Abstract
With the rapid advances of Industry 4.0 technologies, developing rigorous, model-based algorithms for production system performance metrics calculation, control, and optimization has become a critical task to inject intelligence into the smart manufacturing practice, thus facilitating automated decision-making on the factory floor. This paper is intended to contribute to this area. Specifically, we consider serial production lines with finite buffers and machines following the Bernoulli reliability model. In addition, we assume that one can dynamically control the allocation of a shared workforce via real-time production bottleneck identification and mitigation. We first derive formulas to calculate the performance metrics of two-machine systems. Then, we extend the results to multi-machine cases by developing an aggregation-based analytical algorithm, whose accuracy is verified using numerical experiments. Based on this performance evaluation method, we study the optimization of the control policy. In particular, a space reduction technique is applied that decomposes the system into several sub three-machine lines and search algorithms are proposed to identify a good-quality control policy in the reduced policy space. Numerical experiments are used to demonstrate the efficacy of the optimized policy. An illustrative example is given to provide insights into the structure and operation of an effective control policy.
Disclosure statement
No potential conflict of interest was reported by the authors.
Data availability statement
The authors confirm that the data supporting the findings of this study are available within the article.
Additional information
Notes on contributors
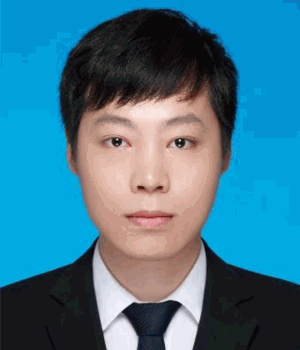
Jiachen Tu
Jiachen Tu received the B.E. degree in Mechanical Engineering and Automation and M.E. degree in Mechanical and Electronic Engineering from Nanjing University of Aeronautics and Astronautics, Nanjing, China, in 2015 and 2018, respectively. He is now working towards the Ph.D. degree at the Department of Electrical and Computer Engineering, University of Connecticut, Storrs, CT, USA. His research interests include modelling, analysis, improvement and visualisation of smart manufacturing systems, the control and optimisation of the production system, and the application of machine learning.
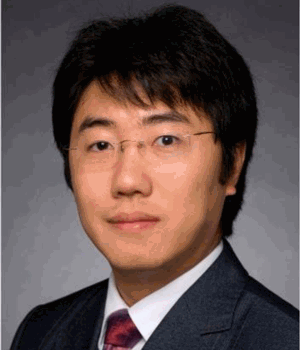
Liang Zhang
Liang Zhang received the B.E. and M.E. degrees from the Center for Intelligent and Networked Systems, Department of Automation, Tsinghua University, Beijing, China, in 2002 and 2004, respectively, and the Ph.D. degree in Electrical Engineering-Systems from the University of Michigan, Ann Arbor, MI, USA, in 2009. He was with the Department of Industrial and Manufacturing Engineering, University of Wisconsin-Milwaukee from 2009 to 2013. He is currently an Associate Professor in the Department of Electrical and Computer Engineering and the Director of the Southern New England Industrial Assessment Center at the University of Connecticut, Storrs, CT, USA. His research interests include modelling, analysis, improvement, design, control, and smart operations of manufacturing systems. He is a co-founder of Smart Production Systems LLC.