Abstract
Sawmills are key elements of the forest product industry supply chain, and they play important economic, social, and environmental roles. Sawmill production planning and control are, however, challenging owing to several factors, including, but not limited to, the heterogeneity of the raw material. The emerging concept of digital twins introduced in the context of Industry 4.0 has generated high interest and has been studied in a variety of domains, including production planning and control. In this paper, we investigate the benefits digital twins would bring to the sawmill industry via a literature review on the wider subject of sawmill production planning and control. Opportunities facilitating their implementation, as well as ongoing challenges from both academic and industrial perspectives, are also studied.
1. Introduction
The forest product industry is important in many economies. For instance, in Canada, it contributed to 1.1% of the nominal gross domestic product in 2019Footnote1 and employed 205,000 people in 2018.Footnote2 Similarly, the forest wood product industry as a whole supported 378,000 jobs in France in 2019.Footnote3 Emerging technologies and new organisation methods introduced in the context of Industry 4.0, among many goals, aim to improve process efficiency and resource consumption. Industry 4.0 is, therefore, expected to provide many benefits for companies and society as a whole. Oláh et al. (Citation2020), in particular, argue that Industry 4.0 may have a positive impact on environmental sustainability if public policies are implemented to align industrial and sustainable development goals.
Lumber production is one such activity that would, with the benefits of improved planning, convey important gains to the industry as a whole. Sawmills are, indeed, an important link in the forest-product supply chain, participating in the first transformation of stems and logs harvested at forest sites into lumber and other by-products. Sawed products, that is, lumber, have different dimensions and qualities. The by-products, that is, sawdust, chips, and bark can be used by other industries to produce energy, pulp and paper, and chemical compounds. This production process is divergent from co-production, meaning that several products and by-products are simultaneously obtained from the sawing of a single piece of raw material (Dumetz et al. Citation2019). This, along with the heterogeneity of the raw material (the logs have various shapes and qualities), complicates the management of sawmill production (Dumetz et al., “Planning and Coordination,” Citation2017).
A general overview of the forest-product and internal sawmill supply chains is presented in Figure . Because the supply chain might vary significantly from country to country (as well as from firm to firm), the figure shows a generic framework. Sawmills (2) are supplied by forest companies (1) with whole tree stems or wood logs. Before processing, this raw material is stored at the sawmill log yard (A), which is usually an exterior storage location. While the exact set of transformation operations taking place at a sawmill depends on its equipment and policies, in general, they can be divided into three sets (Dumetz et al., “Evaluating Order Acceptance Policies,” Citation2017). The first is the initial sawing of raw logs into green lumber and other by-products (B). These can later be dried (C). In contrast to the sawing process, which is a quick transformation of an individual log into lumber, drying of lumber takes several days and is performed in kilns, in batches of similar, if not identical, products. The third set agglomerates any finishing operation (D), for example, the surfacing or grading of logs. In addition to lumber, the transformation process produces other by-products, such as sawdust, chips, and bark. These by-products are used by various industries to produce energy and chipboards (3).
Figure 1. Overview of a forest product supply chain
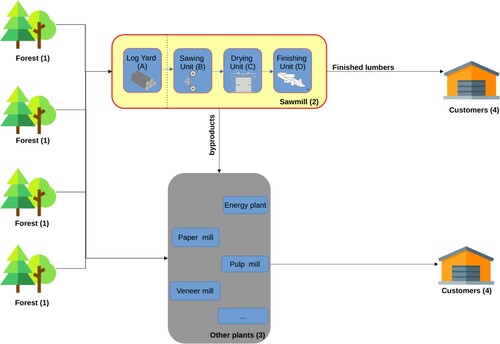
Like any industry subject to international competition, sawmills are subject to pressure to increase their competitiveness and optimise their production processes. Shahi (Citation2016), for example, touches upon the case of the Canadian forest industry which suffered in the past decades from the combined effects of globalisation, changes in the housing market, and a fluctuating currency. Climate change is expected to have an important impact on forests and timber supply. Brecka, Shahi, and Chen (Citation2018), for example, studied the impact of climate change on boreal forests and timber supply and conclude that the increased tree growth rate induced by a warmer climate and more CO2 in the atmosphere does not compensate for the increased tree mortality from forest disturbance such as insects, fire, and drought. This leads to a decrease in the net volume of wood. They also argue that the quality of timber is likely to decline because of the accelerated growth rate. Further, the benefits of wood products, particularly wood construction for CO2 sequestration, have been widely studied (Himes and Busby Citation2020).
This study aims to identify the benefits of digital twins (DT) in the sawmill industry, with a particular focus on operational production planning and control. Opportunities that would facilitate their implementation, as well as challenges and barriers to be tackled, are similarly considered. The potential and limits of DT technologies for industrial operations and production have been presented in various studies and literature reviews (Melesse, Pasquale, and Riemma Citation2020; dos Santos et al. Citation2021; Zheng, Lu, and Kiritsis Citation2021). However, studies on DT often have a very generic purpose or are application dependent. Therefore, there is a real need to deeply analyse the DT in the framework of the sawmill industry. Similarly, while there are several reviews in the scientific literature about the potential effects of Industry 4.0 technologies on the forest product supply chain (Müller, Jaeger, and Hanewinkel Citation2019), as well as the use of optimisation and operational research in the forest product industry (D'Amours, Rönnqvist, and Weintraub Citation2008), none of these focus on the use and potential benefits of DT to the sawmill industry in particular; they focus either on forest operations or other technologies. Therefore, this article first studies DT and sawmill production planning and control independently before discussing DT in the specific context of the sawmill industry.
While the exact definition and requirements of DT are still debated, it is widely accepted that it is a repository of the accumulated data of a product or production system (named the physical twin), augmented with analytic and predictive capabilities. It allows the gathering and coordination of all digital elements associated with the physical twin under a single framework. Numerous benefits are associated with the deployment of DT in manufacturing, such as an increased ability to predict failures or track asset performance.
The remainder of this paper is structured as follows. Section 2 presents the concept of digital twins as a general framework. Section 3 overviews the planning challenges in the sawmill industry and commonly proposed solutions from the existing literature for sawmill production planning and control. Section 4 analyses the application of DT to the sawmill industry. We conclude the study in Section 5.
2. Digital twins
The concept of Industry 4.0 was introduced at the 2011 Hanover Fair as the first anticipated industrial revolution. It may be studied from many angles, including technology, societal, and organisational (Bril El-Haouzi and Valette Citation2021). Lasi et al. (Citation2014), for example, argue that Industry 4.0 may be defined by two development directions. First, a set of economic, political, and social changes, such as increased needs for production flexibility, resource efficiency, and product personalisation change the industry paradigm. The second is the development of new technologies that respond to these needs. These include the Internet of Things and Cyber-Physical Production Systems, characterised by their modularity and flexibility. Among the frameworks developed in the context of Industry 4.0, DT has raised huge expectations. Various functions have been proposed for DT in the context of production, such as product design, health monitoring, and process control or production planning and scheduling. Aivaliotis, Georgoulias, and Chryssolouris (Citation2019), for example, proposed a method based on a digital twin to estimate the remaining useful life of equipment and plan maintenance. Wang et al. (Citation2019) proposed a DT model, based on digital models, including multiphysics simulations, data analytic and a knowledge base, for rotating machinery fault diagnosis. Liu et al. (Citation2019) proposed a DT-driven methodology, based, among other techniques, on simulation and optimisation to rapidly design manufacturing systems. Kousi et al. (Citation2019) exposed a shop floor DT infrastructure to coordinate robots and human workers to plan the robots' actions in this complex and uncertain environment. West et al. (Citation2021) presented 10 case studies where DT is used to support decision-making. In particular, two case studies expose the use of a DT to schedule operations for make-to-order wooden construction component factories and reduce waste production. Wang and Wang (Citation2019) discussed DT to support waste electrical and electronic equipment recycling or remanufacturing because such a process requires precise product knowledge. Zhou et al. (Citation2020) proposed a knowledge-driven digital twin framework with various applications, including production scheduling through a simulation-optimisation approach.
The definition and characterisation of DT have evolved as part of active research on Industry 4.0 and its technologies. In particular, no definition has been widely accepted as a reference to fully characterise DT, and numerous concurrent or field-specific definitions have been proposed. Semeraro et al. (Citation2021) propose a literature review of these definitions, and summarised them in the following general definition:
A set of adaptive models that emulate the behavior of a physical system in a virtual system getting real time data to update itself along its life cycle. The digital twin replicates the physical system to predict failures and opportunities for change to prescribe real-time actions for optimizing and/or mitigating unexpected events by observing and evaluating the operating profile system.
According to this definition, a DT contains at least two essential elements. First, the data describing the current and past states of the system. This data representation is updated in real time from data gathered from various sources, including advanced sensing technologies. Second, a set of models provides DT with analytical and predictive capabilities, making it a powerful decision support tool and allowing it to control the physical twin to some extent. Indeed, a DT should be more than a simple virtual representation of the physical twin, that is, more than a digital shadow (Bergs et al. Citation2021). To achieve the various functions expected from DT, these models should be varied, for example, predictive or optimisation models. While their exact natures may vary, their adaptive, or better, self-adaptive capabilities are crucial. A DT is expected to follow its physical twin along its entire life cycle – from its conception to its end of life. The state, usage conditions, and environment of the physical twin will change in ways that are impossible to predict as early as in DT implementation. To remain useful, DT models will, therefore, have to be continuously updated. dos Santos et al. (Citation2021) argue that while the use of simulations-based DT presents several advantages, they often require an auxiliary system to support decision-making and present problems in term of both the validity of the model through time and the computational requirements involved.
Owing to its real-time monitoring capabilities of the product or system and the induced precise virtual representation, DT may be suitable for short-term, online, or operational decision-making. By providing a precise replica of the system behaviour, it can also be used to support other planning levels. In particular, when implemented at the very beginning of a sawmill life cycle, DT can intervene during the sawmill conception and update phases, which are strategic or tactical decisions. However, the granularity of the data representation and analysis varies depending on DT usage. This also applies to the actual availability of data, as well as to the time available for analysis. All these factors may lead to different model choices depending on the anticipated use of DT.
DT has been studied from both a product point of view and a production system point of view, at various scales (Ding et al. Citation2019b). Tao et al. (Citation2019), for example, proposed a DT-driven framework to support the design of consumer products, while Ding et al. (Citation2019a) proposed a DT-based cyberphysical production system as a step toward smart manufacturing. DT of products may be used, among other things, to bridge all design, production, and use phases, shortening the time to market and allowing continuous improvement of the product by monitoring its usage condition and health in real time. Similarly, the production system DT allows the simulation and design of the shop floor layout, as well as the monitoring of production operations. Therefore, both products and shop floor DT follow their respective physical twins throughout their entire life cycle. While these life cycles are mostly independent, products and shop floor DT should at the very least interact during the production phase (Figure ), to control production and gather data to improve later plans (El Haouzi Citation2017).
Figure 2. Life cycles of products and processes
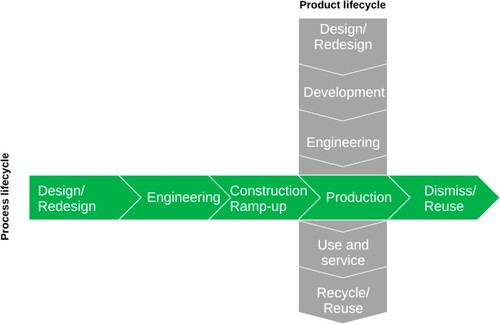
In particular, a general discussion of the nature, interest, and feasibility of using manufacturing system DT models as decision support tools can be found in Savolainen and Knudsen (Citation2021). Several important points are retained here. First, they mentioned the necessity for the various simulation models included in the DT to run faster than real time. This can be extended to any model included in the DT, whose task is to infer information using data from the physical twin to support decision-making. Such models have the objective of inferring information for a time window in the future of the system. Such a need for fast, computationally intensive models is acute when multiple scenarios have to be evaluated, for example, in a simulation-optimisation procedure (Xu et al. Citation2015). Second, while they recognise the gap between DT as often described in the literature and what would actually be realistic by current technology standards, they argue that companies would benefit from preemptively investing in cost-efficient and reliable data collection and storage technologies and base the development of DT systems on existing technologies rather than investing massive amounts in new ‘visionary’ systems. As a consequence, the DT components should be modular to allow for gradual improvement and completion.
Considering the preceding discussion, we focus our analysis on the DT of the manufacturing system, that is, sawmill DT during the production phase. In particular, in what follows, we consider a DT as a decision support system with the following three capabilities:
Descriptive capabilities, based on data describing the current and past states of the manufacturing system, including health monitoring and production data, updated in real time.
Predictive capabilities based on models able to infer from these raw data future states of the system and production, according to various scenarios. Such models may be physics-based simulation models, machine learning (ML) models, or based on any other relevant technology but should be able to generate predictions fast enough for decision-making purposes.
Prescriptive capabilities, to support decision-making in the real world by prescribing optimised plans to mill managers. The prescription process may use predictive and optimisation models in a simulation optimisation framework or use single models combining both optimisation and prediction simultaneously; however, it should be fast enough to support real-time, or at least operational, decision-making and allow fast reaction to unexpected events.
3. Production planning and control in the sawmill industry
As stated in the introduction, the objective of this study is to identify the potential use of DT for production planning and control in the sawmill industry. Therefore, we propose a literature review on general planning problems from the sawmill industry and their challenges, as well as solution methods commonly proposed in the field.
3.1. Methodology
For the purpose of this review, we used the snowballing systematic methodology (Wohlin Citation2014) as follows: the first set of articles, called seeds, was initially gathered. Other articles of interest were collected by backward and forward snowballing. Backward snowballing comprises articles collected from a reference list of seeds and previously gathered articles. Forward snowballing comprises selected articles being referenced in Google Scholar as citing articles already gathered (in the seed or otherwise). We selected articles with relevant titles as priority. Then, we evaluated articles with less relevant titles for which the author was known to have contributed to other selected works, as recommended by Wohlin (Citation2014). After this first selection, we evaluated articles based on their abstracts and then based on a full read of the retained articles. Only one round of backward snowballing and one round of forward snowballing were performed as the number of collected articles was judged to be sufficient.
The seed, which contains 50 articles, was built from searching the keywords ‘sawmill’ and (‘planning’ or ‘production control’ or ‘scheduling’ or ‘sequencing’) in the IEEE, Web of Science, Science Direct, and Scopus article databases. For Science Direct, the terms were searched only in the title, abstract, and authors' keywords of the articles. The search on Web of Science also considered the field Keywords Plus, and the search on IEEE the field indexing terms. These fields define additional keywords that are not necessarily present in the author's keyword list. The remaining snowballing procedure was performed using Google Scholar. In total, 133 documents studying planning problems in the sawmill industry were gathered and used as such in a preliminary analysis. Some of the most relevant papers in this article are further detailed in the following subsections. Only articles in English were considered; however, no limitations were set on the publication date. Additionally, conference and journal articles, Ph.D. theses, and Master's theses were added when considered of interest. Articles were only kept, however, when the full text was accessible through Google Scholar or through university access.
3.2. Preliminary analysis
Figure presents the publication dates distribution of the articles. While no filter has been applied on the publication date, most of these articles are fairly recent. In particular, 75 articles have been published since 2011 onward, the date of the Hanover fair which established the concept of Industry 4.0.
Figure 3. Distribution of the number of published articles per publication dates from 1980 and before to 2020.
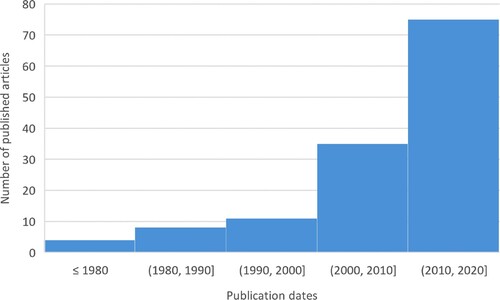
Figure shows the distribution of the authors' countries in the gathered articles. As can be seen, this field is vastly dominated by Canadian researchers, who contributed to nearly half of the gathered articles. The fact that the federal government, provinces, and territories own 90% of Canadian forests and manage the allocation of these resources to the industry may incentivise public research. Other North and South American countries, that is, Chile, the United States of America, and Argentina contribute to 30% of the gathered documents. In contrast, European countries only contribute to approximately 18% of the gathered articles despite the importance of the sector on the continent.
Figure 4. Pie chart of the nationalities of author institutions for the articles gathered during the literature review.
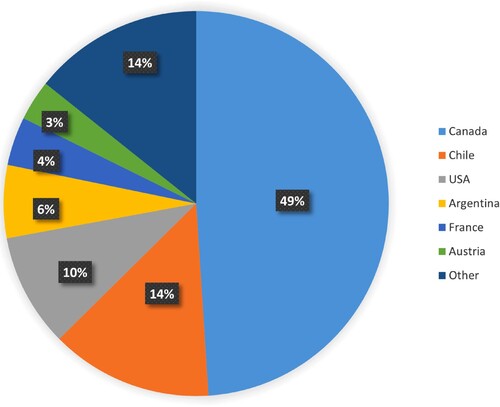
The keywords of the selected articles were gathered and summarised as a co-occurrence graph using VOSviewer software and reshaped using Gephi for clarity. The results are shown in Figure . Only keywords present in more than three articles are presented. In addition, keyword spelling was homogenised. In particular, American spelling was used, and special characters such as ‘−’ were removed. A link between two nodes denotes the co-occurrence of the associated keywords in an article. The thickness of a link is proportional to the number of articles in which the linked keywords co-occur. The size of a node corresponds to the number of articles in which the associated keyword occurs. As can be seen, numerous keywords correspond to either field-related terms or planning problem-solving methods. ‘Sawmills,’ ‘cutting pattern,’ or ‘forestry,’ for example, are all keywords specific to this industry. Similarly, while not being specific to the forest product industry, ‘production planning,’ ‘supply chain management,’ and ‘tactical planning’ are expected from the type of problem considered. Concerning problem solution methods, both ‘optimization’ and ‘simulation,’ are frequent keywords. They occurred in 17 and 14 articles, respectively. For comparison, ‘sawmill’ and ‘production planning,’ which are expected from the seed search string, are keywords in 22 and 16 articles, respectively. Specific optimisation methods are also frequent. ‘Linear programming’ is included in 11 article keyword lists, and ‘mixed integer programming’ in 7.
3.3. Planning in the sawmill industry
This section introduces planning concepts in the sawmill industry.
3.3.1. Planning levels
Planning in the forest wood industry is traditionally divided into four hierarchical levels: strategic, tactical, operational, and online (Rönnqvist Citation2003; D'Amours, Rönnqvist, and Weintraub Citation2008).
The strategic level refers to problems with a long planning horizon, typically more than five years. Examples of strategic problems in the sawmill industry are planning the creation of new mills, their emplacement, or the expansion of an existing mill. Troncoso and Garrido (Citation2005) proposed the use of a mixed-integer programming model to solve three such strategic problems in the forest industry, including transformation facility locations.
Tactical planning involves mid-term operations. Rönnqvist (Citation2003) considers them as having a time horizon from six months to five years. However, some authors present tactical problems with shorter time horizons. For example, Lobos and Vera (Citation2016) considered a tactical-level problem with a tactical rolling time horizon of four months (optimising the monthly amount of log to purchase and labour to hire). An example of the tactical planning problem is the yearly planning of production and sales (Marier et al. Citation2014). As stated in D'Amours, Rönnqvist, and Weintraub (Citation2008), an important feature of tactical plans is that it serves as a bridge between strategic decisions and operational plans.
The operational level involves short-term decision problems. Rönnqvist (Citation2003) classified such problems as having a time horizon lower than six months. D'Amours, Rönnqvist, and Weintraub (Citation2008) additionally stressed the need for such problems to consider very short planning periods, from a few hours to one day at most, to be as close as possible to the fields operations. Daily production planning (Zanjani, Ait-Kadi, and Nourelfath Citation2010) and scheduling of drying operations (Huka, Rindler, and Gronalt Citation2020) are examples of operational problems.
Lastly, the online level is concerned with the immediate production process and operational reality. Rönnqvist (Citation2003) classified as such planning problems as having a horizon of less than a day, from truck dispatching to process control. Todoroki and Rönnqvist (Citation2002) presented an example of such a problem. This article proposed a solution to adjust the product price list online according to actual production. This price list is used by an optimiser to decide what sawing pattern is used for which log. The objective here is to fill an order list with minimal overproduction of unwanted products.
3.3.2. Global challenges
Several global challenges complicate planning in the forest product industry in general and in the sawing industry in particular. The first challenge arises because of the aforementioned nature of the sawing process. The sawing process is divergent and in co-production, and the production of a set of lumbers to fill an order results in the production of unwanted low-value products.
Second, difficulty arises in the uncertainties inherent to the sawmill production process. In addition to the uncertainty over demand, sawmills must consider uncertainties in supply and production output. Several factors make it more difficult to forecast sawmill production (whether for a single log or for several logs), such as the heterogeneity of raw material, and the presence of real-time optimisers in some sawmill machines. Such optimisers are solvers encapsulated in machines that choose the sawing pattern of each log in real time to maximise its return value. They perform local optimisation or may consider a simplified projection of future processing steps. Owing to time constraints, they are often based on heuristics. They further complicate forecasting by enabling further configuration of the equipment and by adding other variables in the process, such as the list of product values. While this effectively maximises the returned value from the available raw material, it complicates planning. The exact effect that changing machine configurations would have on the production as a whole is indeed difficult to assess because it could change the basket of products sawed from each log. This is especially true in the absence of historical data, for example, when introducing new products (Wery et al. Citation2014, Citation2018).
Another difficulty is the integration of sawmilling operations in the supply chain as a whole. In particular, forests, which provide logs supply to sawmills, have, in general, to be managed over extremely long time horizons to take into consideration at least one complete forest rotation. Such a forest rotation can take more than 80 years in cold countries such as Canada (D'Amours, Rönnqvist, and Weintraub Citation2008). Similarly, tactical forestry management can, for example, consider a horizon of 5–10 years (Marinescu and Maness Citation2010). As expressed by Marinescu and Maness (Citation2010), sawmill managers may find it difficult to integrate these data into their planning models because they consider much lower time horizons. Moreover, accumulating data over an 80-year period poses its own unique challenges.
In addition to economic objectives, it may be important for the forest product industry to balance social and environmental aspects when planning operations. This, of course, includes the sawmill operations. Indeed, this value chain is an important actor in forest management, which provides social and ecological services to society as a whole, for example, in terms of wildlife preservation, sightseeing, and carbon sequestration. Additionally, sawmills are important employers in some rural areas.
Among all the articles gathered, only five introduced such objectives. For example, in Boukherroub et al. (Citation2013a), Boukherroub et al. (Citation2013b), and Boukherroub et al. (Citation2015), the same authors proposed models based on goal programming techniques to simultaneously optimise economic, environmental, and social indicators, such as operation costs, greenhouse gas emissions, and local employment, respectively.
Dumont et al. (Citation2019) similarly proposed a method to integrate energy consumption costs into tactical sawmill production planning by adapting a previously existing model. More precisely, they adapted the linear programming model introduced in Marier et al. (Citation2014) to include the energy cost from production processes and heating or cooling the workplaces into the minimised objective function.
Despite these difficulties, a point often stressed in the literature is the interest in coordinating several elements of a sawmill external and internal supply chain. In particular, Rönnqvist et al. (Citation2015) considered the integrated management of the forest product value chain as an open problem in the industry. Several studies have demonstrated the benefit of such coordination. For example, Bajgiran, Zanjani, and Nourelfath (Citation2016) built a mixed-integer programming model to integrate simultaneously ‘harvesting, procurement, production, and sales activities in the lumber supply chain.’ The results were compared with those obtained from decoupled models, and a decrease of 11% was observed when planning was distributed into two models. A decrease of 84% was obtained when decoupling it into three models. Similarly, Troncoso et al. (Citation2015) compared a decoupled model where forest and mill operations are planned sequentially, and the forest planning only aims to maximise the net present value of timber, with an integrated model where both solutions aim to maximise the long-term profit of the company. In comparison to the decoupled model, which is presented as the traditional planning strategy in the forest value chain, the integrated model led to a higher profit for the company despite harvesting fewer trees. While both articles demonstrate the interest in forest-mill integration for planning in the value chain, these two models differ greatly in terms of the planning horizon. Bajgiran, Zanjani, and Nourelfath (Citation2016) only planned operations over a yearly planning horizon, which is too short to take silviculture operations into account, and the study focused on harvest operations, whereas Troncoso et al. (Citation2015) planned forestry operations over at least a full forest rotation. However, downstream operations are only planned over five years because demand becomes too uncertain after this horizon (Troncoso et al. Citation2015). Finally, several articles consider the problem of bucking tree stems into logs. While this problem can occur either in forests or sawmills, it is a divergent step that strongly affects further production and sawmill profits (Dems, Rousseau, and Frayret Citation2015; Vanzetti, Broz, Montagna, et al. Citation2019b).
An important, but seldom mentioned, challenge in the selected articles is the traceability of logs and products from forest to sawmills and throughout the sawing process. The diverging nature of the sawing process renders common discrete markings such as RFID tags ill-suited to track the origin of every lumber (Jover, Thomas, and Bombardier Citation2011). Nonetheless, Boukherroub et al. (Citation2015) mentioned the existence of a strong demand for such product traceability for better tracking of timber origins and carbon footprint calculations. Traceability is also motivated by government regulations. For example, the EU Timber Regulation, which is designed to fight illegal logging .Footnote4 Wood product traceability is, however, further complicated by the divergent nature of the sawing process.
3.3.3. Common planning problems in the scientific literature
The literature includes a wide array of planning problems taking place at sawmills, from the creation of production plans specific to various production units, to the vertical or horizontal integration of plans at various levels. A common problem is the planning of the primary sawing operations. Some authors refer to this as the cutting pattern problem (Vergara, Palma, and Sepúlveda Citation2015 or Palma and Vergara Citation2016) or the sawing stock problem (Pradenas et al. Citation2013). A common formulation of this problem is ‘what quantity of which class of logs to saw with which cutting pattern to optimise a given objective under constraints.’ However, the specific objectives and constraints vary from case to case. The common objectives are to maximise profits or minimise losses. For example, Kazemi Zanjani, Nourelfath, and Aït-Kadi (Citation2011) proposed a stochastic programming model to solve this problem under a 30d planning horizon with a cost minimisation objective. Huka and Gronalt (Citation2017) proposed a mixed-integer program to maximise the operation net revenue over a 10-week planning horizon and compared it with several real-life inspired heuristics. Vanzetti et al. (Citation2018) similarly proposed a mixed-integer linear programming model to maximise the net benefit over a 5d planning horizon, and proposed a preliminary step, which generates the cutting pattern used in the optimisation problem.
Other authors have proposed variations of this problem. For example, in Haberl et al. (Citation1991), Maturana, Pizani, and Vera (Citation2010) and Varas et al. (Citation2014), logs from a certain class are always cut with a pattern that maximises the expected yield over said class. In particular, they consider only the mix of raw materials to process to fulfil an order. They justified this approach as a way for sawmills to minimise waste. Conversely, authors such as Wery et al. (Citation2018) and Maturana, Pizani, and Vera (Citation2010) reported that some sawmills have automated the sawing of logs to the point where the cutting pattern used on a specific log is decided in real time by the hardware to maximise the output volume or value (marker (B) in Figure ). This limits the actions of the manager to the choice of the log mix to process, sawmill configuration, and product price list used by the hardware when optimisation by value is performed.
Ulterior production steps, supply, inventory, and sales management can also be included in these planning models with various levels of precision to plan the whole production of one or several sawmills.
Compared to sawing, fewer examples focus on drying (marker (C) in Figure ) and finishing (marker (D) in Figure ) operations planning and scheduling. For example, Huka, Rindler, and Gronalt (Citation2020) studied planning methods to minimise tardiness when scheduling drying operations over 14d. Vanzetti, Corsano, and Montagna (Citation2020) proposed a disjunctive programming model to plan these operations over 5d to either maximise the number of dried packages or minimise unused space. Marier, Gaudreault, and Noguer (Citation2021) proposed combining mixed-integer and constraint programming to minimise tardiness over a three to four weeks planning horizon. Theresia, Widyadana, and Wahjudi (Citation2019) proposed two linear programming models to successively assign lumbers to different drying facilities then to different kiln chambers to minimise production costs and chamber use.
Table lists articles studying these different planning problems.
Table 1. Examples of articles studying common planning problems from the sawing industry.
Similar to drying and finishing, the sawmill log yard (marker (A) in Figure ) has its own set of problems. For example, Beaudoin, LeBel, and Soussi (Citation2012) proposed the use of a discrete event simulation model to compare loader-to-truck allocation strategies and optimise truck unloading operations. Rathke, Huka, and Gronalt (Citation2013), on the other hand, studied the box assignment problem. This problem refers to the optimisation of log sorting and storage operations to minimise the transportation time.
The coordination of different production steps can be divided into two categories: centralised or distributed. When the planning is performed in a centralised manner, a single optimisation scheme, usually a single model, is proposed to optimise all operations simultaneously. Nonetheless, Gaudreault et al. (Citation2010) argue that more often than not, centralised planning models are ill-suited to the production of detailed operational plans because of the complexity of the underlying operations. The alternative, according to the authors, is to favour a distributed approach along with coordination mechanisms between the different production units.
Examples of centralised plans include Vanzetti, Broz, Corsano, et al. (Citation2019a), who proposed a mixed-integer linear programming model to maximise the sawmill profit from the primary breakdown of the logs into intermediate products and secondary breaking into final products which takes place after drying operations. Gaudreault et al. (Citation2011) proposed two approaches, based on either mixed-integer programming or constraint programming for the planning and scheduling of both drying and finishing operations. Singer and Donoso (Citation2008) proposed a single linear programming model to model and optimise both sawing and drying-finishing operations. More than proposing a framework to produce a feasible plan, their objective is to provide decision-makers with a method to measure the impact of focusing development on either one of these operations.
In contrast, several studies have proposed decentralised plans. In particular, the FORAC Research Consortium (Université Laval, QC, Canada) developed a simulation platform based on multiagent systems to model the interaction between different production units, suppliers, and clients. This platform is described in Frayret et al. (Citation2007). A wide range of problems were studied on this platform. For example, Cid Yáñez et al. (Citation2009) modelled coordination strategies with different push/pull decoupling points. Interestingly, they concluded that while moving this decoupling point upstream of the internal supply chain increases customer satisfaction, the divergent nature of the production process impairs the ability of the sawmill to maximise log value recovery. Gaudreault et al. (Citation2010) propose several coordination mechanisms between production planning agents and test them on the platform. In particular, they compared upstream planning, two-phase planning, and bottleneck-first planning. Upstream planning is a pure pull system in which agents plan their production iteratively starting with the agent closest to the customer and propagating demand upward the supply chain. In two-phase planning, a first planning round is performed, with demand information flowing upward, up to the first production agent, that is, the sawing agent. Then, in the second round, these agents propagate their production plan downstream, adapting them to demand information. Bottleneck-first planning is similar to two-phase planning, but the first round only starts from the drying operation agent, which is considered a bottleneck in the process because of the time required to dry timbers (Dumetz et al., “Planning and Coordination,” Citation2017).
3.4. Common resolution methods
The two most common solution methods encountered in this literature review are optimisation and simulation.
3.4.1. Optimisation
Optimisation methods, particularly operational research methods, have been extensively used in the forest product industry for decades (D'Amours, Rönnqvist, and Weintraub Citation2008; Rönnqvist et al. Citation2015). The literature proposes many use cases of such a model and an approach to solve sawmilling planning problems. For example, Donald, Maness, and Marinescu (Citation2001) proposed the use of linear programming to optimise monthly production planning at a sawmill. Gaudreault et al. (Citation2011) compared a mixed-integer programming model with a constraint programming model for the task of planning and scheduling drying and finishing operations. Considering the important size of the industrial problem they consider, they further proposed a search procedure to find a satisfying solution in a limited time. This problem of limited computing time is similarly stressed by Marier, Gaudreault, and Robichaud (Citation2014), who relate the implementation of a mixed-integer programming model to an industrial partner. A hard requirement was for the model to propose a solution in less than five minutes.
Resolution methods based on metaheuristics to solve these optimisation problems appear promising. Metaheuristics are a general family of methods used to find approximate solutions to complex problems within an acceptable time (Chopard and Tomassini Citation2018). In particular, such methods are considered in the literature when the problem is not convex and/or difficult to solve. For example, Huka, Rindler, and Gronalt (Citation2020) proposed several heuristics to solve a nonlinear programming problem to schedule drying operations. Interestingly, they proposed the use of a metaheuristic – a tabu search, Laguna (Citation2018) – to improve the heuristics results. Similarly, Caballero et al. (Citation2009) proposed the use of a metaheuristic – a scatter search procedure for multiobjective optimisation, Molina et al. (Citation2007) – to solve a nonlinear goal programming model to plan sawmill production and meet demand.
Table presents a summary of the metaheuristics and problems characteristics considered in the surveyed articles.
Table 2. Articles gathered during the literature review using metaheuristics.
Finally, the last use of optimisation models is the creation of plans under uncertainties. According to Zanjani, Nourelfath, and Ait-Kadi (Citation2013a), a common method used in the industry to deal with uncertainty is the use of deterministic optimisation models under a rolling horizon scheme. The optimisation model is solved for a long time horizon using the information available at the time. However, the recommendation (or plan) is not followed until the end of the planning horizon, but is computed again on a regular basis when new information becomes available. However, numerous studies have proposed using models that explicitly consider uncertainties. The two popular methods are stochastic programming and robust optimisation. Both of these have advantages and disadvantages. Table presents a summary of the articles using these methods gathered during the literature review. In particular, Varas et al. (Citation2014) note that stochastic programming requires knowledge of the probability distribution of events, which may not be easy to gather or estimate. Additionally, they may be difficult to understand for a sawmill manager and can be computationally intensive depending on the number of scenarios considered. Diverse decomposition methods and heuristics were , therefore, proposed (e.g. Zanjani, Ait-Kadi, and Nourelfath Citation2013; Zanjani, Nourelfath, and Ait-Kadi Citation2013b). The same authors also used simulation and Monte Carlo methods to generate scenarios, for example, Zanjani, Nourelfath, and Ait-Kadi (Citation2007). While stochastic optimisation requires modelling the probability distribution over scenarios, robust optimisation aims at providing a feasible plan despite uncertainty on some of the model parameters and does not require such probability modelling. However, Rönnqvist et al. (Citation2015) remarked that this may lead to conservative plans with high costs.
Table 3. Articles implementing specific optimisation methods to deal with uncertainty in the planning process.
Table presents a summary of the optimisation methods implemented by the surveyed articles to deal with uncertainty in the planning process.
3.4.2. Simulation
Simulation is becoming an increasingly accepted and important tool in the industry and has been widely used in the forest industry, for example, to identify bottlenecks or study the impact of upgrading production systems (Opacic, Sowlati, and Mobini Citation2018). Noticeably, Masood and Sonntag (Citation2020) classify simulation as an Industry 4.0 technology with high average benefit but low application complexity, making it particularly suitable for small and medium enterprises.
The sawmill industry has several sawmill simulators at its disposal, which can be used in decision processes. A particular interest of such simulators is that they can replace historical production data when they are unavailable, for example, when designing a new sawmill or dealing with unusual products. They can also produce unaggregated data on a log-to-log basis, alleviating production uncertainties.
Sawmill simulators are, therefore, used in several studies to generate data that are used to solve optimisation problems. For example, Maness and Norton (Citation2002) used a simulator to simulate the sawing of logs from X-ray scans. The simulator additionally emulates a sawing optimiser and selects the sawing pattern to maximise a set of values (Sohrabi Citation2013). These data were then fed into a linear programming model. However, this approach was criticised by Zanjani, Nourelfath, and Ait-Kadi (Citation2007), who considered it impractical to require X-ray scans for logs that will be sawed later. In this study, the sawmill simulator was used to simulate the sawing of logs present in a historical database. These simulation results were then used to generate different stochastic output scenarios and solve a stochastic programming optimisation problem.
Similarly, Wery et al. (Citation2018) proposed simulation-optimisation models, where the simulation and optimisation procedures were repetitively solved. The simulation steps generate scenarios that are evaluated and fed to a search procedure that determines the parameters of the next simulation. The sawmill simulator considers logs from a nonevolving database that is representative of two different harvesting areas. Simulation is particularly interesting in that setting because it allows the evaluation of new sawmill configurations in the absence of historical data.
A third use of simulation tools is evaluating and comparing different planning strategies. For example, Mendoza et al. (Citation1991) used a simulation model to evaluate the feasibility of a plan produced by an optimisation model. Dumetz et al. (Citation2015) consider a discrete event simulation model to compare various planning and order management strategies. Similarly, Dumetz et al. (Citation2021) used it to compare coordination strategies between tactical and operational plans, and Cid Yáñez et al. (Citation2009) proposed the use of a multiagent simulation platform to evaluate different push–pull decoupling points in a sawmill internal supply chain composed, among others, of a sawing agent, drying agent, and finishing agent.
Such a multiagent simulation setting similarly appears in numerous articles related to the sawmill industry, even when considering only planning problems at a single sawmill composed of several production units. Multiagent simulation originates from the distributed artificial intelligence field and, therefore, by nature, allows the implementation of a distributed planning strategy. According to Gaudreault et al. (Citation2010), this facilitates the creation of detailed models compared with the centralised approach, which, in general, cannot consider precise operational details. This appears to be of particular interest for a sawmill supply chain because of the divergent nature of several production steps that complicate planning.
Table lists articles using multi-agent simulation technologies to study production planning and control problems in the forest product industry and sawmills in particular.
Table 4. List of articles collected during the literature review using multi-agent simulation technologies.
Finally, sawmill simulators, like SawsimFootnote5 integrate an optimiser to emulate sawing lines integrating these. Such an optimiser can, for example, decide which primary or secondary cutting pattern to use on a log-per-log basis.
4. Digital twins for the sawmilling industry
While none of the papers discussed in the previous section mention sawmill DT, they highlight the existence of many data-intensive models that may constitute elementary components of DT, both in terms of predictive and optimisation capabilities.
As discussed in Section 2, in this study, DT is defined as a decision support system having descriptive, predictive, and prescriptive capabilities, with the capacity to optimise online and operational production planning and control (Figure ).
Figure 6. Outline of the components essential to a sawmill DT.
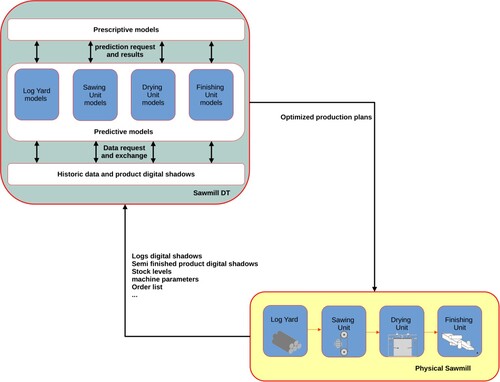
4.1. Descriptive capabilities
Owing to the highly heterogeneous nature of raw materials processed by sawmills, many technologies have been developed by the industry to gain insight into their nature throughout the transformation process. Methods are being developed to gain such information as early as from the standing tree, prior to harvest. Nguyen et al. (Citation2020), for example, propose using Lidar scans, often studied for their potential for forest inventory, to detect and classify defects on standing tree trunks. Similarly, the sawmill industry has been used for a long time, allowing external and internal scans of logs and lumber. Such scans are used in the industry for various purposes, including process optimisation, and different scanner technologies exist. In particular, laser scans of the exterior shape of logs have been used for a long time to scale and grade them ,Footnote6 measuring their length, diameter, and volume. X-ray scanners have been developed to allow for further detection of internal defects in logs. These 3D scans, whether they consider internal defects or only the log shape, are used in the industry to optimise the bucking of the stems into logs or the primary breakdown of logs into lumber. Such scans may also be used to simulate the sawing process in a nondestructive manner and generate useful data for various decision support problems. Examples of studies using such methods include (Maness and Norton Citation2002; Morneau-Pereira et al. Citation2014; Wery et al. Citation2014).
Similar to scans of the full log, scans of the lumbers measured after their primary breakdown may be used for process optimisation,Footnote7 or quality control. Staudhammer, Maness, and Kozak (Citation2007), in particular, reported the use of laser measurement of lumbers surfaces for quality inspection.
Overall, 3D scans, especially those obtained from X-ray technologies, may be considered informative enough to constitute digital shadows of the logs and products. However, this raises the issue of product traceability across several diverging production steps. Traceability is a major challenge in the forest product industry to limit the loss of information taking place at various steps of the forest product supply chain, allowing better guarantees on the product origin and characteristics and a better use of resources at the online production level (Jover et al. Citation2013). Various methods have been studied for this purpose. In particular, the use of X-ray scans appears promising (Skog, Jacobsson, and Lycken Citation2017). Furthermore, equipment manufacturers propose technologies to allow traceability from logs to lumbers based on X-ray scans and what they call a digital fingertip .Footnote8 Another method studied in the literature is the mass marking of logs with chemical markers (Jover et al. Citation2013).
4.2. Predictive capabilities
Many models are already able to benefit from industrial data such as logs and lumber scans to infer information about future transformation steps. In particular, modern sawmill hardware manufacturers provide simulators that integrate real pieces of software running their machines. These simulators can be linked by sawmill managers to simulate the entire production line and can, for example, be used to assess the effects of changes in the mill configuration. Therefore, they appear as important parts of a hypothetical mill DT, with the advantages of being already present, known by sawmill managers, and literally used by the equipment for local optimisation purposes. Interestingly, some of these software programs have the capacity to roughly estimate the results of future production steps in their optimisation and may, therefore, already be considered as DT of the production line to some extent. Similarly, sawmill simulators untied to specific equipment exist, such as SAWSIMFootnote9 or Optitek (Goulet Citation2006). Optitek, for example, has been used in several studies to generate data for optimisation problems. These studies include (Morneau-Pereira et al. Citation2014; Wery et al. Citation2018), who, however, mention the long computation time required by such simulations. Therefore, Morneau-Pereira et al. (Citation2014) ran simulations for only a subset of logs, whereas (Wery et al. Citation2018) proposed a simulation-optimisation framework to reduce the number of required simulations.
In addition to simulation models, a small subset of articles study the use of a second set of technology, that is, ML models and their interactions with simulation and optimisation. ML models are of particular interest in the context of DT. These are naturally data-driven models that leverage historical data. It is also possible to implement a self-update procedure to adapt these models to changes in the physical twin. This coordination between simulation and machine learning technologies was performed following various methods.
Morin et al. (Citation2020), in particular, propose using machine learning algorithms to train metamodels of sawmill simulators. They proposed the use of classic and well-understood ML algorithms, such as random forests or k-nearest neighbours (kNN), to make predictions based on know-how descriptors or logs. Although such sawing simulators are available to the forest wood industry, they are judged to be too slow for operational use, and typical use cases focus on tactical and strategic problems, which can also cause issues when decision-making involves tens of sawmills. Metamodels based on machine learning algorithms appear, therefore, as an interesting solution because they can approximate a simulator output and, in general, are designed to make fast predictions. However, the need for a certain amount of simulation or production data remains in order to train these ML metamodels. Recent studies have extended this study by exploring the use of various other machine learning algorithms, such as dissimilarity-based kNN (Chabanet et al. Citation2021; Chabanet, El-Haouzi, and Thomas Citation2021) and neural networks (Martineau et al. Citation2021).
In two other studies, Thomas, Choffel, and Thomas (Citation2008) and Thomas and Thomas (Citation2009) proposed the use of neural network models to simplify the simulation of a sawmill. Rather than completely replacing the simulator, the objective is to integrate ML inside the simulation to simplify it and speed it up. As with previous studies, the objective remains to alleviate the computational cost of the simulation.
4.3. Prescriptive capabilities
Many models have been proposed in the scientific literature to support decision-making in the sawmill industry, responding to a wide range of problems. In particular, operational research is extensively used by the forest product industry (Rönnqvist Citation2003) and may benefit from more precise data than that currently used (Morin et al. Citation2020). Such models are able to propose optimised production plans for sawmill managers and respond in particular to the sawing stock problem: which set of logs to cut, and with what equipment setting. This is particularly important for optimising raw material usage and limiting waste production. However, it is important to note that a common formulation of this problem requires, at the very least, to have logs sorted into classes based on shared properties. This is not always done efficiently in the industry despite the potential of such a strategy to reduce uncertainties and improve sawmill flexibility (Wery et al. Citation2012; Gil and Frayret Citation2016). While not all sawmills implement the required step to use such sawing operation planning models, other models are extensively used by Canadian sawmills, for example, to plan drying and finishing operations. Simulators are also extensively used to support decision-making at strategic and tactical levels rather than online and operational levels.
Similarly, such models require various data, such as operation costs, product prices, order lists, and expected operation outputs. This output, in particular, is not always available for new products or new machine settings. Simulations have been proposed to supplement these missing data. Nonetheless, this increases the computational cost. Additionally, the heterogeneity of sawmilling firms makes it difficult to implement some of these models for other specific cases. Adaptive frameworks have, however, been proposed to implement such models on a case-by-case basis (Kaltenbrunner, Huka, and Gronalt Citation2020). Simulations should also be used to verify the feasibility of the proposed plans.
The desirable modular structure of a sawmill DT makes the literature on multiagent simulation particularly interesting. A sawmill is composed of several distinct production units. Each one should naturally be attributed to its own set of potentially dependent predictive models. Some models may require the results of models from previous production steps as inputs. Therefore, mechanisms are required to coordinate them inside a single framework and optimise the production process globally, rather than providing a set of locally optimal solutions.
4.4. Discussion
Many building blocks of sawmill DT decision support systems already exist, either in the scientific literature or in the industry. Such a system may be expected to limit the loss of information between different sawmill production steps and improve short- to mid-term production plans by reducing uncertainties, particularly over the raw material and expected output. Better coordination between production units may also yield huge benefits, as shown by numerous studies based on multiagent simulations.
Additionally, while none of the reviewed articles mention the concept of DT, several other articles discuss the advantages of DT and other Industry 4.0 technologies in the forestry sector. Reitz, Schluse, and Roßmann (Citation2019), for example, envisioned a DT network to coordinate and share information between different forestry actors and their customers. This is proposed to deal with specific challenges, such as weak Internet connections in forests and the reluctance of stakeholders to share information. Kogler and Rauch (Citation2020) proposed a set of digital models, as a precursor of DT, to manage transportation from forests to mills considering uncertain natural events. Müller, Jaeger, and Hanewinkel (Citation2019) proposed the concept of digital forest, i.e. a digital representation of a forest. The representation would gather information about soil conditions, topography, and individual trees, using remote sensing, such as satellite, airborne, or LiDAR technologies. Therefore, a virtual forest is a digital shadow of the forest. To complement it with analytical capabilities would allow the creation of a forest DT. Coupling this with a sawmill or sawmills DT could realise a powerful decision support tool, making it possible to coordinate, simulate, and optimise integrated production plans. This further limits the sawmill uncertainty on the available raw material by gathering and transmitting information very early in the supply chain. Multiagent technologies will, once again, play a key role in ensuring a smooth and efficient coordination between these various DTs. Models have been proposed to manage sawmill supply at the operational or online level and respond to unexpected events (Amrouss et al. Citation2017; Kogler and Rauch Citation2020). By analysing the quality and shapes of logs as early as the harvest operations, or even before, and transferring this information to sawmills, managers would have far better guarantees on the raw material being processed and reduce production uncertainties. This would allow greater control of production and increase the sawmill flexibility.
Despite the high interest in these aforementioned ML, optimisation, and simulation models, a few difficulties exist regarding their application to the sawmill industry.
In particular, the computational efficiency, or lack thereof, of some sawmill simulators has been mentioned by several researchers (Morneau-Pereira et al. Citation2014; Wery et al. Citation2018; Morin et al. Citation2020). While the software running the machines and proposed as simulators by manufacturers are constrained to perform real-time optimisation, they may not find a real optimum. Additionally, many such optimisations should be performed to optimise the production plans. Whether these software programs would perform well enough for operational planning remains to be studied. Such studies should consider other works that implement simulation optimisation strategies (Wery et al. Citation2018). Similarly, stochastic optimisation requiring simulated data for only a representative subset of logs has been proposed (Zanjani, Nourelfath, and Ait-Kadi Citation2013a). Marier, Gaudreault, and Robichaud (Citation2014), however, mention the difficulty, in some cases, of solving even a deterministic mixed-integer programming model fast enough for industrial purpose. Additionally, while these model parameters may be changed easily to respond to changes in demand or log input mix, their global structure remains fixed, which may negatively impact the adaptive ability of the DT and its response to context and process changes. To propose models able to react to unexpected changes to some extent, as done in Amrouss et al. (Citation2017) to solve a real-time transportation problem in forestry, appears important. Similarly, some of the mentioned technologies remain out of reach for many sawmills. While it has been proposed, in other contexts, that sawmills may share such equipment (Chalayer Citation2019), this would complicate the implementation of DT by adding additional transportation steps. How to organise this, as well as the development of more affordable robust alternatives, would be an interesting research avenue.
For speeding up the simulation process, sawmill simulator ML metamodels appear to be particularly promising. However, more research remains to be done on both their structure, monitoring, usage, and limits. Sawmill simulator metamodels are, so far, specific to the sawmill and equipment configuration used to generate the labelled database for model building (training). Production processes taking place in the sawmill industry are, however, diverse and vary depending on country market contexts, company strategies, and equipment configuration. Numerous such models would, therefore, have to be trained and implemented, leading to high computational costs and modelling time. A second general challenge is the usual presence of concept drift in the industry. Concept drift refers to changes in the (theoretical) probability distribution generating data fed to the ML models. Several types of concept drifts have been identified and classified in the related literature, including class drift, covariate shift, and novel class appearance (Webb et al. Citation2016).
For example, some sawmills perform online optimisation of the cutting pattern process. This can be achieved by maximising the volume of the final products, or their ‘value.’ However, these values are not necessarily the real market price, but rather a set of command values used to favour the production of some products to meet demand. These command values can be changed depending on market conditions, sawmill order list, and actual production. Changing these parameters will lead to changes in the cutting pattern attributed to a log and, therefore, to the set of lumbers obtained. This is an example of class drift. Therefore, metamodels should either take command prices as input or be easily adapted and retrained when such a change occurs. Similarly, changes in the nature of the log supply, due to a change in the supplier or harvested area, will induce covariate drift, and the addition of new products to a sawmill catalogue will induce a class appearance type of drift.
These different types of drifts make it necessary to continuously adapt and update models used in the sawmill industry, or, in any industry, for that matter. This is particularly important for a DT following its physical twin throughout its entire life cycle. Therefore, approaches that facilitate the training and adaptation of ML models in the context of forest companies, such as transfer learning (Torrey and Shavlik Citation2010) and active learning (Settles Citation2009), are interesting avenues for further research. Transfer learning focuses on the transfer of knowledge learned from a previous task to new models for similar tasks. Alexopoulos, Nikolakis, and Chryssolouris (Citation2020) argued that in cases where DT implement fast and precise simulations, they may be used to quickly generate new synthetic labelled datasets and complement transfer learning algorithms. Similarly, active learning focuses on the guided construction of a training dataset to maximise the trained predictor performance with minimal cost.
Other specific challenges are presented by Sawmill storage areas. Nantel, Gaudreault, and Léger (Citation2020) studied, in particular, the potential of DT to maintain a geolocated inventory in a woodyard, using GPS technologies. Contrary to classic warehouses, these storage areas are placed outside, subject to the weather, and do not necessarily implement predetermined storage emplacements. The organisation of the woodyard was then left at the discretion of the operator. According to Nantel, Gaudreault, and Léger (Citation2020), current commercial GPS technologies are still insufficient to maintain a geolocated inventory of each package in the yard. However, they demonstrate that maintaining such an inventory would be possible by adding other information and not differentiating between packages of the same product or by implementing additional storage rules.
5. Conclusion
DT is, in the context of this study, a collection of various models and data updated in real time. They are more than a precise representation of the physical twin, but are also endowed with predictive and analytical capabilities. Therefore, they are expected to serve various functions such as production planning and control decision support tools.
Sawmills in particular would benefit from the development and deployment of DT. These would, indeed, allow the testing and optimisation of production plans, including optimising the parameters of embedded real-time optimisers. Owing to the divergent nature of sawmill production processes and the heterogeneity of the raw material, the impact of these parameters on the production as a whole is difficult to assess without historical data, which are not always accessible or require costly trials and errors. Additionally, by communicating with forest DT benefiting from new sensing technologies, they could reduce information loss taking place at several points in the forest product supply chain, both before and inside the sawmill.
The state of existing technologies and simulation tools makes the development of DT realistic for the sawmill industry. In particular, equipment manufacturers supply simulators based on real pieces of software running their machines. These simulations are therefore able to precisely model the behaviour of the production line, given sufficient data, and appear as important models to include in a DT. Traceability technologies based on chemical marking or X-ray scanners are being developed with promising results. X-ray-based production optimisation systems are already being commercialised, but they are too expensive for many sawmills. Finally, some countries have sustained active academic research on sawmill production planning and control. In particular, numerous planning optimisation methods have been studied, and specific coordination mechanisms based on multi-agent technologies have been proposed.
However, several challenges remain and need to be investigated to enable the implementation of sawmill DT. It is important to note that the heterogeneity of the forest-wood product supply chain structures, production processes, and raw material makes the development of a solution suitable for all cases improbable. A solution that responds to one sawmill requirement may not respond to another requirement. Therefore, to create flexible methodologies aimed at facilitating their development, it is important to propose finished implementations. Similarly, the adaptive capacities of sawmill DT models over time and their response to changes remain challenging and require further investigation. These models will, indeed, be subject to changes in both the physical twin and its environment caused by multiple factors ranging from variations in the properties of wood logs to tool wear. To efficiently coordinate models of various natures included in the DT will, additionally, generate its own challenges, although current research on multiagent technologies appears promising. These different models will not only respond to different needs, over various time horizons, and be related to different production or storage units, but will also be written in different programming languages, use data in a variety of formats gathered from numerous sensors (e.g. X-ray scanners, laser scanners, ultrasound, cameras), and have very different computation time requirements, ranging from a few milliseconds to several hours.
Disclosure statement
No potential conflict of interest was reported by the author(s).
Data availability statement
The authors confirm that the data supporting the findings of this study are available within the article.
Additional information
Notes on contributors
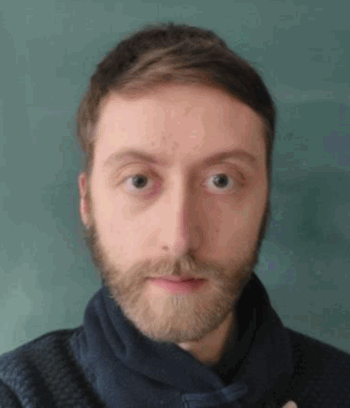
Sylvain Chabanet
Sylvain Chabanet is a Ph.D. student at the CRAN laboratory, Lorraine University. He was born in France in 1996 and graduated from both the Applied Mathematics Department of the Engineering School Ecole Normale Superieure des Mines de Nancy and the master M2MO of university Paris-Diderot in 2019. He started his Ph.D. at the CRAN in 2020, directed by Pr Hind Bril El-Haouzi and Pr Philippe Thomas. His research interests include the application of artificial intelligence, and in particular machine learning, to industrial problems. His Ph.D. focuses particularly on the usage of these methods for production planning and control in the sawmill industry.
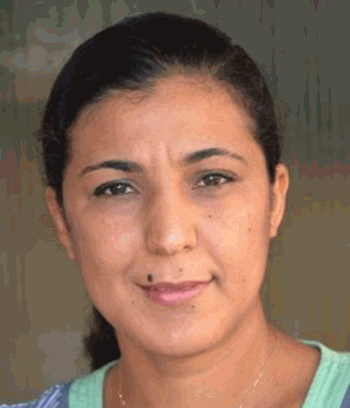
Hind Bril El-Haouzi
Hind Bril El-Haouzi is a full professor at the University of Lorraine where she teaches and conducts research in the field of Computer Engineering and Production Management Control. Since 2018, she is co-leader of the Sustainable Industrial System Engineering Research group (ISET) (about 60 people) at CRAN laboratory. She received her Ph.D. in Computer Science, Automatic Control, Production Engineering in 2008. She has a strong industrial experience in an international group as digitisation project leader where she worked for many years before joining the University of Lorraine. Her research deals with decision support and knowledge formalisation within the Intelligent Manufacturing context.
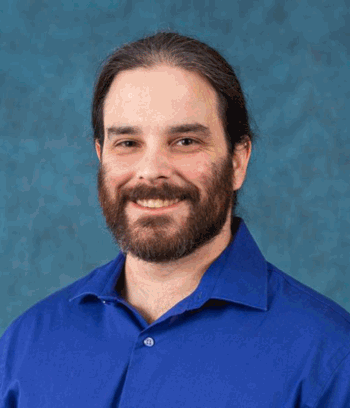
Michael Morin
Michael Morin is Assistant Professor at the Department of Operations and Decision Systems of Université Laval. He obtained his Ph.D. from the Department of Computer Science and Software Engineering of Université Laval in 2015. Afterwards, he completed two postdoctoral fellowships, one at Université Laval in 2016, and the second at the University of Toronto in 2017. He is an active member of multiple research groups including the FORAC Research Consortium (decision-making in the forest products industry), the CRISI Research Consortium (Industry 4.0 and its applications), and the CERMID Research Center (modelling, information and decision systems). His current research focuses on optimisation and machine learning for decision-making and decision systems. His main research interests include operations research, artificial intelligence, and the application of such systems to humanitarian, business, and industrial problems.
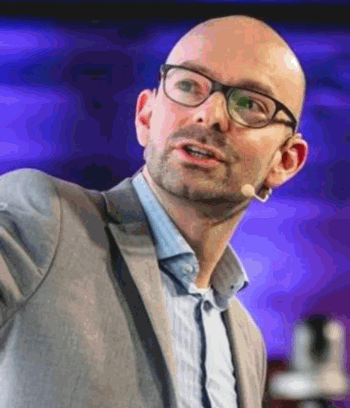
Jonathan Gaudreault
Jonathan Gaudreault is the director of CRISI, a research consortium dedicated to Industry 4.0 systems engineering. Over the last 15 years, he has led a number of key projects related to operations planning and scheduling, bringing together ideas from the artificial intelligence and operations research fields. He previously worked as an R&D manager in the software industry and was awarded as ‘One to watch’ by the Federation de l'Informatique du Québec (OCTAS award). Pr. Gaudreault and other researchers from the FORAC Research Consortium were recognised with the Brockhouse Canada Prize for Interdisciplinary Research in Science and Engineering, Canada's premier award for interdisciplinary research.
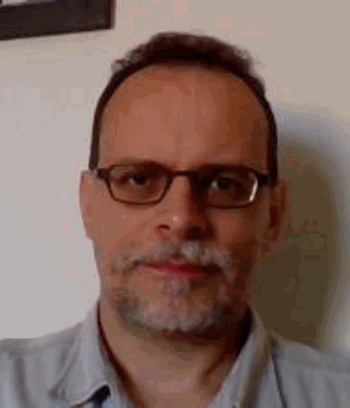
Philippe Thomas
Philippe Thomas was born in 1969 in France and received his Ph.D. from the University Henri Poincaré Nancy 1 in 1997 and his professorial thesis from the Lorraine University in 2014. He spent five years with the Systèmes et Transports Laboratory at the Technical University of Belfort-Montbéliard, where he studied model-based diagnosis using neural networks. He is currently Associate Professor and Researcher at the Centre de Recherche en Automatique de Nancy (CRAN-UMR7039), which is affiliated with the CNRS and Lorraine University. His research interests focus on the use of learning machines, and more particularly neural networks, in the production management domain. In particular, he collaborates with ACTA mobilier company to exploit a machine learning approach to improve quality in a lacquering process.
Notes
1 https://www.nrcan.gc.ca/our-natural-resources/forests-forestry/state-canadas-forests-report/how-does-forest-sector-contribut/indicator-gross-domestic-product/16556, last accessed in December 2021.
2 https://www.nrcan.gc.ca/our-natural-resources/forests-forestry/state-canadas-forests-report/forest-industry-contribute/16517, last accessed in December 2021.
3 https://agriculture.gouv.fr/infographie-la-filiere-foret-bois-en-france, last accessed in December 2021.
4 https://ec.europa.eu/environment/forests/timber_regulation.htm, last accessed on August 2021.
5 https://www.halcosoftware.com/software-1-sawsim, last accessed on December 2021.
6 https://library.fpinnovations.ca/media/FOP/Infonote2017N27.pdf, last accessed December 2021.
7 https://library.fpinnovations.ca/media/FOP/Infonote2017N27.pdf, last accessed December 2021.
8 https://microtec.eu/en/applications/all/smart-link-digital-fingerprint/, last accessed in December 2021.
9 https://www.halcosoftware.com/software-1-sawsim, last accessed on December 2021.
References
- Aivaliotis, P., K. Georgoulias, and G. Chryssolouris. 2019. “The Use of Digital Twin for Predictive Maintenance in Manufacturing.” International Journal of Computer Integrated Manufacturing 32 (11): 1067–1080.
- Alayet, C., N. Lehoux, L. LeBel, and M. Bouchard. 2013. “Planning and Control Model for A Forest Supply Chain.” In Proceedings of the 2nd International Conference on Operations Research and Enterprise Systems. Vol. 1: ICORES, 5–13. SciTePress.
- Alexopoulos, K., N. Nikolakis, and G. Chryssolouris. 2020. “Digital Twin-Driven Supervised Machine Learning for the Development of Artificial Intelligence Applications in Manufacturing.” International Journal of Computer Integrated Manufacturing 33 (5): 429–439.
- Alvarez, P. P., A. Espinoza, S. Maturana, and J. Vera. 2020. “Improving Consistency in Hierarchical Tactical and Operational Planning Using Robust Optimization.” Computers & Industrial Engineering139: 106112.
- Alvarez, P. P., and J. R. Vera. 2014. “Application of Robust Optimization to the Sawmill Planning Problem.” Annals of Operations Research 219 (1): 457–475.
- Amrouss, A., N. El Hachemi, M. Gendreau, and B. Gendron. 2017. “Real-Time Management of Transportation Disruptions in Forestry.” Computers & Operations Research 83: 95–105.
- Arabi, M. 2013. “An Optimization and Simulation Framework for Integrated Tactical Planning of Wood Harvesting Operations and Lumber Production.” Master's thesis, Université Laval.
- Baesler, F., and C. Palma. 2014. “Multiobjective Parallel Machine Scheduling in The Sawmill Industry Using Memetic Algorithms.” The International Journal of Advanced Manufacturing Technology 74 (5): 757–768.
- Bajgiran, O. S., M. K. Zanjani, and M. Nourelfath. 2014. “Integrated tactical planning in lumber sypply chain. Industrial and System Engineering Research Conference. Montreal, Canada. May 31–June 3, 2014.
- Bajgiran, O. S., M. K. Zanjani, and M. Nourelfath. 2016. “The Value of Integrated Tactical Planning Optimization in the Lumber Supply Chain.” International Journal of Production Economics 171: 22–33.
- Beaudoin, D., J.-M. Frayret, and L. LeBel. 2010. “Negotiation-based Distributed Wood Procurement Planning Within a Multi-firm Environment.” Forest Policy and Economics 12 (2): 79–93.
- Beaudoin, D., L. LeBel, and M. A. Soussi. 2012. “Discrete Event Simulation to Improve Log Yard Operations.” INFOR: Information Systems and Operational Research 50 (4): 175–185.
- Bergs, T., S. Gierlings, T. Auerbach, A. Klink, D. Schraknepper, and T. Augspurger. 2021. “The Concept of Digital Twin and Digital Shadow in Manufacturing.” Procedia CIRP 101: 81–84.
- Boukherroub, T., A. Ruiz, A. Guinet, and J. Fondrevelle. 2013a. “An Integrated Approach for the Optimization of the Sustainable Performance: A Wood Supply Chain.” IFAC Proceedings Volumes 46 (9): 186–191.
- Boukherroub, T., A. Ruiz, A. Guinet, and J. Fondrevelle. 2013b. An Integrated Approach for Sustainable Supply Chain Planning: The Case of Divergent Processes. Montreal, Canada: Centre Interuniversitaire de Recherche sur les Réseaux d'Entreprise, la Logistique et le Transport.
- Boukherroub, T., A. Ruiz, A. Guinet, and J. Fondrevelle. 2015. “An Integrated Approach for Sustainable Supply Chain Planning.” Computers & Operations Research 54: 180–194.
- Brecka, A. F., C. Shahi, and H. Y. Chen. 2018. “Climate Change Impacts on Boreal Forest Timber Supply.” Forest Policy and Economics 92: 11–21.
- Bril El-Haouzi, H., and E. Valette. 2021. “Human System Integration as a Key Approach to Design Manufacturing Control System for Industry 4.0 : Challenges, Barriers, and Opportunities.” In 17th IFAC Symposium on Information Control Problems in Manufacturing (INCOM), 6. Budapest: IFAC.
- Broz, D., N. Vanzetti, G. Corsano, and J. M. Montagna. 2019. “Goal Programming Application for the Decision Support in the Daily Production Planning of Sawmills.” Forest Policy and Economics 102: 29–40.
- Caballero, R., T. Gomez, J. Molina, O. Fosado, M. A. Leon, M. Garofal, and B. Saavedra. 2009. “Sawing Planning Using a Multicriteria Approach.” Journal of Industrial & Management Optimization 5 (2): 303.
- Chabanet, S., H. B. El-Haouzi, and P. Thomas. 2021. “Coupling Digital Simulation and Machine Learning Metamodel Through an Active Learning Approach in Industry 4.0 Context.” Computers in Industry133: 103529.
- Chabanet, S., P. Thomas, H. B. El-Haouzi, M. Morin, and J. Gaudreault. 2021. “A kNN Approach based on ICP Metrics for 3d Scans Matching: An application to the Sawing Process.17th IFAC Symposium on Information Control Problems in Manufacturing. Budapest, Hungary. June 2021.
- Chalayer, M. 2019. Les évolutions de la Scierie Française. Paris, France: l'Harmattan.
- Chopard, B., and M. Tomassini. 2018. An Introduction to Metaheuristics for Optimization. Cham, Switzerland: Springer.
- Cid, F., J. Frayret, A. Rousseau, and F. Léger. 2007. “Evaluation of Pull Strategies in Lumber Production Planning: A Case Stude. 19th International Conference on Production Research. Valparaiso, Chile. July, 2007.
- Cid Yáñez, F., J.-M. Frayret, F. Léger, and A. Rousseau. 2009. “Agent-based Simulation and Analysis of Demand-driven Production Strategies in the Timber Industry.” International Journal of Production Research 47 (22): 6295–6319.
- Cifuentes, N., G. Gatica, and R. Linfati. 2017. “Alinear Programming Model for the Parallel Non-related Machines Problem, in the Drying Area of a Chilean Sawmill.” Revista Facultad de Ingeniería 26 (46): 9–17.
- Corro, H., M. Singer, and P. Donoso. 2007. “Internal Supply Chain Management in the Chilean Sawmill Industry.” International Journal of Operations & Production Management 27: 524–541.
- D'Amours, S., M. Rönnqvist, and A. Weintraub. 2008. ��Using Operational Research for Supply Chain Planning in the Forest Products Industry.” INFOR: Information Systems and Operational Research 46 (4): 265–281.
- Dems, A., L.-M. Rousseau, and J.-M. Frayret. 2015. “Effects of Different Cut-to-Length Harvesting Structures on the Economic Value of a Wood Procurement Planning Problem.” Annals of Operations Research 232 (1): 65–86.
- Ding, K., F. T. Chan, X. Zhang, G. Zhou, and F. Zhang. 2019a. “Defining a Digital Twin-based Cyber-physical Production System for Autonomous Manufacturing in Smart Shop Floors.” International Journal of Production Research 57 (20): 6315–6334.
- Ding, K., F. T. Chan, X. Zhang, G. Zhou, and F. Zhang. 2019b. “Defining A Digital Twin-based Cyber-physical Production System for Autonomous Manufacturing in Smart Shop Floors.” International Journal of Production Research 57 (20): 6315–6334.
- Donald, W. S., T. C. Maness, and M. V. Marinescu. 2001. “Production Planning for Integrated Primary and Secondary Lumber Manufacturing.” Wood and Fiber Science 33 (3): 334–344.
- dos Santos, C. H., J. A. B. Montevechi, J. A. de Queiroz, R. de Carvalho Miranda, and F. Leal. 2021. “Decision Support in Productive Processes Through DES and ABS in the Digital Twin Era: A Systematic Literature Review.” International Journal of Production Research 1–20.
- Dumetz, L., J. Gaudreault, H. Bril El-Haouzi, A. Thomas, N. Lehoux, and P. Marier. 2021. “Tactical-Operational Coordination of a Divergent Production System with Coproduction: The Sawmilling Challenge.” INFOR: Information Systems and Operational Research 59: 377–399.
- Dumetz, L., J. Gaudreault, H. B. El-Haouzi, A. Thomas, N. Lehoux, and P. Marier. 2019. “Simulation and Evaluation of Coordination Mechanisms for a Decentralized Lumber Production System with Coproduction.” Forest Products Journal 69 (4): 260–271.
- Dumetz, L., J. Gaudreault, A. Thomas, H. Bril El-Haouzi, N. Lehoux, and P. Marier. 2017. Planning and Coordination for Decentralised Business Units in a Lumber Production Company, January. Working Paper or Preprint.
- Dumetz, L., J. Gaudreault, A. Thomas, N. Lehoux, P. Marier, and H. El-Haouzi. 2017. “Evaluating Order Acceptance Policies for Divergent Production Systems with Co-production.” International Journal of Production Research 55 (13): 3631–3643.
- Dumetz, L., J. Gaudreault, A. Thomas, P. Marier, N. Lehoux, and H. El Haouzi. 2015. “A Simulation Framework for the Evaluation of Production Planning and Order Management Strategies in the Sawmilling Industry.” IFAC-PapersOnLine 48 (3): 622–627.
- Dumont, L. B., P. Marier, N. Lehoux, L. Gosselin, and H. Fortin. 2019. “Integrating Electric Energy Cost in Lumber Production Planning.” IFAC-PapersOnLine 52 (13): 2249–2254.
- El Haouzi, H. B. 2017. “Contribution à La Conception et à l'évaluation des Architectures de Pilotage des systèmes de Production Adaptables: vers une approche anthropocentrée pour la simulation et le pilotage.” PhD diss., Université de lorraine.
- Forget, P., S. D'Amours, and J.-M. Frayret. 2008. “Multi-Behavior Agent Model for Planning in Supply Chains: An Application to the Lumber Industry.” Robotics and Computer-Integrated Manufacturing24 (5): 664–679.
- Forget, P., S. D'Amours, J.-M. Frayret, and J. Gaudreault. 2009. “Study of the Performance of Multi-behaviour Agents for Supply Chain Planning.” Computers in Industry 60 (9): 698–708.
- Frayret, J.-M., S. D'Amours, A. Rousseau, S. Harvey, and J. Gaudreault. 2007. “Agent-Based Supply-Chain Planning in the Forest Products Industry.” International Journal of Flexible Manufacturing Systems 19 (4): 358–391.
- Fuentealba, S., L. Pradenas, R. Linfati, and J. A. Ferland. 2019. “Forest Harvest and Sawmills: An Integrated Tactical Planning Model.” Computers and Electronics in Agriculture 156: 275–281.
- Galvez, J. L. A. P., D. Borenstein, and E. da Silveira Farias. 2018. “Application of Optimization for Solving a Sawing Stock Problem with a Cant Sawing Pattern.” Optimization Letters 12 (8): 1755–1772.
- Gaudreault, J., P. Forget, J.-M. Frayret, A. Rousseau, S. Lemieux, and S. D'Amours. 2010. “Distributed Operations Planning in the Lumber Supply Chain: Models and Coordination.” International Journal of Industrial Engineering: Theory, Applications and Practice 17 (3): 168–189.
- Gaudreault, J., J.-M. Frayret, and G. Pesant. 2009. “Distributed Search for Supply Chain Coordination.” Computers in Industry 60 (6): 441–451.
- Gaudreault, J., J.-M. Frayret, A. Rousseau, and S. D'Amours. 2011. “Combined Planning and Scheduling in a Divergent Production System with Co-production: A Case Study in the Lumber Industry.” Computers & Operations Research 38 (9): 1238–1250.
- Gaudreault, J., G. Pesant, J.-M. Frayret, and S. D'Amours. 2012. “Supply Chain Coordination Using an Adaptive Distributed Search Strategy.” IEEE Transactions on Systems, Man, and Cybernetics, Part C (Applications and Reviews) 42 (6): 1424–1438.
- Gil, A., and J.-M. Frayret. 2016. “Log Classification in the Hardwood Timber Industry: Method and Value Analysis.” International Journal of Production Research 54 (15): 4669–4688.
- Goulet, P. 2006. Optitek: User's Manual.
- Haberl, J., C. Nowak, H. Stettner, G. Stoiser, and H. Woschitz. 1991. “A Branch-and-Bound Algorithm for Solving a Fixed Charge Problem in the Profit Optimization of Sawn Timber Production.” Zeitschrift für Operations Research 35 (2): 151–166.
- Himes, A., and G. Busby. 2020. “Wood Buildings as a Climate Solution.” Developments in the Built Environment 4: 100030.
- Huka, M. A., and M. Gronalt. 2017. “Model Development and Comparison of Different Heuristics for Production Planning in Large Volume Softwood Sawmills.” Engineering Optimization 49 (11): 1829–1847.
- Huka, M. A., C. Rindler, and M. Gronalt. 2020. “Scheduling and Loading Problem for Multiple, Identical Dry Kilns.” Flexible Services and Manufacturing Journal 33: 312–336.
- Jerbi, W., J. Gaudreault, S. D'Amours, M. Nourelfath, S. Lemieux, P. Marier, and M. Bouchard. 2012. “Optimization/Simulation-Based Framework for the Evaluation of Supply Chain Management Policies in the Forest Product Industry. 2012 IEEE International Conference on Systems, Man, and Cybernetics (SMC). Seoul, Korea (south). October, 2012.
- Jover, J., A. Thomas, and V. Bombardier. 2011. “Marquage du Bois dans la Masse : Intérêts et perspectives.9e Congrès International de Génie Industriel.St Sauveur, Canada.Octobre, 2011.
- Jover, J., A. Thomas, J.-M. Leban, and D. Canet. 2013. “Pertinence of New Communicating Material Paradigm: A First Step Towards Wood Mass Marking.” Journal of Physics: Conference Series 416 (1): 012–031. doi:10.1088/1742-6596/416/1/012031.
- Kaltenbrunner, M., M. A. Huka, and M. Gronalt. 2020. “Adaptive Model Building Framework for Production Planning in The Primary Wood Industry.” Forests 11 (12): 1256.
- Kazemi Zanjani, M., D. Ait-Kadi, and M. Nourelfath. 2013. “A Stochastic Programming Approach for Sawmill Production Planning.” International Journal of Mathematics in Operational Research 5 (1): 1–18.
- Kazemi Zanjani, M., M. Nourelfath, and D. Ait-Kadi. 2010. “A Multi-stage Stochastic Programming Approach for Production Planning with Uncertainty in the Quality of Raw Materials and Demand.” International Journal of Production Research 48 (16): 4701–4723.
- Kazemi Zanjani, M., M. Nourelfath, and D. Aït-Kadi. 2011. “Production Planning with Uncertainty in the Quality of Raw Materials: A Case in Sawmills.” Journal of the Operational Research Society 62 (7): 1334–1343.
- Kogler, C., and P. Rauch. 2020. “Contingency Plans for the Wood Supply Chain Based on Bottleneck and Queuing Time Analyses of a Discrete Event Simulation.” Forests 11 (4): 396.
- Kousi, N., C. Gkournelos, S. Aivaliotis, C. Giannoulis, G. Michalos, and S. Makris. 2019. “Digital Twin for Adaptation of Robots' Behavior in Flexible Robotic Assembly Lines.” Procedia Manufacturing 28: 121–126.
- Laguna, M. 2018. Tabu Search, 741–758. Cham: Springer International Publishing.
- Lasi, H., P. Fettke, H.-G. Kemper, T. Feld, and M. Hoffmann. 2014. “Industry 4.0.” Business & Information Systems Engineering 6 (4): 239–242.
- Lemieux, S., S. D'Amours, J. Gaudreault, and J.-M. Frayret. 2009. “Agent-Based Simulation to Anticipate Impacts of Tactical Supply Chain Decision-Making in the Lumber Industry.” International Journal of Flexible Manufacturing Systems 19 (4): 358–391.
- Lindner, B., T. Visser, and B. Wessels. 2013. “A Model to Optimise the Linked Sawing and Ripping Decision in the South African Pine Wood Industry. 42nd Annual Conference of the Operations Research Society of South Africa. Stellenbosch, South Africa.
- Lindner, B. G., P. Vlok, and C. B. Wessels. 2015. “Determining Optimal Primary Sawing and Ripping Machine Settings in the Wood Manufacturing Chain.” Southern Forests: a Journal of Forest Science 77 (3): 191–201.
- Liu, Q., H. Zhang, J. Leng, and X. Chen. 2019. “Digital Twin-Driven Rapid Individualised Designing Of Automated Flow-Shop Manufacturing System.” International Journal of Production Research 57 (12): 3903–3919.
- Lobos, A., and J. R. Vera. 2016. “Intertemporal Stochastic Sawmill Planning: Modeling and Managerial Insights.” Computers & Industrial Engineering 95: 53–63.
- Maness, T. C., and D. M. Adams. 1991. “The Combined Optimization of Log Bucking and Sawing Strategies.” Wood and Fiber Science 23 (2): 296–314.
- Maness, T. C., and S. E. Norton. 2002. “Multiple Period Combined Optimization Approach to Forest Production Planning.” Scandinavian Journal of Forest Research 17 (5): 460–471.
- Marier, P., S. Bolduc, M. B. Ali, and J. Gaudreault. 2014. “S&OP Network Model for Commodity Lumber Products. MOSIM 2014, 10ème Conférence Francophone de Modélisation, Optimisation et Simulation. Nancy, France. November, 2014.
- Marier, P., J. Gaudreault, and T. Noguer. 2021. “Kiln Drying Operations Scheduling with Dynamic Composition of Loading Patterns.” Forest Products Journal 71 (2): 101–110.
- Marier, P., J. Gaudreault, and B. Robichaud. 2014. “Implementing a Mip Model to Plan and Schedule Wood Finishing Operations in a Sawmill: Lessons Learned. MOSIM 2014, 10ème Conférence Francophone de Modélisation, Optimisation et Simulation. Nancy, France.
- Marinescu, M. V., and T. C. Maness. 2010. “A Hierarchical Timber Allocation Model to Analyze Sustainable Forest Management Decisions.” Mathematical & Computational Forestry & Natural Resource Sciences 2 (2): 117–134.
- Martineau, V., M. Morin, J. Gaudreault, P. Thomas, and H. B. El-Haouzi. 2021. “Neural Network Architectures and Feature Extraction for Lumber Production Prediction.The 34th Canadian Conférence on Artificial Intelligence. Vancouver, Canada. May, 2021.
- Masood, T., and P. Sonntag. 2020. “Industry 4.0: Adoption Challenges and Benefits for SMES.” Computers in Industry 121: 103261.
- Maturana, S., E. Pizani, and J. Vera. 2010. “Scheduling Production for a Sawmill: A Comparison of a Mathematical Model Versus a Heuristic.” Computers & Industrial Engineering 59 (4): 667–674.
- Melesse, T. Y., V. D. Pasquale, and S. Riemma. 2020. “Digital Twin Models in Industrial Operations: A Systematic Literature Review.” Procedia Manufacturing 42: 267–272. International Conference on Industry 4.0 and Smart Manufacturing (ISM 2019).
- Mendoza, G., R. Meimban, W. Luppold, and P. A. Araman. 1991. “Combining Simulation and Optimization Models for hardwood Lumber Production.” In Pacific Rim Forestry -- Bridging the World. Proceedings of the 1991 Society of American Foresters National Convention San Francisco, CA, August 4-7, 356–361. Bethesda, MD: Society of American Foresters.
- Molina, J., M. Laguna, R. Martí, and R. Caballero. 2007. “Sspmo: A Scatter Tabu Search Procedure for Non-linear Multiobjective Optimization.” INFORMS Journal on Computing 19 (1): 91–100.
- Morin, M., J. Gaudreault, E. Brotherton, F. Paradis, A. Rolland, J. Wery, and F. Laviolette. 2020. “Machine Learning-Based Models of Sawmills for Better Wood Allocation Planning.” International Journal of Production Economics 222: 107508.
- Morneau-Pereira, M., M. Arabi, J. Gaudreault, M. Nourelfath, and M. Ouhimmou. 2014. “An Optimization and Simulation Framework for Integrated Tactical Planning of Wood Harvesting Operation, Wood Allocation and Lumber Production. MOSIM 2014, 10th Conférence Francophone de Modélisation, Optimisation et Simulation. Nancy, France. November 2014.
- Müller, F., D. Jaeger, and M. Hanewinkel. 2019. “Digitization in Wood Supply – A Review on How Industry 4.0 Will Change the Forest Value Chain.” Computers and Electronics in Agriculture 162: 206–218.
- Nantel, S., J. Gaudreault, and F. Léger. 2020. “Jumeau Numérique: Approches pour le Maintien d'un Inventaire Géolocalisé en Temps Réel d'une cour à bois .13ème Conférence Internationale de Modélisation, Optimisation et Simulation (MOSIM 2020). Agadir, Maroc. November 2020.
- Nguyen, V.-T., T. Constant, B. Kerautret, I. Debled-Rennesson, and F. Colin. 2020. “A Machine-Learning Approach for Classifying Defects on Tree Trunks Using Terrestrial Lidar.” Computers and Electronics in Agriculture 171: 105332.
- Oláh, J., N. Aburumman, J. Popp, M. A. Khan, H. Haddad, and N. Kitukutha. 2020. “Impact of Industry 4.0 on Environmental Sustainability.” Sustainability 12 (11): 4674.
- Opacic, L., T. Sowlati, and M. Mobini. 2018. “Design and Development of a Simulation-Based Decision Support Tool to Improve the Production Process at an Engineered Wood Products Mill.” International Journal of Production Economics 199: 209–219.
- Palma, C. D., and F. P. Vergara. 2016. “A Multiobjective Model for the Cutting Pattern Problem with Unclear Preferences.” Forest Science 62 (2): 220–226.
- Paradis, G., S. D'Amours, M. Bouchard, and L. LeBel. 2015. Extending the Classic Wood Supply Model to Anticipate Industrial Fibre Consumption. Vol. 6. University of Montreal, Montreal, Canada: Centre Inter-universitaire de Recherche sur les Réseaux d'Entreprise.
- Pradenas, L., C. Alvarez, and J. Ferland. 2009. “A Solution for the Aggregate Production Planning Problem in a Multi-plant, Multi-period and Multi-product Environment.” Acta Mathematica Vietnamica 34 (1): 11–17.
- Pradenas, L., G. Bravo, and R. Linfati. 2020. “Optimization Model for Remanufacturing in a Real Sawmill.” Journal of Industrial Engineering, International 16 (2): 32–40.
- Pradenas, L., J. Garcés, V. Parada, and J. Ferland. 2013. “Genotype–Phenotype Heuristic Approaches for a Cutting Stock Problem with Circular Patterns.” Engineering Applications of Artificial Intelligence26 (10): 2349–2355.
- Pradenas, L., F. Peñailillo, and J. Ferland. 2004. “Aggregate Production Planning Problem. A New Algorithm.” Electronic Notes in Discrete Mathematics 18: 193–199.
- Rathke, J., M. A. Huka, and M. Gronalt. 2013. “The Box Assignment Problem in Log Yards.” Silva Fennica 47 (3): 1–13.
- Reinders, M. 1992. “Cutting Stock Optimization and Integral Production Planning for Centralized Wood Processing.” Mathematical and Computer Modelling 16 (1): 37–55.
- Reinders, M. 1993. “Tactical Planning for a Cutting Stock System.” Journal of the Operational Research Society 44 (7): 645–657.
- Reitz, J., M. Schluse, and J. Roßmann. 2019. “Industry 4.0 Beyond the Factory: An Application to Forestry.” Tagungsband des 4: 107–116.
- Rönnqvist, M. 2003. “Optimization in Forestry.” Mathematical Programming 97 (1): 267–284.
- Rönnqvist, M., S. D'Amours, A. Weintraub, A. Jofre, E. Gunn, R. G. Haight, D. Martell, A. T. Murray, and C. Romero. 2015. “Operations Research Challenges in Forestry: 33 Open Problems.” Annals of Operations Research 232 (1): 11–40.
- Santa-Eulalia, L. A., D. Aït-Kadi, S. D'Amours, J.-M. Frayret, and S. Lemieux. 2011. “Agent-based Experimental Investigations of the Robustness of Tactical Planning and Control Policies in a Softwood Lumber Supply Chain.” Production Planning & Control 22 (8): 782–799.
- Savolainen, J., and M. S. Knudsen. 2021. “Contrasting Digital Twin Vision of Manufacturing with the Industrial Reality.” International Journal of Computer Integrated Manufacturing 35: 165–182.
- Semeraro, C., M. Lezoche, H. Panetto, and M. Dassisti. 2021. “Digital Twin Paradigm: A Systematic Literature Review.” Computers in Industry 130: 103469.
- Settles, B. 2009. Active Learning Literature Survey. Computer Sciences Technical Report 1648. University of Wisconsin-Madison Department of Computer Sciences.
- Shahi, S. K. 2016. “Supply Chain Management of The Canadian Forest Products Industry under Supply and Demand Uncertainties: A Simulation-based Optimization Approach.” PhD diss., Faculty of Natural Resources Management.
- Shahi, S., and R. Pulkki. 2015. “A Simulation-Based Optimization Approach to Integrated Inventory Management of a Sawlog Supply Chain with Demand Uncertainty.” Canadian Journal of Forest Research 45 (10): 1313–1326.
- Sinclair, M., and S. Erasmus. 1992. “A Microcomputer-Based Decision Support System for the Management of Lumber Mill Production.” Computers & Industrial Engineering 22 (4): 435–446.
- Singer, M., and P. Donoso. 2008. “Upstream or Downstream in The Value Chain?” Journal of Business Research 61 (6): 669–677.
- Skog, J., M. Jacobsson, and A. Lycken. 2017. “Traceability and Adaptive Production in the Digital Sawmill.” Pro Ligno 13 (4): 162–167.
- Sohrabi, P. 2013. “A Three-Stage Control Mechanism for the Lumber Production Process of a Sawmill Based on a Powers-of-two Modelling Approach.” Master's thesis, Dalhousie University.
- Staudhammer, C., T. C. Maness, and R. A. Kozak. 2007. “Profile Charts for Monitoring Lumber Manufacturing Using Laser Range Sensor Data.” Journal of Quality Technology 39 (3): 224–240.
- Tao, F., F. Sui, A. Liu, Q. Qi, M. Zhang, B. Song, Z. Guo, S. C.-Y. Lu, and A. Y. Nee. 2019. “Digital Twin-Driven Product Design Framework.” International Journal of Production Research 57 (12): 3935–3953.
- Theresia, J., I. G. A. Widyadana, and D. Wahjudi. 2019. “Optimal Kiln Dry Allocation for Dry Timber Preparation to Minimize Cost.” Jurnal Teknik Industri 21 (1): 43–48.
- Thomas, P., D. Choffel, and A. Thomas. 2008. “Simulation Reduction Models Approach Using Neural Network.10th International Conference on Computer Modeling and Simulation (uksim 2008). Sydney, Australia. January 8-10, 2018.
- Thomas, P., and A. Thomas. 2009. “How Deals with Discrete Data for the Reduction of Simulation Models Using Neural Network.” IFAC Proceedings Volumes 42 (4): 1172–1177.
- Todoraki, C., and M. Ronnqvist. 2001. “Log Sawing Optimisation Directed by Market Demand.” New Zealand Journal of Forestry 45: 29–33.
- Todoroki, C., and M. Rönnqvist. 2002. “Dynamic Control of Timber Production at a Sawmill with Log Sawing Optimization.” Scandinavian Journal of Forest Research 17 (1): 79–89.
- Torrey, L., and J. Shavlik. 2010. “Transfer Learning.” In Handbook of Research on Machine Learning Application and Trends: Algorithms, Methods, and Techniques. Vol. 1, 242–264. Hershey, New York: Information Science Reference.
- Troncoso, J., S. D'Amours, P. Flisberg, M. Rönnqvist, and A. Weintraub. 2015. “A Mixed Integer Programming Model to Evaluate Integrating Strategies in the Forest Value Chain – A Case Study in the Chilean Forest Industry.” Canadian Journal of Forest Research 45 (7): 937–949.
- Troncoso, J. J., and R. A. Garrido. 2005. “Forestry Production and Logistics Planning: An Analysis Using Mixed-Integer Programming.” Forest Policy and Economics 7 (4): 625–633.
- Vahid, S. 2011. “An Agent-based Supply Chain Model for Strategic Analysis in Forestry.” PhD diss., University of British Columbia.
- Vahidian, N. 2012. “Comparison of Deterministic and Stochastic Production Planning Approaches in Sawmills by Integrating Design of Experiments and Monte-Carlo simulation.” PhD diss., Concordia University Montreal.
- Vanzetti, N., D. Broz, G. Corsano, and J. M. Montagna. 2018. “An Optimization Approach for Multiperiod Production Planning in a Sawmill.” Forest Policy and Economics 97: 1–8.
- Vanzetti, N., D. Broz, G. Corsano, and J. M. Montagna. 2019. “A Detailed Mathematical Programming Model for the Optimal Daily Planning of Sawmills.” Canadian Journal of Forest Research 49 (11): 1400–1411.
- Vanzetti, N., D. Broz, J. M. Montagna, and G. Corsano. 2019. “Integrated Approach for the Bucking and Production Planning Problems in Forest Industry.” Computers & Chemical Engineering 125: 155–163.
- Vanzetti, N., G. Corsano, and J. M. Montagna. 2020. “Drying Operation Planning in a Sawmill.” Computers & Chemical Engineering 137: 106817.
- Varas, M., S. Maturana, R. Pascual, I. Vargas, and J. Vera. 2014. “Scheduling Production for a Sawmill: A Robust Optimization Approach.” International Journal of Production Economics 150: 37–51.
- Vergara, F. P., C. D. Palma, and H. Sepúlveda. 2015. “A Comparison of Optimization Models for Lumber Production Planning.” Bosque 36 (2): 239–246.
- Vila, D., R. Beauregard, and A. Martel. 2009. “The Strategic Design of Forest Industry Supply Chains.” INFOR: Information Systems and Operational Research 47 (3): 185–202.
- Wang, X. V., and L. Wang. 2019. “Digital Twin-based WEEE Recycling, Recovery and Remanufacturing in the Background of Industry 4.0.” International Journal of Production Research 57 (12): 3892–3902.
- Wang, J., L. Ye, R. X. Gao, C. Li, and L. Zhang. 2019. “Digital Twin for Rotating Machinery Fault Diagnosis in Smart Manufacturing.” International Journal of Production Research 57 (12): 3920–3934.
- Webb, G. I., R. Hyde, H. Cao, H. L. Nguyen, and F. Petitjean. 2016. “Characterizing Concept Drift.” Data Mining and Knowledge Discovery 30 (4): 964–994.
- Wery, J., J. Gaudreault, A. Thomas, and P. Marier. 2012. “Improving Sawmill Agility Through Log Classification. 4th International Conference on Information Systems, Logistics and Supply Chain, ILS 2012. Québec, Canada. August 2012.
- Wery, J., J. Gaudreault, A. Thomas, and P. Marier. 2018. “Simulation-Optimisation Based Framework for Sales and Operations Planning Taking into Account New Products Opportunities in a Co-production Context.” Computers in Industry 94: 41–51.
- Wery, J., P. Marier, J. Gaudreault, and A. Thomas. 2014. “Decision-Making Framework for Tactical Planning Taking into Account Market Opportunities (New Products and New Suppliers in a Co-production Context.10ème Conférence Francophone de Modélisation, Optimisation et Simulation (MOSIM 2014). Nancy, France. November 2014.
- Wessels, C. B., C. S. Price, P. Turner, and M. P. Dell. 2006. “Integrating harvesting and Sawmill Operations Using an Optimized Sawmill Production Planning System.International Precision Forestry Symposium. Stellenbosch, South Africa. March, 2006.
- West, S., O. Stoll, J. Meierhofer, and S. Züst. 2021. “Digital Twin Providing New Opportunities for Value Co-creation Through Supporting Decision-Making.” Applied Sciences 11 (9): 3750.
- Wohlin, C.. 2014. “Guidelines for Snowballing in Systematic Literature Studies and A Replication in Software Engineering.” In Proceedings of the 18th International Conference on Evaluation and Assesment in Software Engineering (EASE 2014), 321–330. London, United Kingdom: ACM.
- Xu, J., E. Huang, C.-H. Chen, and L. H. Lee. 2015. “Simulation Optimization: A Review and Exploration in the New Era of Cloud Computing and Big Data.” Asia-Pacific Journal of Operational Research 32 (03): 1550019.
- Zanjani, M. K., D. Ait-Kadi, and M. Nourelfath. 2010. “Robust Production Planning in A Manufacturing Environment with Random Yield: A Case in Sawmill Production Planning.” European Journal of Operational Research 201 (3): 882–891.
- Zanjani, M. K., D. Ait-Kadi, and M. Nourelfath. 2013. “An Accelerated Scenario Updating Heuristic for Stochastic Production Planning with Set-up Constraints in Sawmills.” International Journal of Production Research 51 (4): 993–1005.
- Zanjani, M. K., M. Nourelfath, and D. Ait-Kadi. 2007. A Stochastic Programming Approach for Production Planning in a Manufacturing Environment with Random Yield. Technical Report. CIRRELT, Centre interuniversitaire de recherche sur les réseaux d'entreprise ….
- Zanjani, M. K., M. Nourelfath, and D. Ait-Kadi. 2013a. “Sawmill Production Planning Under Uncertainty: Modelling and Solution Approaches.” In Stochastic Programming: Applications in Finance, Energy, Planning and Logistics. Vol. 4, 347–395. Singapore: World Scientific.
- Zanjani, M. K., M. Nourelfath, and D. Ait-Kadi. 2013b. “A Scenario Decomposition Approach for Stochastic Production Planning in Sawmills.” Journal of the Operational Research Society 64 (1): 48–59.
- Zheng, X., J. Lu, and D. Kiritsis. 2021. “The Emergence of Cognitive Digital Twin: Vision, Challenges and Opportunities.” International Journal of Production Research 1–23. doi:10.1080/00207543.2021.2014591.
- Zhou, G., C. Zhang, Z. Li, K. Ding, and C. Wang. 2020. “Knowledge-Driven Digital Twin Manufacturing Cell Towards Intelligent Manufacturing.” International Journal of Production Research 58 (4): 1034–1051.