, in 2010, a M.S. degree in Industrial and Systems Engineering, Rutgers University, in 2014, and M.S. degree in Statistics from Rutgers University in 2015. He then received a Ph.D. in Industrial and Systems Engineering, Rutgers University, in 2020. His research interests include statistical process modelling, quality monitoring, artificial intelligence, data science including data mining, machine learning, deep learning and data collection and analysis.
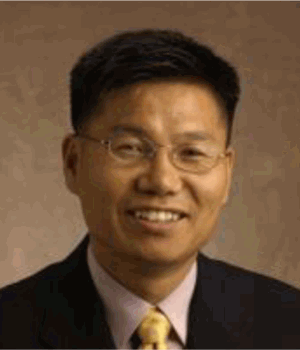
Myong K. Jeong
Dr. Myong K. (MK) Jeong is a Professor in the Department of Industrial and Systems Engineering and the Rutgers Centre for Operations Research at Rutgers University. He has focused on developing data mining techniques, process monitoring and control procedures, and optimisation techniques for machine learning. He has published over 60 journal papers including Technometrics, IEEE Transaction on Semiconductor Manufacturing, IEEE Transactions on Systems, Man, Cybernetics, Pattern Recognition Letters, and IIE Transaction on Quality and Reliability. He is an associate editor of the IEEE Transactions on Automation Science and Engineering, International Journal of Quality, Statistics and Reliability, and Advisory Board Member of International Journal of Advanced Manufacturing Technology. He is a senior member of IEEE.

Elsayed A. Elsayed
E. A. Elsayed is Distinguished Professor in the Department of Industrial and Systems Engineering, Rutgers University. He is also the Director of the NSF/ Industry/ University Co-operative Research Centre for Quality and Reliability Engineering. His research interests are in the areas of quality and reliability engineering and Production Planning and Control. He served as the Editor-in-Chief of the IIE Transactions and the Editor of the IIE Transactions on Quality and Reliability Engineering. He is Editor-in-Chief of Quality Technology and Quality Management. Dr. Elsayed is also the Editor of the International Journal of Reliability, Quality and Safety Engineering. He serves on the editorial boards of eight journals in different capacities.