Abstract
COVID-19 has been a severe crisis for global health, which caused significant loss of life and property. One of the most effective ways to prevent the spread of the virus during an epidemic is to provide nucleic-acid tests for the population. Management of testing resources is both critical and challenging because outbreaks are irregular and resources are scarce. In this study, we develop a decision support tool for city governments by districting testing facilities and determining their capacities. Considering the stochastic testing demand during a disease outbreak, a set-partitioning model embedded with a two-stage distributionally robust optimisation is formulated. Tractable reformulations are derived to solve the problems efficiently and a conservative approximation method is introduced to achieve acceptable accuracy while reducing the computational burden. Compared with different benchmark models, the numerical analyses demonstrate the effectiveness of the proposed territory design, which realises a robust testing infrastructure network and saves the cost while pursuing capability.
Disclosure statement
No potential conflict of interest was reported by the author(s).
8. Data availability statement
The data that support the findings of this study are available from the corresponding author, Xiaolei Xie, upon reasonable request.
Additional information
Funding
Notes on contributors
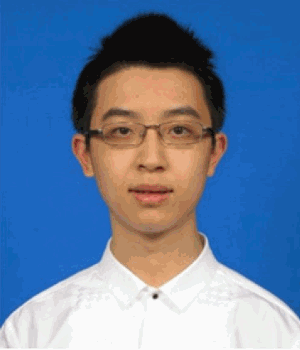
Zhenghao Fan
Zhenghao Fan received the B.S. degree in industrial engineering from Tsinghua University, Beijing, China, in 2017, where he is currently pursuing the Ph.D. degree with the Department of Industrial Engineering. His current research interests include stochastic modelling, robust optimisation, and optimal design in healthcare systems.
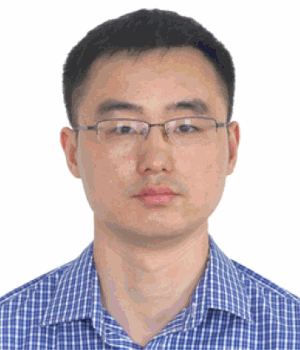
Xiaolei Xie
Xiaolei Xie received the B.S. degree in mechanical engineering from the Beijing University of Aeronautics and Astronautics, Beijing, China, in 2008, the M.S.E. degree in industrial and operations engineering from the University of Michigan, Ann Arbor, MI, USA, in 2009, and the Ph.D. degree from the Department of Industrial and Systems Engineering, University of Wisconsin, Madison, WI, USA, in 2014.
He is an Associate Professor with the Department of Industrial Engineering, Tsinghua University, Beijing. His research interests are in stochastic modelling, optimisation, and advanced data analytics in healthcare systems.
Dr. Xie is a Senior Member of the IEEE Robotics and Automation Society (RAS), the Institute for Operations Research and the Management Sciences (INFORMS), and the Institute of Industrial and Systems Engineers (IISE).