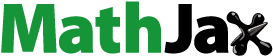
Abstract
The study aims to identify the current trends, gaps, and research opportunities in research pertaining to the disruptive field of artificial intelligence (AI) applications in supply chain management (SCM). Since SCM represents managerial innovation due to its new way of integrated system thinking, SCM has emerged as one of the most fruitful business disciplines for AI applications. The study utilises bibliometric review in tracing the evolution of AI research in SCM and further synthesises decades of past AI research efforts to develop viable solutions for various supply chain problems and then proposes promising future research themes that would enrich supply chain decision-aid tools. The study identified five main research clusters through scholarly network and content analysis. The identified themes were: (a) supply chain network design (SCND), (b) supplier selection, (c) inventory planning, (d) demand planning, and (e) green supply chain management. As the role of AI in SCM continues to grow, there is a growing need for exploiting AI as a way to add value to supply chain process. The study proposes a research framework which will help academicians and practitioners in identifying current research patterns of AI in SCM.
1. Introduction
In the era of the fourth industrial revolution characterised by a fusion of disruptive technologies such as artificial intelligence (AI) and robotics, a traditional boundary between man and machine becomes blurred. As the role of humans in supply chain operations changes, management paradigm shifts has accelerated for the last decade. For example, conventional manufacturing has been transformed into smart, data-driven cyber manufacturing with the aid of cyber physical systems (CPS) and the internet of things (IoT) (Lee, Azamfar, and Singh Citation2019, Citation2018, Citation2015; Rüßmann et al. Citation2015). This kind of transformation not only improves the operational efficiency and productivity of the supply chain management (SCM), but also enhances supply chain visibility throughout the end-to-end supply chain processes (Ben-Daya, Hassini, and Bahroun Citation2019). One of the emerging catalysts for supply chain transformation is artificial intelligence due to its interoperability, data storage, and business analytics capabilities (Baryannis, Dani, and Antoniou Citation2019a; Spanaki, Karafili, and Despoudi Citation2019). Generally, AI is referred to as the use of computers for reasoning, recognising patterns, learning, or understanding certain behaviours from experience, acquiring, and retaining knowledge, and developing various forms of inference to solve problems in decision-making situations where optimal or exact solutions are either too expensive or difficult to produce (Min Citation2010). In a nutshell, AI provides a machine with the capability to carry out any cognitive functions that human used to handle through its self-awareness and self-learning. AI aims to design computer systems that can mimic human behavioural patterns and create knowledge relevant to problem-solving (Min Citation2010). Thus, AI is essential for automation and digitalization of supply chain activities and the subsequent improvement of supply chain productivity (Dolgui and Ivanov Citation2021). In particular, the use of AI in SCM can fundamentally metamorphose current business practices and managerial tasks. Gartner’s 2018 report has touted AI as the number one strategic technology and expected that global investments on AI based applications would surpass USD 50.2 billion by 2021 (IDC Citation2018). As such, the worldwide revenues from the AI market are expected to reach USD 2.59 trillion by 2021 (Statista Citation2018). AI is shaping, reinventing, and transforming the next generation business models and ecosystems such as digital twins, continuous adaptive security, and event-thinking (Gartner Citation2017).
AI was introduced in the early 1970s to create intelligent thinking machines capable of learning, aiding, and eventually, replacing human intelligence (Pomerol Citation1997). Despite the widespread popularity and enormous potentials of AI as a decision-making tool, AI has not been fully exploited in SCM (Min Citation2010). In the recent past, however, this trend seems to be slowly changing with the growing body of literature on AI applications to SCM. This paper intends to capture such trend shifts and assess the potential impact of AI on SCM through a bibliometric analysis. This paper also synthesises which themes of SCM have been studied and what has been learned from AI applications to SCM by reviewing a pool of more than 3500 published articles and filtering this collection to include significant works and investigators. This review uncovers important roles of AI in SCM. Using rigorous bibliometric tools aided by a comprehensive network analysis (e.g. citation and co-citation analyses), we identified five major clusters of research areas. These algorithmically identified clusters set the stage for topical classification of the published models and further investigation of the evolution of these clusters over the years. From these clusters, we gained detailed insights into research streams and common research interests, while pinpointing potential directions for future research. The bibliometric analysis reveals that there is a dearth of research dealing with analytical models and empirical studies.
Although AI is deployed by thousands of organizations around the world, the business potential of AI still remains untapped (Brynjolfsson and Mcafee Citation2017). As AI emerges from theoretical backgrounds to become the frontier of game-changing technologies, there is an urgent need for systematic development and implementation of AI to see its real impact in the next generation of data-driven supply chains (Kamble and Gunasekaran Citation2020; Lee et al. Citation2018). Therefore, in order to address the identified research gaps, the following research questions (RQs) will be answered:
RQ1: What is the extent of research pertaining to AI in SCM?
RQ2: What is the future outlook of AI applications to SCM?
The remainder of the paper is organised as follows: Section 2 describes the genesis of AI research. Section 3 highlights the review methodology used for the study. Bibliometric results and analysis are highlighted in Section 4. Section 5 presents the conceptualised proposed framework. Finally, research gaps, implications from the study, and future research directions are discussed.
2. Genesis of AI research
Artificial Intelligence (AI) was first coined by a team of Prof. John McCarthy and scientists in the year 1955 (Brynjolfsson and Mcafee Citation2017). Put simply, AI allows the machines to mimic human intelligence and helps them to recognise, analyse, and do things in ways like humans do (Pan Citation2016). Its abilities in executing the tasks are acquired through machine learning and deep learning algorithms. In a broad sense, highlights the various applications and domains of AI in fields such as industrial systems and engineering, data science, societal impacts of AI, supply chain risk management, and industry 4.0 based manufacturing systems.
Table 1. Application areas and domains of AI.
Practitioners often classify AI into two broad areas viz. artificial general intelligence (AGI) and artificial narrow intelligence (ANI). AGI can perform tasks that match or surpass human intelligence and ANI performs specific tasks (Bawack, Fosso Wamba, and Carillo Citation2019). AI has brought biggest advancements in data science through perception and cognition, which are the distinguished features of AI. The application areas of AI include machine learning, deep learning, computer vision, robotic process automation, speech and voice recognition, and neural networks as depicted in . The recent COVID-19 pandemic has highlighted how fragile global supply chains are (with longer physical flows and dependency on multiple tier suppliers across geographies). Thereby, the demand for resiliency, agility, and flexibility from the stakeholders has drastically increased and this calls in for AI enabled solutions for SCM. Almost all application areas of AI find their use across one supply chain decision-making area or the other (for example, predicting supply chain risks using machine learning (Baryannis et al. Citation2019b); trend forecasting in the fashion supply chain using logistic regression (Chakraborty, Hoque, and Kabir Citation2020); prediction of backorder scenarios in the supply chain using gradient boosting machine learning techniques (Islam and Amin Citation2020); forecasting (Nguyen et al. Citation2021); and machine failure mode analysis (Okabe and Otsuka Citation2021)). The wide adoption of AI in SCM is because of AIs ability to improve the decision-making capabilities, reduce cycle-times, and improve the overall operational efficiency. As the supply chains are becoming global, the level of complexity and uncertainty increases therefore, organizations worldwide are investing in autonomous AI enabled systems for improving their supply chain processes (Kohtamäki et al. Citation2019). The advantages of AI in SCM are powerful optimization capabilities, accurate forecasting capabilities, improved quality, lower supply chain costs, and safe working conditions. Studies in the recent past (see Toorajipour et al. Citation2021) have highlighted that AI in SCM can lend unprecedented value and competitiveness through effective forecasting and efficient risk management techniques.
AI in SCM will enable end-to-end visibility and transparency leading to quick and responsive decision-making (Pournader et al. Citation2021; Riahi et al. Citation2021; Belhadi et al. Citation2021; Dora et al. Citation2021; Rodríguez-Espíndola et al. Citation2020). By implementing AI in their SCM systems, organizations can effectively predict bottlenecks for streamlining production planning, smart maintenance, smart service operations, smart manufacturing, and scheduling (Helo and Hao Citation2021; Wang, Skeete, and Owusu Citation2021). Based on the real-time information, manufacturers can predict seasonal fluctuations and reduce the bullwhip effects through improved resource planning and demand-driven manufacturing. In case of inventory optimization, drawing upon from the supply-demand data, AI based systems help in accurate planning which leads to reduced wastage and costs (Oroojlooyjadid et al. Citation2021; Pillai et al. Citation2021). In case of procurement and supplier selection, spend analytics plays a critical role as it helps in analysing the procurement data which is then used to track procurement and supplier performance. Majority of the organizations deploy AI-enabled SCM optimization software/ enterprise platforms (such as ELI by Throughput Inc.; Luminate by Blue Yonder; NEXXE by GEP; BEO by NebulARC; llama.ai by LLamasoft to name a few) which deliver analytical insights based on cognitive predictions. The insights (based on cost, time, and revenue data) delivered by these software help in improving the overall supply chain performance.
A brief overview of AI algorithms and their applications in SCM are highlighted in the Appendix 1.
3. Review methodology
A literature review lays the foundation for any research. It helps deliver useful insights into main research streams, emerging fields, and new knowledge guiding future research initiatives (Fahimnia, Sarkis, and Davarzani Citation2015; Govindan et al. Citation2015b). Subsequently, a complete analysis and thorough assessment of the relevant literature was done, for identifying research gaps which need to be filled for charting future research directions (Tranfield, Denyer, and Smart Citation2003). The present study adopted the review procedure as highlighted by Saunders, Lewis, and Thornhill (Citation2016). The literature review is achieved using the three phases as proposed by Tranfield, Denyer, and Smart (Citation2003) comprised of planning the review, review execution, and finally, reporting the review. To ensure a wide scope of article selection for the most updated and comprehensive review, we searched all electronic databases such as Web of Science and Scopus. In particular, we chose Scopus database for referring and searching the published articles since it has the largest collectionsFootnote1 of refereed literature (Geraldi, Maylor, and Williams Citation2011). The bibliometric review methodology in this research followed the PRISMA framework (see ) (Moher et al. Citation2009).
Figure 2. Bibliometric review methodology.
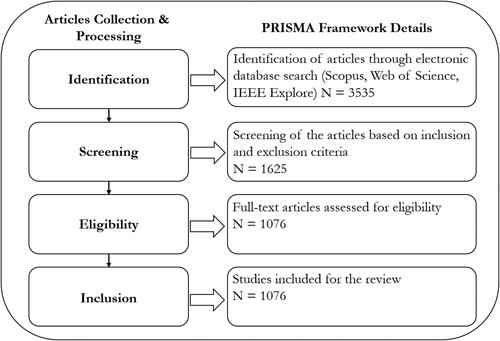
3.1. Keyword selection
For establishing a comprehensive, unbiased, and a reproducible article search procedure in this current bibliometric review, we cited only the most significant and widely cited articles relevant to the field of AI in SCM (AI-SCM). To check the relevancy, we used the following keyword search queries (see ):
Table 2. Keywords.
The use of aforementioned keywords also set the tone for the bibliometric analysis in this paper.
3.2. Collection and filtering of articles
The time period involved for the literature review covers the studies published during 1994–2021. The initial search returned 3535 research papers (search was carried in January ‘21). This search excluded academic sources editorial notes, book chapters, working papers, conference proceedings, unpublished reports, and doctoral dissertations, since they often represent preliminary research work or unverified practical viewpoints (Lamba and Singh Citation2017; Ramos-Rodríguez and Ruíz-Navarro Citation2004). A further refinement based on the above exclusion criteria led to a drop in the number of articles to 1625. Among these articles, we segregated the shortlisted articles into articles from top 25 SCM journals (based on impact factor). The final list of articles selected for this bibliometric analysis was 1076. highlights a yearly breakdown of these articles, and their publication counts and shows a dramatic surge of AI-SCM publications for the last 15 years. Its exponential growth started in 2012, a year after the Industry 4.0 was introduced by Germany as the next wave of industrial revolution that theorises the AI thinking of man-machine interfaces.
3.3. Data analysis
The structural dimensions of the citation analysis that allowed us to categorise the past literature could be carried out inductively or deductively. For a citation analysis, we began with an inductive approach (Fahimnia, Sarkis, and Davarzani Citation2015). To carry out the literature analysis, we also took deductive approach prior to actual data analysis. Herein, an inductive citation analysis helped us to determine the structural dimensions and categories of the published literature through generalization, while a deductive citation analysis helped us categorise the past articles prior to their selection and inclusion for our literature review (Mayring Citation2003). In addition, we conducted the data analysis using a bibliometric analysis and a network analysis. Particularly, the bibliometric analysis was carried out using R which is helpful in the analysis of a dynamic field of study such as bibliometrics (Aria and Cuccurullo Citation2017). For network analysis part, we utilised VOS Viewer (Van Eck and Waltman Citation2009). Both software programs are open source and are able to work on large datasets with a wide range of visualization, innovative analysis, and investigation options.
4. Analysis and results
As a structured methodology to classify and categorise existing AI-SCM literature, we performed bibliometric analysis and network analysis to be described in detail in the following subsections.
4.1. Bibliometric analysis
According to Feng, Zhu, and Lai (Citation2017), a bibliometric analysis provides the following advantages over the other traditional methods such as the content analysis. First, bibliometric analysis can efficiently handle many articles. Second, it enables us to analyse the relationships among articles, keywords, citations, and co-citations and thus provide comprehensive information about the research area. Additionally, bibliometric analysis helps visualise research streams and thus easily identify the future research avenues in the field. The top publishing outlets are highlighted in Appendix 2.
4.1.1 Descriptive analysis
The preliminary data analysis helps capture the basic information of the literature. recapitulates the generic descriptions of the AI-SCM literature reviewed for the preliminary data analysis. shows the publication trend starting from 1994 during which the first AI-SCM article was published in the scholarly journal. A number of published articles in the AI-SCM field was scarce until the year 1999. In fact, there were a total of mere nine AI-SCM articles during the period of 1994 through 2001. The domain started to gain traction from the year 2002 with 12 articles in that year to 151 articles in the year 2019. Interestingly, research outputs published for the last five years (2015–2020) add up to nearly 45% of the total articles published in this field. This growing trend is expected to continue into the foreseeable future. This trend reflects the growing scholarly recognition and the subsequent popularity of the AI-SCM field.
Table 3. Descriptive statistics of bibliographic collection.
4.1.2 Author influence
highlights the most prolific authors based on the number of articles published. M K Tiwari, with 30 articles followed by F T S Chan with 22 articles, turned out to be two most prolific authors.
Table 4. Author contribution to the AI literature.
4.1.3 Keyword analysis
As He (Citation1999) argues that keyword analysis is a scientific approach for discovering the linkages among sub-fields of a particular research field. summarises a list of the top 20 keywords based on the frequency of their usage by the authors in their articles. ‘Supply chain management’ with 1036 occurrences is the most frequent keyword within the reviewed articles. Other frequently used keywords include ‘supply chains’, ‘decision making’, ‘genetic algorithms’, and so forth.
Table 5. Frequency of keyword occurrences.
depicts the trends of various emerging keywords (research focuses) across the timeline of literature. The keywords reflecting most frequently studied subjects have emerged during the period 2012–2018. AI-SCM research started with subjects tied to ‘intelligent agents’ and ‘inventory control’. In the recent past, such research covered latest topics such as ‘sustainability’, ‘sustainable development’, ‘sustainable supply chains’, and so forth. It can also be observed that topics such as sustainable supplier selection, green supply chain, green supplier selection, and supply chain finance in combination with AI/ ML techniques are garnering a lot of attention from the practitioners and academia.
4.2. Scholarly network and content analysis
Rapid recent advancement in AI theory and its application to SCM produced an enormous amount of digital scholarly data on AI-SCM. These data contained various forms of publications such as journal articles, books, reports, and proceeding papers. Among these, journal articles themselves allowed us to compile and analyse scholarly source of information using scholarly network analysis (SNA). Generally, SNA is designed to provide deep insights into the interaction of research aggregates such as citation, co-citation, co-authorship, and bibliographic coupling through the data-driven, network-based bibliometric analysis (Yan and Ding Citation2012). SNA helps us discover the scholarly collaboration between authors and depict how individual scientific ideas of authors can generate meaningful scientific findings through collaborative research. It also helps us find relationships between cited papers and a set of papers which cited those papers. This proves useful for a detection of academic communities working in the related area. Furthermore, it allows us to discover the current research areas as well as their evolution over time, not to mention the identification of the relationships between topics in the specific research field through finding the co-occurrence of keywords. Recognising the aforementioned usefulness of the SNA, we conducted SNA for the AI-SCM literature (see ). As a complementary tool, we used a content analysis.
The content analysis basically serves to record and identify intersubjective and objective characteristics from the selected studies (Maditati et al. Citation2018; Fahimnia, Sarkis, and Davarzani Citation2015). Thus, the results derived from a content analysis are credible and consistent if multiple researchers are engaged in the similar process (Duriau, Reger, and Pfarrer Citation2007). We systematically reviewed the contents of 44 selected articles written by researchers who conformed the underlying sub-themes of AI-SCM. These 44 articles had at least 150 citations for citation mapping after examining competing maps with at 145 citations and at least 155 citations, since these many citations reflected the criticality and vitality of their contributions to the literature of a particular topic (i.e. AI-SCM). To facilitate the content analysis, a concept matrix was formulated based on the study by Salipante, Notz, and Bigelow (Citation1982) which consists of the following attributes: title of the article, author(s), publication year, keywords, research question(s), methodology used, theories used, main themes, sub-themes, and important findings of the selected 44 articles. The five major AI in SCM research clusters were considered as potential themes for each of the articles in the concept matrix. However, as the analysis progressed, article themes and sub-themes were defined through an iterative analysis of the contents of the selected 44 articles. summarises five different clusters of 44 most frequently cited articles and their authors. depicts a number of clusters that were generated by using the VosViewer software.
Table 6. Clusters of most frequently cited AI-SCM research work.
As a result, five key research streams of AI applications in SCM were identified: (a) supply chain network design (SCND), (b) supplier selection, (c) inventory planning, (d) demand planning, and (e) green supply chain management. shows that supplier selection is the most popular theme of AI applications with 128 frequencies; genetic algorithms are most dominant AI tools for solving SC problems with a frequency of 397 articles.
In the following sub-sections, the key theories, methods, and findings of the selected articles are discussed briefly in the context of their respective research streams and sub-streams.
4.3. AI taxonomy by the application area
To have a general panorama of prior AI studies and their key research themes, the present study highlights a taxonomy depicting two broad classifications viz. (1) application area as a basis for identifying particular fields of SCM problems for which AI is most suitable; (2) the methodology as a basis for assessing the theoretical progress and development in AI-SCM studies. Figure shows the yearly trends of published AI-SCM studies with respect to their application areas for the last two decades. The following subsections will summarise and synthesise selected (noteworthy) AI studies that applied various AI tools to a spectrum of challenging SCM problems.
4.3.1 Supply chain network design
Supply chain network design (SCND) is one of the most critical area of strategic decision-making in SCM. SCND is primarily concerned with determining the optimum location and size of facilities (e.g. plants and warehouses) and the flow of products through the facilities. Due to its criticality, SCND has one of the popular subjects of scientific inquiries within the AI framework. For example, one of the earliest AI-SCM research efforts includes Syarif, Yun, and Gen (Citation2002) who developed a spanning tree-based genetic algorithm (GA) built upon Prüfer number representation to solve SCND problems. Min, Ko, and Ko (Citation2006a, Citation2006b) proposed a nonlinear integer program and utilised GA for solving the multi-echelon SCND problem involving product returns and freight consolidation across geographical areas and holding time. Altiparmak et al. (Citation2006) developed steady-state GA combined with a multiple-objective programing technique (MOPT) to find a set of Pareto-optimal solutions for the multi-product SCND problem. In their study, the proposed GA was complemented by simulated annealing and Lagrangian relaxation heuristics. Ko and Evans (Citation2007) presented a mixed integer nonlinear programming model for designing a distribution network that integrated both forward and reverse product flows. Similarly, Pishvaee, Farahani, and Dullaert (Citation2010) proposed MOPT for solving SCND problems in such a way that they avoided a sub-optimality caused by a separate, sequential design of forward and reverse logistics networks. Govindan et al. (Citation2014) introduced a MOPT model that took into account sustainability for designing a perishable food distribution network. They developed an AI-based meta-heuristics to solve the MOPT problems within a two-echelon, distribution network framework. Other noteworthy AI-SCM studies that used similar solution procedures such as GA for tacking variants of SCND problems include Kannan, Sasikumar, and Devika (Citation2010), Wang and Hsu (Citation2010), and Yeh and Chuang (Citation2011).
4.3.2 Supplier selection
One of the critical steps of sourcing in the SCM is to find right sources of supply by evaluating and selecting the right supplier(s) (Min Citation2015b). Due to its importance to the upper stream of SCM, it garnered interest from many researchers and has become the popular subject of quantitative analysis including AI methodologies. Another aspect of AI in SCM related to procurement and supplier selection is spend analytics wherein procurement data is collected, processed, and analysed. Organizations then use insights from spend analytics for managing their suppliers, tracking procurement and supplier performance, and optimising costs. As such, there exist the abundant AI-SCM literature focusing on supplier selection problems (Weber, Current, and Benton Citation1991; Pal, Gupta, and Garg Citation2013). All the AI-SCM studies in this cluster utilised a combination of fuzzy sets theory and multi-criteria decision-making (MCDM) models for supplier selection due to the vagueness of supplier attribute information and conflicting goals of identifying low-cost and reliable suppliers. For instance, Kumar, Vrat, and Shankar (Citation2004) developed a fuzzy, mixed-integer, goal programming model for supplier selection problem with conflicting goals of minimising the net cost, minimising the net rejections, and minimising the net late deliveries subject to constraints of the buyer's demand, the supplier’s capacity, the supplier’s quota flexibility, purchased value of items, and budget allocation to individual supplier. Chen, Lin, and Huang (Citation2006) utilised the Technique for Order of Preference by Similarity to Ideal Solution (TOPSIS) method and fuzzy set theory for dealing with the supplier selection problems. Chan et al. (Citation2008) discussed the fuzzy based Analytic Hierarchy Process (fuzzy-AHP) for efficiently tackling both quantitative and qualitative decision factors involved in global supplier selection. Boran et al. (Citation2009) proposed a TOPSIS method combined with intuitionistic fuzzy sets for selecting the right supplier in a group decision-making environment. They utilised the intuitionistic fuzzy weighted averaging (IFWA) operator for aggregating individual opinions of decision makers for rating the importance of supplier selection criteria. Other AI-SCM studies similar to the aforementioned studies included Amid, Ghodsypour, and O’Brien (Citation2006), Lin, Chiu, and Chu (Citation2006), Chou, Chang, and Shen (Citation2008), and Sanayei, Mousavi, and Yazdankhah (Citation2010) utilised the variations of fuzzy set theory in the MCDM environments to select the most desirable supplier. Unlike these studies, Kuo, Wang, and Tien (Citation2010) developed an artificial neural network (ANN) combined with data envelopment analysis (DEA) and analytic network process (ANP) to select green (most environment-friendly) supplier while making tradeoffs among the multiple criteria of cost, time, damage/loss, flexibility, and documentation ability of potential suppliers.
4.3.3 Green SCM
With the increased awareness of sustainability and mounting environmental pressures across the world, green SCM drew considerable interest from many academic communities (Min and Kim Citation2012; Kannan, de Sousa Jabbour, and Jabbour Citation2014). Recognising the emergence of green SCM, Govindan, Khodaverdi, and Jafarian (Citation2013) presented a fuzzy MCDM model to green sourcing problem with sustainable supply chain initiatives based on the Triple Bottom Line (TBL). Similarly, Kannan, de Sousa Jabbour, and Jabbour (Citation2014) proposed a fuzzy TOPSIS model for tackling green sourcing problem encountered by a Brazilian electronics company. Other notable AI-SCM studies that used variants of fuzzy set theory for green SCM included Tseng (Citation2011), Barari et al. (Citation2012), Lin (Citation2013), Tseng and Chiu (Citation2013), Govindan et al. (2015), Awasthi and Kannan (Citation2016), and Qin, Liu, and Pedrycz (Citation2017).
4.3.4 Inventory planning
Inventory planning and control systems are implemented by firms for controlling demand variability, reducing costs throughout the SC processes, maintaining sufficient inventory levels, and meeting adequate customer service levels. To tackle inventory planning problems, Petrovic, Roy, and Petrovic (Citation1999) proposed a hybrid fuzzy sets and simulation model in an uncertain decision environment. Following suit, Giannoccaro, Pontrandolfo, and Scozzi (Citation2003) exploited fuzzy set theory to define a multi-echelon inventory planning problem. Liang (Citation2008) developed a fuzzy multi-objective linear programming (FMOLP) model with a piecewise linear membership function to solve multi-period, multi-product inventory planning problems with vague objectives. Similarly, Peidro et al. (Citation2010) developed a fuzzy linear programming model for solving an inventory planning problem in a multi-echelon, multi-product, multi-period supply chain network. Rather than using the fuzzy set theory, Carbonneau, Laframboise, and Vahidov (Citation2008) applied advanced machine learning techniques such as neural networks, recurrent neural networks, and support vector machines to forecast the distorted inventory demand caused by bullwhip effect.
4.3.5 Demand planning
Demand planning sets the tone for supply chain activities since it has a trickle-down effect on the upper stream supply chain activities involving logistics, manufacturing, and sourcing. Effective demand planning enhances labour productivity, speeds up product flows, and increases profits and revenues (Quariguasi Frota et al. Citation2010). In addition, accurate demand planning helps mitigate supply chain risks. Recognising such importance of demand planning, Yu, Graham, and Min (Citation2002) developed an agent-based demand forecasting technique that combined human expertise and data mining techniques to predict the aggregate demand for new products. Their experiments indicated that the dynamic pattern matching procedure built on AI outperformed exponential smoothing techniques with respect to forecasting accuracy. Liang and Huang (Citation2006) proposed a multi-agent system and employed genetic algorithm to control inventory and minimise the total supply chain cost by creating forecasting knowledge obtained from multiple agents. Aburto and Weber (Citation2007) presented a hybrid intelligent system combining Autoregressive Integrated Moving Average (ARIMA) models with neural networks for a volatile demand forecasting problem. Efendigil, Önüt, and Kahraman (Citation2009) proposed artificial neural networks and adaptive network-based fuzzy inference system techniques to manage uncertain demand with incomplete demand information.
4.3.6 AI in healthcare SCM
To cope with the mounting health care cost resultant from recent healthcare crisis such as the ongoing COVOID-19 pandemic, a growing number of healthcare service providers made conscious efforts to improve their healthcare productivity. One of the best ways to improve such productivity is technical innovation. Examples of technical innovation that have wide-application potentials for healthcare SCM include robotics and expert systems. For instance, robotics can help healthcare professionals monitor the patient’s medical condition and recovery progress by managing his/her medical charts and vital signs without human intervention. An expert system can also diagnose the patient’s symptoms by using the pre-developed If–Then rules (Min Citation2014). With the aid of wearable technology, the medical doctor can generate such diagnosis and alert the patient about his/her health concerns in real time. In addition, AI can be useful for developing plans of smart delivery of medical supplies and pharmaceuticals essential for patient care.
Cognizant of the AI’s usefulness for healthcare SCM, a growing number of recent studies reported the successful applications of AI to healthcare supply chain settings (Min Citation2017; Mathur et al. Citation2018). For example, Sabegh, Mohammadi, and Naderi (Citation2017) developed the artificial neural network and hybrid genetic algorithm to respond to natural disasters in such a way that a pharmaceutical company could minimise costs of poor product quality and transportation in the pharmaceutical supply chain. Rath, Rajaram, and Mahajan (Citation2017) proposed a two-stage, mixed-integer stochastic dynamic programming model with recourse for solving the problems of staffing and scheduling at one of the largest medical centres in the U.S. Fan et al. (Citation2018) investigated how receptive medical professionals are of artificial intelligence-based medical diagnosis decision support systems (AIMDSS) for disease treatments in the Chinese hospitals in terms of trustworthiness. Zahiri, Jula, and Tavakkoli-Moghaddam (Citation2018) presented a novel robust possibilistic optimization model to minimise the total costs and maximum unmet demand of perishable drugs in a uncertain pharmaceutical SC network. Aswani, Shen, and Siddiq (Citation2019) designed a principal-agent problem highlighting the interaction between Medicare Shared Savings Program (MSPP) and Medicare provider. In their study, the MSPP sets the financial benchmark for each Medicare provider, and each Medicare provider decides what investment to make in order to reduce costs.
More recently, Kargar, Paydar, and Safaei (Citation2020) proposed a multi-item multi-objective linear programming model under uncertainty for designing a medical waste reverse supply chain. Their proposed model had three objective functions viz. minimising total costs, utilization of best medical waste treatment technology, and minimising the total stored medical waste. Abbassi et al. (Citation2020) addressed a two-echelon location-distribution problem of non-medical products in healthcare supply chain logistics using multi-objective particle swarm optimization and genetic algorithm and develop a multi-objective mathematical model for minimising distribution cost and distribution time. Sadjadi, Ziaei, and Pishvaee (Citation2019) designed a vaccine supply chain using the mixed integer programming model considering strategic decisions for each echelon and tactical decisions among the echelons of the vaccine supply chain. Dehghani, Abbasi, and Oliveira (Citation2021) employed a two-stage stochastic programming model along with the Quasi-Monte Carlo sampling approach to manage inventories of perishable blood in uncertain demand environments. They also tested the performance of their proposed model in proactive transshipment from the central flood bank to a network of hospitals in Australia.
4.4. AI classification by methodology
As discussed in earlier section 4.3, a multitude of AI tools was utilised to solve various SCM problems. These tools include genetic algorithm, fuzzy set theory (or logic), TOPSIS, AHP/ANP, artificial neural network as shown in . Though AI tools are still evolving, and their application potentials are growing, we noticed that some of them had been frequently adopted to solve SCM problems. shows the yearly trend of published AI-SCM articles with respect to their particular AI-tools during the period of 1998 through 2020. With that in mind, the following subsections recapitulate a list of most popular AI tools relevant to SCM.
4.4.1 Genetic algorithm
The roots of genetic algorithm (GA) can be traced back to the theory of evolution. The rules of natural selection processes found in the nature to create the best-fit organisms are impersonated in GA to solve challenging combinatorial optimization problems (Min Citation2015a). Combinatorial optimization problems, which possess the possibility of building a function which evaluates the fitness of a given solution to a given problem, have often been solved by using GAs. GA translates the potential solutions of the problem into numerical strings known as chromosomes. Through multiple iterations on chromosomes using genetic operators (viz. crossover, mutation, and selection) to the whole population, GA provides solutions that are not necessarily optimal, but satisfice the fitness criteria to the optimization problem. GAs find their application to a variety of challenging supply chain network design and inventory management problems (Min Citation2015a). These problems include but are not limited to: vehicle routing and scheduling (Wang et al. Citation2016; Biesinger, Hu, and Raidl Citation2018), minimum spanning tree (Contreras-Bolton et al. Citation2016; Contreras-Bolton et al. Citation2016; Shi et al. Citation2016; Singh and Sundar Citation2020); delivery and pickup (Al Chami et al. Citation2017; Jia, Jing, and Hong Citation2019; Rüther and Rieck Citation2020); and location – allocation problems (Zhou, Min, and Gen Citation2002; Min, Ko, and Ko Citation2006a); and inventory planning and control problems (Disney, Naim, and Towill Citation2000; Pasandideh, Niaki, and Nia Citation2011; Petering, Chen, and Hsieh Citation2019).
4.4.2 Artificial neural networks
Artificial neural networks (ANN) are theoretically designed to function like the brain cells of a living organ and are classified as a subset of deep learning. The deep learning neural network uses interconnected network of computer memories to learn from experience, recognise different features and patterns, and even process ambiguous or abstract information using weights, data inputs, and bias. The deep neural networks comprise interconnected nodes forming multiple layers. Some of the complex deep learning neural networks are convolutional neural networks (CNN) which find applications in computer vision; and recurrent neural networks (RNN) which find applications in speech recognition and natural language processing. Although ANNs are of wide variety and type, feed-forward error back-propagation type neural nets are mostly deployed in SCM. In SCM, ANN was successfully utilised for developing hierarchical SC planning that determined the time/capacity needed for setups, estimated optimal lot-size between successive SC processes, and linked inventory and scheduling decisions at the lower level to demand and production planning decisions at the higher level (Rohde Citation2004). As illustrated in section 4.3, ANN was one of the AI tools that were proven to be effective for handling various SC problems including demand forecasting (Aburto and Weber Citation2007).
Özkan and İnal (Citation2014) proposed the Adaptive Neuro-Fuzzy Inference System (ANFIS) for solving multi-criteria decision-making problems involving supplier evaluation and selection. ANFIS was a feed-forward, hybrid learning ANN where each layer is a neuro-fuzzy system component and tended to perform better for clustering and pattern recognition than the standalone ANN did based on the comparative experiments. Similarly, Tavana et al. (Citation2016) developed a hybrid ANFIS–ANN model for evaluating and ranking potential suppliers based on their past performances. Liu et al. (Citation2019) also exploited the ANFIS-ANN model to identify factors significantly affecting price fluctuations in a steel supply chain in China. Lima-Junior and Carpinetti (Citation2019) proposed a SC performance prediction system based on ANNs (multilayer perceptron neural networks) and SCOR model. Their proposed AI-based methodology was useful for evaluating and comparing historical SC performance data. Carrera, Mayorga, and Peng (Citation2020) utilised ANNs for developing a hybrid neuro-fuzzy analytical network (NFANP) approach that was intended to solve group decision-making problems. The NFANP approach was applied for selecting a right supplier with respect to ongoing supplier performance, supplier characteristics, and project management capabilities. When it came to supplier selection, there were several Neuro-Fuzzy applications in an AI domain. Nezamoddini, Gholami, and Aqlan (Citation2020) proposed a risk-based optimization framework using ANNs and genetic algorithms which could aid SC professionals in making SC decisions at all decision hierarchies (i.e. strategic, tactical, and operational levels).
4.4.3 Fuzzy logic/set theory
Fuzzy logic was introduced by Zadeh (Citation1965) and is an extension of Boolean logic that was designed for handling the ambiguity, inaccuracy, and uncertainty of objects. It aids the decision-making process without having to set clear-cut boundaries. It helps make definite decisions based on imprecise and ambiguous data (Ordoobadi Citation2009). It is an extension of the classical set theory which is based on fuzzy sets and provides a medium for highlighting the vagueness, subjectivity, and ambiguity with the help of mathematical formulations. Earlier studies such as Patel, Shah, and Chhinkaniwala (Citation2019) and Goularte et al. (Citation2019) utilised fuzzy logics to integrate with expert systems and pattern recognition to handle SC problems with uncertainty and/or opaqueness (Castillo et al. Citation2016; Wang et al. Citation2016; Hamamoto et al. Citation2018; Baykasoğlu and Gölcük Citation2019; Chaturvedi et al. Citation2019; dos Santos, Godoy, and Campos Citation2019; Jain and Singh Citation2020). Fuzzy set theory has diverse SC application potentials and has been widely explored for SC decisions with uncertainty and imprecise information. As discussed earlier in Section 4.3, many AI-SCM studies applied fuzzy set theory as one of the popular AI tools for addressing various SCM issues.
4.4.4 MCDM techniques with machine learning capabilities
Though MCDM techniques are generally viewed as one of the O.R. tools, AI and MCDM shares the same traits such as interactivity, reasoning of decision alternatives, decision support orientation, and user involvement in decision making (Perny and Pomerol Citation1999; Doumpos and Grigoroudis Citation2013). In particular, most MCDM techniques were designed to make tradeoffs among multiple conflicting criteria by rules (Perny and Pomerol Citation1999). These rules can be learned by machines and then can be applied automatically by those machines. Thus, some popular MCDM techniques can be categorised as a subset of AI tools. Earlier AI-SCM studies utilised various MCDM techniques with machine learning capabilities such as linear weighted average methods (Timmerman Citation1986); cost-ration method (Dobler, Lee, and Burt Citation1990); vendor profile analysis (Thompson Citation1990); and dimensional analysis (Kools et al. Citation1996) for sourcing problems in the upstream SCM. Though an integral part of AI tools, these MCDM techniques posed challenges for assigning weights (or relative importance) to various attributes as the weights were purely based on personal judgments and intuitions of the decision-maker. To overcome such a shortcoming of the aforementioned techniques, researchers proposed alternative scoring methods such as analytical hierarchy process (AHP), analytic network process (ANP), ELECTRE, TOPSIS, to name a few. These techniques provide a systematic way for determining the weights of multiple attributes following a series of pairwise comparison of all attributes including qualitative attributes that are difficult to express mathematically. These MCDM techniques are widely used for solving various SCM problems including supplier selection (Sari Citation2017), production planning and control (Rasmi, Kazan, and Türkay Citation2019), reverse logistics (Gu et al. Citation2019), and SC performance evaluation (Khan, Chaabane, and Dweiri Citation2019). However, these MCDM techniques have been used primarily as a complementary technique for a popular AI tool such as fuzzy set theory rather than a stand-alone AI tool.
The previous sub-sections highlighted the review findings, and it can be observed that AI techniques find their applications across all phases of the SC. Today’s turbulent business environment demands for tailor-made AI solutions that can drive the enterprise forward in terms of effective risk management (Baryannis et al. Citation2019b), overcoming operational challenges, sustainability related issues (Bechtsis et al. Citation2021), effective forecasting (Badakhshan et al. Citation2020), and consumer demand. The SC organizations will greatly benefit from the AI and other emerging technological advancements such as blockchain technology, additive manufacturing, and big data analytics (Olan et al. Citation2021).
Owing to the high amounts of data generation in production, operations, and supply chains, AI promises to drive the SC digitalization capabilities by delivering predictive insights which enhance organizational competitiveness (Dubey et al. Citation2020). Successful AI integration in the SC depends on the cultural SC enablers (such as processes, autonomy, information sharing) which help in improving SC performance (Cadden et al. Citation2021; Helo and Hao Citation2021). Overall, AI enabled SCs can create value and drastically improve organizational performance and competitiveness in this digital age.
The next sub-sections will discuss about the research and managerial implications followed by future research directions, and conclusion.
5. Implications, future research directions and conclusion
5.1. Research implications
The following sub-sections highlight the study implications.
5.1.1 Theoretical implications of this study
This paper makes a number of theoretical contribution to the existing body of SCM literature. First, it expands the knowledge bases of existing AI-SCM literature by reviewing and synthesising hundreds of AI-SCM articles published in the latest two decades and summarising notable trends that point specific research directions. These directions will boost research interest in the AI-SCM field. Second, unlike past literature survey articles that simply categorised past research efforts and developed taxonomies, this paper goes beyond the traditional literature survey approach by using science mapping techniques. Thirdly, it identifies research clusters that will help academicians better understand the dynamic relationship among past AI-SCM studies and visualise topical connections and level of collaboration among researchers. Fourthly, it provides substantial support to the argument on emerging technologies, operational performance, and competitiveness of organizations by highlighting various AI in SCM application areas. Coincidentally, the review highlights the importance of AI as an emerging technology in IT capability building and creating sustainable competitive advantage (Bhatt and Grover Citation2005). AI capabilities will strengthen the sensing and seizing capabilities which further enhance SC competitive advantage (Teece Citation2007; Pournader et al. Citation2021).
5.1.2 Practical implications of this study
This paper not only gave a birds’ eye view of AI-SCM research outcomes, but also illustrated how AI has been applied to a number of actual SC problems successfully. Practitioners can learn from the past AI-SCM applications and develop their own best AI-SCM practices based on the summary of past AI-SCM application efforts. In particular, this paper identified the most popular SC application areas of AI and pinpointed the most fruitful (or rewarding) application areas of AI-SCM such as SC network design, supplier selection, demand planning, and inventory planning where AI played a significant role in boosting SC productivity. In novel areas of research such as supply chain financing, AI techniques will help practitioners by enabling focal industries and other value chain players to gain analytical advantage and thereby improve the decision-making processes (Olan et al. Citation2021). The AI tools can accurately forecast the consumer demands with minimal errors and thereby help in proactively reducing the SC risks and disruptions (Baryannis et al. Citation2019b). Adding further, practitioners can make use of AI enabled teleworking activities to improvise human resources in their organizations. As AI can be integrated with other emerging technologies, it can be used for improving the real-time production system performance and eliminate waste thereby paving the way for sustainable production systems. On the behavioural operations front, the real-time information sharing enabled by AI systems can improve SC relationships, SC integration, and coordination among the stakeholders (Helo and Hao Citation2021). Therefore, the practitioners should focus on the key cultural enablers for successful AI integration in the supply chains (Cadden et al. Citation2021). In production and operations management areas, AI techniques can be seen as an advantage on the socio-technical front. AI implementation will not only minimise errors and reduce setup times but will improve workers safety by improvising the human–robot interaction.
To summarise, this study finding can help practitioners make wise AI investments in the specific SC functions with the highest return-on-investment of emerging AI tools. In addition, this paper identified and proposed specific AI tools (e.g. artificial neural network, agent-based systems, MCDM-machine learning, meta-heuristics) that were most relevant to SC applications.
5.2. Future research directions
Despite the relative youth of supply chain disciplines, SCM fields have emerged as one of the most popular research themes due to their breadth of coverage encompassing any business activities of sourcing, making, delivering, and selling. As the SCM fields continue to mature, SCM research paradigm has shifted from asset management to knowledge management due to the importance of information exchange among various supply chain partners to SCM success (Min Citation2016). This paradigm shift sparked a widespread interest in the application of AI tools to SCM. Though still limited, AI applications to SCM begun to grow rapidly for the last decade (see Table 9). However, our current study indicates that AI has not been fully exploited to solve some challenging SC problems that are too complex or too ‘ill-structured’ (loosely defined) to solve. Indeed, our study has discovered a pattern that most AI applications in the SCM area remain confined to relatively well structured (or pre-defined or narrowly defined), tactical and operational SC problems such as SC network design, inventory planning, demand planning (esp. forecasting), and supplier selection problems. That is to say, a vast majority of existing AI-SCM studies proposed AI tools mainly as the better alternatives to conventional business analytics tools or operations research/statistics techniques for tackling SC problems. This may explain why well-defined, well-known supplier selection or inventory planning problems turned out to be the most studied AI-SCM subjects and continued to garner huge interest as evidenced by the explosive growth of publications focusing on those two subjects. The relative youth of the SCM discipline may have contributed to a lack of well-defined SC problems. shows a synthesis framework consolidating the drivers of AI in SCM which emphasises the growing need of using AI to address the SC problems. It also indicates the application of AI tools being implemented to build capabilities and realising the potential of AI in improved SC performance.
Although this pattern has not been fully reversed at this moment, some recent AI studies such as Choy, Fan, and Lo (Citation2003) and Mazumdar and Mishra (Citation2010) have shown the great potential of AI tools (especially agent-based systems and data mining techniques) for addressing a variety of soft but strategic issues involving customer relationship management (CRM), supplier relationships, consumer behaviours, social marketing, and contract negotiation that have often been overlooked by more traditional analytical models. Another finding is that the genetic algorithm has emerged as one of the most dominant AI tools for tackling various aspects of well-defined SC problems, while the popularity of other promising AI tools such as ANN have not grown significantly as compared to GA and MCDM techniques (see ). Another noticeable trend that we observed is the combined use of multiple AI tools such as the hybrid MCDM and genetic algorithm and the hybrid MCDM and fuzzy set theory. Once the AI tools began to advance faster and a variety of new AI tools can be invented, we expect that the trend of integrating multiple AI tools would continue. Despite the increased popularity of AI tools for solving SCM problems, we should be aware of some challenges of using AI. These inherent challenges of AI applications to SCM include:
The effectiveness of AI tools relies heavily on the effectiveness and accuracy of AI computer software. Thus, the indiscreet use of AI tools for SCM may lead to wrong SC decisions, if it is programmed incorrectly.
AI solutions may not be easy to implement because they are so esoteric and relatively new. Thus, it may be hard for decision-makers with limited technical skills to comprehend and exploit.
Despite these challenges, as AI-SCM will continue to draw more attention from both practitioners and academicians alike. As the AI-SCM research has begun to take off as a subfield of main-stream supply chain studies, we should not lose sight of major drivers of AI-SCM: the incorporation of Industry 4.0 principles (e.g. man-machine interfaces) into value chains, links among sourcing, making, and delivering activities, and information/communication networks coordinating and synchronising those activities.
5.3. Conclusion and limitations
Despite the numerous contributions of this paper, it is subject to a number of limitations that can be addressed by future research. The bibliometric data that we collected are primarily confined to the database of scholarly journals in business disciplines. Thus, this paper cannot capture some past studies published in non-business journals mainly covering health-care, geography, and social science fields. Considering current research limitations, the continued investigation of the aforementioned AI-SCM drivers is worth pursuing as the promising future research agenda since it would help the AI-SCM research field mature and refresh. In particular, we would like to suggest the following lines of research for the subjects of further scientific inquiries.
As the world gets closer with improved transportation means, infectious diseases can spread out more quickly and widely all across the world (Min Citation2014). Especially, developing countries with a rapid population growth but limited medical resources are highly susceptible to deadly disease outbreaks. Although some ground works have been established by a few pioneering researchers, more AI-SCM research from a health care perspective is needed to tackle public health care issues associated with infectious disease outbreaks such as the COVID-19 pandemic. The example of such research includes tracing of disease hot-spots and adequate, timely distribution of medical equipment and supplies to the hospitals.
The diverse applications of AI can be seen along vertical sectors such as food processing and manufacturing industry where AI can be used for investigating the food safety, quality parameters, and product recalls.
The AI/ ML techniques can be used for exploring legal aspects and research applications in fintech and banking operations (such as credit card frauds, loan defaults, etc.).
AI applications can be explored in marketing operations wherein AI based robots (chatbots) can help in providing creative customer engagement.
AI applications can have their potential impact on sustainable operations for new startups.
Despite the enormous benefits and potentials of AI, factory automation powered by AI may increase anxiety among workers for their job security and then face strong organizational resistance. Since such resistance can derail AI success in the SC operations, empirical studies that can assess the extent of organizational resistance against AI-SCM would be needed.
Disclosure statement
No potential conflict of interest was reported by the author(s).
Data availability statement
Data will be made available upon request.
Additional information
Notes on contributors
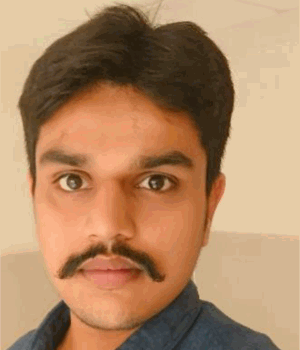
Rohit Sharma
Dr. Rohit Sharma is presently working as an Assistant Professor in the Decision Sciences area at the Jaipuria Institute of Management, Noida. His main research areas include operations and supply chain management, sustainable operations management, agribusiness management and logistics management. He holds a Ph.D. in Operations and Supply Chain Management from the National Institute of Industrial Engineering, Mumbai. His publications have appeared in leading journals such as Computers and Electronics in Agriculture, International Journal of Information Management, Computers in Industry, Computers and Operations Research, Technological Forecasting and Social Change, Journal of Enterprise Information Management, the International Journal of Logistics Management, and International Journal of Logistics: Research and Applications. He was awarded the ‘Industry 4.0 Award’ by the IEOM Society in 2021 and the Tata-Cornell Ag Initiative Scholarship at the second International Global Food Security Conference in 2015.
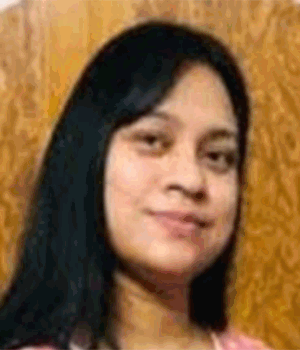
Anjali Shishodia
Dr. Anjali Shishodia is presently working as an Assistant Professor at LMTSM, Patiala, Punjab. She has completed her Doctoral programme in operations management from National Institute of Industrial Engineering (NITIE), Mumbai. She holds a master’s degree in Industrial Engineering from Visvesvaraya National Institute of Technology and bachelor’s degree in Mechanical Engineering from Dr. A.P.J. Abdul Kalam Technical University. She is a recipient of academic excellence award. Her teaching and research interests include production and operations management, quantitative techniques, project management and supply chain management. Her work has been published in leading journals such as Production Planning and Control, Computers and Industrial Engineering, Journal of Enterprise Information Management, International Journal of Logistics: Research and Applications, and the International Journal of Logistics Management.
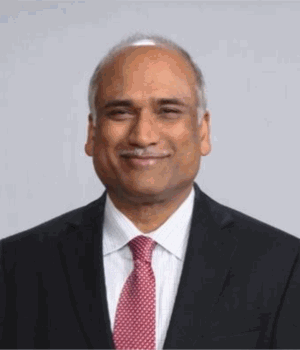
Angappa Gunasekaran
Dr. Angappa Gunasekaran is presently the director of the School of Business Administration, Penn State Harrisburg. Prior to joining Penn State, Dr. Gunasekaran served as a special assistant to the provost for Academic Affairs and Student Success at California State University in Bakersfield, California, where he also has served as dean of the School of Business and Public Administration, and as the principal investigator for the Small Business Development Center in Bakersfield. He also is professor of operations management. Previously, he served as dean of the Charlton College of Business, chairperson of the Department of Decision and Information Sciences, and the founding director of Business Innovation Research Center at the University of Massachusetts Dartmouth. He has more than 10 years of global work experience. Dr. Gunasekaran has published more than 400 peer reviewed journal articles with over 60,000 plus citations. He has served on the editorial board of over 30 peer-reviewed journals and has organised several international workshops and conferences in the emerging areas of operations management and information systems.
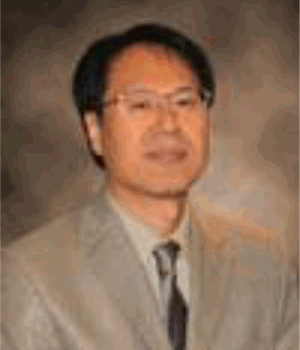
Hokey Min
Dr. Hokey Min is James R. Good Chair in Global Supply Chain Strategy in the College of Business Administration at the Bowling Green State University. Prior to joining the Bowling Green State University, he was the Distinguished University Scholar and Founding Director of the Logistics and Distribution Institute (LoDI), the UPS Center for World-wide Supply Chain Management and the Center for Supply Chain Workforce Development at the University of Louisville. He earned his Ph.D. degree in Management Sciences and Logistics from the Ohio State University. His research interests include global logistics strategy, health-care supply chains, e-synchronised supply chains, business intelligence, and supply chain modelling. He has published more than 175 articles in various refereed journals including European Journal of Operational Research, Journal of Business Logistics, Journal of the Operational Research Society, International Journal of Production Research, Transportation Journal, and Transportation Research. He is an editor of International Journal of Logistics: Research and Applications and is currently serving on the editorial review board of Journal of Business Logistics, International Journal of Logistics Systems and Management, and International Journal of Integrated Supply Management. He also got involved in various outreach or consulting projects for more than 50 organizations including UPS Air, UPS Supply Chain Solutions, Exel Logistics, and the Track Chair of Warehousing Education and Research Council (WERC) to name a few.
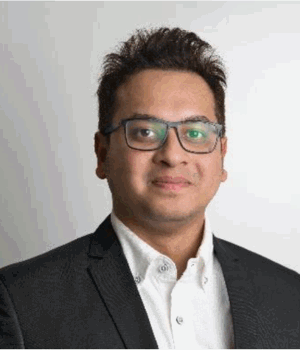
Ziaul Haque Munim
Dr Ziaul Haque Munim is presently an Associate Professor of Maritime Logistics at the Faculty of Technology, Natural and Maritime Sciences at the University of South-Eastern Norway. His main research interests include supply chain management, maritime logistics, machine learning and research methods. He holds a PhD degree in International Management from the University of Agder and MSc degree in Supply Chain Management from the Vienna University of Economics and Business. His publications have appeared in leading journals such as the Journal of Business Research, Transportation Science, Resources, Conservation & Recycling, Journal of Cleaner Production, and others. He received the Palgrave Macmillan Best Paper Award at the IAME 2016 conference, the KLU Young Researcher Best Paper Award at the IAME 2018 conference, and the MPM Best Journal Paper Award 2020.
Notes
1 The Scopus database encompass research articles from reputable publishers, such as Emerald, Elsevier, IEEE, Indersciences, Springer, Taylor and Francis, and Wiley (Mongeon and Paul-Hus Citation2016).
References
- Abbassi, A., S. Kharraja, A. El Hilali Alaoui, J. Boukachour, and D. Paras. 2020. “Multi-objective Two-Echelon Location-Distribution of Non-medical Products.” International Journal of Production Research. doi:10.1080/00207543.2020.1777479.
- Aburto, L., and R. Weber. 2007. “Improved Supply Chain Management Based on Hybrid Demand Forecasts.” Applied Soft Computing 7 (1): 136–144.
- Adadi, A., and M. Berrada. 2018. “Peeking Inside the Black-Box: A Survey on Explainable Artificial Intelligence (XAI).” IEEE Access 6: 52138–52160.
- Al Chami, Z., H. Manier, M. A. Manier, and C. Fitouri. 2017. “A Hybrid Genetic Algorithm to Solve a Multi-Objective Pickup and Delivery Problem.” IFAC-PapersOnLine 50 (1): 14656–14661.
- Aliev, R. A., B. Fazlollahi, B. G. Guirimov, and R. R. Aliev. 2007. “Fuzzy-genetic Approach to Aggregate Production-Distribution Planning in Supply Chain Management.” Information Sciences 177 (20): 4241–4255.
- Altiparmak, F., M. Gen, L. Lin, and T. Paksoy. 2006. “A Genetic Algorithm Approach for Multi-Objective Optimization of Supply Chain Networks.” Computers & Industrial Engineering 51 (1): 196–215.
- Amid, A., S. H. Ghodsypour, and C. O’Brien. 2006. “Fuzzy Multiobjective Linear Model for Supplier Selection in a Supply Chain.” International Journal of Production Economics 104 (2): 394–407.
- Aria, M., and C. Cuccurullo. 2017. “Bibliometrix: An R-tool for Comprehensive Science Mapping Analysis.” Journal of Informetrics 11 (4): 959–975.
- Aswani, A., Z. J. M. Shen, and A. Siddiq. 2019. “Data-driven Incentive Design in the Medicare Shared Savings Program.” Operations Research 67 (4): 1002–1026.
- Awasthi, A., and G. Kannan. 2016. “Green Supplier Development Program Selection Using NGT and VIKOR Under Fuzzy Environment.” Computers & Industrial Engineering 91: 100–108.
- Badakhshan, E., P. Humphreys, L. Maguire, and R. McIvor. 2020. “Using Simulation-Based System Dynamics and Genetic Algorithms to Reduce the Cash Flow Bullwhip in the Supply Chain.” International Journal of Production Research 58 (17): 5253–5279.
- Barari, S., G. Agarwal, W. C. Zhang, B. Mahanty, and M. K. Tiwari. 2012. “A Decision Framework for the Analysis of Green Supply Chain Contracts: An Evolutionary Game Approach.” Expert Systems with Applications 39 (3): 2965–2976.
- Baryannis, G., S. Dani, and G. Antoniou. 2019a. “Predicting Supply Chain Risks Using Machine Learning: The Trade-off Between Performance and Interpretability.” Future Generation Computer Systems 101: 993–1004.
- Baryannis, G., S. Validi, S. Dani, and G. Antoniou. 2019b. “Supply Chain Risk Management and Artificial Intelligence: State of the art and Future Research Directions.” International Journal of Production Research 57 (7): 2179–2202.
- Bawack, R. E., S. Fosso Wamba, and K. Carillo. 2019. “Artificial Intelligence in Practice: Implications for IS Research.” https://aisel.aisnet.org/amcis2019/ai_semantic_for_intelligent_info_systems/ai_semantic_for_intelligent_info_systems/2/.
- Baykasoğlu, A., and İ Gölcük. 2019. “A Dynamic Multiple Attribute Decision Making Model with Learning of Fuzzy Cognitive Maps.” Computers & Industrial Engineering 135: 1063–1076.
- Bechtsis, D., N. Tsolakis, E. Iakovou, and D. Vlachos. 2021. “Data-driven Secure, Resilient and Sustainable Supply Chains: Gaps, Opportunities, and a New Generalised Data Sharing and Data Monetisation Framework.” International Journal of Production Research, 1–21. doi:10.1080/00207543.2021.1957506.
- Belhadi, A., S. Kamble, S. Fosso Wamba, and M. M. Queiroz. 2021. “Building Supply-Chain Resilience: An Artificial Intelligence-Based Technique and Decision-Making Framework.” International Journal of Production Research, 1–21. doi:10.1080/00207543.2021.1950935.
- Ben-Daya, M., E. Hassini, and Z. Bahroun. 2019. “Internet of Things and Supply Chain Management: A Literature Review.” International Journal of Production Research 57 (15–16): 4719–4742.
- Bhatt, G. D., and V. Grover. 2005. “Types of Information Technology Capabilities and Their Role in Competitive Advantage: An Empirical Study.” Journal of Management Information Systems 22 (2): 253–277.
- Biesinger, B., B. Hu, and G. R. Raidl. 2018. “A Genetic Algorithm in Combination with a Solution Archive for Solving the Generalized Vehicle Routing Problem with Stochastic Demands.” Transportation Science 52 (3): 673–690.
- Boran, F. E., S. Genç, M. Kurt, and D. Akay. 2009. “A Multi-Criteria Intuitionistic Fuzzy Group Decision Making for Supplier Selection with TOPSIS Method.” Expert Systems with Applications 36 (8): 11363–11368.
- Brynjolfsson, E., and A. Mcafee. 2017. “The Business of Artificial Intelligence.” Harvard Business Review, 1–20. https://hbr.org/2017/07/the-business-of-artificial-intelligence.
- Cadden, T., D. Dennehy, M. Mantymaki, and R. Treacy. 2021. “Understanding the Influential and Mediating Role of Cultural Enablers of AI Integration to Supply Chain.” International Journal of Production Research, 1–29. doi:10.1080/00207543.2021.1946614.
- Carbonneau, R., K. Laframboise, and R. Vahidov. 2008. “Application of Machine Learning Techniques for Supply Chain Demand Forecasting.” European Journal of Operational Research 184 (3): 1140–1154.
- Carrera, D. A., R. V. Mayorga, and W. Peng. 2020. “A Soft Computing Approach for Group Decision Making: A Supply Chain Management Application.” Applied Soft Computing 91. doi:10.1016/j.asoc.2020.
- Castillo, O., L. Amador-Angulo, J. R. Castro, and M. Garcia-Valdez. 2016. “A Comparative Study of Type-1 Fuzzy Logic Systems, Interval Type-2 Fuzzy Logic Systems and Generalized Type-2 Fuzzy Logic Systems in Control Problems.” Information Sciences 354: 257–274.
- Chakraborty, S., S. A. Hoque, and S. F. Kabir. 2020. “Predicting Fashion Trend Using Runway Images: Application of Logistic Regression in Trend Forecasting.” International Journal of Fashion Design, Technology and Education 13 (3): 376–386.
- Chan, F. T., N. Kumar, M. K. Tiwari, H. C. Lau, and K. Choy. 2008. “Global Supplier Selection: A Fuzzy-AHP Approach.” International Journal of Production Research 46 (14): 3825–3857.
- Chaturvedi, I., R. Satapathy, S. Cavallari, and E. Cambria. 2019. “Fuzzy Commonsense Reasoning for Multimodal Sentiment Analysis.” Pattern Recognition Letters 125: 264–270.
- Chen, C. T., C. T. Lin, and S. F. Huang. 2006. “A Fuzzy Approach for Supplier Evaluation and Selection in Supply Chain Management.” International Journal of Production Economics 102 (2): 289–301.
- Chou, S. Y., Y. H. Chang, and C. Y. Shen. 2008. “A Fuzzy Simple Additive Weighting System Under Group Decision-Making for Facility Location Selection with Objective/Subjective Attributes.” European Journal of Operational Research 189 (1): 132–145.
- Choy, K. L., K. K. Fan, and V. Lo. 2003. “Development of an Intelligent Customer-Supplier Relationship Management System: The Application of Case-Based Reasoning.” Industrial Management & Data Systems 103 (4): 263–274.
- Contreras-Bolton, C., G. Gatica, C. R. Barra, and V. Parada. 2016a. “A Multi-Operator Genetic Algorithm for the Generalized Minimum Spanning Tree Problem.” Expert Systems with Applications 50: 1–8.
- Contreras-Bolton, C., C. Rey, S. Ramos-Cossio, C. Rodríguez, F. Gatica, and V. Parada. 2016b. “Automatically Produced Algorithms for the Generalized Minimum Spanning Tree Problem.” Scientific Programming 2016: 1–11.
- Dehghani, M., B. Abbasi, and F. Oliveira. 2021. “Proactive Transshipment in the Blood Supply Chain: A Stochastic Programming Approach.” Omega 98. doi:10.1016/j.omega.2019.102112.
- Disney, S. M., M. M. Naim, and D. R. Towill. 2000. “Genetic Algorithm Optimisation of a Class of Inventory Control Systems.” International Journal of Production Economics 68 (3): 259–278.
- Dobler, D. W., L. Lee Jr, and D. N. Burt. 1990. Purchasing and Materials Management: Text and Cases. 5th ed. New York: McGraw-Hill.
- Dolgui, A., and D. Ivanov. 2021. “5G in Digital Supply Chain and Operations Management: Fostering Flexibility, End-to-End Connectivity and Real-Time Visibility Through Internet-of-Everything.” International Journal of Production Research, 1–10. doi:10.1080/00207543.2021.2002969.
- Dora, M., A. Kumar, S. K. Mangla, A. Pant, and M. M. Kamal. 2021. “Critical Success Factors Influencing Artificial Intelligence Adoption in Food Supply Chains.” International Journal of Production Research, 1–20. doi:10.1080/00207543.2021.1959665.
- dos Santos, B.M., Godoy, L.P. and Campos, L.M. 2019. “Performance Evaluation of Green Suppliers Using Entropy-TOPSIS-F.” Journal of Cleaner Production 207: 498–509.
- Doumpos, M., and E. Grigoroudis. 2013. Multicriteria Decision Aid and Artificial Intelligence: Links, Theory and Applications. Sussex: Wiley.
- Dubey, R., A. Gunasekaran, S. J. Childe, D. J. Bryde, M. Giannakis, C. Foropon, D. Roubaud, and B. T. Hazen. 2020. “Big Data Analytics and Artificial Intelligence Pathway to Operational Performance Under the Effects of Entrepreneurial Orientation and Environmental Dynamism: A Study of Manufacturing Organisations.” International Journal of Production Economics 226: 107599. doi:10.1016/j.ijpe.2019.107599.
- Duriau, V. J., R. K. Reger, and M. D. Pfarrer. 2007. “A Content Analysis of the Content Analysis Literature in Organization Studies: Research Themes, Data Sources, and Methodological Refinements.” Organizational Research Methods 10 (1): 5–34.
- Efendigil, T., S. Önüt, and C. Kahraman. 2009. “A Decision Support System for Demand Forecasting with Artificial Neural Networks and Neuro-Fuzzy Models: A Comparative Analysis.” Expert Systems with Applications 36 (3): 6697–6707.
- Fahimnia, B., J. Sarkis, and H. Davarzani. 2015. “Green Supply Chain Management: A Review and Bibliometric Analysis.” International Journal of Production Economics 162: 101–114.
- Fan, W., J. Liu, S. Zhu, and P. M. Pardalos. 2018. “Investigating the Impacting Factors for the Healthcare Professionals to Adopt Artificial Intelligence-Based Medical Diagnosis Support System (AIMDSS).” Annals of Operations Research, 1–26. DOI:10.1007/s10479-018-2818-y.
- Feng, Y., Q. Zhu, and K. H. Lai. 2017. “Corporate Social Responsibility for Supply Chain Management: A Literature Review and Bibliometric Analysis.” Journal of Cleaner Production 158: 296–307.
- Gartner. 2017. “Top 10 Strategic Technology Trends for 2018.” Accessed April 16 2020. [Online]. https://www.gartner.com/ doc/3811368?srcId = 1-6595640781.
- Geraldi, J., H. Maylor, and T. Williams. 2011. “Now, Let’s Make it Really Complex (Complicated) A Systematic Review of the Complexities of Projects.” International Journal of Operations and Production Management 31 (9): 966–990.
- Giannoccaro, I., P. Pontrandolfo, and B. Scozzi. 2003. “A Fuzzy Echelon Approach for Inventory Management in Supply Chains.” European Journal of Operational Research 149 (1): 185–196.
- Goularte, F. B., S. M. Nassar, R. Fileto, and H. Saggion. 2019. “A Text Summarization Method Based on Fuzzy Rules and Applicable to Automated Assessment.” Expert Systems with Applications 115: 264–275.
- Govindan, K., A. Jafarian, R. Khodaverdi, and K. Devika. 2014. “Two-Echelon Multiple-Vehicle Location–Routing Problem with Time Windows for Optimization of Sustainable Supply Chain Network of Perishable Food.” International Journal of Production Economics 152: 9–28.
- Govindan, K., R. Khodaverdi, and A. Jafarian. 2013. “A Fuzzy Multi Criteria Approach for Measuring Sustainability Performance of a Supplier Based on Triple Bottom Line Approach.” Journal of Cleaner production 47: 345–354.
- Govindan, K., R. Khodaverdi, and A. Vafadarnikjoo. 2015a. “Intuitionistic Fuzzy Based DEMATEL Method for Developing Green Practices and Performances in a Green Supply Chain.” Expert Systems with Applications 42 (20): 7207–7220.
- Govindan, K., H. Soleimani, and D. Kannan. 2015b. “Reverse Logistics and Closed-Loop Supply Chain: A Comprehensive Review to Explore the Future.” European Journal of Operational Research 240 (3): 603–626.
- Gu, W., C. Wang, S. Dai, L. Wei, and I. R. Chiang. 2019. “Optimal Strategies for Reverse Logistics Network Construction: A Multi-Criteria Decision Method for Chinese Iron and Steel Industry.” Resources Policy, doi:10.1016/j.resourpol.2019.02.008. (in press)
- Hamamoto, A. H., L. F. Carvalho, L. D. H. Sampaio, T. Abrão, and M. L. Proença Jr. 2018. “Network Anomaly Detection System Using Genetic Algorithm and Fuzzy Logic.” Expert Systems with Applications 92: 390–402.
- He, Q. 1999. “Knowledge Discovery Through Co-word Analysis.” https://www.ideals.illinois.edu/bitstream/handle/2142/8267/?sequence=1.
- Helo, P., and Y. Hao. 2021. “Artificial Intelligence in Operations Management and Supply Chain Management: An Exploratory Case Study.” Production Planning & Control, 1–18. doi:10.1080/09537287.2021.1882690.
- IDC, International Data Corporation. 2018. “Worldwide Semiannual Cognitive Artificial Intelligence Systems Spending Guide.” Accessed April 15, 2020. [Online]. https://www.idc.com/getdoc.jsp? containerId = prUS43662418.
- Islam, S., and S. H. Amin. 2020. “Prediction of Probable Backorder Scenarios in the Supply Chain Using Distributed Random Forest and Gradient Boosting Machine Learning Techniques.” Journal of Big Data 7 (1): 1–22.
- Jain, N., and A. R. Singh. 2020. “Sustainable Supplier Selection Under Must-be Criteria Through Fuzzy Inference System.” Journal of Cleaner Production 248: 119–275.
- Jia, T., Z. Jing, and M. Hong. 2019, October. “A Genetic Algorithm for the Two-Echelon Vehicle Routing Problem with Simultaneous Pickup and Delivery.” In 2019 IEEE 1st International Conference on Civil Aviation Safety and Information Technology (ICCASIT) (pp. 283–287). IEEE.
- Kamble, S. S., and A. Gunasekaran. 2020. “Big Data-Driven Supply Chain Performance Measurement System: A Review and Framework for Implementation.” International Journal of Production Research 58 (1): 65–86.
- Kannan, D., A. B. L. de Sousa Jabbour, and C. J. C. Jabbour. 2014. “Selecting Green Suppliers Based on GSCM Practices: Using Fuzzy TOPSIS Applied to a Brazilian Electronics Company.” European Journal of Operational Research 233 (2): 432–447.
- Kannan, G., P. Sasikumar, and K. Devika. 2010. “A Genetic Algorithm Approach for Solving a Closed Loop Supply Chain Model: A Case of Battery Recycling.” Applied Mathematical Modelling 34 (3): 655–670.
- Kargar, S., M. M. Paydar, and A. S. Safaei. 2020. “A Reverse Supply Chain for Medical Waste: A Case Study in Babol Healthcare Sector.” Waste Management 113: 197–209.
- Khan, S. A., A. Chaabane, and F. Dweiri. 2019. “A Knowledge-Based System for Overall Supply Chain Performance Evaluation: A Multi-Criteria Decision Making Approach.” Supply Chain Management: An International Journal 24 (3): 377–396.
- Ko, H. J., and G. W. Evans. 2007. “A Genetic Algorithm-Based Heuristic for the Dynamic Integrated Forward/Reverse Logistics Network for 3PLs.” Computers & Operations Research 34 (2): 346–366.
- Kohtamäki, M., V. Parida, P. Oghazi, H. Gebauer, and T. Baines. 2019. “Digital Servitization Business Models in Ecosystems: A Theory of the Firm.” Journal of Business Research 104: 380–392.
- Kools, S., M. McCarthy, R. Durham, and L. Robrecht. 1996. “Dimensional Analysis: Broadening the Conception of Grounded Theory.” Qualitative Health Research 6 (3): 312–330.
- Kulak, O., and C. Kahraman. 2005. “Multi-attribute Comparison of Advanced Manufacturing Systems using Fuzzy vs. Crisp Axiomatic Design Approach.” International Journal of Production Economics 95 (3): 415–424.
- Kumar, M., P. Vrat, and R. Shankar. 2004. “A Fuzzy Goal Programming Approach for Vendor Selection Problem in a Supply Chain.” Computers & Industrial Engineering 46 (1): 69–85.
- Kuo, R. J., Y. C. Wang, and F. C. Tien. 2010. “Integration of Artificial Neural Network and MADA Methods for Green Supplier Selection.” Journal of Cleaner Production 18 (12): 1161–1170.
- Lamba, K., and S. P. Singh. 2017. “Big Data in Operations and Supply Chain Management: Current Trends and Future Perspectives.” Production Planning & Control 28 (11-12): 877–890.
- Lee, J., M. Azamfar, and J. Singh. 2019. “A Blockchain Enabled Cyber-Physical System Architecture for Industry 4.0 Manufacturing Systems.” Manufacturing Letters 20: 34–39.
- Lee, J., B. Bagheri, and H. A. Kao. 2015. “A Cyber-Physical Systems Architecture for Industry 4.0-Based Manufacturing Systems.” Manufacturing Letters 3: 18–23.
- Lee, Y. H., M. K. Cho, S. J. Kim, and Y. B. Kim. 2002. “Supply Chain Simulation with Discrete-Continuous Combined Modeling.” Computers & Industrial Engineering 43 (1–2): 375–392.
- Lee, J., H. Davari, J. Singh, and V. Pandhare. 2018. “Industrial Artificial Intelligence for Industry 4.0-Based Manufacturing Systems.” Manufacturing Letters 18: 20–23.
- Liang, T. F. 2008. “Fuzzy Multi-Objective Production/Distribution Planning Decisions with Multi-Product and Multi-Time Period in a Supply Chain.” Computers & Industrial Engineering 55 (3): 676–694.
- Liang, W., and C. Huang. 2006. “Agent-based Demand Forecast in Multi-Echelon Supply Chain.” Decision Support Systems 42 (1): 390–407.
- Lieckens, K., and N. Vandaele. 2007. “Reverse Logistics Network Design with Stochastic Lead Times.” Computers & Operations Research 34 (2): 395–416.
- Lima-Junior, F. R., and L. C. R. Carpinetti. 2019. “Predicting Supply Chain Performance Based on SCOR® Metrics and Multilayer Perceptron Neural Networks.” International Journal of Production Economics 212: 19–38.
- Lin, R. J. 2013. “Using Fuzzy DEMATEL to Evaluate the Green Supply Chain Management Practices.” Journal of Cleaner Production 40: 32–39.
- Lin, C. T., H. Chiu, and P. Y. Chu. 2006. “Agility Index in the Supply Chain.” International Journal of Production Economics 100 (2): 285–299.
- Liu, Y., H. Li, J. Guan, X. Liu, Q. Guan, and Q. Sun. 2019. “Influence of Different Factors on Prices of Upstream, Middle and Downstream Products in China's Whole Steel Industry Chain: Based on Adaptive Neural Fuzzy Inference System.” Resources Policy 60: 134–142.
- Liu, R., B. Yang, E. Zio, and X. Chen. 2018. “Artificial Intelligence for Fault Diagnosis of Rotating Machinery: A Review.” Mechanical Systems and Signal Processing 108: 33–47.
- Maditati, D. R., Z. H. Munim, H. J. Schramm, and S. Kummer. 2018. “A Review of Green Supply Chain Management: From Bibliometric Analysis to a Conceptual Framework and Future Research Directions.” Resources, Conservation and Recycling 139: 150–162.
- Makridakis, S. 2017. “The Forthcoming Artificial Intelligence (AI) Revolution: Its Impact on Society and Firms.” Futures 90: 46–60.
- Mathur, B., S. Gupta, M. L. Meena, and G. S. Dangayach. 2018. “Healthcare Supply Chain Management: Literature Review and Some Issues.” Journal of Advances in Management Research 15 (3): 265–287.
- Mayring, P. 2003. “Qualitative Inhaltanalyse Grundlagenund Techniken (Qualitative Content Analysis).” Beltz.
- Mazumdar, B. D., and R. B. Mishra. 2010. “Multi-agent Negotiation in B2C e-Commerce Based on Data Mining Methods.” International Journal of Intelligent Information Technologies 6 (4): 46–70.
- Min, H. 2010. “Artificial Intelligence in Supply Chain Management: Theory and Applications.” International Journal of Logistics: Research and Applications 13 (1): 13–39.
- Min, H. 2014. Healthcare Supply Chain Management: Basic Concepts and Principles. New York, NY: Business Expert Press.
- Min, H. 2015a. “Genetic Algorithm for Supply Chain Modelling: Basic Concepts and Applications.” International Journal of Services and Operations Management 22 (2): 143–164.
- Min, H. 2015b. The Essentials of Supply Chain Management: New Business Concepts and Applications. Saddle River, NJ: Pearson Education.
- Min, H. 2016. “Emerging Trends of Supply Chain Management: Where are we Going?” Korean Journal of Logistics 24 (1): 1–9.
- Min, H. 2017. “Health Care Supply Chain Research: Where Are we Going?” Logistics Research 10 (5): 1–19.
- Min, H., and I. Kim. 2012. “Green Supply Chain Research: Past, Present, and Future.” Logistics Research 4 (1-2): 39–47.
- Min, H., H. K. Ko, and C. S. Ko. 2006a. “A Genetic Algorithm Approach to Developing the Multi-Echelon Reverse Logistics Network for Product Returns.” Omega 34 (1): 56–59.
- Min, H., C. S. Ko, and H. K. Ko. 2006b. “The Spatial and Temporal Consolidation of Returned Products in a Closed-Loop Supply Chain Network.” Computers and Industrial Engineering 51 (2): 309–320.
- Mirhedayatian, S. M., M. Azadi, and R. F. Saen. 2014. “A Novel Network Data Envelopment Analysis Model for Evaluating Green Supply Chain Management.” International Journal of Production Economics 147: 544–554.
- Moher, D., A. Liberati, J. Tetzlaff, D. G. Altman and Prisma Group. 2009. “Preferred Reporting Items for Systematic Reviews and Meta-Analyses: The PRISMA Statement.” PLoS Medicine 6 (7): e1000097.
- Mongeon, P., and A. Paul-Hus. 2016. “The Journal Coverage of Web of Science and Scopus: A Comparative Analysis.” Scientometrics 106 (1): 213–228.
- Nezamoddini, N., A. Gholami, and F. Aqlan. 2020. “A Risk-Based Optimization Framework for Integrated Supply Chains Using Genetic Algorithm and Artificial Neural Networks.” International Journal of Production Economics 225. doi:10.1016/j.ijpe.2019.107569.
- Nguyen, H. D., K. P. Tran, S. Thomassey, and M. Hamad. 2021. “Forecasting and Anomaly Detection Approaches Using LSTM and LSTM Autoencoder Techniques with the Applications in Supply Chain Management.” International Journal of Information Management 57: 102282.
- Okabe, T., and Y. Otsuka. 2021. “Proposal of a Validation Method of Failure Mode Analyses Based on the Stress-Strength Model with a Support Vector Machine.” Reliability Engineering & System Safety 205: 107247.
- Olan, F., S. Liu, J. Suklan, U. Jayawickrama, and E. O. Arakpogun. 2021. “The Role of Artificial Intelligence Networks in Sustainable Supply Chain Finance for Food and Drink Industry.” International Journal of Production Research, 1–16. doi:10.1080/00207543.2021.1915510.
- Ordoobadi, S. M. 2009. “Development of a Supplier Selection Model Using Fuzzy Logic.” Supply Chain Management 14 (4): 314–327.
- Oroojlooyjadid, A., M. Nazari, L. V. Snyder, and M. Takáč. 2021. “A Deep Q-Network for the Beer Game: Deep Reinforcement Learning for Inventory Optimization.” Manufacturing & Service Operations Management. doi:10.1287/msom.2020.0939.
- Özkan, G., and M. İnal. 2014. “Comparison of Neural Network Application for Fuzzy and ANFIS Approaches for Multi-Criteria Decision Making Problems.” Applied Soft Computing 24: 232–238.
- Pal, O., A. K. Gupta, and R. K. Garg. 2013. “Supplier Selection Criteria and Methods in Supply Chains: A Review.” International Journal of Economics and Management Engineering 7 (10): 2667–2673.
- Pan, Y. 2016. “Heading Toward Artificial Intelligence 2.0.” Engineering 2 (4): 409–413.
- Pasandideh, S. H. R., S. T. A. Niaki, and A. R. Nia. 2011. “A Genetic Algorithm for Vendor Managed Inventory Control System of Multi-Product Multi-Constraint Economic Order Quantity Model.” Expert Systems with Applications 38 (3): 2708–2716.
- Patel, D., S. Shah, and H. Chhinkaniwala. 2019. “Fuzzy Logic Based Multi Document Summarization with Improved Sentence Scoring and Redundancy Removal Technique.” Expert Systems with Applications 134: 167–177.
- Peidro, D., J. Mula, M. Jiménez, and M. del Mar Botella. 2010. “A Fuzzy Linear Programming Based Approach for Tactical Supply Chain Planning in an Uncertainty Environment.” European Journal of Operational Research 205 (1): 65–80.
- Perny, P., and J. C. Pomerol. 1999. “Use of Artificial Intelligence in MCDM.” In Multicriteria Decision Making: Advances in MCDM Models, Algorithms, Theory, and Applications, edited by Thomas Gal, Theodor J. Stewart, and Thomas Hanne, 457–499. Boston, MA: Springer.
- Petering, M. E., X. Chen, and W. H. Hsieh. 2019. “Inventory Control with Flexible Demand: Cyclic Case with Multiple Batch Supply and Demand Processes.” International Journal of Production Economics 212: 60–77.
- Petrovic, D. 2001. “Simulation of Supply Chain Behaviour and Performance in an Uncertain Environment.” International Journal of Production Economics 71 (1–3): 429–438.
- Petrovic, D., R. Roy, and R. Petrovic. 1998. “Modelling and Simulation of a Supply Chain in an Uncertain Environment.” European Journal of Operational Research 109 (2): 299–309.
- Petrovic, D., R. Roy, and R. Petrovic. 1999. “Supply Chain Modelling Using Fuzzy Sets.” International Journal of Production Economics 59 (1-3): 443–453.
- Pillai, R., B. Sivathanu, M. Mariani, N. P. Rana, B. Yang, and Y. K. Dwivedi. 2021. “Adoption of AI-Empowered Industrial Robots in Auto Component Manufacturing Companies.” Production Planning & Control, 1–17. doi:10.1080/09537287.2021.1882689.
- Pishvaee, M. S., R. Z. Farahani, and W. Dullaert. 2010. “A Memetic Algorithm for Bi-objective Integrated Forward/Reverse Logistics Network Design.” Computers & Operations Research 37 (6): 1100–1112.
- Pomerol, J. C. 1997. “Artificial Intelligence and Human Decision Making.” European Journal of Operational Research 99 (1): 3–25.
- Pournader, M., H. Ghaderi, A. Hassanzadegan, and B. Fahimnia. 2021. “Artificial Intelligence Applications in Supply Chain Management.” International Journal of Production Economics 241: 108250.
- Qin, J., X. Liu, and W. Pedrycz. 2017. “An Extended TODIM Multi-Criteria Group Decision Making Method for Green Supplier Selection in Interval Type-2 Fuzzy Environment.” European Journal of Operational Research 258 (2): 626–638.
- Quariguasi Frota, N. J., G. Walther, J. A. E. E. Bloemhof, J. A. E. E. Van Nunen, and T. Spengler. 2010. “From Closed-Loop to Sustainable Supply Chains: The WEEE Case.” International Journal of Production Research 48 (15): 4463–4481.
- Ramos-Rodríguez, A. R., and J. Ruíz-Navarro. 2004. “Changes in the Intellectual Structure of Strategic Management Research: A Bibliometric Study of the Strategic Management Journal, 1980–2000.” Strategic Management Journal 25 (10): 981–1004.
- Rasmi, S. A. B., C. Kazan, and M. Türkay. 2019. “A Multi-Criteria Decision Analysis to Include Environmental, Social, and Cultural Issues in the Sustainable Aggregate Production Plans.” Computers & Industrial Engineering 132: 348–360.
- Rath, S., K. Rajaram, and A. Mahajan. 2017. “Integrated Anesthesiologist and Room Scheduling for Surgeries: Methodology and Application.” Operations Research 65 (6): 1460–1478.
- Riahi, Y., T. Saikouk, A. Gunasekaran, and I. Badraoui. 2021. “Artificial Intelligence Applications in Supply Chain: A Descriptive Bibliometric Analysis and Future Research Directions.” Expert Systems with Applications 173: 114702.
- Rodríguez-Espíndola, O., S. Chowdhury, A. Beltagui, and P. Albores. 2020. “The Potential of Emergent Disruptive Technologies for Humanitarian Supply Chains: The Integration of Blockchain, Artificial Intelligence and 3D Printing.” International Journal of Production Research 58 (15): 4610–4630.
- Rohde, J. 2004. “Hierarchical Supply Chain Planning using Artificial Neural Networks to Anticipate Base-level Outcomes.” OR Spectrum 26 (4): 471–492.
- Rüßmann, M., M. Lorenz, P. Gerbert, M. Waldner, J. Justus, P. Engel, and M. Harnisch. 2015. “Industry 4.0: The Future of Productivity and Growth in Manufacturing Industries.” Boston Consulting Group 9 (1): 54–89.
- Rüther, C., and J. Rieck. 2020, April. “A Grouping Genetic Algorithm for Multi Depot Pickup and Delivery Problems with Time Windows and Heterogeneous Vehicle Fleets.” In Evolutionary Computation in Combinatorial Optimization: 20th European Conference, EvoCOP 2020, Held as Part of EvoStar 2020, edited by L. Paquete, and C. Zarges, Seville, Spain, April 15–17, 2020, Proceedings (Vol. 12102). Springer Nature.
- Sabegh, M. H. Z., M. Mohammadi, and B. Naderi. 2017. “Multi-objective Optimization Considering Quality Concepts in a Green Healthcare Supply Chain for Natural Disaster Response: Neural Network Approaches.” International Journal of System Assurance Engineering and Management 8 (2): 1689–1703.
- Sadjadi, S. J., Z. Ziaei, and M. S. Pishvaee. 2019. “The Design of the Vaccine Supply Network Under Uncertain Condition.” Journal of Modelling in Management 14 (4): 841–871.
- Salipante, P., W. Notz, and J. Bigelow. 1982. “A Matrix Approach to Literature Reviews.” Research in Organizational Behavior 4: 321–348.
- Sanayei, A., S. F. Mousavi, and A. Yazdankhah. 2010. “Group Decision Making Process for Supplier Selection with VIKOR Under Fuzzy Environment.” Expert Systems with Applications 37 (1): 24–30.
- Sari, K. 2017. “A Novel Multi-Criteria Decision Framework for Evaluating Green Supply Chain Management Practices.” Computers & Industrial Engineering 105: 338–347.
- Saunders, M., P. Lewis, and A. Thornhill. 2016. “Research Methods for Business Students” (Seventh). Nueva York: Pearson Education.
- Shi, K., Q. Song, S. Lin, G. Xu, and Z. Cao. 2016. “An Improved Genetic Algorithm for Degree Constrained Minimum Spanning Trees.” In 2016 Chinese Control and Decision Conference (CCDC) (pp. 4603–4607). IEEE.
- Singh, K., and S. Sundar. 2020. “A Hybrid Genetic Algorithm for the Degree-Constrained Minimum Spanning Tree Problem.” Soft Computing 24 (3): 2169–2186.
- Spanaki, K., E. Karafili, and S. Despoudi. 2019. “Data Sharing in Agriculture 4.0: Applications of AI for Access Control Decisions.” https://repository.lboro.ac.uk/articles/Data_sharing_in_Agriculture_4_0_Applications_of_AI_for_access_control_decisions/9497639.
- Statista. 2018. “Revenues from the Artificial Intelligence (AI) Market Worldwide from 2016 to 2025.” Accessed April 15, 2020. [Online]. https://www.statista.com/statistics/607716/worldwide-artificialintelligence-market-revenues/.
- Syarif, A., Y. Yun, and M. Gen. 2002. “Study on Multi-Stage Logistic Chain Network: A Spanning Tree-Based Genetic Algorithm Approach.” Computers & Industrial Engineering 43 (1-2): 299–314.
- Tako, A. A., and S. Robinson. 2012. “The Application of Discrete Event Simulation and System Dynamics in the Logistics and Supply Chain Context.” Decision Support Systems 52 (4): 802–815.
- Tavana, M., A. Fallahpour, D. Di Caprio, and F. J. Santos-Arteaga. 2016. “A Hybrid Intelligent Fuzzy Predictive Model with Simulation for Supplier Evaluation and Selection.” Expert Systems with Applications 61: 129–144.
- Teece, D. J. 2007. “Explicating Dynamic Capabilities: The Nature and Microfoundations of (Sustainable) Enterprise Performance.” Strategic Management Journal 28 (13): 1319–1350.
- Thompson, K. N. 1990. “Vendor Profile Analysis.” Journal of Purchasing and Materials Management 26 (1): 11–18.
- Timmerman, E. 1986. “An Approach to Vendor Performance Evaluation.” Journal of Purchasing and Materials Management 22 (4): 2–8.
- Toorajipour, R., V. Sohrabpour, A. Nazarpour, P. Oghazi, and M. Fischl. 2021. “Artificial Intelligence in Supply Chain Management: A Systematic Literature Review.” Journal of Business Research 122: 502–517.
- Tranfield, D., D. Denyer, and P. Smart. 2003. “Towards a Methodology for Developing Evidence Informed Management Knowledge by Means of Systematic Review.” British Journal of Management 14 (3): 207–222.
- Tseng, M. L. 2011. “Green Supply Chain Management with Linguistic Preferences and Incomplete Information.” Applied Soft Computing 11 (8): 4894–4903.
- Tseng, M. L., and A. S. Chiu. 2013. “Evaluating Firm's Green Supply Chain Management in Linguistic Preferences.” Journal of Cleaner Production 40: 22–31.
- Van Eck, N., and L. Waltman. 2009. “Software Survey: VOSviewer, a Computer Program for Bibliometric Mapping.” Scientometrics 84 (2): 523–538.
- Wang, J., O. K. Ersoy, M. He, and F. Wang. 2016. “Multi-offspring Genetic Algorithm and its Application to the Traveling Salesman Problem.” Applied Soft Computing 43: 415–423.
- Wang, H. F., and H. W. Hsu. 2010. “A Closed-Loop Logistic Model with a Spanning-Tree Based Genetic Algorithm.” Computers & Operations Research 37 (2): 376–389.
- Wang, Y., J. P. Skeete, and G. Owusu. 2021. “Understanding the Implications of Artificial Intelligence on Field Service Operations: A Case Study of BT.” Production Planning & Control, 1–17. doi:10.1080/09537287.2021.1882694.
- Weber, C. A., J. R. Current, and W. C. Benton. 1991. “Vendor Selection Criteria and Methods.” European Journal of Operational Research 50 (1): 2–18.
- Yan, E., and Y. Ding. 2012. “Scholarly Network Similarities: How Bibliographic Coupling Networks, Citation Networks, Cocitation Networks, Topical Networks, Coauthorship Networks, and Coword Networks Relate to Each Other.” Journal of the American Society for Information Science and Technology 63 (7): 1313–1326.
- Yeh, W. C., and M. C. Chuang. 2011. “Using Multi-Objective Genetic Algorithm for Partner Selection in Green Supply Chain Problems.” Expert Systems with Applications 38 (4): 4244–4253.
- Yu, W., W. Graham, and H. Min. 2002, December. “Dynamic Pattern Matching for Demand Forecasting Using Temporal Data Mining.” Proceedings of the 2nd International Conference on Electronic Business, Taipei, Taiwan, 400–402.
- Zadeh, L. A. 1965. “Fuzzy Sets.” Information and Control 8 (3): 338–353.
- Zahiri, B., P. Jula, and R. Tavakkoli-Moghaddam. 2018. “Design of a Pharmaceutical Supply Chain Network Under Uncertainty Considering Perishability and Substitutability of Products.” Information Sciences 423: 257–283.
- Zhou, G., H. Min, and M. Gen. 2002. “The Balanced Allocation of Customers to Multiple Distribution Centers in the Supply Chain Network: A Genetic Algorithm Approach.” Computers & Industrial Engineering 43 (1-2): 251–261.