Abstract
Because of advances in computational power and machine learning algorithms, the automated design of scheduling rules using Genetic Programming (GP) is successfully applied to solve dynamic job shop scheduling problems. Although GP-evolved rules usually outperform dispatching rules reported in the literature, intensive computational costs and rule interpretability persist as important limitations. Furthermore, the importance of features in the terminal set varies greatly among scenarios. The inclusion of irrelevant features broadens the search space. Therefore, proper selection of features is necessary to increase the convergence speed and to improve rule understandability using fewer features. In this paper, we propose a new representation of the GP rules that abstracts the importance of each terminal. Moreover, an adaptive feature selection mechanism is developed to estimate terminals’ weights from earlier generations in restricting the search space of the current generation. The proposed approach is compared with three GP algorithms from the literature and 30 human-made rules from the literature under different job shop configurations and scheduling objectives, including total weighted tardiness, mean tardiness, and mean flow time. Experimentally obtained results demonstrate that the proposed approach outperforms methods from the literature in generating more interpretable rules in a shorter computational time without sacrificing solution quality.
Disclosure statement
No potential conflict of interest was reported by the author(s).
Data availability statement
The authors confirm that the data supporting the findings of this study are available within the article.
Additional information
Notes on contributors
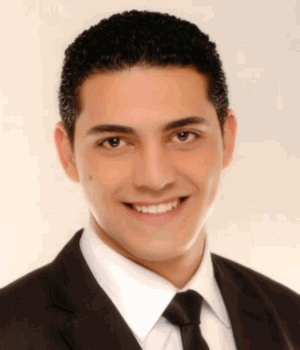
Salama Shady
Salama Shady received a master's degree in industrial engineering and systems management from the Egypt Japan University of Science and Technology in 2018. He is currently a PhD student at the graduate school of system informatics, Kobe University, Japan. His research interests include production planning and optimisation, evolutionary computation, and simulation.
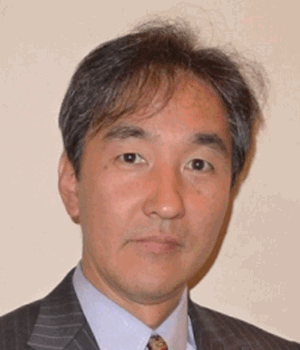
Toshiya Kaihara
Toshiya Kaihara is a Professor of Graduate School of System Informatics and a Director of Value Creation Smart Production Centre at Kobe University, Japan. He received his B.E. and M.E. degrees from Kyoto University, Japan, and his Ph.D. degree from Imperial College London, UK. He is an author of more than 250 publications of journal papers and books. He is a fellow member of JSME and CIRP (The International Academy of Production Engineering), and a member of IFAC, IFIP, IEEE, ASME, SICE, ISCIE, IEEJ, and many others. His research interests include systems optimisation theory, and its application into production, logistics, and social systems.
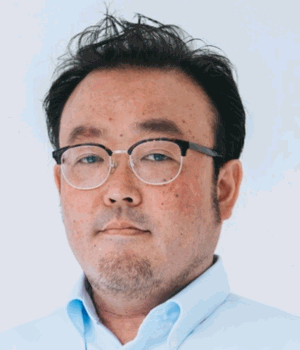
Nobutada Fujii
Nobutada Fujii is an Associate Professor of Graduate School of System Informatics, Kobe University, Japan. He received his bachelor and master degrees in engineering from Kobe University, Japan in 1996 and 1998, and Ph.D. in engineering from the University of Tokyo, Japan in 2004, respectively. His major research topics are about Autonomous and Decentralized Manufacturing Systems, Agent-based Modeling and Simulation of Social Systems, and Service Engineering.
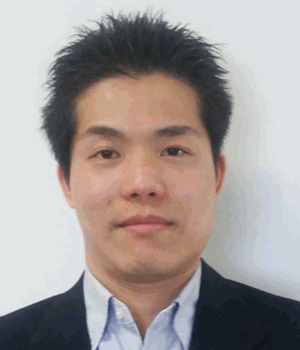
Daisuke Kokuryo
Daisuke Kokuryo received a Ph. D. degree in engineering from Kobe University in 2007. He is currently an assistant professor at Graduate School of System Informatics, Kobe University, Japan. His research interests include mathematical optimisation for medical systems, biomedical engineering and manufacturing systems.