Abstract
As industrial Internet of things (IIoT) for Manufacturing Service Collaboration (MSC) is becoming the current trend to accelerate the upgrade iteration of manufacturing capability, developing the robust IIoT platform operation mechanism for MSC is crucial to promote the continuous and stable service collaboration in the presence of supply and demand uncertainties. This paper studies the operational robustness of the IIoT platform for MSC. Firstly, the operation performances, requirements, and challenges of the IIoT platform towards manufacturing collaboration are analysed in classified platform practices, which can provide a comprehensive cognition about platform operation for manufacturing collaboration. Then, to evaluate the tolerance and persistence capabilities of MSC under supply and demand uncertainties, a graph-based operational robustness analysis method of the IIoT platform for MSC is proposed. The IIoT platform operation network for MSC is modelled as an interdependent network-of-network structure based on graph theory, which helps to characterise MSC performance properties under complexities. By combining manufacturing properties with network statistics, the evaluation metrics of operational robustness are established, which is done to quantise the MSC effectiveness under uncertainty effects. A case about customised manufacturing of automobiles illustrates the application of the proposed methods. Finally, future studies about robust MSC regulation are discussed.
Disclosure statement
No potential conflict of interest was reported by the author(s).
Data availability statement
The authors confirm that the data supporting the findings of this study are available within the article.
Additional information
Funding
Notes on contributors
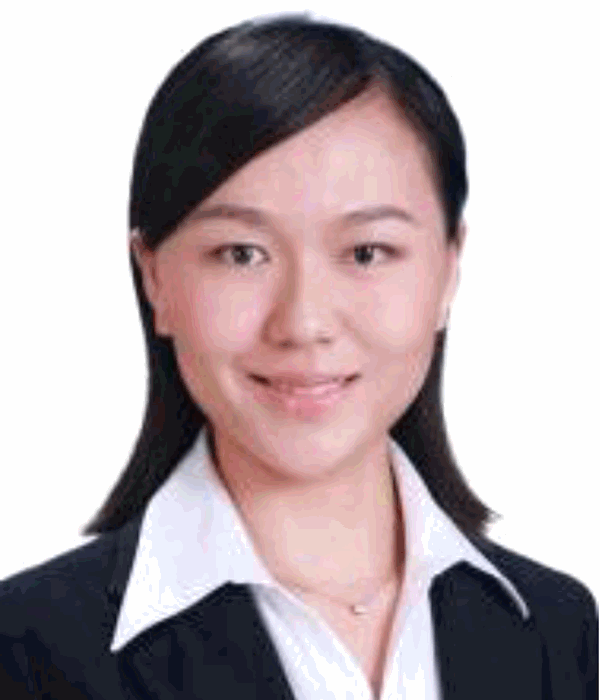
Ying Cheng
Ying Cheng received the B.S. degree in Mechanical Engineering from Wuhan University of Technology, Wuhan, China in 2010, and received the Ph.D. degree in Control Science and Engineering from Beihang University, Beijing, China in 2016. She is currently an Associate Professor with the School of Automation Science and Electrical Engineering, Beihang University, Beijing, China. Her research interests include service-oriented smart manufacturing, manufacturing services supply-demand matching and collaboration. She has authored over 40 journal or conference papers in the above areas.
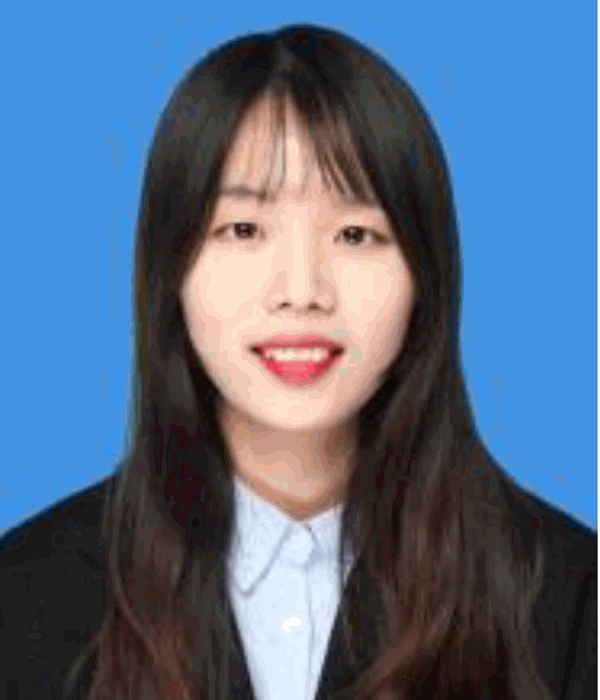
Yanshan Gao
Yanshan Gao received the B.S. and Master degrees in Control Science and Engineering from Henan Polytechnic University, Jiaozuo, China, in 2012 and 2016. She is currently pursuing the Ph.D. degree in School of Automation Science and Electrical Engineering, Beihang University, Beijing, China. Her research interests cover manufacturing service collaboration and robust control in complex networked systems.
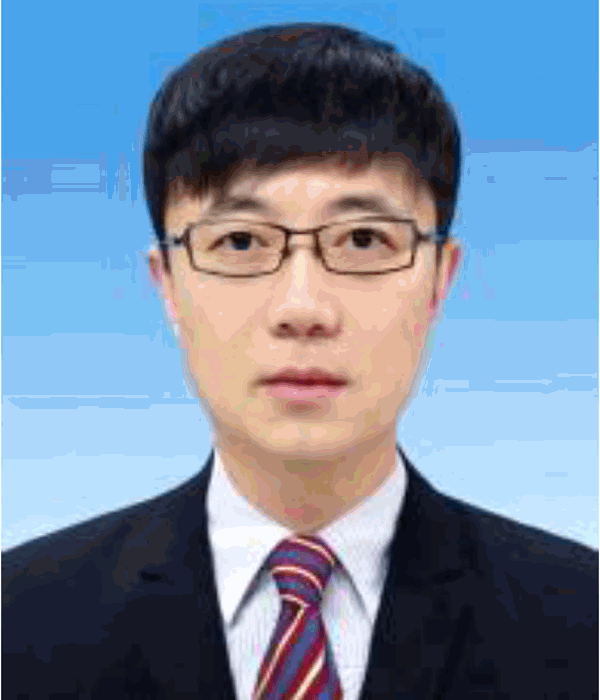
Lei Wang
Lei Wang received the B.E. degree in automation, the B.S. degree in applied mathematics, and the Ph.D. degree in Control Science and Engineering from Zhejiang University, Hangzhou, China, in 2004, 2004 and 2009, respectively. He is currently a Professor with the School of Automation Science and Electrical Engineering, Beihang University, Beijing, China. His current research interests include modelling, control and optimisation in complex networked systems. He has authored over 40 journal papers in the above areas.
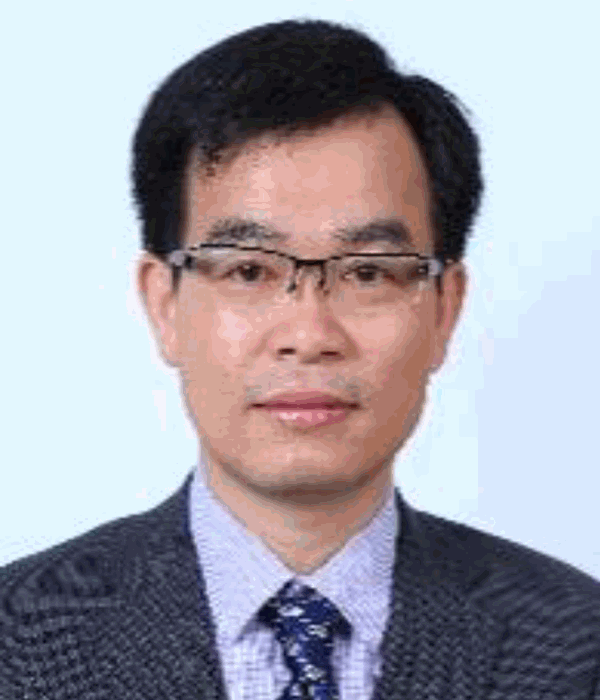
Fei Tao
Fei Tao received the B.S. and Ph.D. degrees in Mechanical Engineering from Wuhan University of Technology, Wuhan, China, in 2003 and 2008. He is currently a Professor with the School of Automation Science and Electrical Engineering, Beihang University, Beijing, China. His research interests include service-oriented smart manufacturing, manufacturing service management, and digital twin driven product design/manufacturing/service. He has authored five monographs and over 100 journal papers in the above areas.
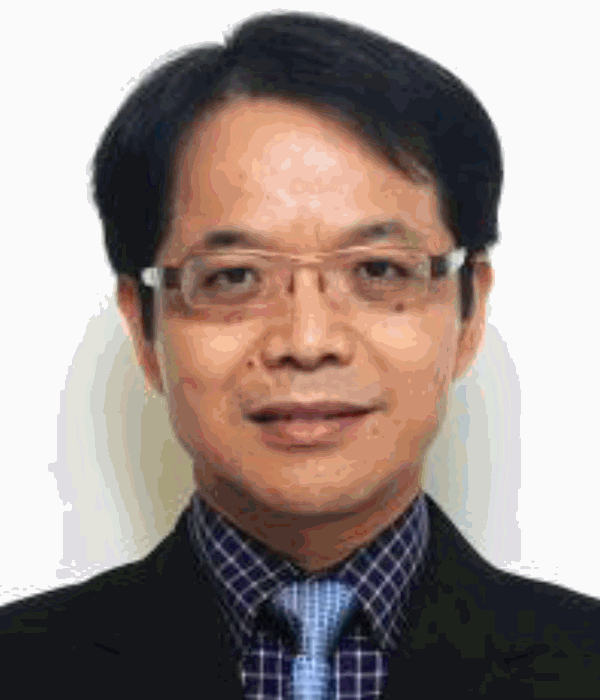
Qing-Guo Wang
Qing-Guo Wang received B.Eng. in Chemical Engineering, M. Eng. and Ph.D. degrees in Industrial Automation from Zhejiang University, China, in 1982, 1984, and 1987, respectively. He is currently a Chair Professor in the Institute of Artificial Intelligence and Future Networks from Beijing Normal University-Hong Kong Baptist University United International College, Zhuhai, China. His present research interests are mainly in modelling, estimation, prediction, control, optimisation and automation for complex systems, including but not limited to, industrial and environmental processes, new energy devices, defense systems, medical engineering, and financial markets. He has published over 300 international journal papers and 7 research monographs in the above areas.