ABSTRACT
A newly emerging mass-individualisation concept has attracted increasing attention in recent years. However, this concept increases the complexity of manufacturing systems within organisations. In such systems, one of the main challenges is the sequencing problem, especially in dynamic environments where unpredictable events demand new constraints. In this context, the ability to use real-time data to make efficient, quick decisions has become one of the main priorities of managers. In this paper, based on a real-world case from Fiat Powertrain Technologies, we define a dynamic mixed-model assembly line sequencing problem with walking workers. In this context, each worker is assigned to a product for all assembly operations and moves from one station to another. A mathematical model is proposed to minimise production time. Since the problem is NP-hard, a hyper-heuristic is also developed to solve the problem. Moreover, a simulation-optimisation model is developed using FlexSim software to solve a real-world problem in a dynamic environment. Comparison of the results illustrates the effectiveness of using the simulation approach to dynamically solve such problems, especially in real-world cases. Finally, a thorough description of managerial insights is provided to indicate the applicability of the proposed approach.
Disclosure statement
No potential conflict of interest was reported by the author(s).
Data availability statement
The authors confirm that the data supporting the findings of this study are available within the article [and/or] its supplementary materials.
Additional information
Notes on contributors
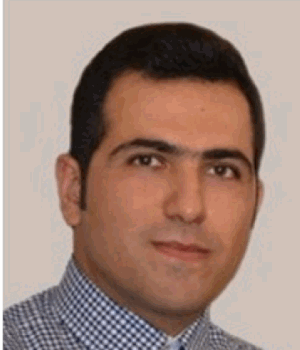
Mojtaba Ebrahimi
Mojtaba Ebrahimi is currently a Postdoc Research Associate at LIRIS laboratory (Laboratoire d'InfoRmatique en Image et Systèmes d'information), University of INSA Lyon in France. He is working on the development of a digital twin for the known industrial problems like sequencing and scheduling. He obtained his PhD and MSc degrees in Industrial Engineering from Department of Industrial Engineering of INSA Lyon-France (National Institute of Applied Sciences). His research interests include Evolution of World Class Manufacturing system, production planning in manufacturing systems, dynamic sequencing in manufacturing systems, utilisation of simulation software for operational industrial problem and applied operations research. He has published several papers in international conferences.
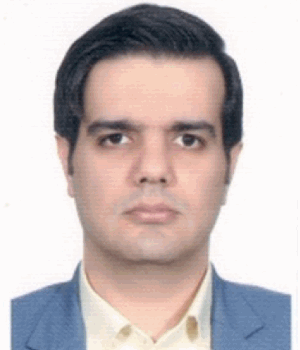
Mehdi Mahmoodjanloo
Mehdi Mahmoodjanloo is currently a Postdoc Research Associate at LIRIS laboratory (Laboratoire d'InfoRmatique en Image et Systèmes d'information), University of INSA Lyon in France. He obtained his PhD, MSc and BSc degrees in Industrial Engineering from the University of Tehran (2021), Amirkabir University of Technology (Tehran Polytechnic) (2007), and Sharif University of Technology (2005), respectively. His research interests include scheduling in manufacturing systems, applied operations research, logistics optimisation, and machine learning. He has published several papers in reputable journals and international conferences.
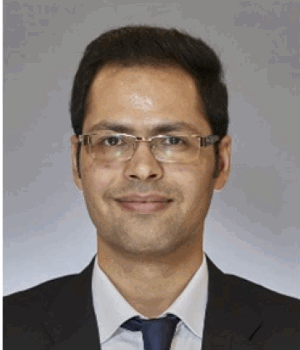
Behnam Einabadi
Behnam Einabadi is a PhD candidate in Industrial Engineering at the University of INSA Lyon in France. He obtained his M.Sc. double degrees in Industrial Engineering from the University of Tehran and Arts et Métiers ParisTech and he has obtained his B.Sc. from Kharazmi University. Respectively, He does his research as a CIFRE (Industrial Training Convention by Research) PhD candidate at the LIRIS laboratory. His research interests include predictive maintenance, maintenance and production planning and scheduling in manufacturing systems, applied operations research, data science, and machine learning. He has published some papers in reputable international conferences.
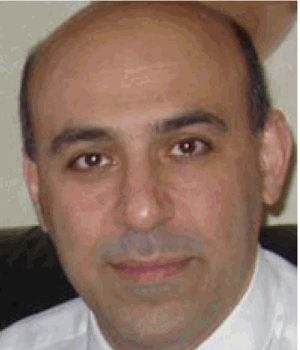
Armand Baboli
Armand Baboli is currently an Associate Professor in the Department of Industrial Engineering of INSA Lyon-France (National Institute of Applied Sciences) and he carries out his research activities in LIRIS laboratory (Laboratoire d'InfoRmatique en Image et Systèmes d'information). His recent research focuses on intelligence, robustness, flexibility, and agility in the design, configuration, and optimisation of production systems and the supply chain, as well as the evolution of traditional production systems into intelligent production systems (the factory of the future & industry 4.0). He is currently working on the decision support system & methods, called ‘dynamic predictive decision making’. He develops the methods and tools by combining the concepts that come from data science, operation research, and simulation. He is the creator and principal investigator of several industrial collaborations. Actually, he is Chair of three long projects with Dassault FalconJet-USA (2015-2021) concerning ‘smart supply chain’, Fiat Powertrain Technologies (2015-2022) concerning ‘Transformation of the production system towards industry 4.0’, and Volvo Group (2018-2024) concerning ‘Dynamic predictive production planning sequencing and line balancing’.
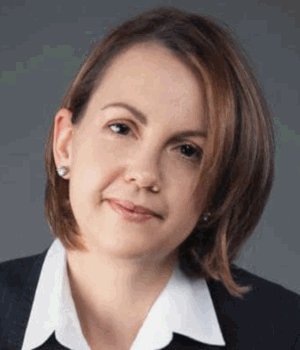
Eva Rother
Eva Rother is currently Plant Manager in FPT Industrial BLY, France and in charge of Industry 4.0 for FPT Industrial Manufacturing (Europe & South-America). She graduated industrial Engineering PhD from Department of Industrial Engineering of INSA Lyon-France (National Institute of Applied Sciences) after 18 years as operational manager in different automotive compagnies. Her research interests include managerial evolution in digital transformation context, lean & World Class Manufacturing system evolution and applied operations research.