Abstract
In the in-situ quality detection of yarn production, image deblurring plays a critical role in the vision-based detection systems to restore a sharp image and provide more accurate input for inspection. However, image deblurring is still challenging since the current methods are mainly based on the pre-defined blur degree. In dynamic yarn production, the relationship between the defocus blur degrees and the poses of the yarn body is highly associated, which can be excavated to prior knowledge in image deblurring to achieve more effective restoration. Thus, a knowledge augmented deep learning model is proposed to adaptively deblur yarn images with variable defocus blur degrees. A pose classification module designed by prior knowledge is embedded into the deep neural network, which classifies the yarn poses and feeds them into multi-scale deblurring channels. In each channel, we incorporate the image gradient prior into the specially designed loss function to attract the attention of the deblurring network on the edge details of the yarn. The experimental results from actual spinning processes demonstrate that the proposed method performs a better effect not only in the variable-scale deblurring of the global image but also in the restoration of the edge details.
Disclosure statement
No potential conflict of interest was reported by the author(s).
Data availability statement
The data that support the findings of this study are available on request from the corresponding author, J. Zhang. The data are not publicly available due to their containing information that could compromise the privacy of cooperative enterprise.
Additional information
Funding
Notes on contributors
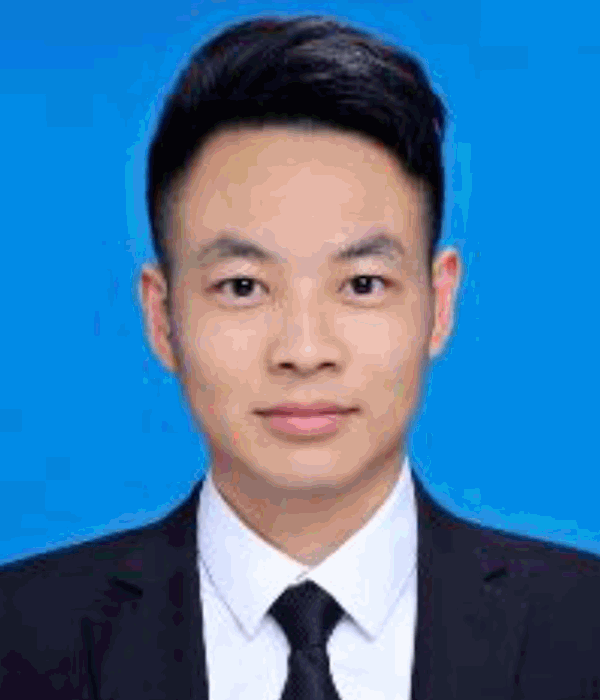
Chuqiao Xu
Chuqiao Xu received the M.S. degree in mechanical engineering from Xinjiang University, Urumchi, China, in 2017. He is currently working toward the Ph.D. degree in big data driven product quality inspection for industrial engineering at School of Mechanical Engineering, Shanghai Jiao Tong University, Shanghai, China. His current research focuses on big data analytics and computer vision solutions for yarn spinning systems.
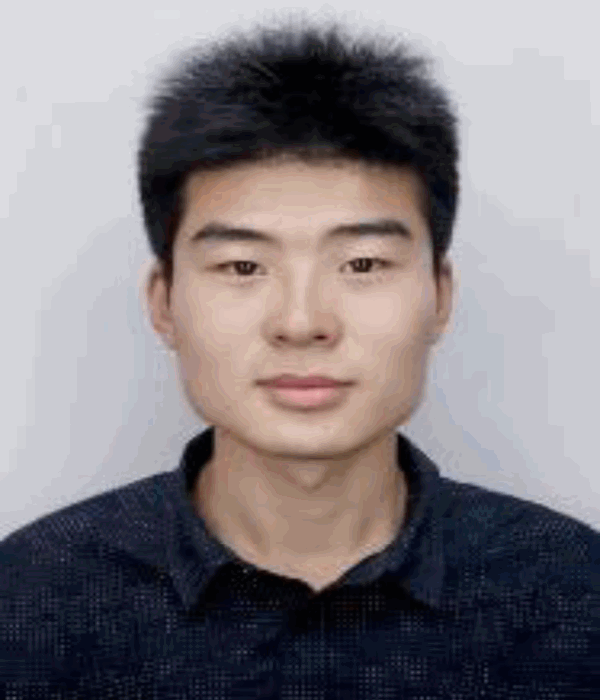
Junliang Wang
Junliang Wang received the B.S. degree in scheduling of manufacturing systems from Wuhan University of Technology, Wuhan, China, in 2009 and the Ph.D. degree in big data driven operation for industrial engineering at School of Mechanical Engineering, Shanghai Jiao Tong University, Shanghai, China, in 2018. Since 2018, he is a Research Assistant with College of Mechanical Engineering, Donghua University, Shanghai, China. He is the author of 2 books, more than 30 articles. His current research focuses on big data analytics and operation in complex manufacturing systems.
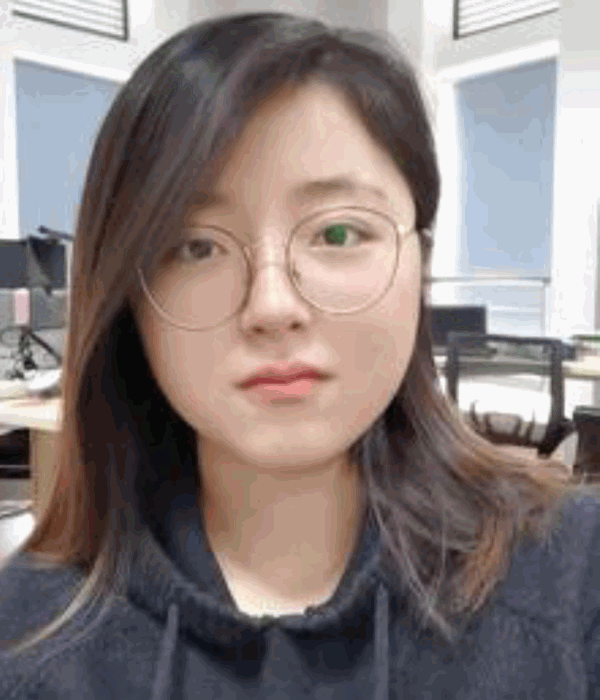
Jing Tao
Jing Tao received the Bachelor's degree in mechanical engineering from Donghua University, Shanghai, China,in 2020. She is currently working towards the M.S. degree in mechanical engineering at Donghua University. Her current research focuses on computer vision.
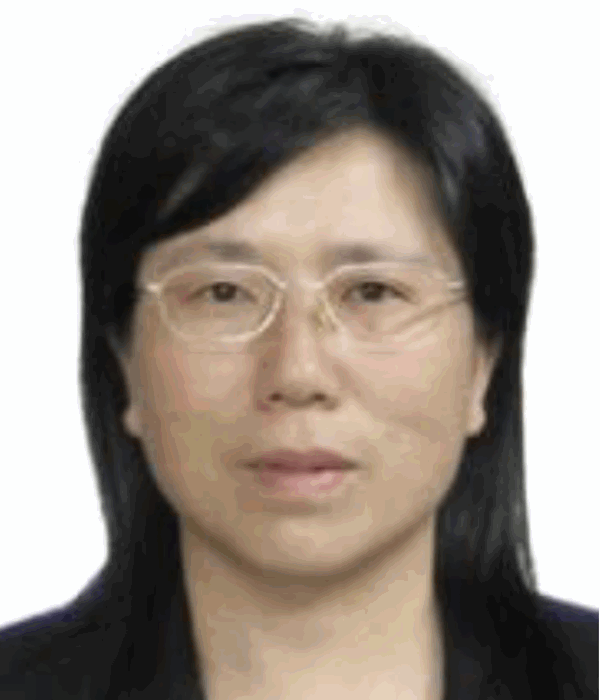
Jie Zhang
Jie Zhang received the Ph.D. degree in scheduling of manufacturing systems from Nanjing University of Aeronautics and Astronautics, Nanjing, China, in 1997. She is currently the Dean of College of Mechanical Engineering, Donghua University, Shanghai, China. Before joining Donghua University, she was a Professor with the Institute of Intelligent Manufacturing and Information Engineering, Shanghai Jiao Tong University, Shanghai, China. She is the author of 6 books, more than 100 articles. Her research interests include industrial big data analysis, intelligent production scheduling, production control in intelligent manufacturing system, and intelligent quality analysis.
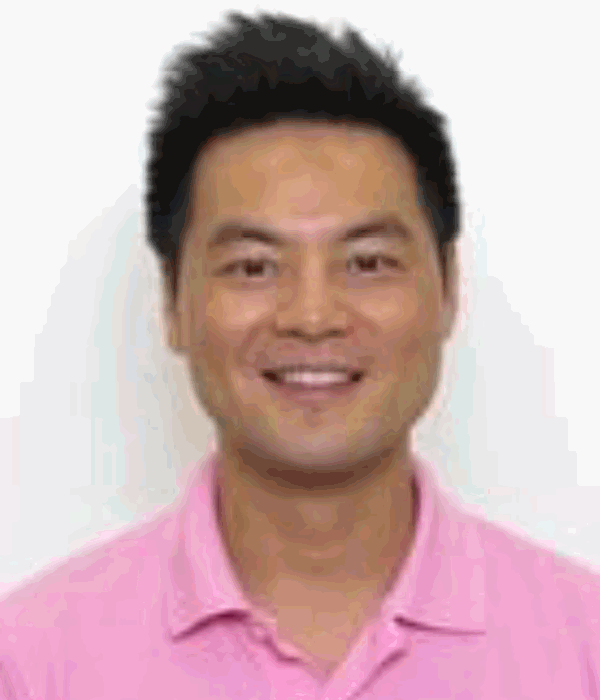
Ray Y. Zhong
Ray Y. Zhong received the B.S. degree in mathematics and computer science and technology from Gannan Normal University, Ganzhou, China, in 2004, the M.S. degree in signal and information processing from the Guangdong University of Technology, Guangzhou, China, in 2009, and the Ph.D. degree in industrial and manufacturing systems engineering from the University of Hong Kong, Hong Kong, in 2013. He is now an Assistant Professor with the Department of Industrial and Manufacturing Systems Engineering, University of Hong Kong. His current research interests include big data in manufacturing and service, advanced planning and scheduling, and RFID in Internet of manufacturing things. Dr. Zhong is a member of CIRP RA, Logistics and Supply Chain Management Hong Kong, Institution of Engineering and Technology, American Society of Mechanical Engineers, and IEEE. Ray was awarded the 2018 IJPR Best Paper with the title of ‘Big Data Analytics for Physical Internet-based intelligent manufacturing shop floor,’ Best Conference Paper Award with the title of ‘Analysis of RFID Datasets for Smart Manufacturing Shop Floors’ in 15th IEEE International Conference on Networking, Sensing and Control, New Zealand Chinese Youth Scientist Award (NZCYSA) 2017 and the Young Author Prize in the 15th IFAC/IEEE/IFIP/IFORS Symposium on Information Control Problems in Manufacturing.