Abstract
Quality fraud seriously damages the right-to-known of customers. Therefore, it is necessary for customers to recheck the process quality level declared by suppliers, which is often measured by process capability indices (PCIs). However, there exist two practical problems when rechecking PCIs based on a quality characteristic (QC). First, QC data often do not follow normal distributions. Second, to enhance the market competitiveness, the supplier usually conducts a full inspection and eliminates the non-conforming items before selling them, which causes the QC data to be truncated. To overcome these problems, motivated by the radio-frequency (RF) power output data, this paper proposes a two-phase process capability recheck method, including data-filling and generalised p-value (GE-P) test. The novel data-filling method integrates the quantile-filling (QA) and bias-correction closed-form (BC) estimator for Gamma distributions, and simulation results show it performs better than the traditional methods on unbiasedness and consistency. Based on the filling pseudo-complete data, GE-P is adopted to complete the recheck by testing whether the process capability reaches the supplier declared level. Numerical analysis indicates it performs well on two types of errors. Finally, a real case study on the motivating example is presented to verify the effectiveness of the proposed method.
Disclosure statement
No potential conflict of interest was reported by the author(s).
Data availability statement
The data that support the findings of this study are available from the corresponding author, [JY], upon reasonable request.
Additional information
Funding
Notes on contributors
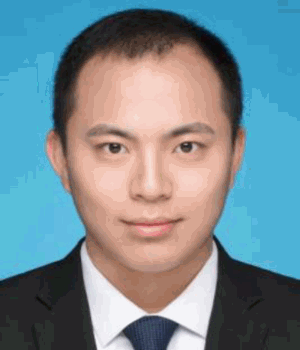
Fanbing Meng
Fanbing Meng is a Ph.D. candidate at School of Reliability and Systems Engineering, Beihang University. He also received a master’s degree and a bachelor’s degree both at School of Reliability and Systems Engineering, Beihang University. His recent research interests include industrial statistics, process capability analysis and reliability assessment.
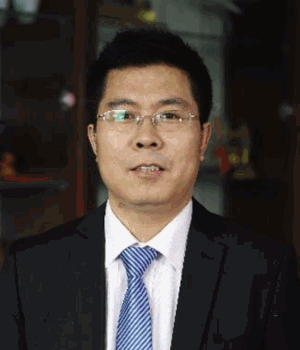
Jun Yang
Jun Yang received the B.S. degree in mathematics and information science from Yantai University, Yantai, China, in 1998, and the Ph.D. degree in probability and statistics from the Chinese Academy of Sciences, Beijing, China, in 2006. He is currently a Professor in systems engineering with the School of Reliability and Systems Engineering, Beihang University, Beijing, China. His scientific interests include reliability modelling, applied statistics, statistical process monitoring, accelerated experiment design and analysis, network reliability, transfer learning.
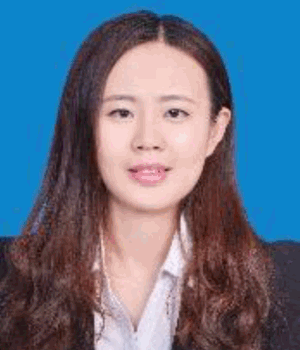
Qi Li
Qi Li is a Ph.D. candidate at the Department of School of Reliability and Systems Engineering at Beihang University. Her recent research interests include process capability analysis, manufacturing, maintenance, optimisation, reliability and scheduling, manufacturing and quality control.