Abstract
Transportation is an important component in the logistics and production processes. To accurately match rapidly growing demand and limited transport capacity, the goal of minimising costs while ensuring high service quality under existing infrastructure has received significant attention. This paper presents an integrated optimisation approach for the short-term operational management under daily fluctuating demand, with a focus on two key strategic decisions: train timetabling and coupling. In particular, an integrated two-stage stochastic model and a combined heuristic local search algorithm with the branch-and-bound method are developed to (1) obtain the optimal demand assignment to the rail network, (2) investigate trains’ coupling plans to avoid waste of resources when demand is low, and (3) add candidate trains to generate new feasible timetables when demand surges. To verify the solving method, a lower bound algorithm is introduced. Using a hypothetical small-scale and a real-world China high-speed rail network as numerical experiments, different demand scales and critical parameters are tested to obtain optimised timetables. The results show that good solutions are achieved in several seconds, making it possible to adjust trains’ schedules efficiently and effectively according to the variable demand in short-term operational management.
Acknowledgments
The authors thank editors and reviewers for their useful suggestions and helpful comments on the earlier version of our paper.
Disclosure statement
No potential conflict of interest was reported by the author(s).
Data availability statement
The passenger demand of OD pairs data for the large-scale experiment can be found at https://doi.org/10.1057/s41274-017-0248-x.
Additional information
Funding
Notes on contributors
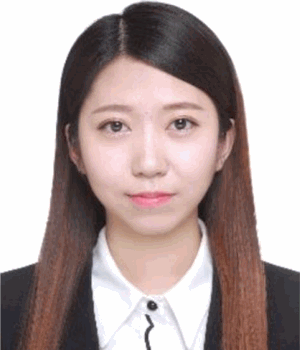
Ziyan Feng
Ziyan Feng is a Ph.D. candidate in the School of Traffic and Transportation, Beijing Jiaotong University, China. She is a member of the State Key Laboratory of Rail Traffic Control and Safety in Beijing Jiaotong University. Her research interests include transportation planning and stochastic optimization.
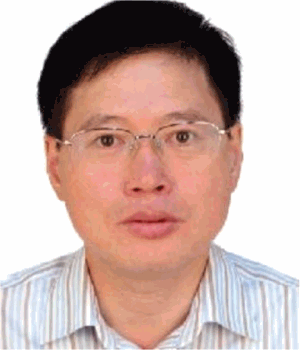
Chengxuan Cao
Chengxuan Cao is currently a Professor with the State Key Laboratory of Rail Traffic Control and Safety, Beijing Jiaotong University. His research interests include transportation planning and management, logistics transportation, optimization of the railway system, etc.
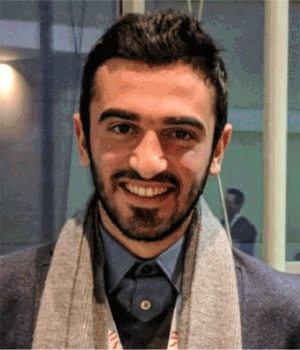
Alireza Mostafizi
Alireza Mostafizi is a Postdoctoral Scholar in Transportation Engineering Group at Oregon State University. He received his Ph.D. in Transportation Engineering with a minor in Computer Science from Oregon State University in 2019. His expertise is in machine learning, artificial intelligence, and robotics.
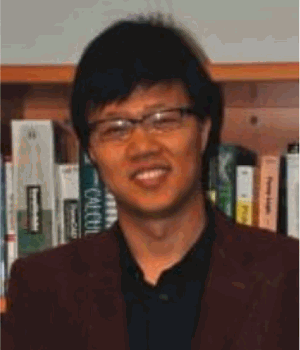
Haizhong Wang
Haizhong Wang is currently an Associate Professor with the School of Civil and Construction Engineering, Oregon State University, Corvallis, OR, USA. His research goal is to advance the theoretical and practical understanding of how social, natural, and engineered systems interact to enhance human life safety and infrastructure network resilience in normative and disruptive scenarios.
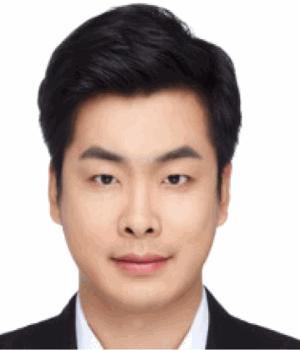
Ximing Chang
Ximing Chang is a Ph.D. candidate in the School of Traffic and Transportation, Beijing Jiaotong University, China. He is also a member of the State Key Laboratory of Rail Traffic Control and Safety in Beijing Jiaotong University. His research interests include travel behavior analyses, travel demand management, urban mobility based on traffic (big) data, and sustainable transportation.