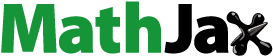
Abstract
The research interest in topics related to production and supply chain planning and control in engineer-to-order (ETO) environments has grown significantly over the last three decades. One of the strategically important and challenging decision areas for planning and control in ETO environments is estimating and quoting feasible delivery dates and assessing the feasibility of customer-imposed delivery dates, collectively referred to as delivery date setting (DDS). While DDS has received substantial attention in literature, research supporting the process in ETO companies is fragmented – lacking clear guidelines for industrial practice and gaps to guide future research on the topic. To address these issues, this study systematically reviews literature supporting DDS in ETO environments, identifying tools and practices proposed in the extant literature, and proposing an agenda for future research. Findings suggest that most of the research has focused on developing planning and decision-support tools for tactical capacity planning to support reliable DDS, however, with a noticeable lack of alignment with industrial decision-support needs of ETO environments. Furthermore, despite previous research emphasising the importance of high levels of coordination and formalisation in the DDS process, there is a lack of research to guide practitioners in achieving high levels of coordination and formalisation.
1. Introduction
Manufacturing companies producing big-sized electromechanical equipment with complex product structures, such as machine tools, power generation equipment, maritime equipment, etc., are often characterised by an Engineer-To-Order (ETO) strategy, which entails designing and/or re-designing products to customise them based on customer-specific requirements (Alfnes et al. Citation2021; Hicks, McGovern, and Earl Citation2000). Order-fulfilment activities, i.e. activities performed to fulfil specific customer orders, in ETO environments include physical activities, such as procurement, fabrication, assembly, testing, etc.; as well as non-physical activities, such as tendering, design, engineering, process planning, etc. (Adrodegari et al. Citation2015; Amaro, Hendry, and Kingsman Citation1999; Bertrand and Muntslag Citation1993; Wikner and Rudberg Citation2005). Order-specific product customisation and the cumbersome and high-value components used in such products necessitate that these activities are partially or fully order-driven instead of forecast-driven (Adrodegari et al. Citation2015; Gosling and Naim Citation2009; Olhager Citation2003). The order-specificity of these activities creates a complex environment for production and supply chain planning processes in ETO companies (Mello et al. Citation2017; Stavrulaki and Davis Citation2010).
One of the production and supply chain planning processes, which is of high strategic importance for ETO companies, is the delivery date setting (DDS) process, which comprises of (1) estimating the delivery dates quoted before order-confirmation, e.g. in tendering, bidding, responding to customer enquiries or requests-for-proposal (RFPs), etc.; and (2) assessing the feasibility of meeting delivery dates requested or imposed by customers, alternatively known as the order acceptance decision (Carvalho, Oliveira, and Scavarda Citation2015; Hicks, McGovern, and Earl Citation2000; Zijm Citation2000; Zorzini, Stevenson, and Hendry Citation2012). The main challenge within DDS in ETO environments is determining the delivery lead time (i.e. “the time from the receipt of an order to the delivery of the product” (Chapman et al. Citation2017, 15)) required for executing the order-fulfilment activities while meeting the company’s strategic objectives vis-à-vis operating costs, profitability, customer service, etc.
ETO environments are typically characterised by long delivery lead times, owing to many order-driven activities (Zennaro et al. Citation2019). These long delivery lead times are comprised of design and engineering lead times, procurement or supplier lead times, and lead times for production, including fabrication, assembly, and testing (Alfnes et al. Citation2021). In some ETO contexts, lead times for commissioning, installation, etc., may also constitute significant parts of the delivery lead time (Adrodegari et al. Citation2015; Semini et al. Citation2014). Moreover, the different components of delivery lead times often overlap, e.g. due to parallel execution of engineering, procurement, and production activities (Cannas et al. Citation2019; Iakymenko et al. Citation2018). Figure illustrates the delivery lead time components in a generic ETO order-fulfilment timeline.
Figure 1. Typical order-fulfilment timeline in ETO manufacturing - adapted from illustrations by Iakymenko et al. (Citation2018) and Semini et al. (Citation2014).
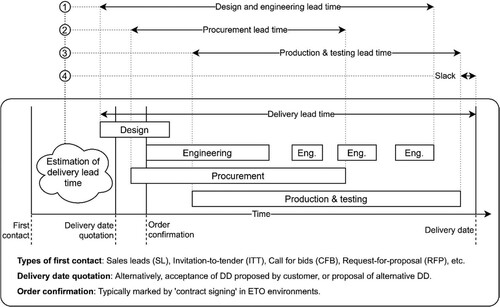
Due to the characteristically long delivery lead times, delivery precision can be a key performance indicator for customer service levels in ETO environments, and in the long run, be a source of competitive advantage (Amaro, Hendry, and Kingsman Citation1999; Cannas et al. Citation2020; Grabenstetter and Usher Citation2014; Hicks, McGovern, and Earl Citation2000). Improving the reliability of delivery lead time estimation in the DDS process is one of the critical components of improving delivery performance in ETO environments (Hicks, McGovern, and Earl Citation2000). While the DDS process and its sub-processes, e.g. delivery lead time estimation, delivery date quotation, and delivery date feasibility assessment or order acceptance, have received a lot of attention in the literature, many extant studies focus on the needs of Make-to-Order (MTO) environments with no order- or customer-specific design and engineering, and do not address the needs of ETO environments.
Customer-specific design and engineering are vital features differentiating the ETO and MTO order-fulfilment strategies. This differentiation is crucial for delivery lead time estimation since customer-specific engineering activities introduce significant uncertainty in the delivery lead time in ETO environments (Alfnes et al. Citation2021; Shurrab, Jonsson, and Johansson Citation2020). Specifically, customer-specific engineering adds two main elements of uncertainty to delivery lead times, namely:
Uncertainty in design and engineering lead times, as it is often difficult to predict the duration and number of iterations required before product drawings are finalised. This varies across customers due to, e.g. variations in customers’ technical knowledge of the product, change behaviour, etc. (Shurrab, Jonsson, and Johansson Citation2020).
Uncertainty in procurement and production lead times, as planning procurement and production activities is challenging before product specifications, process specifications, material requirements, capacity requirements, etc., are finalised (Alfnes et al. Citation2021).
Moreover, up to 70-80% of the value of ETO products may be created in the upstream supply chain (Gourdon and Steidl Citation2019), which further contributes to the uncertainty in delivery lead times in ETO environments.
Another factor differentiating DDS in ETO and MTO environments is the hierarchical decision level for setting delivery dates and executing the associated planning and estimation tasks. Within operations management literature, planning decisions and tasks are often classified hierarchically as strategic, tactical, or operational (Anthony Citation1965; Pereira, Oliveira, and Carravilla Citation2020; Stevenson Citation2015) – where the decisions and tasks on these levels usually differ in terms of their:
planning horizons, i.e. long, mid-range, and short.
decision scope, e.g. organisation or enterprise-wide decisions, cross-functional decisions, and function-specific decisions or tasks.
organisational level, e.g. top-management level, middle-management level, and supervisory, execution or operative level.
The appropriate decision level for DDS in MTO environments has been debated by researchers for several years, with Kingsman, Tatsiopoulos, and Hendry (Citation1989) perhaps marking the initiation of this debate. While some have argued that DDS should be considered a tactical decision in MTO environments (Ebadian et al. Citation2008; Ebadian et al. Citation2009), separating it from operational tasks of dispatching and detailed scheduling on the shop floor (Huang Citation2017), many others have continued to treat it as an operational level decision, integrating it with detailed scheduling in the shop-floor (Li and Ventura Citation2020; Ouz, Sibel Salman, and Bilgintürk Yalçın Citation2010). However, for ETO environments, the classification of DDS as a tactical decision is almost unequivocal in literature, as described below.
In the hierarchical classification framework of strategic, tactical, and operational decisions, DDS in ETO environments best fits as a tactical decision that is characterised by high planning complexity and uncertainty, and should be addressed as part of the organisation’s sales and operations planning (S&OP) process (Carvalho, Oliveira, and Scavarda Citation2015; Shurrab, Jonsson, and Johansson Citation2020). The high complexity of DDS emerges from that ETO environments are often multi-project manufacturing environments where a large number of factors must be considered for reliable DDS, e.g. engineering requirements and capacity, production capacity, material availability, suppliers’ lead times, overtime and subcontracting costs, the strategic importance of individual customers, etc. (Adrodegari et al. Citation2015; Carvalho, Oliveira, and Scavarda Citation2015; Grabenstetter and Usher Citation2014; Shurrab, Jonsson, and Johansson Citation2020; Zorzini, Corti, and Pozzetti Citation2008; Zorzini, Stevenson, and Hendry Citation2012). Trends such as globalisation and global competition (Cannas et al. Citation2019, Citation2020) and outsourcing and offshoring (Stavrulaki and Davis Citation2010; Zorzini, Stevenson, and Hendry Citation2012) have further added to this complexity. The high uncertainty characterising DDS emerges from (1) other ‘floating’ quotations or unconfirmed orders (also known as contingent demand) when delivery dates for an order are quoted, and (2) partially undefined product and process specifications when delivery dates are quoted (Carvalho, Oliveira, and Scavarda Citation2015; Hicks, McGovern, and Earl Citation2000; Wullink et al. Citation2004). Due to typically long delivery lead times, DDS in ETO environments requires mid-range to long planning horizons (Zennaro et al. Citation2019), and significant uncertainty dictates that estimation of delivery lead times is usually based on rough-cut or aggregate planning (Adrodegari et al. Citation2015; Carvalho, Oliveira, and Scavarda Citation2015; Hans et al. Citation2007), which aim to balance supply and demand (Shurrab, Jonsson, and Johansson Citation2020). Collectively, these characteristics justify the classification of DDS in ETO environments as a tactical S&OP decision.
Previous literature suggests four main practices to manage the high complexity and uncertainty of DDS in ETO environments, namely,
cross-functional coordination, i.e. information-sharing between different functions or departments, and joint decision-making to mitigate risks emerging from misaligned or conflicting objectives of different functions, and scatteredness of information such as customers’ requirements, suppliers’ lead times, engineering workloads, production workloads, etc. among different functions (Hendry and Kingsman Citation1989, 1993; Kingsman et al. Citation1993; Konijnendijk Citation1994; Zorzini, Corti, and Pozzetti Citation2008; Zorzini et al. Citation2008).
supply chain coordination, i.e. information-exchange with key downstream actors, e.g. customers and sales agents, and upstream actors such as suppliers and subcontractors; and collaborative decision-making to mitigate risks emerging from unrealistic assumptions regarding suppliers’ capacity availability, lead times, etc. (Alfnes et al. Citation2021; Hicks, McGovern, and Earl Citation2000; Zorzini, Stevenson, and Hendry Citation2012).
formalisation of the DDS process, i.e. establishing clear and systematic process flows for activities that are performed for quoting delivery dates, and formalising the underlying decision-making procedures and decision-rules (Adrodegari et al. Citation2015; Zorzini, Corti, and Pozzetti Citation2008; Zorzini et al. Citation2008; Zorzini, Stevenson, and Hendry Citation2012).
the use of software tools for planning and decision-support for effectively and systematically considering relevant factors for estimating lead times and determining delivery dates to be quoted (Adrodegari et al. Citation2015; Carvalho, Oliveira, and Scavarda Citation2015; Corti, Pozzetti, and Zorzini Citation2006; Grabenstetter and Usher Citation2014).
The main practices for DDS in ETO environments highlighted above point to four corresponding industrial application areas that research should support, i.e. mechanisms for cross-functional coordination, mechanisms for supply chain coordination, process frameworks and methodologies for formalising the DDS process, and development of planning and decision-support tools to support DDS. The identified application areas lead us to pose the research questions (RQs) that motivate this study.
RQ1: What tools, methods and frameworks are proposed in the literature to support delivery date quotation and order acceptance decisions in ETO manufacturing?
RQ2: What gaps and challenges should future research address to better support DDS in ETO manufacturing?
While several published studies address different issues within DDS in ETO and MTO environments, research on the topic is fragmented, lacking clear guidelines for industrial practice in ETO environments and a set of gaps to guide future research on the topic. To address this shortcoming of DDS literature vis-à-vis ETO environments, it is essential to (1) assess the extent to which DDS literature supports the application areas within DDS in ETO environments and (2) outline an agenda for future research on the topic. The current study aims to accomplish this through a systematic review of literature. The characteristics of extant DDS literature, as described below, necessitate a systematic review to address these research questions.
Firstly, as highlighted earlier, the type of industrial contexts that previous DDS studies have aimed to support varies from ETO (Ghiyasinasab et al. Citation2021; Micale et al. Citation2021) to MTO (Li and Ventura Citation2020; Ouz, Sibel Salman, and Bilgintürk Yalçın Citation2010) and hybrid MTO/Make-to-Stock (MTS) (Rafiei and Rabbani Citation2012; Wang et al. Citation2019) environments. Therefore, while there are vast volumes of literature on the topics of DDS, lead time estimation, and order acceptance, not all of this literature offers relevant insights for the application areas within DDS in ETO environments. Secondly, while MTO and ETO environments may have different requirements vis-à-vis DDS, tools and frameworks proposed for MTO environments can sometimes be adapted to address the needs of ETO environments (Adrodegari et al. Citation2015). Therefore, it is also essential to assess which studies contextualised in MTO environments provide relevant tools, methods or frameworks that can be utilised or adapted for ETO environments. Finally, the ETO strategy has been adopted in a wide variety of industrial contexts that differ in (1) the complexity and level of customisation of products, (2) production processes and systems, (3) level of vertical integration, (4) planning methodologies and planning systems used, etc. (Adrodegari et al. Citation2015; Alfnes et al. Citation2021; Aslan, Stevenson, and Hendry Citation2015; Hicks, McGovern, and Earl Citation2000; Zennaro et al. Citation2019; Zorzini, Corti, and Pozzetti Citation2008; Zorzini et al. Citation2008; Zorzini, Stevenson, and Hendry Citation2012). As a result, different ETO environments also have unique requirements for tools and practices to support DDS that strategically fit the characteristics of their planning environment (Buer et al. Citation2016; Zorzini et al. Citation2008). This further necessitates analysis of tools and practices proposed in literature to assess their generalisability to different ETO contexts.
There are reviews in extant literature that address some DDS issues in ETO environments, however, only partly since they focus on other topics. For instance, Aslan, Stevenson, and Hendry (Citation2012) focus in their literature review on assessing the applicability of enterprise resource planning (ERP) systems in MTO and ETO environments, identifying a need to develop tools for DDS that can be embedded within ERP systems. The authors corroborate this finding in a later mixed-method study (Aslan, Stevenson, and Hendry Citation2015). Hendry and Kingsman (Citation1989) and Stevenson, Hendry, and Kingsman (Citation2005) are older reviews with a similar perspective. Zennaro et al. (Citation2019) review literature on MTO and ETO production environments, focusing on production systems that manufacture big-sized products. They identify models for defining price and delivery times, and models for capacity planning as two of the main research areas in these environments and identify some key contributions in these areas that are relevant for DDS, e.g. Grabenstetter and Usher (Citation2014); Carvalho, Oliveira, and Scavarda (Citation2015); etc. However, due to the exploratory nature and broader scope of their literature review, their coverage of the DDS-related research areas is limited to planning and decision-support tools. Other related literature reviews include Cannas and Gosling (Citation2021) and Gosling and Naim (Citation2009), where also, the authors adopt a broader perspective of supply chain management in ETO environments. In addition to identifying relevant planning and estimation tools (similar to Zennaro et al. (Citation2019)), these papers also identify literature relevant for coordination between functions and with suppliers in ETO environments, e.g. Mello et al. (Citation2017); Zorzini, Corti, and Pozzetti (Citation2008); etc. However, these reviews also have a broader conceptual scope and do not focus on DDS-related research areas. Other reviews, such as Cheng and Gupta (Citation1989); Gordon, Proth, and Chu (Citation2002); Ragatz and Mabert (Citation1984a); and Slotnick (Citation2011), focus on operational level DDS, where DDS is often integrated with detailed scheduling and sequencing on the shop floor, which is not common practice in ETO contexts. Therefore, to the best of our knowledge, our study is the first to take the perspective of ETO manufacturing environments in systematically reviewing the literature on DDS, delivery lead time estimation, and order acceptance.
The remainder of the paper is organised as follows. Section 2 describes the methodology adopted for identifying, selecting, and analysing relevant literature. Section 3 presents descriptive statistics about the reviewed literature and summarises the main contributions from extant research in the four application areas identified earlier, addressing RQ1. Section 4 discusses the main gaps observed in the extant literature and proposes an agenda for future DDS research to better support ETO companies, addressing RQ2. Section 5 summarises the findings and contributions of this paper.
2. Methodology
This study adopts a systematic literature review (SLR) approach for answering the RQs presented in Section 1. The SLR approach is suitable for answering this study’s main RQs as the underlying aim of the RQs is to (1) establish the state of the art on the topic (DDS) within a context of interest (ETO manufacturing), and (2) identify research gaps to serve as research agenda for future knowledge development on the topic to support industrial practice. The SLR approach focuses on transparency of the steps adopted in reviewing literature and has therefore been argued as an effective tool for laying the foundation for future research by uncovering areas where more research is required through analysis and synthesis of past research (Watson and Webster Citation2020; Webster and Watson Citation2002). Furthermore, in their seminal paper on systematic reviews in management studies, Tranfield, Denyer, and Smart (Citation2003) highlight that SLRs not only contribute to theory development, but also support practitioners by developing “a reliable knowledge base by accumulating knowledge from a range of studies”.
Our SLR follows the typical steps suggested by methodological references on SLRs within operations and supply chain management (Thomé, Scavarda, and Scavarda Citation2016; Tranfield, Denyer, and Smart Citation2003), similar to other recent SLRs (Cannas and Gosling Citation2021; Kristensen and Jonsson Citation2018; Pereira, Oliveira, and Carravilla Citation2020), namely: (1) formulating the problem; (2) searching and selecting literature; (3) analysing the data, and synthesising and interpreting the results. The following subsections provide an overview of these steps.
2.1. Problem formulation
The main factors motivating this SLR are described in Section 1. They can be summarised as (1) the strategic importance of DDS in ETO environments, (2) the complexity and uncertainty characterising DDS that make it a challenging task in practice, and (3) the lack of guidelines for practitioners and a future research agenda based on the state of the art of the topic in extant literature. These factors are further reinforced by industrial trends observed over the last decades, namely, increased globalisation and advancements in information and communication technologies. Globalisation has led to fiercer competition among globally dispersed ETO manufacturers and supply chains on performance dimensions such as price and delivery reliability (Alfnes et al. Citation2021; Cannas et al. Citation2020). Meanwhile, the growing emergence and viability of advanced communication and computation technologies, which are often encapsulated within the Industry 4.0 concept, create opportunities to address industrial problems through applications of technology that have not been feasible before (Zheng et al. Citation2021). However, establishing the state of the art and its gaps are prerequisites for exploring whether and how technological solutions could further the state of the art within DDS in ETO environments.
2.2. Literature identification and selection
The relevant literature for this SLR was identified using two databases - Scopus and WebOfScience. The search string used to identify literature was organised into three blocks of keywords – one concept-related keyword block and two context-related keyword blocks. Figure shows the search string, visualising the three keyword blocks as components of a ‘scoping funnel’.
Figure 2. Keyword-search string visualised.
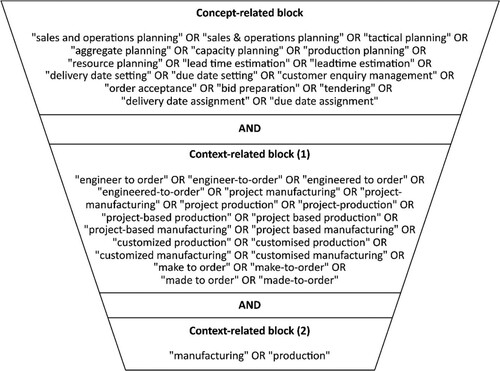
The concept-related keyword block was initialised with terms such as ‘lead time estimation’ and ‘delivery date setting’, and gradually expanded as other relevant terms, e.g. ‘customer enquiry management’, ‘tendering’, etc., were identified using references from Zennaro et al. (Citation2019). Initially, the search string was only comprised of the first two keyword blocks. The third keyword block was later added to focus the search results on manufacturing contexts since the ETO strategy has also been adopted in non-manufacturing contexts, such as the construction industry (Shurrab, Jonsson, and Johansson Citation2020). The keywords within each search block were connected with the or Boolean operator, and the three search blocks were connected with the and operator as shown in Figure . The search was constrained only to identify papers that contained the specified keywords in their abstracts, titles, or the paper’s list of keywords.
Using the specified keywords and searching for literature up to and including October 2021, 582 results were retrieved from Scopus, and 398 from WebofScience, including journal articles, conference proceedings, and book sections. These results were exported into an EndNote library, where, after automated removal of duplicates, 627 results remained. Data for these 627 results, e.g. title, authors, year of publication, source name, etc., were exported to an Excel spreadsheet for record-keeping and analysis. After a manual screening for duplicates, nine other duplicate papers were identified and excluded, leaving 618 unique results.
The titles and abstracts of the 618 papers were screened for identifying and excluding irrelevant papers. During this screening step, we excluded papers (1) that were not in English; (2) focusing on topics unrelated or vaguely related to delivery date quotation, delivery lead time estimation, and order acceptance; and (3) concerning products or production environments producing products that cannot be characterised as structurally complex, e.g. food, apparel, etc. Consequently, 362 papers were excluded, leaving 256 papers for further consideration.
The full texts of the 256 papers were assessed to identify papers that should be included in the review. In this step, besides the three exclusion criteria stated above, we also excluded papers that (4) were literature reviews, conceptual or discussion papers that did not propose a specific tool, method, or framework for the decision-area of DDS; (5) did not have a full text published and available online; and (6) were not from a peer-reviewed source. Consequently, 182 of the papers were excluded, leaving 74 papers for the next steps in the review. Based on these 74 papers, 33 additional papers were identified through backwards and forward citation searches, resulting in 107 papers. The reader is referred to methodological papers on SLRs, e.g. Thomé, Scavarda, and Scavarda (Citation2016), for a description of the backward and forward citation search technique.
The 107 identified papers focus on issues relevant to DDS, delivery lead time estimation or order acceptance, albeit in different production environments, namely, ETO, MTO, hybrid MTO/ATO, and hybrid MTO/MTS contexts. We performed a preliminary content analysis of these 107 papers to select papers that offered relevant insights, tools, methods, or frameworks for tactical DDS in ETO environments, leaving 54 papers for the final review and detailed content analysis. For the transparency of this preliminary content analysis, Table in the Appendix summarises the 53 papers that were excluded in this step. Figure summarises the literature identification and selection process in a PRISMA (Preferred Reporting Items for Systematic Reviews and Meta-Analyses) flowchart, adapted from Buer, Strandhagen, and Chan (Citation2018) and Moher et al. (Citation2009).
Figure 3. PRISMA flowchart illustrating the literature identification and selection process (adapted from Buer, Strandhagen, and Chan (Citation2018) and Moher et al. (Citation2009)).
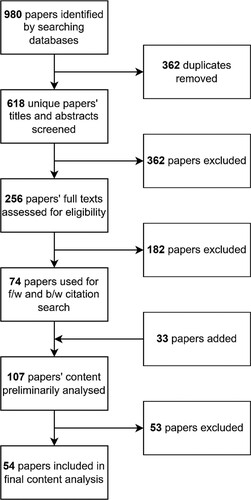
2.3. Data analysis and results’ synthesis
The content of papers included in the review was analysed using the four application areas within DDS (introduced in Section 1) as a guiding framework. The papers were classified according to each paper’s application area(s). Different strategies were adopted for analysing the contributions within each application area.
For contributions within cross-functional coordination and supply chain coordination, the content analysis focused on (1) identifying relevant coordination mechanisms (tools and practices that facilitate coordination) proposed or described in the papers; and (2) identifying insights on the effect of contextual factors on coordination needs or requirements. For contributions within DDS formalisation, the content analysis focused on identifying frameworks or methodologies that can serve as a reference for designing and developing formal DDS processes in ETO companies. Finally, for contributions within planning and decision-support tools for DDS, the content analysis focused on (1) identifying the main problems within DDS addressed by the proposed tools; (2) identifying the different types of tools and techniques proposed for addressing the problems; and (3) identifying relevant dimensions to describe the proposed tools and logically cluster them. Table shows how the results and research agenda for the four application areas are organised to address RQ1 and RQ2.
Table 1. Structure of results and research agenda for the four main application areas.
3. Results
This section presents the results of the content analysis of the reviewed literature. First, we present the distribution of the identified literature across different journals and years. These distributions are presented for DDS literature relevant for ETO environments, i.e. papers concerning tactical DDS; and the overall DDS literature, which includes papers from Table in the Appendix. Next, subsection 3.1 categorises relevant papers based on the DDS application areas within which their contributions are positioned. Subsection 3.1 also describes the additional categorical dimensions for classifying the relevant literature identified inductively during the content analysis. Subsections 3.2, 3.3, and 3.4 summarise contributions from literature in the different application areas.
Table 2. Distribution of papers in different sources (journals and conference proceedings).
Table shows the distribution of papers across journals and conference proceedings for papers relevant for tactical DDS and papers on DDS in general. As evident, the International Journal of Production Research and the International Journal of Production Economics have been the two leading outlets for research on the topic of DDS, irrespective of the decision level (i.e. tactical or operational). Moreover, almost 50% of the reviewed papers are from these two journals.
Table 3. Summary of reviewed papers.
Figure shows the distribution of published papers across the years. As noticeable, the early years until 1988 show no papers within tactical DDS, while the overall trend of the number of papers has been upward since 1989. This is consistent with the fact that Tatsiopoulos and Kingsman (Citation1983) perhaps pioneered tactical DDS, being the first to argue that DDS is not necessarily an operational decision and should be considered on higher levels of decision hierarchy, following up on this in their later paper in 1989 (Kingsman, Tatsiopoulos, and Hendry Citation1989). A surprising observation is that despite the growing interest in literature on ETO manufacturing (Cannas and Gosling Citation2021; Zennaro et al. Citation2019), there have been no publications addressing DDS in ETO environments during recent years 2018–20.
Figure 4. Distribution of publications across years - the overall number of papers and the number of papers concerning tactical DDS.
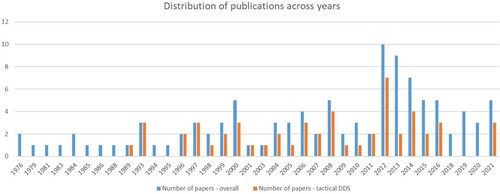
3.1. Classification of literature supporting DDS in ETO manufacturing
The relevant literature has been selected such that each paper contributes towards one or more of the four main application areas within DDS in ETO environments, as identified in Section 1. Table classifies the reviewed papers into one or more application areas based on the papers’ contributions. In addition, the content analysis of papers revealed other dimensions that can be used to classify the papers, as described below.
Type of production system or configuration of production resources, which identifies the type of manufacturing system considered, e.g. single-resource, job shop, assembly job shop (i.e. a job shop with an assembly stage), flow shop or line, etc. If the type of manufacturing system is considered an independent variable in the study, this dimension is labelled ‘varied’, implying that different resource configurations are considered.
Industrial context and order-fulfilment strategy, which identify the industrial environment that the research is contextualised in or is motivated by, i.e. the industry sector or main products produced; and the order-fulfilment strategy of the industrial context, i.e. ETO, MTO, MTO/ATO or MTO/MTS.
Empirical nature, which identifies the methodological positioning of a paper, following the classification adapted from Carvalho, Oliveira, and Scavarda (Citation2015) and Jahangirian et al. (Citation2010), into one of the following categories – practical problem-solving paper (class A), hypothetical problem-solving paper (class B), and methodological paper (class C). A paper is classified under class A if (1) the paper proposes a method or tool that has been applied in an industrial case, and (2) the paper provides implementation details and/or describes post-implementation improvements. A paper is classified under class B if (1) the paper addresses a problem that is motivated analytically, or (2) the paper briefly mentions a motivating practical context but does not explicitly present a specific industrial case or describe how data from the case has been used. A paper is classified under class C if (1) the paper addresses an analytically or practically motivated problem, testing the proposed method or technique on case data, but does not include practical implementation details or a post-implementation performance assessment, or (2) the paper addresses an analytically motivated problem without a specific industrial case and does not quantitatively assess the performance of the proposed method or technique. N.B. Exceptions from this classification scheme are papers studying industrial practice in multiple companies, that are classified as ‘multiple case study’.
Besides the dimensions described above, we identified additional dimensions that apply to papers in the application area of planning and decision-support tools, as described below and used in Table .
Type of tool, which identifies whether a paper proposes or describes (1) an optimisation model (and the problem that the model addresses), or (2) mathematical models for representing lead times or delivery dates as functions of other variables and parameters, or (3) heuristics for tactical capacity planning or resource-loading, or (4) other decision-making methodologies and decision-support systems (and the decision addressed).
Technique(s) for optimisation modelling identifies the approaches adopted for formulating and/or solving optimisation problems in the papers proposing optimisation models.
Optimisation objective or performance indicators used, which identifies (1) the main objective pursued in optimisation in papers proposing optimisation models, or (2) the performance indicators used, if any, to evaluate the performance of proposed heuristics, mathematical models, or other decision-making methodologies.
As evident from Table , most of the papers are positioned within the application area of planning and decision-support tools, with some of these papers also addressing other application areas. Expectedly, most relevant papers are from MTO and ETO environments, and some others are from hybrid MTO/ATO or MTO/MTS environments.
3.2. Cross-functional and supply chain coordination
The positive influence of cross-functional coordination and supply chain coordination on the effectiveness of DDS has been extensively highlighted in literature, not only by studies focusing on DDS, e.g. Kingsman et al. (Citation1993); Zorzini et al. (Citation2008); and Zorzini, Stevenson, and Hendry (Citation2012); but also studies focusing on other issues in ETO environments. For instance, in a mixed-methods study on the interface between marketing and manufacturing functions in ETO environments, Konijnendijk (Citation1994) identify the uncertainty during lead time estimation and DDS for customer orders as the most significant challenge necessitating coordination between the marketing and manufacturing functions. Hicks, McGovern, and Earl (Citation2000) highlight the importance of coordination during the quotation phase between different functions within the enterprise, e.g. sales/marketing, engineering, design, and procurement, and coordination with key suppliers. Highlighting the importance of aggregate capacity planning in the DDS phase, Zijm (Citation2000) points out that this aggregate capacity planning in ETO companies should be cross-functional, considering not only manufacturing but also the design and engineering department(s).
Despite the criticality of cross-functional coordination and supply chain coordination for effective DDS, we found few studies with contributions that aim to enable or improve coordination in the DDS process in ETO environments. The few papers identified in this area can be grouped into two main clusters based on their contribution type. The first cluster consists of three studies that contribute insights into contextual factors that influence the need for cross-functional coordination and supply chain coordination in the DDS process in ETO environments. The second cluster consists of seven studies that propose methods, models, or frameworks for decision-support in cross-functional planning or collaborative planning and negotiation across tiers in the supply chain.
3.2.1. Contextual factors influencing coordination needs
In a multiple case study of DDS practices in 15 ETO and MTO capital goods manufacturers, Zorzini, Corti, and Pozzetti (Citation2008) identify the main contextual factors affecting the cross-functional coordination requirements for effective DDS and order acceptance. These factors should be considered in the strategic selection of relevant coordination mechanisms based on the required level of coordination. The main factors affecting the level of cross-functional coordination needed are identified by Zorzini, Corti, and Pozzetti (Citation2008) as (1) level of product complexity; (2) degree of product customisation; (3) flexibility of production capacity; and (4) relevance of delivery time as an order winning criterion; while two other secondary factors, namely, (5) company size and (6) the number of tenders and orders managed annually are also found to be relevant in some cases. They also propose four different levels of cross-functional coordination, namely, no coordination, occasional coordination, ongoing coordination, and advanced coordination, linking each coordination-level to a set of coordination mechanisms with increasing degree of sophistication, namely, e-mails and phone, on-demand meetings, standard documentation, periodic follow-up meetings, integrated information systems, and dedicated organisational roles for coordination.
Building on findings from Zorzini, Corti, and Pozzetti (Citation2008), Zorzini et al. (Citation2008) develop a contingency framework for the customer enquiry management or DDS process to study the effect of the design of DDS practices on delivery performance in 18 ETO capital goods manufacturing companies. Zorzini et al. (Citation2008) identify contextual uncertainty as another important factor affecting the level of cross-functional coordination and upstream supply chain coordination (i.e. coordination with suppliers and subcontractors) required in the DDS process. Furthermore, Zorzini et al. (Citation2008) find a high level of cross-functional coordination in the DDS process a best practice.
Zorzini, Stevenson, and Hendry (Citation2012) expand the contingency framework proposed by Zorzini et al. (Citation2008) through a study of the DDS process in seven ETO and MTO capital goods manufacturing companies, taking a supply chain perspective rather than scoping in on individual manufacturing enterprises. They identify factors affecting the required level of supply chain coordination in the DDS process as (1) the level of vertical integration; (2) the number of tiers in the supply chain; (3) the number of actors in each tier; (4) the level of the geographical dispersion of suppliers and subcontractors; and (5) downstream actors’ (customers/sales agents) level technical knowledge of the product. They also find the level of technical knowledge of the product and production system in different departments within the company as a factor influencing the required level of cross-functional coordination in the DDS process. Furthermore, they identify the proportion of customised orders as a moderating factor between DDS process design and delivery performance, which has been overlooked in their previous studies (Zorzini, Stevenson, and Hendry Citation2012).
Figure synthesises the findings from the papers summarised above into a conceptual framework of contextual factors affecting cross-functional and supply chain coordination needs in the DDS process. The proposed framework can serve as a decision-support tool for identifying the appropriate level of coordination and selecting relevant coordination mechanisms while designing or redesigning the DDS process in ETO companies.
Figure 5. Conceptual framework of contextual factors affecting coordination needs based on findings from Zorzini, Corti, and Pozzetti (Citation2008); Zorzini et al. (Citation2008); and Zorzini, Stevenson, and Hendry (Citation2012).
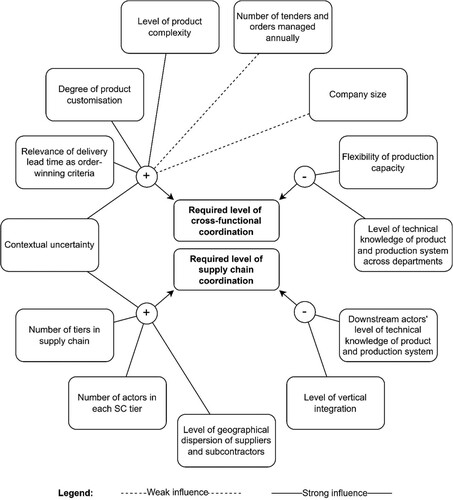
3.2.2. Decision-support for planning across functions and supply chains
Kingsman et al. (Citation1993) focus on the coordination between sales/marketing and production functions, proposing the use of strike rate matrices to facilitate this coordination. Their proposed methodology entails combining information from the production function, namely, pairs of production lead times and production costs for different scenarios, and information from the marketing function, i.e. order-winning probabilities (strike rates) for different pairs of lead times and prices (calculated from production costs) into a matrix that can serve as decision-support for the marketing function while quoting delivery dates. Kingsman and Mercer (Citation1997) further elaborate the description of the methodology, describing the procedure for initialising and updating the matrices, and illustrate its application with an example of a military equipment supplier.
Azevedo and Sousa (Citation2000) propose a component-based architectural design of a decentralised information system to address the decision-support requirements for order-promising in distributed MTO manufacturing enterprises. Calosso et al. (Citation2003) model the process of interfirm negotiations with suppliers and customers in the DDS process in an MTO business-to-business electronic-commerce environment and propose mixed-integer linear programming models to address the decision-support needs of the focal MTO manufacturing firm as well as the customer and suppliers. Ebadian et al. (Citation2008) focus on the decision-support requirements of the focal MTO firm and propose two mixed-integer programming models to (1) determine the delivery time and price to be quoted for new orders by minimising production costs, and (2) determine the best set of suppliers and subcontractors for an order by minimising raw-material purchasing costs, subcontracting costs, and procurement lead times.
In recent contributions, Brachmann and Kolisch (Citation2021) and Ghiyasinasab et al. (Citation2021) propose decision-support models for tactical or aggregate planning in ETO environments taking a cross-functional perspective. Brachmann and Kolisch (Citation2021) model a flexible resource-constrained multi-project scheduling problem (FRCMPSP) using mixed-integer programming and considering engineering and production resources in the model; and illustrate the utility of the model through the example of an ETO manufacturer of packaging machines for the pharmaceutical industry. Ghiyasinasab et al. (Citation2021) propose a multi-objective optimisation approach for multi-project scheduling to support order acceptance in an ETO SME (small/medium-sized enterprise) supplying engineered wood to the construction industry, where engineering and production activities are incorporated into the proposed set of models.
3.3. Formalisation
Formalising the DDS process entails establishing predefined procedures, decision rules, roles and responsibilities of the actors involved, information flows and workflows among the different actors, etc. Such formalisation can systematise decision-making in the DDS process, ensuring that any relevant factors and constraints are considered. Furthermore, predefined procedures and actors’ roles and responsibilities may lower cross-functional barriers for information flow. However, achieving high levels of formalisation of the DDS process in practice can be challenging in ETO environments, and the level to which the DDS process can be formalised depends on a particular context’s characteristics (Kingsman Citation2000; Kingsman et al. Citation1993; Konijnendijk Citation1994; Zorzini et al. Citation2008).
Due to the diversity or lack of homogeneity across different ETO contexts, designing and developing widely applicable planning systems and generally valid process models and frameworks has been difficult (Hicks and Braiden Citation2000; Zorzini et al. Citation2008). Nevertheless, process models and frameworks have been suggested as valuable tools for supporting the formalisation of business processes by externalising and making explicit the information flows and decision mechanisms that might otherwise remain tacit and hinder continuous improvement (Kalpic and Bernus Citation2002). Therefore, the contributions of the majority of the studies relevant for the application area of DDS process-formalisation are context-specific frameworks, which are summarised in Table . These frameworks can serve as initial references for developing process models or frameworks for DDS process-formalisation in other contexts. Exceptions to this type of contribution are the studies by Zorzini et al. (Citation2008) and Zorzini, Stevenson, and Hendry (Citation2012), which establish the DDS process's formalisation as a best practice through multiple case studies.
Table 4. Contributions in the area of DDS formalisation.
Table 5. Summary of papers focusing on operational DDS (excluded from final content analysis).
3.4. Planning and decision-support tools
As highlighted earlier, planning and decision-support tools represent the application area that most research within DDS has focused on. Contributions in this area include (1) optimisation models for planning and decision-support with explicitly stated objective function(s) and constraints, e.g. linear programming models (Özdamar and Yazgaç Citation1997), mixed-integer programming models (Calosso et al. Citation2003), dynamic programming models (Kapuscinski and Tayur Citation2007), stochastic programming models (Alfieri, Tolio, and Urgo Citation2012), etc., solved either with exact solution methods (Carvalho, Oliveira, and Scavarda Citation2015), or heuristics (Wullink et al. Citation2004; Yang and Fung Citation2014) and metaheuristics (Manavizadeh et al. Citation2013); (2) mathematical models or polynomial models representing lead times and/or delivery dates as functions of other variables and parameters, derived analytically or using regression-analysis (Grabenstetter and Usher Citation2014; Ioannou and Dimitriou Citation2012; Thürer et al. Citation2012); (3) heuristics for capacity planning or resource-loading (Corti, Pozzetti, and Zorzini Citation2006; Thürer et al. Citation2012) and insights for developing resource-loading heuristics in different contexts (Ebben, Hans, and Olde Weghuis Citation2005; Robinson and Moses Citation2006; Zorzini, Corti, and Pozzetti Citation2008); and (4) other procedures and methods for decision-making and decision-support systems (Kingsman, Tatsiopoulos, and Hendry Citation1989; Kingsman et al. Citation1996; Mourtzis et al. Citation2014; Parsaei et al. Citation2012). Using the criteria introduced in subsection 3.1, Table summarises relevant papers’ contributions. The main insights and observable trends, similarities, and differences from this body of literature are outlined below.
3.4.1. Optimisation models
The content analysis of papers proposing optimisation models to support DDS suggests the possibility to differentiate the proposed papers based on four main criteria, namely, (1) the decisions or problems that the proposed model addresses within the DDS process, (2) their main underlying objective in the decision-making process, (3) the paper’s empirical nature, and (4) the context guiding the formulation and assumptions of the proposed model. The main objective(s), the empirical nature, and the context of the papers in terms of the type of production system or configuration of resources, the industrial context, and the order-fulfilment strategy are summarised in Table . The main decisions addressed by the proposed models are summarised below, along with a discussion of their corresponding objectives.
Most of the optimisation models proposed for supporting DDS focus on the tactical capacity planning- or resource-loading problem, which entails planning production and/or engineering by allocating capacity to tenders/customer enquiries and confirmed customer orders over a time horizon of several weeks/months and quoting delivery dates (or assessing the feasibility of customer-requested delivery dates) based on such a finite capacity plan (Alfieri, Tolio, and Urgo Citation2011), where subcontracting and overtime can be used for adding capacity flexibility (Carvalho, Oliveira, and Scavarda Citation2015). The two exceptions are Hegedus and Hopp (Citation2001), who focus on determining the optimal policy for quoting delivery dates under customer-requested delivery dates and uncertain procurement lead times; and Piya, Khadem, and Shamsuzzoha (Citation2016), who focus on simultaneous negotiation of multiple orders. Two of the papers integrate tactical capacity planning with other decisions, namely, Easton and Moodie (Citation1999), who integrate tactical capacity planning with pricing; and Calosso et al. (Citation2003), who integrate tactical capacity planning with pricing and supplier selection.
Carvalho, Oliveira, and Scavarda (Citation2015, Citation2016); Ebadian et al. (Citation2008); Ghiyasinasab et al. (Citation2021); Kalantari, Rabbani, and Ebadian (Citation2011); Kapuscinski and Tayur (Citation2007); Manavizadeh et al. (Citation2013); Wullink et al. (Citation2004); and Özdamar and Yazgaç (Citation1997) propose models for tactical capacity planning with cost minimisation objectives. Besides a cost-minimisation model, Ghiyasinasab et al. (Citation2021) propose additional models with project/order makespan minimisation and setup time minimisation objectives to facilitate comparative analysis of plans with different objectives. Alfieri, Tolio, and Urgo (Citation2011, Citation2012) also propose models with makespan minimisation objectives. Other objectives for tactical capacity planning models include maximising profit or revenue (Arredondo and Martinez Citation2010; Calosso et al. Citation2003; Easton and Moodie Citation1999; Micale et al. Citation2021; Yang and Fung Citation2014), minimising the difference between promised- and customer-requested delivery dates (Wang, Fang, and Hodgson Citation1998), minimising lateness and overtime (Brachmann and Kolisch Citation2021), and minimising fluctuations in usage and utilisation rate of resources (Calosso et al. Citation2003).
While some of the papers consider delivery dates as endogenous or internally set, treating them as a model output (Easton and Moodie Citation1999; Kapuscinski and Tayur Citation2007; Özdamar and Yazgaç Citation1997), most others assume exogenous or customer-requested delivery dates as model constraints, e.g. Alfieri, Tolio, and Urgo (Citation2011); and Carvalho, Oliveira, and Scavarda (Citation2015). Ebadian et al. (Citation2008) differentiate between negotiable and non-negotiable exogenous delivery dates, which suggests that delivery dates can be a ‘hard’ constraint in some problem instances while being a ‘soft’ constraint in other cases (Hvolby and Steger-Jensen Citation2010; Zorzini, Corti, and Pozzetti Citation2008).
Some of the above papers also incorporate uncertainty in optimisation, considering primarily two sources of uncertainty, namely, (1) uncertainty in resource requirements due to unpredictability of order-confirmation or acceptance of tender/bid by customer(s), i.e. contingent orders; and (2) uncertain activity duration or resource-requirements due to incomplete product- and process specifications, i.e. bill-of-materials (BOM) and routing. Uncertainty in resource requirements due to contingent orders is considered by Easton and Moodie (Citation1999) in their capacity planning and pricing model and by Piya, Khadem, and Shamsuzzoha (Citation2016) in their negotiation model. Uncertainty of activity duration and/or resource requirements due to incomplete BOM and routing has been addressed in literature through different scenario-based models for planning. Alfieri, Tolio, and Urgo (Citation2012) propose a two-stage stochastic programming model, Carvalho, Oliveira, and Scavarda (Citation2016) propose a robust optimisation model supported by Monte Carlo simulation, and Wullink et al. (Citation2004) propose a single-stage stochastic programming model for tactical capacity planning under uncertain activity duration and/or resource requirements. Other papers use deterministic models to support scenario-based planning with a ‘what-if’ analysis of alternative plans (Carvalho, Oliveira, and Scavarda Citation2015; Ghiyasinasab et al. Citation2021).
3.4.2. Mathematical models
The second stream of literature within the application area of tools for planning and decision-support consists of papers that develop and/or test mathematical models to represent lead times as a function of other factors that are known or can be estimated. This stream consists of five papers whose contributions and findings are summarised and discussed below.
Adam et al. (Citation1993) and Thürer et al. (Citation2012) compare different mathematical models and heuristic procedures for lead time estimation in assembly job shops using simulation. Factors used in these papers for estimating production lead times include total work content or sum of processing times for a new order, work content on the critical path of a new order, and different measures of unprocessed work in the shopfloor, e.g. estimated waiting time and workload in queues at relevant work centres. Ruben and Mahmoodi (Citation2000) also compare different mathematical models and heuristic procedures for lead time estimation, but in a shop with a single bottleneck work centre whose position varies. The considered mathematical models estimate lead times: (1) using workload in queues at all relevant work centres; or (2) only using workload in the queue at the bottleneck work centre; or (3) using workload in queues at the bottleneck and non-bottleneck work centres while differentiating between the two. Ioannou and Dimitriou (Citation2012) derive analytical expressions for estimating lead times for dynamically updating manufacturing lead times in MRP (Material Requirements Planning)/ERP systems in MTO manufacturing environments with different resource configurations, where they model lead times as a function of the sum of processing times at the machines included in an order’s production routing and the time spent waiting in queues in front of these machines. Grabenstetter and Usher (Citation2014) develop the ‘regression-driven complexity-based flow time prediction’ (RegComp) method for estimating engineering lead times in ETO environments and illustrate the utility of the proposed method through the example of an ETO motor control centre manufacturer. They model engineering lead times as a function of (1) number of functional requirements, (2) number of basic components, (3) number of design interdependencies, (4) number of technologies, (5) number of regulations and standards, (6) number of sub-systems, and (7) presence of a reference job. Grabenstetter and Usher (Citation2014) and the authors’ earlier paper (Grabenstetter and Usher Citation2013) are valuable contributions to engineering lead time estimation within the DDS literature, which predominantly comprises papers focusing on production lead times.
While the papers from Adam et al. (Citation1993); Ruben and Mahmoodi (Citation2000); and Thürer et al. (Citation2012) are originally positioned within operational DDS, these studies also offer valuable insights for production lead time estimation within tactical DDS in ETO environments because of (1) being contextualised in a production system with a moving bottleneck (Ruben and Mahmoodi Citation2000), and (2) being contextualised in assembly job shops (Adam et al. Citation1993; Thürer et al. Citation2012). Estimating production lead times based on bottleneck work centres can help simplify the task of estimation (Zorzini, Corti, and Pozzetti Citation2008). However, in practice, bottlenecks in the production process are often not stationary but shifting based on the production orders in the backlog (Mestry, Damodaran, and Chen Citation2011). The findings from Ruben and Mahmoodi (Citation2000) provide insights into the conditions under which bottleneck-based production lead time estimation for tactical DDS may be effective in ETO environments. They find that bottleneck-based lead time estimation is most effective when the bottleneck is located early in the production process. Not surprisingly, they also conclude that the effectiveness of bottleneck-based lead time estimation decreases with increasing ‘shiftiness’ of the bottleneck.
Assembly job shops often characterise production environments producing complex products with multi-level product structures (Thürer et al. Citation2012), as is often the case for complex ETO products. Therefore, the studies of Adam et al. (Citation1993) and Thürer et al. (Citation2012) provide valuable insights into (1) the behaviour of assembly job shops, and consequently, (2) the type of methods that can be used in these shops to estimate production lead times. Findings from both of these studies suggest that simple heuristic procedures (i.e. critical path flow time procedure (Adam et al. Citation1993) and forward-finite-loading (Thürer et al. Citation2012)) outperform regression-analysis based mathematical models in lead time estimation accuracy. Furthermore, the findings of Thürer et al. (Citation2012) demonstrate the utility of carefully developed resource-loading heuristics for production lead time estimation and DDS in complex production systems that characterise ETO environments.
The above discussion highlights (1) the inadequacy of using simple mathematical models or closed-form analytic expressions for estimating production lead times in tactical DDS in ETO environments and (2) the utility of heuristic procedures for the same. The discussion also underlines the trade-off between the simplicity of estimation methods and their corresponding estimation accuracy. Simple analytical expressions may be better received by practitioners as the basis for decision-support and estimation tools as compared to black-box optimisation tools (de Man and Strandhagen Citation2018), which may also be computationally burdensome for large problem instances (Alfieri, Tolio, and Urgo Citation2011, 2012). However, findings from Thürer et al. (Citation2012) suggest that such simple mathematical models may not provide sufficient estimation accuracy. Therefore, carefully designed heuristics for resource-loading or tactical capacity planning can provide the practically essential balance between the simplicity of mathematical models and the accuracy of optimisation models for tactical capacity planning in ETO environments.
3.4.3. Heuristics for tactical capacity planning
This sub-subsection summarises the stream of literature that concerns the development of tactical capacity planning heuristics for DDS without formulating optimisation problems with formally stated objectives and constraints. Such heuristics can be effective planning and decision-support tools in industrial practice in ETO environments. They allow for the utilisation of domain- and context-specific knowledge of researchers and practitioners for circumventing the complexity of formulating and solving large-scale optimisation problems for tactical capacity planning, which often grow drastically in their computational complexity (Alfieri, Tolio, and Urgo Citation2012; Brachmann and Kolisch Citation2021; Micale et al. Citation2021) and may be under-preferred by practitioners in comparison to simpler, well-understood heuristic methods (de Man and Strandhagen Citation2018). This stream of literature includes (1) papers proposing heuristics that can be utilised as-is or adapted for tactical capacity planning and (2) papers providing insights that can be utilised for developing heuristics for tactical capacity planning.
Adam et al. (Citation1993) propose the critical path flow time (CPFT) heuristic procedure for lead time estimation in assembly job shops and find its application advantageous for (1) single-level assembly jobs in terms of tardiness and percentage of tardy jobs, (2) two-level assembly jobs in terms of percentage of tardy jobs, and (3) three-level assembly jobs in terms of tardiness. Thürer et al. (Citation2012) suggest further improvements to the CPFT procedure and find it outperformed by the forward-finite-loading (FFL) heuristic, suggesting FFL as the heuristic that should be preferred in practice due to its consideration of time-phased workload. Thürer et al. (Citation2012) attribute the original development of the FFL heuristic to Bertrand (Citation1983), referring to it as the Bertrand approach. In their comparative studies of different DDS methods, both, Thürer et al. (Citation2012) and Moses et al. (Citation2004) consider simulation-based heuristics called simulation-based due date setting (SIM) and incremental forward simulation (IFS) respectively for estimating delivery dates, which are both based on the simulation-based heuristic called total work based on simulation (TWSIM) proposed by Roman and del Vallei (Citation1996). Results from the study of Thürer et al. (Citation2012) suggest that the FFL heuristic also outperforms these simulation-based heuristics.
Park et al. (Citation1999) develop a heuristic entitled heuristic delivery date decision algorithm (HDDDA) for DDS in an MTO electric motor manufacturing context, where the focus is on the bottleneck process, i.e. the final assembly. Corti, Pozzetti, and Zorzini (Citation2006) propose a methodology for the feasibility assessment of customer-requested delivery dates based on capacity requirements, arguing that the ‘what if’ analysis feature distinguishes their approach from previous work. The proposed approach classifies potential orders under three classes: feasible, feasible with capacity adjustments, or infeasible. Wu and Liu (Citation2008) propose procedures for determining delivery dates and assessing the feasibility of customer-requested delivery dates based on the bottleneck resource in a drum-buffer-rope system, illustrating the application of the proposed Capable-to-Promise (CTP) approach in MTO manufacturing of integrated circuit packaging. Their proposed approach provides a time-phased view of capacity-constrained resources’ unused capacity and allows sales personnel to sell idle capacity more effectively.
Ebben, Hans, and Olde Weghuis (Citation2005) and Robinson and Moses (Citation2006) study the effect of granularity in resource-loading heuristics, where Ebben, Hans, and Olde Weghuis (Citation2005) focus on the effect of considering aggregated capacity from multiple resources (Aggregate Resource Loading or ARL heuristic) versus considering the capacity for each resource individually (Resource Loading per Resource or RLR heuristic); and Robinson and Moses (Citation2006) focus on the size of the time-buckets considered in resource-loading. Findings from these studies suggest that (1) heuristics considering individual resources should be preferred over those considering aggregated resources (Ebben, Hans, and Olde Weghuis Citation2005); and (2) the size of time-buckets used for resource-loading heuristics should be carefully selected based on context-specific considerations such as target utilisation and mean processing time (Robinson and Moses Citation2006). Other important factors to be considered in this decision are identified by Zorzini, Corti, and Pozzetti (Citation2008) and Zorzini et al. (Citation2008) in their multiple-case studies, e.g. level of product complexity, degree of product customisation, flexibility of production capacity, relevance of delivery time as an order winning criterion, contextual uncertainty, etc., with further supporting evidence provided by Zorzini, Stevenson, and Hendry (Citation2012).
One of the essential inputs for developing tactical capacity planning heuristics is the capacity allocation strategy, i.e. forward loading or backward loading (Thürer et al. Citation2012), alternatively referred to as forward and backward planning (Zorzini, Corti, and Pozzetti Citation2008). Thürer et al. (Citation2014) and numerous other papers (Corti, Pozzetti, and Zorzini Citation2006; Ebadian et al. Citation2008; Thürer et al. Citation2012; Zorzini, Corti, and Pozzetti Citation2008) highlight the link between the type of delivery dates (i.e. endogenous or internally set or negotiable versus exogenous or customer-imposed) and the type of capacity allocation strategy used in tactical capacity planning. The forward planning strategy is more suitable when delivery dates are set internally and then quoted to a customer or when delivery dates are requested by customers but are negotiable. On the other hand, the backward planning strategy is more suitable for order acceptance decisions for orders with fixed customer-imposed delivery dates. In practice, managers and planners may encounter both types of orders, which necessitates that heuristics proposed for tactical capacity planning can utilise both forward and backward planning strategies, as required.
3.4.4. Decision-making methodologies and decision-support systems
Besides the three categories of tools supporting DDS summarised in the previous three subsections, namely, (1) optimisation models, primarily for tactical capacity planning; (2) mathematical models for estimating lead times and delivery dates; and (3) tactical capacity planning heuristics; there are few other methodologies, frameworks, and decision-support systems proposed in the literature to support different decisions and tasks within DDS. The methodologies, high-level frameworks, and decision-support systems proposed in this stream of literature are summarised below.
In one of the earliest papers considering DDS as a tactical decision, Kingsman, Tatsiopoulos, and Hendry (Citation1989) propose a methodology for managing customer enquiries in MTO environments that comprises three elements: aggregate production planning, strike rate analysis, and delivery date and price quotation. The production planning component of the methodology is further described by Hendry and Kingsman (Citation1993) and Kingsman (Citation2000), and the strike rate analysis component by Kingsman et al. (Citation1993) and Kingsman and Mercer (Citation1997). Kingsman et al. (Citation1996) combine the methodological elements to conceptualise a decision-support system to address the needs of ETO capital goods manufacturers and MTO subcontracting companies. Later, Stevenson (Citation2006) utilise methodological elements from Hendry and Kingsman (Citation1993); Kingsman et al. (Citation1993); and Kingsman (Citation2000) to develop a decision-support system and study issues related to the implementation of the system in an MTO subcontracting company.
De Boer, Schutten, and Zijm (Citation1997) discuss the elements of a decision-support system developed for capacity planning in an ETO ship maintenance facility, outlining a forward planning heuristic for tactical capacity planning for DDS (similar to the FFL heuristic from Thürer et al. (Citation2012)), and discussing the resource-constrained project scheduling problem formulation for detailed project scheduling. Park et al. (Citation1999) describe a decision-support system and underlying heuristics for bottleneck-based planning developed for capacity planning and DDS in MTO production of electric motors. Azevedo and Sousa (Citation2000) describe the ‘component-based’ architecture of a decentralised information system developed for addressing DDS in an MTO production network in the semiconductor industry. Hing, van Harten, and Schuur (Citation2007) explore the application of Reinforcement Learning (RL) for order acceptance in job shops using a Q-learning RL algorithm.
Ebadian et al. (Citation2009) propose a methodology for prioritising customer orders based on a customer attractiveness analysis of different customers in MTO contexts, extending their previous work on decision-making within DDS (Ebadian et al. Citation2008). Kalantari, Rabbani, and Ebadian (Citation2011) focus on the decision-support requirements for DDS in hybrid MTS/MTO production, proposing the use of fuzzy TOPSIS (Technique for Order of Preference by Similarity to Ideal Solution) to prioritise customer orders. Later, Hemmati, Ebadian, and Nahvi (Citation2012) propose the application of TOPSIS for prioritising customer orders in the case of an MTO domestic appliance manufacturer. Parsaei et al. (Citation2012) combine fuzzy AHP (Analytical Hierarchy Process) and TOPSIS for prioritising customer orders in the case of an MTO manufacturer of vehicle safety belts. In the case of a hybrid MTS/MTO producer of wood products, Rafiei and Rabbani (Citation2012) propose a heuristic for determining the lot sizes for MTS and MTS/MTO product families.
Wattanapornprom and Li (Citation2013) propose a framework for integrating Available-to-Promise (ATP) and CTP functions with Master Production Scheduling (MPS) in the case of an MTO/ATO parasol manufacturer. Mourtzis et al. (Citation2014) propose a methodology for estimating lead times for orders based on their similarity to previous orders using Case-Based Reasoning (CBR), and Mourtzis, Doukas, and Vlachou (Citation2016) implement the methodology as a mobile application that aims to address the needs of an ETO manufacturer of injection moulds.
4. Research gaps and future research agenda
Based on the content analysis of the papers summarised in the previous section, this section (1) outlines the main research gaps within DDS in ETO environments and (2) proposes an agenda for future research activities to address the outlined research gaps. Based on an overview of the reviewed literature and the distribution of contributions in different application areas, we consider it appropriate to organise the discussion of research gaps and proposed research agenda under two subsections, namely, (1) formalisation and coordination, which discusses the gaps and research agenda for three application areas within DDS – formalisation, cross-functional coordination, and supply chain coordination; and (2) planning and decision-support tools, which discusses the gaps and research agenda for the application area of the same name.
4.1. Formalisation and coordination
Within research relevant for DDS in ETO environments, we observe a general dearth of contributions to support (1) the formalisation of the DDS process; and (2) coordination among different functions or departments and across the supply chain. Only 14 of the 54 reviewed papers contribute towards formalisation or coordination in the DDS process. Despite findings from previous empirical research suggesting high levels of formalisation of the DDS process and coordination in the DDS process as best practices (Zorzini et al. Citation2008; Zorzini, Stevenson, and Hendry Citation2012), there is little research to support practitioners in achieving these high levels of formalisation and coordination.
There is a need to develop process models and reference frameworks for the DDS process that explicate the information flow between different actors involved to support practitioners in ETO environments in formalising the DDS process. Most of the previously proposed process models and frameworks (summarised in subsection 3.3) are contextualised in MTO environments. Furthermore, the modelling or mapping methodologies vary across extant models and frameworks, suggesting the need to explore which methodologies are suitable for mapping and modelling the DDS process in ETO environments. As previous literature has highlighted, ETO environments can differ significantly in their characteristics, and consequently, in their requirements for the DDS process (Hicks, McGovern, and Earl Citation2000; Zorzini et al. Citation2008; Zorzini, Stevenson, and Hendry Citation2012). Therefore, case studies in different ETO environments are expected to be vital for understanding these differences, and subsequently, for developing process models and reference frameworks for the DDS process. The three multiple case studies on DDS in the extant literature, i.e. Zorzini, Corti, and Pozzetti (Citation2008); Zorzini et al. (Citation2008); Zorzini, Stevenson, and Hendry (Citation2012), are indispensable contributions for the general understanding of the DDS process in ETO environments. However, the diversity of manufacturing companies operating with an ETO strategy necessitates further case studies for improving our understanding of similarities and differences in DDS practices and norms across different industry sectors and geographical regions. Evidence from multiple case studies can also serve as a basis for developing typologies or taxonomies of ETO companies (Hicks, McGovern, and Earl Citation2001; Willner et al. Citation2016) based on their DDS requirements. Such typologies can facilitate clarification of generalisability and contextual limitations of DDS tools and practices across different ETO environments.
Extant operations management literature suggests maturity models as valuable tools for (1) documenting and diagnosing the current state of business processes and strategy implementation (Grimson and Pyke Citation2007; Wagire et al. Citation2021); and (2) planning process improvements and designing evolutionary paths to increase the effectiveness of business processes (Danese, Molinaro, and Romano Citation2018). Due to the lack of maturity models in the extant literature on DDS in ETO environments, developing maturity models for the DDS process is another important area for future research. There is also a need for empirical research to identify coordination mechanisms that ETO companies can use to address different coordination problems in the DDS process. While broader research on cross-functional coordination (Konijnendijk Citation1994; Nam et al. Citation2018) and supply chain coordination (Mello et al. Citation2017) in ETO environments can provide some insights for this, further research focusing on coordination problems within DDS is required to support coordination improvements in practice.
Exploring the applications of Industry 4.0 technologies for improving cross-functional and supply chain coordination in the DDS process in ETO environments represents another promising area for future research. Industry 4.0 technologies such as cyber-physical systems (CPS), Internet-of-Things (IoT), big data analytics (BDA), cloud computing, etc., have significantly expanded the solution space for solving many industrial problems in manufacturing companies over the last decade (Zheng et al. Citation2021). However, opportunities for utilising these technologies to address problems in ETO environments have not been sufficiently explored (Strandhagen et al. Citation2020; Zennaro et al. Citation2019), and the DDS process is no exception from this trend. Therefore, future studies should investigate whether and how Industry 4.0 technologies can be utilised for coordination improvements in ETO companies and supply chains in the DDS process.
Summarising the discussion above, the following points are proposed as agenda for future research on coordination and formalisation in the DDS process in ETO environments:
Developing process models and reference frameworks to support DDS process design.
Exploring suitable mapping or process modelling methodologies for DDS.
Case research contextualised in different ETO industrial contexts and geographical locations.
Developing typologies or taxonomies of ETO companies based on DDS requirements.
Developing maturity models for the DDS process.
Exploring applications of Industry 4.0 technologies for improving coordination in the DDS process.
4.2. Planning and decision-support tools
While the body of literature on planning and decision-support tools for DDS in ETO environments is larger than the literature on formalisation and coordination, there are crucial gaps that necessitate further research and development in this area. Firstly, we observe that most of the literature in this area has focused on supporting the estimation of production lead times and production resource-loading or capacity planning. While these are valuable contributions, they only partially address planning and decision-support requirements for delivery lead time estimation and DDS in ETO environments, where engineering and procurement lead times are essential elements of the delivery lead time. Very few papers propose tools or systems for planning and decision-support across functions, e.g. sales and production (Kingsman and Mercer Citation1997; Kingsman et al. Citation1993), or engineering and production (Brachmann and Kolisch Citation2021; Ghiyasinasab et al. Citation2021); and across supply chains (Azevedo and Sousa Citation2000; Calosso et al. Citation2003). Therefore, we consider a comprehensive view of delivery lead times in developing planning and decision-support tools in future research an important aspect of enriching DDS literature vis-à-vis ETO environments. The regression-based approach for estimating engineering lead times proposed by Grabenstetter and Usher (Citation2013, Citation2014) is perhaps the only method of its kind in the literature. However, since the approach has been developed based on data from a single case company, it is essential to assess the broader validity of this approach in ETO environments. Therefore, future studies should undertake similar studies in other ETO environments to test and further develop the RegComp approach proposed by Grabenstetter and Usher (Citation2014).
One of the main challenges in developing and implementing planning and decision-support tools for DDS in ETO environments is the lack of formalised knowledge and information; and reliance on managerial experience, expertise, and tacit knowledge (Adrodegari et al. Citation2015; Zennaro et al. Citation2019). Commercial enterprise information systems have been found inadequate in supporting business processes and decisions in ETO environments, partly because of the lack of alignment between business processes in ETO environments and the traditional manufacturing enterprise structures underlying commercial enterprise information systems (Aslan, Stevenson, and Hendry Citation2012, 2015). While managerial experience and expertise may continue to be essential for effective DDS in ETO environments, advances in data storage, processing, and computation technologies provide new opportunities for supporting managerial intuition with data-driven insights. Design and development of effective enterprise information systems focusing on business processes and decision-support needs of ETO companies is an important step in this, which computational or formal ontologies can support. Formal ontologies have been suggested in the extant literature as tools for “formal, explicit specification of a shared conceptualisation of a domain of interest” (Scheuermann and Leukel Citation2014; Studer, Richard Benjamins, and Fensel Citation1998), that can be utilised in the development of industrial information systems (Ameri et al. Citation2021; Křemen and Kouba Citation2011; Usman et al. Citation2013). Therefore, developing high-level and application-specific ontologies to support the development of planning and decision-support systems for DDS in ETO environments can be valuable contributions in future research.
As evident from the reviewed literature, almost half of all reviewed papers have focused on the tactical capacity planning or resource-loading problem, either proposing optimisation models for the problem with different objectives or proposing and/or testing different heuristics for tactical capacity planning without formulating it as a formal optimisation problem. Findings from papers proposing optimisation models for tactical capacity planning suggest that while these models provide useful insights into the behaviour of the modelled ETO systems, the computation times for solving these models with exact algorithms can grow drastically with an increase in the size and complexity of the modelled problem, e.g. number of activities in a project, degree of parallel execution of activities in a project, number of projects planned or replanned, etc. (Alfieri, Tolio, and Urgo Citation2011, 2012; Carvalho, Oliveira, and Scavarda Citation2015; Micale et al. Citation2021). For instance, Alfieri, Tolio, and Urgo (Citation2011, Citation2012) call for future research to develop more efficient ad-hoc or heuristic algorithms for their proposed models due to the long computation times for larger problem instances. Furthermore, complex optimisation models that are not well-understood by users (planners and managers) and require long computational times have been found to negatively influence users’ willingness to use such models in practice (de Man and Strandhagen Citation2018; Ivert and Jonsson Citation2011). Instead, simple, well-understood heuristics developed using tacit domain knowledge of managers and the understanding of system behaviour gained from optimisation models can be helpful decision aids for practitioners. Furthermore, we observe that most of the models proposed in the reviewed literature have been theoretically motivated without explicitly linking model development to the industrial need in a specific context, with few exceptions (Carvalho, Oliveira, and Scavarda Citation2015, 2016; Ghiyasinasab et al. Citation2021). To narrow the gap between theory and practice in the area of planning and decision-support for DDS, we call for more class A (i.e. practical problem-solving) research in this area and emphasise that future development of tactical capacity planning tools should be motivated by practitioners’ decision-support needs within DDS in specific ETO contexts, regardless of whether the proposed tools are exact optimisation-based or heuristics. Moreover, focusing on users’ or stakeholders’ needs and decision-support requirements may also provide better insights into whether users prefer sophisticated models that provide optimal solutions or near-optimal solutions derived from simple heuristics.
Finally, based on literature proposing heuristics for tactical capacity planning, forward- and backward-finite-loading are the most straightforward approaches for determining delivery dates and for assessing the feasibility of customer-imposed delivery dates, respectively. However, for using these loading methods for DDS in ETO environments, additional methods are required for developing comprehensive capacity planning heuristics. For instance, ETO products are often characterised by wide BOMs with numerous diverging branches for different sub-assemblies. As a result, it may be possible to produce components for various sub-assemblies in parallel. However, if the same resources are used for fabricating components for different sub-assemblies, this introduces new precedence constraints in tactical capacity planning, adding to the planning complexity. Therefore, if forward- or backward loading approaches are to be used, it is also essential to define the appropriate method for BOM-traversal for specifying which components and sub-assemblies should be prioritised in resource-loading, at least on the bottleneck resources. The problem is further complicated by the ‘bottleneck shiftiness’ in such contexts, which means that each resource-loading scenario may have a different set of bottlenecks. Therefore, while extant literature provides preliminary inputs and insights, there is a need for further research to develop effective resource-loading heuristics for ETO environments, which should also be motivated by practical needs in specific industrial contexts.
Summarising the discussion above, the following points are proposed as agenda for future research on planning and decision-support tools in the DDS process in ETO environments:
Developing tools for cross-functional planning and decision-support in DDS.
Further testing of engineering lead time estimation tools proposed in the literature.
Developing ontologies to support the development of effective planning and decision-support systems for DDS.
Developing heuristic algorithms for optimisation formulations proposed in extant literature that are computationally inefficient to solve with exact solution algorithms.
Documenting managerial decision-support needs for effective DDS in ETO environments and developing planning and decision-support tools based on industrial requirements.
Further developing tactical capacity planning heuristics such that practical aspects such as complex product structures, parallel execution of activities, bottleneck shiftiness, etc., are accounted for.
5. Conclusion
This paper investigates the state of the art within DDS and delivery lead time estimation focusing on the needs of ETO manufacturing environments. A systematic literature review approach is adopted to (1) identify the tools, methods and frameworks proposed in the literature for supporting DDS in ETO environments; and (2) identify the gaps in extant research and formulate an agenda for future research. We differentiate between tactical DDS and operational DDS from the outset, considering only the former to be relevant for ETO environments. This differentiation also serves as the basis for one of the literature exclusion criteria in our methodology. The content analysis and summary of contributions from the reviewed literature are structured using a framework of four industrial application areas for research within DDS, namely, cross-functional coordination, supply chain coordination, process formalisation, and tools for planning and decision-support. These application areas also serve as the basis for discussing gaps in extant research and formulating an agenda for future research.
We find that despite previous research emphasising the importance of high levels of cross-functional coordination, supply chain coordination and formalisation in the DDS process in ETO environments, there is little research focusing on how these high levels of coordination and formalisation should be achieved in practice. Various initiatives that can be undertaken in future research to address this gap are discussed, e.g. empirical and inductive development of process models and frameworks for DDS, development of maturity models for the DDS process, cross-industry and cross-geography studies to elucidate differences and similarities in DDS practices, etc.
While many papers propose tools for planning and decision-support, most of these tools only address lead time estimation for the production process. To address the needs of ETO environments, where procurement and engineering lead times form significant portions of the delivery lead times, we call for a more comprehensive perspective of delivery lead times in future research on DDS in ETO environments. Furthermore, we find the majority of the papers proposing tools for planning and decision-support to be theoretically motivated, where industrial cases are used, if at all, to illustrate the application of the proposed tool. Instead, we call for future research to adopt more inductive methodologies, similar to Carvalho, Oliveira, and Scavarda (Citation2015, Citation2016); Ghiyasinasab et al. (Citation2021), and use industrial cases and practitioners’ decision-support needs as the fundament for developing planning and decision-support tools. As a prerequisite for this, future studies should also focus on describing and documenting industrial problems and the decision-support needs of practitioners.
To the best of our knowledge, this paper is the first systematic literature review on DDS in ETO environments where the existing knowledge on the topic has been analysed, and the agenda for future knowledge development has been presented. Furthermore, the paper compiles and summarises contributions from extant literature, which is expected to be helpful for the interested researchers and practitioners. Moreover, a secondary contribution of this paper is the identification of papers within operational DDS, which were excluded in the last stage of the literature identification process and are summarised in the Appendix in Table . A limitation of this study is that only two scholarly databases were used for identifying literature, which may have limited the coverage of literature. We expect that the forward and backward citation searches have contributed to minimising the negative impact of this limitation.
Data availability statement (DAS)
The data related to the final list of papers included in the systematic literature review is included in the manuscript, and any additional data about the papers can be made available by the authors upon request.
Disclosure statement
No potential conflict of interest was reported by the author(s).
Additional information
Funding
Notes on contributors
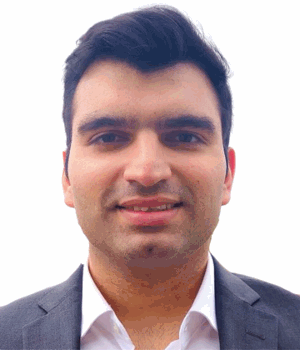
Swapnil Bhalla
Swapnil Bhalla is a PhD candidate at the Department of Mechanical and Industrial Engineering, Norwegian University of Science and Technology (NTNU) in Trondheim, Norway. He holds an MSc in Global Manufacturing Management from NTNU and a BEng in Mechanical Engineering from Delhi Technological University in New Delhi, India. He has three years of experience in manufacturing process analysis from the automotive industry in India. In his PhD, he is working on solutions for effective tactical sales and operations planning and delivery date quotation in engineer-to-order manufacturing environments focusing on maritime equipment suppliers. His research interests include information systems, data science and analytics, and decision-support in manufacturing supply chains.
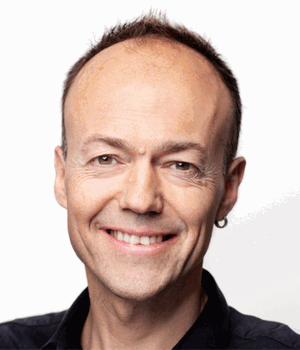
Erlend Alfnes
Erlend Alfnes is an associate professor at the Department of Mechanical and Industrial Engineering, Norwegian University of Science and Technology in Trondheim, Norway. He holds a PhD in Manufacturing Logistics. He has 15 years of experience as a manager of national and international research projects. His main research interests include manufacturing planning and control, enterprise resource planning systems, manufacturing strategy, lean manufacturing, and the integration of lean manufacturing and Industry 4.0, and his research activities focus on engineer-to-order industries.
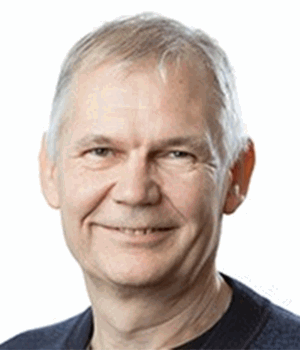
Hans-Henrik Hvolby
Hans-Henrik Hvolby is a Full Professor at the Centre for Logistics, Aalborg University in Denmark and a visiting professor at the University of Tasmania, Australia. For seven years, he was a visiting professor at the University of South Australia. He holds a PhD in Manufacturing Information Systems, has published more than 150 peer-reviewed papers and serves as an editorial board member of several international journals. For over two decades, he has been the project manager or partner in large research projects raising national and European research funding. His research areas include manufacturing information systems (MRP, ERP, APS and VMI), logistics, supply chain planning, supply chain integration, value chain management and order management (BPR).
References
- Adam, N. R., J. W. M. Bertrand, D. C. Morehead, and J. Surkis. 1993. “Due Date Assignment Procedures with Dynamically Updated Coefficients for Multi-Level Assembly job Shops.” European Journal of Operational Research 68 (2): 212–27.
- Adrodegari, F., A. Bacchetti, R. Pinto, F. Pirola, and M. Zanardini. 2015. “Engineer-to-order (ETO) Production Planning and Control: An Empirical Framework for Machinery-Building Companies.” Production Planning and Control 26 (11): 910–32. doi:10.1080/09537287.2014.1001808.
- Alenezi, A., S. A. Moses, and T. B. Trafalis. 2008. “Real-time Prediction of Order Flowtimes Using Support Vector Regression.” Computers and Operations Research 35 (11): 3489–503. doi:10.1016/j.cor.2007.01.026.
- Alfieri, Arianna, Tullio Tolio, and Marcello Urgo. 2011. “A Project Scheduling Approach to Production Planning with Feeding Precedence Relations.” International Journal of Production Research 49 (4): 995–1020.
- Alfieri, Arianna, Tullio Tolio, and Marcello Urgo. 2012. “A two-Stage Stochastic Programming Project Scheduling Approach to Production Planning.” International Journal of Advanced Manufacturing Technology 62 (1): 279–90.
- Alfnes, Erlend, Jonathan Gosling, Mohamed Naim, and Heidi C. Dreyer. 2021. “Exploring Systemic Factors Creating Uncertainty in Complex Engineer-to-Order Supply Chains: Case Studies from Norwegian Shipbuilding First Tier Suppliers.” International Journal of Production Economics 108211, doi:10.1016/j.ijpe.2021.108211.
- Amaro, Graça, Linda Hendry, and Brian Kingsman. 1999. “Competitive Advantage, Customisation and a new Taxonomy for non Make-to-Stock Companies.” International Journal of Operations & Production Management 19 (4): 349–371.
- Ameri, Farhad, Dusan Sormaz, Foivos Psarommatis, and Dimitris Kiritsis. 2021. “Industrial Ontologies for Interoperability in Agile and Resilient Manufacturing.” International Journal of Production Research 60 (2): 420–441.
- Anthony, Robert Newton. 1965. Planning and control systems: a framework for analysis: Division of Research, Graduate School of Business Administration, Harvard University.
- Arredondo, F., and E. Martinez. 2010. “Learning and Adaptation of a Policy for Dynamic Order Acceptance in Make-to-Order Manufacturing.” Computers and Industrial Engineering 58 (1): 70–83. doi:10.1016/j.cie.2009.08.005.
- Aslan, B., M. Stevenson, and L. C. Hendry. 2012. “Enterprise Resource Planning Systems: An Assessment of Applicability to Make-To-Order Companies.” Computers in Industry 63 (7): 692–705. doi:10.1016/j.compind.2012.05.003.
- Aslan, B., M. Stevenson, and L. C. Hendry. 2015. “The Applicability and Impact of Enterprise Resource Planning (ERP) Systems: Results from a Mixed Method Study on Make-To-Order (MTO) Companies.” Computers in Industry 70: 127–43. doi:10.1016/j.compind.2014.10.003.
- Azevedo, A. L., and J. P. Sousa. 2000. “Component-based Approach to Support Order Planning in a Distributed Manufacturing Enterprise.” Journal of Materials Processing Technology 107 (1-3): 431–8. doi:10.1016/S0924-0136(00)00680-4.
- Baker, Kenneth R. 1984. “Sequencing Rules and due-Date Assignments in a job Shop.” Management Science 30 (9): 1093–104.
- Bector, C. R., Y. P. Gupta, and M. C. Gupta. 1988. “Determination of an Optimal Common due Date and Optimal Sequence in a Single Machine job Shop.” International Journal of Production Research 26 (4): 613–28.
- Bender, J., and J. Ovtcharova. 2021. “Prototyping Machine-Learning-Supported Lead Time Prediction Using AutoML.” Paper presented at the Procedia Computer Science.
- Bertrand, J. W. M. 1983. “The Effect of Workload Dependent due-Dates on job Shop Performance.” Management Science 29 (7): 799–816.
- Bertrand, J. W. M., and Dennis R Muntslag. 1993. “Production Control in Engineer-to-Order Firms.” International Journal of Production Economics 30: 3–22.
- Bıçakcı, P. S., and İ Kara. 2019. “Order Acceptance and Scheduling Problem: A Proposed Formulation and the Comparison with the Literature.” Advances in Intelligent Systems and Computing 971: 310–316.
- Bıçakcı, P. S., İ Kara, and M. Sağır. 2021. “Single-Machine Order Acceptance and Scheduling Problem Considering Setup Time and Release Date Relations.” Arabian Journal for Science and Engineering 46 (2): 1549–59. doi:10.1007/s13369-020-04759-1.
- Bookbinder, James H, and Afzal Ibn Noor. 1985. “Setting job-Shop due-Dates with Service-Level Constraints.” Journal of the Operational Research Society 36 (11): 1017–26.
- Brachmann, R., and R. Kolisch. 2021. “The Impact of Flexibility on Engineer-to-Order Production Planning.” International Journal of Production Economics 239, doi:10.1016/j.ijpe.2021.108183.
- Buer, Sven-Vegard, Jan Ola Strandhagen, and Felix TS Chan. 2018. “The Link Between Industry 4.0 and Lean Manufacturing: Mapping Current Research and Establishing a Research Agenda.” International Journal of Production Research 56 (8): 2924–40.
- Buer, Sven-Vegard, Jo Wessel Strandhagen, Jan Ola Strandhagen, and Erlend Alfnes. 2016. “Strategic fit of Planning Environments: Towards an Integrated Framework.” Paper presented at the International Conference on information systems, Logistics and supply chain.
- Calosso, T., M. Cantamessa, D. Vu, and A. Villa. 2003. “Production Planning and Order Acceptance in Business to Business Electronic Commerce.” International Journal of Production Economics 85 (2): 233–49. doi:10.1016/S0925-5273(03)00112-9.
- Cannas, Violetta Giada, and Jonathan Gosling. 2021. “A Decade of Engineering-to-Order (2010-2020): Progress and Emerging Themes.” International Journal of Production Economics 241: 108274.
- Cannas, Violetta G, Jonathan Gosling, Margherita Pero, and Tommaso Rossi. 2019. “Engineering and Production Decoupling Configurations: An Empirical Study in the Machinery Industry.” International Journal of Production Economics 216: 173–89.
- Cannas, Violetta G, Jonathan Gosling, Margherita Pero, and Tommaso Rossi. 2020. “Determinants for Order-Fulfilment Strategies in Engineer-to-Order Companies: Insights from the Machinery Industry.” International Journal of Production Economics 228: 107743.
- Carvalho, A. N., F. Oliveira, and L. F. Scavarda. 2015. “Tactical Capacity Planning in a Real-World ETO Industry Case: An Action Research.” International Journal of Production Economics 167: 187–203. doi:10.1016/j.ijpe.2015.05.032.
- Carvalho, A. N., F. Oliveira, and L. F. Scavarda. 2016. “Tactical Capacity Planning in a Real-World ETO Industry Case: A Robust Optimization Approach.” International Journal of Production Economics 180: 158–71. doi:10.1016/j.ijpe.2016.07.019.
- Cesaret, B., C. O g˘uz, and F. Sibel Salman. 2012. “A Tabu Search Algorithm for Order Acceptance and Scheduling.” Computers and Operations Research 39 (6): 1197–205. doi:10.1016/j.cor.2010.09.018.
- Chapman, S. N., J. R. T. Arnold, A. K. Gatewood, T. Arnold, and L. M. Clive. 2017. Introduction to Materials Management. Harlow: Pearson Education Limited.
- Cheng, T. C. E. 1986. “Optimal due-Date Assignment in a job Shop.” International Journal of Production Research 24 (3): 503–15.
- Cheng, T. C. E., and M. C. Gupta. 1989. “Survey of Scheduling Research Involving due Date Determination Decisions.” European Journal of Operational Research 38 (2): 156–66.
- Corti, D., A. Pozzetti, and M. Zorzini. 2006. “A Capacity-Driven Approach to Establish Reliable due Dates in a MTO Environment.” International Journal of Production Economics 104 (2): 536–54. doi:10.1016/j.ijpe.2005.03.003.
- Danese, Pamela, Margherita Molinaro, and Pietro Romano. 2018. “Managing Evolutionary Paths in Sales and Operations Planning: Key Dimensions and Sequences of Implementation.” International Journal of Production Research 56 (5): 2036–53.
- De Boer, R., J. M. J. Schutten, and W. H. M. Zijm. 1997. “A Decision Support System for Ship Maintenance Capacity Planning.” CIRP Annals - Manufacturing Technology 46 (1): 391–X82. doi:10.1016/s0007-8506(07)60850-6.
- de Man, Johannes Cornelis, and Jan Ola Strandhagen. 2018. “Spreadsheet Application Still Dominates Enterprise Resource Planning and Advanced Planning Systems.” IFAC-PapersOnLine 51 (11): 1224–9.
- Easton, F. F., and D. R. Moodie. 1999. “Pricing and Lead Time Decisions for Make-to-Order Firms with Contingent Orders.” European Journal of Operational Research 116 (2): 305–18. doi:10.1016/s0377-2217(98)00101-5.
- Ebadian, M., M. Rabbani, F. Jolai, S. A. Torabi, and R. Tavakkoli-Moghaddam. 2008. “A new Decision-Making Structure for the Order Entry Stage in Make-to-Order Environments.” International Journal of Production Economics 111 (2): 351–67. doi:10.1016/j.ijpe.2007.01.004.
- Ebadian, M., M. Rabbani, S. A. Torabi, and F. Jolai. 2009. “Hierarchical Production Planning and Scheduling in Make-to-Order Environments: Reaching Short and Reliable Delivery Dates.” International Journal of Production Research 47 (20): 5761–89. doi:10.1080/00207540802010799.
- Ebben, Mark JR, Erwin W Hans, and F. M. Olde Weghuis. 2005. “Workload Based Order Acceptance in job Shop Environments.” OR Spectrum 27 (1): 107–22.
- Eilon, Samuel, and I. G. Chowdhury. 1976. “Due Dates in job Shop Scheduling.” International Journal of Production Research 14 (2): 223–37.
- ElHafsi, M. 2000. “An Operational Decision Model for Lead-Time and Price Quotation in Congested Manufacturing Systems.” European Journal of Operational Research 126 (2): 355–70. doi:10.1016/s0377-2217(99)00294-5.
- Germs, R., and N. D. Van Foreest. 2013. “Order Acceptance and Scheduling Policies for a Make-to-Order Environment with Family-Dependent Lead and Batch Setup Times.” International Journal of Production Research 51 (3): 940–51. doi:10.1080/00207543.2012.693638.
- Ghiyasinasab, M., N. Lehoux, S. Ménard, and C. Cloutier. 2021. “Production Planning and Project Scheduling for Engineer-to-Order Systems- Case Study for Engineered Wood Production.” International Journal of Production Research 59 (4): 1068–87. doi:10.1080/00207543.2020.1717009.
- Gordon, Valery, Jean-Marie Proth, and Chengbin Chu. 2002. “A Survey of the State-of-the-art of Common due Date Assignment and Scheduling Research.” European Journal of Operational Research 139 (1): 1–25.
- Gosling, Jonathan, and Mohamed M Naim. 2009. “Engineer-to-order Supply Chain Management: A Literature Review and Research Agenda.” International Journal of Production Economics 122 (2): 741–54.
- Gourdon, Karin, and Christian Steidl. 2019. “Global value chains and the shipbuilding industry.”.
- Grabenstetter, D. H., and J. M. Usher. 2013. “Determining job Complexity in an Engineer to Order Environment for due Date Estimation Using a Proposed Framework.” International Journal of Production Research 51 (19): 5728–40.
- Grabenstetter, D. H., and J. M. Usher. 2014. “Developing due Dates in an Engineer-to-Order Engineering Environment.” International Journal of Production Research 52 (21): 6349–61. doi:10.1080/00207543.2014.940072.
- Grimson, J Andrew, and David F Pyke. 2007. “Sales and Operations Planning: An Exploratory Study and Framework.” The International Journal of Logistics Management 18 (3): 322–346.
- Hans, Erwin W, Willy Herroelen, Roel Leus, and Gerhard Wullink. 2007. “A Hierarchical Approach to Multi-Project Planning Under Uncertainty.” Omega 35 (5): 563–77.
- Hao, J., J. Yu, M. Wu, and X. Chen. 2013. “Customer-value-based Order Acceptance Policy in Make-to-Order Manufacturing.” Paper presented at the proceedings - 2013 International Conference on computational and information sciences, ICCIS 2013.
- Heard, E. L. 1976. “Due-dates and Instantaneous Load in the one-Machine Shop.” Management Science 23 (4): 444–50.
- Hegedus, M. G., and W. J. Hopp. 2001. “Due Date Setting with Supply Constraints in Systems Using MRP.” Computers and Industrial Engineering 39 (3-4): 293–305. doi:10.1016/S0360-8352(01)00007-9.
- Hemmati, S., M. Ebadian, and A. Nahvi. 2012. “A new Decision Making Structure for Managing Arriving Orders in MTO Environments.” Expert Systems with Applications 39 (3): 2669–76. doi:10.1016/j.eswa.2011.08.122.
- Hendry, L. C., and B. G. Kingsman. 1989. “Production Planning Systems and Their Applicability to Make-to-Order Companies.” European Journal of Operational Research 40 (1): 1–15. doi:10.1016/0377-2217(89)90266-X.
- Hendry, L. C., and B. G. Kingsman. 1993. “Customer Enquiry Management: Part of a Hierarchical System to Control Lead Times in Make-to-Order Companies.” Journal of the Operational Research Society 44 (1): 61–70. doi:10.1057/jors.1993.7.
- Hicks, C., and P. M. Braiden. 2000. “Computer-aided Production Management Issues in the Engineer-to-Order Production of Complex Capital Goods Explored Using a Simulation Approach.” International Journal of Production Research 38 (18): 4783–810. doi:10.1080/00207540010001019.
- Hicks, C., T. McGovern, and C. F. Earl. 2000. “Supply Chain Management: A Strategic Issue in Engineer to Order Manufacturing.” International Journal of Production Economics 65 (2): 179–90. doi:10.1016/S0925-5273(99)00026-2.
- Hicks, Chris, Tom McGovern, and Chris F Earl. 2001. “A Typology of UK Engineer-to-Order Companies.” International Journal of Logistics 4 (1): 43–56.
- Hing, M Mainegra, Aart van Harten, and P. C. Schuur. 2007. “Reinforcement Learning Versus Heuristics for Order Acceptance on a Single Resource.” Journal of Heuristics 13 (2): 167–87.
- Hopp, Wallace J, and Melanie L Roof Sturgis. 2000. “Quoting Manufacturing due Dates Subject to a Service Level Constraint.” Iie Transactions 32 (9): 771–84.
- Hsu, S. Y., and D. Y. Sha. 2004. “Due Date Assignment Using Artificial Neural Networks Under Different Shop Floor Control Strategies.” International Journal of Production Research 42 (9): 1727–45.
- Huang, Y. 2017. “Information Architecture for Effective Workload Control: An Insight from a Successful Implementation.” Production Planning and Control 28 (5): 351–66. doi:10.1080/09537287.2017.1288278.
- Hvolby, Hans-Henrik, and Kenn Steger-Jensen. 2010. “Technical and Industrial Issues of Advanced Planning and Scheduling (APS) Systems.” Computers in Industry 61 (9): 845–51.
- Iakymenko, Natalia, Anita Romsdal, Marco Semini, and Jan Ola Strandhagen. 2018. “Managing Engineering Changes in the Engineer-to-Order Environment: Challenges and Research Needs.” IFAC-PapersOnLine 51 (11): 144–51.
- Ioannou, G., and S. Dimitriou. 2012. “Lead Time Estimation in MRP/ERP for Make-to-Order Manufacturing Systems.” International Journal of Production Economics 139 (2): 551–63. doi:10.1016/j.ijpe.2012.05.029.
- Ivert, Linea Kjellsdotter, and Patrik Jonsson. 2011. “Problems in the Onward and Upward Phase of APS System Implementation: Why do They Occur?” International Journal of Physical Distribution & Logistics Management 41 (4): 343–363.
- Jahangirian, Mohsen, Tillal Eldabi, Aisha Naseer, Lampros K Stergioulas, and Terry Young. 2010. “Simulation in Manufacturing and Business: A Review.” European Journal of Operational Research 203 (1): 1–13.
- Kalantari, M., M. Rabbani, and M. Ebadian. 2011. “A Decision Support System for Order Acceptance/Rejection in Hybrid MTS/MTO Production Systems.” Applied Mathematical Modelling 35 (3): 1363–77. doi:10.1016/j.apm.2010.09.015.
- Kalpic, Brane, and Peter Bernus. 2002. “Business Process Modelling in Industry—the Powerful Tool in Enterprise Management.” Computers in Industry 47 (3): 299–318.
- Kapuscinski, R., and S. Tayur. 2007. “Reliable due-Date Setting in a Capacitated MTO System with two Customer Classes.” Operations Research 55 (1): 56–74. doi:10.1287/opre.1060.0339.
- Kingsman, B. G. 2000. “Modelling Input-Output Workload Control for Dynamic Capacity Planning in Production Planning Systems.” International Journal of Production Economics 68 (1): 73–93. doi:10.1016/S0925-5273(00)00037-2.
- Kingsman, B., L. Hendry, A. Mercer, and A. De Souza. 1996. “Responding to Customer Enquiries in Make-to-Order Companies Problems and Solutions.” International Journal of Production Economics 46-47: 219–31. doi:10.1016/0925-5273(95)00199-9.
- Kingsman, B. G., and A. Mercer. 1997. “Strike Rate Matrices for Integrating Marketing and Production During the Tendering Process in Make-to-Order Subcontractors.” International Transactions in Operational Research 4 (4): 251–7. doi:10.1111/j.1475-3995.1997.tb00081.x.
- Kingsman, B. G., I. P. Tatsiopoulos, and L. C. Hendry. 1989. “A Structural Methodology for Managing Manufacturing Lead Times in Make-to-Order Companies.” European Journal of Operational Research 40 (2): 196–209. doi:10.1016/0377-2217(89)90330-5.
- Kingsman, B., L. Worden, L. Hendry, A. Mercer, and E. Wilson. 1993. “Integrating Marketing and Production Planning in Make-to-Order Companies.” International Journal of Production Economics 30-31 (C): 53–66. doi:10.1016/0925-5273(93)90081-U.
- Konijnendijk, Paul A. 1994. “Coordinating Marketing and Manufacturing in ETO Companies.” International Journal of Production Economics 37 (1): 19–26.
- Křemen, Petr, and Zdeněk Kouba. 2011. “Ontology-driven Information System Design.” IEEE Transactions on Systems, Man, and Cybernetics, Part C (Applications and Reviews) 42 (3): 334–44.
- Kristensen, Jesper, and Patrik Jonsson. 2018. “Context-based Sales and Operations Planning (S&OP) Research.” International Journal of Physical Distribution & Logistics Management 48 (1): 19–46.
- Li, X., and J. A. Ventura. 2020. “Exact Algorithms for a Joint Order Acceptance and Scheduling Problem.” International Journal of Production Economics 223), doi:10.1016/j.ijpe.2019.107516.
- Liao, C. J., and H. T. Lin. 1998. “A Case Study in a Dual Resource Constrained job Shop.” International Journal of Production Research 36 (11): 3095–111. doi:10.1080/002075498192319.
- Lin, S. W., and K. C. Ying. 2013. “Increasing the Total net Revenue for Single Machine Order Acceptance and Scheduling Problems Using an Artificial bee Colony Algorithm.” Journal of the Operational Research Society 64 (2): 293–311. doi:10.1057/jors.2012.47.
- Lin, S. W., and K. C. Ying. 2015. “Order Acceptance and Scheduling to Maximize Total net Revenue in Permutation Flowshops with Weighted Tardiness.” Applied Soft Computing 30: 462–74. doi:10.1016/j.asoc.2015.01.069.
- Manavizadeh, N., A. Hasani Goodarzi, M. Rabbani, and F. Jolai. 2013. “Order Acceptance/Rejection Policies in Determining the Sequence in Mixed Model Assembly Lines.” Applied Mathematical Modelling 37 (4): 2531–51. doi:10.1016/j.apm.2012.06.012.
- Mello, Mario Henrique, Jonathan Gosling, Mohamed M Naim, Jan Ola Strandhagen, and Per Olaf Brett. 2017. “Improving Coordination in an Engineer-to-Order Supply Chain Using a Soft Systems Approach.” Production Planning & Control 28 (2): 89–107.
- Mestry, Siddharth, Purushothaman Damodaran, and Chin-Sheng Chen. 2011. “A Branch and Price Solution Approach for Order Acceptance and Capacity Planning in Make-to-Order Operations.” European Journal of Operational Research 211 (3): 480–95.
- Micale, R., C. M. La Fata, M. Enea, and G. La Scalia. 2021. “Regenerative Scheduling Problem in Engineer to Order Manufacturing: An Economic Assessment.” Journal of Intelligent Manufacturing, doi:10.1007/s10845-020-01728-1.
- Moher, David, Alessandro Liberati, Jennifer Tetzlaff, Douglas G Altman, and Prisma Group. 2009. “Preferred Reporting Items for Systematic Reviews and Meta-Analyses: The PRISMA Statement.” PLoS Medicine 6 (7): e1000097.
- Moses, Scott A. 1999. “Due Date Assignment Using Feedback Control with Reinforcement Learning.” Iie Transactions 31 (10): 989–99.
- Moses, Scott, Hank Grant, L. Gruenwald, and S. Pulat. 2004. “Real-time due-Date Promising by Build-to-Order Environments.” International Journal of Production Research 42 (20): 4353–75.
- Mourtzis, D., M. Doukas, K. Fragou, K. Efthymiou, and V. Matzorou. 2014. “Knowledge-Based Estimation of Manufacturing Lead Time for Complex Engineered-To-Order Products.” Paper presented at the Procedia CIRP.
- Mourtzis, D., M. Doukas, and E. Vlachou. 2016. “A Mobile Application for Knowledge-Enriched Short-Term Scheduling of Complex Products.” Logistics Research 9 (1): 1–17. doi:10.1007/s12159-015-0130-7.
- Mundt, C., and H. Lödding. 2020. Order Acceptance and Scheduling with a Throughput Diagram.” In IFIP Advances in Information and Communication Technology, 351-359.
- Nam, S., H. Shen, C. Ryu, and J. G. Shin. 2018. “SCP-Matrix Based Shipyard APS Design: Application to Long-Term Production Plan.” International Journal of Naval Architecture and Ocean Engineering 10 (6): 741–61. doi:10.1016/j.ijnaoe.2017.10.003.
- Nguyen, Su. 2016. “A Learning and Optimizing System for Order Acceptance and Scheduling.” International Journal of Advanced Manufacturing Technology 86 (5): 2021–36.
- Nguyen, S., M. Zhang, and M. Johnston. 2014. Enhancing Branch-and-Bound Algorithms for Order Acceptance and Scheduling with Genetic Programming.” In Lecture Notes in Computer Science (including subseries Lecture Notes in Artificial Intelligence and Lecture Notes in Bioinformatics), 124-136.
- Nguyen, S., M. Zhang, M. Johnston, and K. C. Tan. 2013. Learning reusable initial solutions for multi-objective order acceptance and scheduling problems with genetic programming.” In Lecture Notes in Computer Science (including subseries Lecture Notes in Artificial Intelligence and Lecture Notes in Bioinformatics), 157-168.
- O g˘uz, Ceyda, F. Sibel Salman, and Zehra Bilgintürk Yalçın. 2010. “Order Acceptance and Scheduling Decisions in Make-to-Order Systems.” International Journal of Production Economics 125 (1): 200–11.
- Olhager, J. 2003. “Strategic Positioning of the Order Penetration Point.” International Journal of Production Economics 85 (3): 319–29. doi:10.1016/S0925-5273(03)00119-1.
- Özdamar, L., and T. Yazgaç. 1997. “Capacity Driven due Date Settings in Make-to-Order Production Systems.” International Journal of Production Economics 49 (1): 29–44. doi:10.1016/S0925-5273(96)00116-8.
- Öztürk, A., S. Kayaligil, and N. E. Özdemirel. 2006. “Manufacturing Lead Time Estimation Using Data Mining.” European Journal of Operational Research 173 (2): 683–700. doi:10.1016/j.ejor.2005.03.015.
- Park, J., S. Nguyen, M. Zhang, and M. Johnston. 2014. Enhancing heuristics for order acceptance and scheduling using genetic programming.” In Lecture Notes in Computer Science (including subseries Lecture Notes in Artificial Intelligence and Lecture Notes in Bioinformatics), 723-734.
- Park, C., J. Song, J. G. Kim, and I. Kim. 1999. “Delivery Date Decision Support System for the Large Scale Make-to-Order Manufacturing Companies: A Korean Electric Motor Company Case.” Production Planning and Control 10 (6): 585–97. doi:10.1080/095372899232885.
- Parsaei, S., M. A. Keramati, F. Zorriassatine, and M. R. Feylizadeh. 2012. “An Order Acceptance Using FAHP and TOPSIS Methods: A Case Study of Iranian Vehicle Belt Production Industry.” International Journal of Industrial Engineering Computations 3 (2): 211–24. doi:10.5267/j.ijiec.2011.08.002.
- Pereira, Daniel Filipe, José Fernando Oliveira, and Maria Antónia Carravilla. 2020. “Tactical Sales and Operations Planning: A Holistic Framework and a Literature Review of Decision-Making Models.” International Journal of Production Economics 228: 107695.
- Philipoom, Patrick R, Loren Paul Rees, and Lars Wiegmann. 1994. “Using Neural Networks to Determine Internally-Set Due-Date Assignments for Shop Scheduling.” Decision Sciences 25 (5-6): 825–851.
- Piya, S., M. M. R. K. Khadem, and A. Shamsuzzoha. 2016. “Negotiation Based Decision Support System for Order Acceptance.” Journal of Manufacturing Technology Management 27 (3): 443–68. doi:10.1108/JMTM-04-2015-0023.
- Rafiei, H., and M. Rabbani. 2012. “Capacity Coordination in Hybrid Make-to-Stock/Make-to-Order Production Environments.” International Journal of Production Research 50 (3): 773–89. doi:10.1080/00207543.2010.543174.
- Ragatz, Gary L, and Vincent A Mabert. 1984a. “A Framework for the Study of due Date Management in job Shops.” International Journal of Production Research 22 (4): 685–95.
- Ragatz, Gary L, and Vincent A Mabert. 1984b. “A Simulation Analysis of due Date Assignment Rules.” Journal of Operations Management 5 (1): 27–39.
- Rahman, H. F., M. N. Janardhanan, and I. E. Nielsen. 2019. “Real-time Order Acceptance and Scheduling Problems in a Flow Shop Environment Using Hybrid Ga-PSO Algorithm.” IEEE Access 7: 112742–55. doi:10.1109/ACCESS.2019.2935375.
- Rahman, Humyun Fuad, Ruhul Sarker, and Daryl Essam. 2015. “A Real-Time Order Acceptance and Scheduling Approach for Permutation Flow Shop Problems.” European Journal of Operational Research 247 (2): 488–503.
- Rasti-Barzoki, M., and S. R. Hejazi. 2013. “Minimizing the Weighted Number of Tardy Jobs with due Date Assignment and Capacity-Constrained Deliveries for Multiple Customers in Supply Chains.” European Journal of Operational Research 228 (2): 345–57. doi:10.1016/j.ejor.2013.01.002.
- Robinson, K. R., and S. A. Moses. 2006. “Effect of Granularity of Resource Availability on the Accuracy of due Date Assignment.” International Journal of Production Research 44 (24): 5391–414. doi:10.1080/00207540600665810.
- Roman, D. B., and A. G. del Vallei. 1996. “Dynamic Assignation of due-Dates in an Assembly Shop Based in Simulation.” International Journal of Production Research 34 (6): 1539–54.
- Ruben, Robert A, and Farzad Mahmoodi. 2000. “Lead Time Prediction in Unbalanced Production Systems.” International Journal of Production Research 38 (7): 1711–29.
- Sawik, T. 2009. “Multi-objective due-Date Setting in a Make-to-Order Environment.” International Journal of Production Research 47 (22): 6205–31. doi:10.1080/00207540902810585.
- Scheuermann, Andreas, and Joerg Leukel. 2014. “Supply Chain Management Ontology from an Ontology Engineering Perspective.” Computers in Industry 65 (6): 913–23.
- Schneckenreither, M., S. Haeussler, and C. Gerhold. 2020. “Order Release Planning with Predictive Lead Times: A Machine Learning Approach.” International Journal of Production Research, doi:10.1080/00207543.2020.1859634.
- Seidmann, Abraham, and Milton L Smith. 1981. “Due Date Assignment for Production Systems.” Management Science 27 (5): 571–81.
- Semini, Marco, Dag E Gotteberg Haartveit, Erlend Alfnes, Emrah Arica, Per Olaf Brett, and Jan Ola Strandhagen. 2014. “Strategies for Customized Shipbuilding with Different Customer Order Decoupling Points.” Proceedings of the Institution of Mechanical Engineers, Part M: Journal of Engineering for the Maritime Environment 228 (4): 362–72.
- Shurrab, Hafez, Patrik Jonsson, and Mats I Johansson. 2020. “A Tactical Demand-Supply Planning Framework to Manage Complexity in Engineer-to-Order Environments: Insights from an in-Depth Case Study.” Production Planning & Control 33 (5): 462–479.
- Silva, Yuri Laio TV, Anand Subramanian, and Artur Alves Pessoa. 2018. “Exact and Heuristic Algorithms for Order Acceptance and Scheduling with Sequence-Dependent Setup Times.” Computers & Operations Research 90: 142–60.
- Slotnick, Susan A. 2011. “Order Acceptance and Scheduling: A Taxonomy and Review.” European Journal of Operational Research 212 (1): 1–11.
- Smith, C. H., E. D. Minor, and H. J. Wen. 1995. “Regression-based due Date Assignment Rules for Improved Assembly Shop Performance.” International Journal of Production Research 33 (9): 2375–85.
- Stavrulaki, Euthemia, and Mark Davis. 2010. “Aligning Products with Supply Chain Processes and Strategy.” The International Journal of Logistics Management 21 (1): 127–151.
- Stevenson, M. 2006. “Refining a Workload Control (WLC) Concept: A Case Study.” International Journal of Production Research 44 (4): 767–90. doi:10.1080/00207540500338070.
- Stevenson, W. J. 2015. Operations Management. New York: McGraw-Hill Education.
- Stevenson, Mark, Linda C Hendry, and Brian G Kingsman. 2005. “A Review of Production Planning and Control: The Applicability of key Concepts to the Make-to-Order Industry.” International Journal of Production Research 43 (5): 869–98.
- Strandhagen, Jo Wessel, Sven-Vegard Buer, Marco Semini, Erlend Alfnes, and Jan Ola Strandhagen. 2020. “Sustainability Challenges and how Industry 4.0 Technologies Can Address Them: A Case Study of a Shipbuilding Supply Chain.” Production Planning & Control, 1–16.
- Studer, Rudi, V. Richard Benjamins, and Dieter Fensel. 1998. “Knowledge Engineering: Principles and Methods.” Data & Knowledge Engineering 25 (1-2): 161–97.
- Tatsiopoulos, I. P., and B. G. Kingsman. 1983. “Lead Time Management.” European Journal of Operational Research 14 (4): 351–8.
- Thevenin, S., N. Zufferey, and M. Widmer. 2015. “Metaheuristics for a Scheduling Problem with Rejection and Tardiness Penalties.” Journal of Scheduling 18 (1): 89–105. doi:10.1007/s10951-014-0395-8.
- Thomé, Antônio Márcio Tavares, Luiz Felipe Scavarda, and Annibal José Scavarda. 2016. “Conducting Systematic Literature Review in Operations Management.” Production Planning & Control 27 (5): 408–20.
- Thürer, Matthias, George Huang, Mark Stevenson, Cristovao Silva, and Moacir Godinho Filho. 2012. “The Performance of Due Date Setting Rules in Assembly and Multi-Stage job Shops: An Assessment by Simulation.” International Journal of Production Research 50 (20): 5949–65.
- Thürer, M., and M. Stevenson. 2016. “Card-based Delivery Date Promising in Pure Flow Shops with Order Release Control.” International Journal of Production Research 54 (22): 6798–811. doi:10.1080/00207543.2016.1177672.
- Thürer, M., M. Stevenson, C. Silva, and M. Land. 2013. “Towards an Integrated Workload Control (WLC) Concept: The Performance of due Date Setting Rules in job Shops with Contingent Orders.” International Journal of Production Research 51 (15): 4502–16. doi:10.1080/00207543.2013.774485.
- Thürer, M., M. Stevenson, C. Silva, M. J. Land, L. D. Fredendall, and S. A. Melnyk. 2014. “Lean Control for Make-to-Order Companies: Integrating Customer Enquiry Management and Order Release.” Production and Operations Management 23 (3): 463–76. doi:10.1111/poms.12058.
- Tranfield, David, David Denyer, and Palminder Smart. 2003. “Towards a Methodology for Developing Evidence-Informed Management Knowledge by Means of Systematic Review.” British Journal of Management 14 (3): 207–22.
- Usman, Zahid, Robert IM Young, Nitishal Chungoora, Claire Palmer, Keith Case, and Jennifer A Harding. 2013. “Towards a Formal Manufacturing Reference Ontology.” International Journal of Production Research 51 (22): 6553–72.
- Van Foreest, N. D., J. Wijngaard, and T. Van Der Vaart. 2010. “Scheduling and Order Acceptance for the Customised Stochastic lot Scheduling Problem.” International Journal of Production Research 48 (12): 3561–78. doi:10.1080/00207540802448882.
- Wagire, Aniruddha Anil, Rohit Joshi, Ajay Pal Singh Rathore, and Rakesh Jain. 2021. “Development of Maturity Model for Assessing the Implementation of Industry 4.0: Learning from Theory and Practice.” Production Planning & Control 32 (8): 603–22.
- Wang, D., S. C. Fang, and T. J. Hodgson. 1998. “A Fuzzy due-Date Bargainer for the Make-to-Order Manufacturing Systems.” IEEE Transactions on Systems, Man and Cybernetics Part C: Applications and Reviews 28 (3): 492–7. doi:10.1109/5326.704596.
- Wang, Z., Y. Qi, H. Cui, and J. Zhang. 2019. “A Hybrid Algorithm for Order Acceptance and Scheduling Problem in Make-to-Stock/Make-to-Order Industries.” Computers and Industrial Engineering 127: 841–52. doi:10.1016/j.cie.2018.11.021.
- Wang, X., X. Xie, and T. C. E. Cheng. 2013. “A Modified Artificial bee Colony Algorithm for Order Acceptance in two-Machine Flow Shops.” International Journal of Production Economics 141 (1): 14–23. doi:10.1016/j.ijpe.2012.06.003.
- Wang, L., Z. Zhang, and Y. Yin. 2019. Order Acceptance and Scheduling Considering Lot-Spitting in seru Production System.” Paper presented at the IEEE International Conference on Industrial Engineering and Engineering Management.
- Watanapa, B., and A. Techanitisawad. 2005a. “A Genetic Algorithm for the Multi-Class Contingent Bidding Model.” OR Spectrum 27 (4): 525–49. doi:10.1007/s00291-004-0192-4.
- Watanapa, Bunthit, and Anulark Techanitisawad. 2005b. “Simultaneous Price and due Date Settings for Multiple Customer Classes.” European Journal of Operational Research 166 (2): 351–68.
- Watson, Richard T, and Jane Webster. 2020. “Analysing the Past to Prepare for the Future: Writing a Literature Review a Roadmap for Release 2.0.” Journal of Decision Systems 29 (3): 129–47.
- Wattanapornprom, W., and T. K. Li. 2013. The Merging of MPS and Order Acceptance in a Semi-Order-Driven Industry: A Case Study of the Parasol Industry.” In 2013 IEEE International Conference on Industrial Engineering and Engineering Management, 996-1001. New York: IEEE.
- Webster, Jane, and Richard T Watson. 2002. “Analyzing the Past to Prepare for the Future: Writing a Literature Review.” MIS Quarterly 26 (2): xiii–xxiii.
- Weeks, James K. 1979. “A Simulation Study of Predictable due-Dates.” Management Science 25 (4): 363–73.
- Wikner, Joakim, and Martin Rudberg. 2005. “Integrating Production and Engineering Perspectives on the Customer Order Decoupling Point.” International Journal of Operations & Production Management 25 (7): 623–641.
- Willner, Olga, Daryl Powell, Markus Gerschberger, and Paul Schönsleben. 2016. “Exploring the Archetypes of Engineer-to-Order: An Empirical Analysis.” International Journal of Operations & Production Management 36 (3): 242–264.
- Wu, G. H., C. Y. Cheng, H. I. Yang, and C. T. Chena. 2018. “An Improved Water Flow-Like Algorithm for Order Acceptance and Scheduling with Identical Parallel Machines.” Applied Soft Computing 71: 1072–84. doi:10.1016/j.asoc.2017.10.015.
- Wu, H. H., and J. Y. Liu. 2008. “A Capacity Available-to-Promise Model for Drum-Buffer-Rope Systems.” International Journal of Production Research 46 (8): 2255–74. doi:10.1080/00207540601039783.
- Wullink, Gerhard, A. J. R. M. Gademann, Erwin W Hans, and Aart van Harten. 2004. “Scenario-based Approach for Flexible Resource Loading Under Uncertainty.” International Journal of Production Research 42 (24): 5079–98.
- Xiao, Yi-Yong, Ren-Qian Zhang, Qiu-Hong Zhao, and Ikou Kaku. 2012. “Permutation Flow Shop Scheduling with Order Acceptance and Weighted Tardiness.” Applied Mathematics and Computation 218 (15): 7911–26.
- Yang, W., and R. Y. K. Fung. 2014. “An Available-to-Promise Decision Support System for a Multi-Site Make-to-Order Production System.” International Journal of Production Research 52 (14): 4253–66. doi:10.1080/00207543.2013.877612.
- Zennaro, I., S. Finco, D. Battini, and A. Persona. 2019. “Big Size Highly Customised Product Manufacturing Systems: A Literature Review and Future Research Agenda.” International Journal of Production Research 57 (15-16): 5362–85. doi:10.1080/00207543.2019.1582819.
- Zhang, R., and C. Wu. 2012. “A Double-Layered Optimisation Approach for the Integrated due Date Assignment and Scheduling Problem.” International Journal of Production Research 50 (1): 5–22. doi:10.1080/00207543.2011.571440.
- Zheng, Ting, Marco Ardolino, Andrea Bacchetti, and Marco Perona. 2021. “The Applications of Industry 4.0 Technologies in Manufacturing Context: A Systematic Literature Review.” International Journal of Production Research 59 (6): 1922–54.
- Zhong, Xueling, Jinwen Ou, and Guoqing Wang. 2014. “Order Acceptance and Scheduling with Machine Availability Constraints.” European Journal of Operational Research 232 (3): 435–41.
- Zijm, W. H. M. 2000. “Towards Intelligent Manufacturing Planning and Control Systems.” OR Spektrum 22 (3): 313–45. doi:10.1007/s002919900032.
- Zorzini, M., D. Corti, and A. Pozzetti. 2008. “Due Date (DD) Quotation and Capacity Planning in Make-to-Order Companies: Results from an Empirical Analysis.” International Journal of Production Economics 112 (2): 919–33. doi:10.1016/j.ijpe.2007.08.005.
- Zorzini, M., L. Hendry, M. Stevenson, and A. Pozzetti. 2008. “Customer Enquiry Management and Product Customization: An Empirical Multi-Case Study Analysis in the Italian Capital Goods Sector.” International Journal of Operations and Production Management 28 (12): 1186–218. doi:10.1108/01443570810919369.
- Zorzini, Marta, Mark Stevenson, and Linda C Hendry. 2012. “Customer Enquiry Management in Global Supply Chains: A Comparative Multi-Case Study Analysis.” European Management Journal 30 (2): 121–40.
Appendix
Table summarises papers excluded from the review because of their focus on operational DDS. The items of reporting for these papers are:
Focus, which identifies whether the paper focuses on assigning delivery dates (delivery date assignment/due date assignment/DDA) or assessing the feasibility of customer-specified delivery dates based on detailed scheduling (order acceptance and scheduling/OAS).
Production system (described in subsection 3.1).
Method/technique used in the paper for DDA or OAS.
Empirical nature (described in subsection 3.1).
Industrial context (described in subsection 3.1).