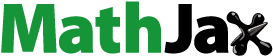
Abstract
Industrial manufacturing is a core component of a nation’s economic wealth. It is driven by technology, and its paradigm has shifted from craft production to mass production to lean production to mass customisation (MC). We identify how emerging technologies offer a new way to shift MC research focusing on the scientific communities that have been driving MC research, changes in MC throughout the years, and the technological advances that influence future research trends of MC. We then identify MC paradigms. We analyse 1,947 MC papers from 1992 to 2019 and discover the research attention changes from the perspectives of the communities and themes using topic modelling. Our result indicates that MC was explained in terms of the business and marketing strategy (BMS) and the operations management (OM), in accordance with technological development. In BMS, MC topics moved from demand uncertainty to industry transformation. In OM, topics changed from assembly line for product differentiation to additive manufacturing. We discuss the future research agenda according to the technological advances in computer-aided design, additive manufacturing, machine learning, identification technologies, virtual reality, and human–robot collaboration. Not only researchers but practitioners and policymakers can utilise our approach to analyse and formulate MC strategies considering new technologies.
1. Introduction
Mass customisation is a production strategy that integrates the good sides of material flow controls in both push manufacturing and pull manufacturing paradigms. It is important for a company to choose one production system, mixing push and pull production paradigms to seek a core competence. We now clearly know about different production paradigms (Pyke and Cohen Citation1990) and how new technologies have changed production paradigms over time (Figure ). However, we still do not know how emerging technologies revolutionised mass customisation, making individually specialised products or services available on a large scale and shifting its paradigm.
Figure 1. Production paradigms according to production variety and volume.
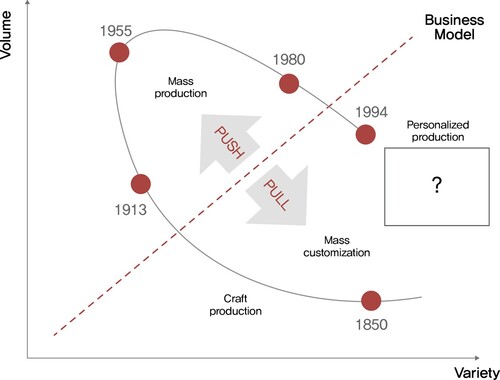
Pull production is based on actual demand, and the product is manufactured after the customer’s order arrives. Pull production benefits from an agile response to demand without demand forecasting. However, it cannot be applied to a product that requires a long lead time. Also, there are low-cost savings from an increased level of production. An example is the craft production system – which is also called the European system relative to the American system after World War II – that uses general-purpose machines and skilled craftsmen in a workshop (Hounshell Citation1985).
Push production is not based on actual demand but rather anticipated demand. In push production, a company forecasts demand and decides the amount of stock. Pull production has advantages of economies of scale and a short lead time. However, it cannot respond to fast-changing demand. An example is the mass production system, also known as the American system, Fordism, or the Ford system, which is based on standardisation of parts, simplification of products, and specialisation of jobs (Moore Citation1950). This American system, originally from the French armoury production system, penetrated across industries. Workers in the Springfield armoury developed gauge, special-purpose machines, and interchangeable parts; this approach later moved to factories in other industries (Hounshell Citation1985).
Yet choosing either a pull or push production system is insufficient to cope simultaneously with a rapidly changing market and secure economic feasibility. Thus, production systems between pull and push systems have been introduced. From the push production side, Alfred Sloan introduced a flexible mass production system, creating a full line of different automobiles to respond to the automobile market and changes in consumer taste without hesitation (Hounshell Citation1985). From the pull production side, Toyota introduced just-in-time production, also called lean production, that reduces manufacturing and response times from suppliers to customers.
Along with these production systems, mass customisation has been considered a new alternative for the past 30 years. Yet mass customisation has been doubted and criticised due to its difficult realisation (Agrawal, Mercer, and Kumaresh Citation2001; Hart Citation1995; Zipkin Citation1997; Zipkin Citation2001). Traditionally, mass customisation involves assembling parts in lines differently to make an individualised product. Recently, additive manufacturing changed the paradigm of mass customisation to include making a product without assembling parts. Mass customisation through assembly manufacturing can produce a higher volume but lower product mix compared to additive manufacturing. However, mass customisation seems to be a false promise, because we do not see successful mass customisation cases.
Nevertheless, new technologies have empowered progress in mass customisation of both assembly and additive manufacturing. They also helped implement mass customisation at a more detailed level. In mass customisation based on assembly manufacturing, automation, computer-integrated manufacturing and modelling, computer-aided design and manufacturing (CAD/CAM), and flexible manufacturing systems (FMS) have been important technologies (Eastwood Citation1996; Kotha Citation1995; Partanen and Haapasalo Citation2004). In mass customisation based on additive manufacturing, 3D printing (Attaran Citation2017), also known as stereolithography apparatus (Bogue Citation2013), is the representative technology.
Production technology shapes the organisational structure and management characteristics of a firm (Woodward Citation1965). And the product and market evolution of the firm are associated with manufacturing process characteristics (Hayes and Wheelwright Citation1979). In particular, advances in manufacturing and information technology can help a firm implement mass customisation (Pine, Pictor, and Boynton Citation1993). It is rational to consider mass customisation as a mixture between pull and push production paradigms, which are divided into business, marketing, strategy (push), and operations management (pull) driven by emerging technologies.
Thus, we dissect mass customisation research to identify how emerging technologies offer a new way to shift mass customisation from the perspective of scientific communities of two production paradigms. We examine the following three questions: (a) What scientific communities have been driving mass customisation research?; (b) What changes have been made in mass customisation research throughout the years?; and (c) What technologies have been introduced to mass customisation research and how can they influence future research trends?
The originality of this study is as follows.
This study is based on a novel quantitative approach, including period classification and topic modelling method, to review the paradigm changes in the mass customisation research area.
Due to the quantitative methods, the study could analyse more than 1,947 papers about mass customisation, while previous review papers considered a limited number of papers by a heuristic approach.
This study presents how research attention has moved among different fields and topics in mass customisation over the past.
This study attempts to establish the connection between the research attention changes on the mass customisation topics and technological advances.
2. Related works
2.1. Review of mass customisation
After the introduction of mass customisation, numerous studies with various approaches and techniques have been published in this research area. In some studies, mass customisation was the main subject of analysis, and the researchers tried to answer questions related to defining mass customisation, identifying the main differences of mass customisation compared to traditional strategies such as mass production and customisation, and breaking mass customisation down into several levels. Other studies focused on developing different methods and methodologies that were directly and indirectly related to achieving mass customisation. In other studies, mass customisation was only mentioned as a new environment for firms or a market.
One of the early definitions of the mass customisation strategy is ‘the low-cost production of high variety, even individually customised goods and services’ (Pine Citation1993). In Da Silveira et al.’s (Citation2001) study, mass customisation relates to the ability to provide customised products or services through flexible processes at high volumes and reasonably low costs. Among the different definitions, it is generally agreed that the distinguishable feature of mass customisation is a high variety of products at reasonably low costs. To do that, firms should achieve the two goals of mass customisation, flexibility and cost-efficiency, at the same time.
However, realising mass customisation could be challenging because there is a trade-off relationship between the two goals. Researchers have tried to find a balance between flexibility and cost-efficiency, which indicates different levels of mass customisation (Pine Citation1993; Lampel and Mintzberg Citation1996; Gilmore and Pine Citation1997; Duray et al. Citation2000; Montreuil and Poulin Citation2005).
These conceptual analyses were followed by studies with more specific perspectives and methods to realise mass customisation. Some researchers focused on the fragmented demands of the market (Goldsmith Citation1999; Goldsmith and Freiden Citation2004), whereas other researchers investigated decision-support methods for mass customisation companies (Ellena et al. Citation2018; Lohmann et al. Citation2019; Lei et al. Citation2016).
Meanwhile, some scholars chose a holistic approach and reviewed the mass customisation literature to draw meaningful insights for future research directions. In the early days, Da Silveira, Borenstein, and Fogliatto (Citation2001) identified six factors that may lead to the successful implementation of mass customisation from the literature and discussed the enabling process and methodologies related to implementation. Later, their work was extended by Fogliatto, Da Silveira, and Borenstein (Citation2012), who addressed economics, success factors, enablers, and customer–manufacturer interaction in mass customisation by reviewing 143 papers. Although they categorised publications into several fields, including general management, industrial or manufacturing engineering, information systems, marketing, operations management, and operations research or management science, less attention has been paid to changes among fields throughout the years.
As the number of paper publications has increased, researchers have broken down the mass customisation literature into several topics and chosen one with a narrower view. For example, Zhao, He, and Wu (Citation2008) focused on quality assurance in mass customisation, and Ferguson, Olewnik, and Cormier (Citation2014) used the product development framework and linked mass customisation papers to specific stages of the design process. Most recently, Guo et al. (Citation2019) reviewed the mass customisation literature from the perspective of inventory management and addressed key improvements and limitations of inventory management methods for regular inventories and leftover products.
Previous reviews of mass customisation summarised the literature, addressed methods and technologies, and discussed challenges for strategy, which provided meaningful insights to mass customisation researchers. However, no study has analysed how research attention has moved among different fields and topics in mass customisation. Although some reviews mentioned several research fields of mass customisation, few attempts have been made to investigate the relationships among topics and their changes over time. Also, most previous review papers depend highly on a heuristic reviewing method, so a limited number of papers have been reviewed.
2.2 Identifying themes and trends using topic modelling
An alternative way to review themes and trends of mass customisation research systematically is to use a text summarisation tool such as topic modelling to perform a science of science (Fortunato et al. Citation2018). Topic modelling statistically clusters words in documents, recognising groups of words as topics. By applying topic modelling to a collection of research papers in a certain field, we can uncover themes and trends. Topic modelling is better than semantic network analysis (Lee et al. Citation2017; Lee and Jung Citation2019) if the number of documents is large (Leydesdorff and Nerghes Citation2017). There are variants such as topic network analysis (Lee, Jung, and Song Citation2016), topic simulation analysis (Lee and Kim Citation2014), and topic ensemble analysis (Song, Kim, and Lee Citation2017).
Some studies uncovered topics in transportation research over time (Boyer, Scherer, and Smith Citation2017; Das, Sun, and Dutta Citation2016; Das et al. Citation2017; Sun and Yin Citation2017). Sun and Yin (Citation2017) discovered that sustainability, travel behaviour, and non-motorised mobility are increasing general research trends in transportation. Boyer, Scherer, and Smith (Citation2017) found that transportation research became holistic and global. Of note, topics including energy and fuel, alternative transportation modes, accessibility and health, and mobility technology are rising in popularity. At the same time, topics including construction and infrastructure also rose slightly. Das et al. (Citation2017) discovered that traffic safety and traffic operational research had high probability, frequency, and exclusivity compared with pavement-related research.
Scholars identified research trends regarding a growing manufacturing issue, the smart factory, both statically and dynamically (Chang and Yang Citation2017; Yang, Chang, and Choi Citation2018). Yang, Chang, and Choi (Citation2018) utilised topic modelling and regression analysis to determine the major research directions of smart factories in Korea and all countries. They found that the research topics of information and communications technology, policies by country, and economic changes are rising in Korea. Also, they found that research topics related to the Internet of Things, information and communications technology, research and development, technological innovation, operations management software, and manufacturing hardware are rising internationally. Xiong et al. (Citation2019) conducted an analysis of research topic changes in manufacturing research. They discovered that topic coverage has increased over time and manufacturing research became more interdisciplinary. In particular, manufacturing research not only covers traditional manufacturing topics such as manufacturing processes, mechanical transmission, and machining tools, but also advanced manufacturing techniques such as laser-based manufacturing, semiconductor fabrication, and planning of production processes. Additionally, in manufacturing research, management science and materialogy have been found to be more important. Most recently, Wang and Hsu (Citation2021) used topic modelling and generalised linear mixed models to identify technological topics in smart manufacturing based on patent data. The technological topics were categorised into vertical integration, virtualisation, automation, traceability, and flexibility. The most valued topics were smart connection, cyber–physical systems, manufacturing data analytics, and the powder bed fusion approach in additive manufacturing.
However, production and mass customisation, which is the science of a complex and evolving network of research, have been unexplored. In addition, their evolving characteristics and patterns have gone unidentified. This has resulted in companies’ low core competence in mass customisation and the shortage of successful mass customisation. To help mass customisation be successful in a company, we analyse mass customisation research and identify its sequential paradigm shifts using topic modelling and time series analysis, as a part of a science of science.
3. Methods
The data were analysed using two approaches. First, to capture the generic trends of mass customisation research, we compare the number of research papers related to business and marketing strategy (BMS) and operations management (OM) over time. Second, we draw some main topics in the research area of mass customisation and compare their ranking changes from the perspectives of business and marketing strategy and operations management.
Because mass customisation research varies from mass customisation as the main subject to mass customisation as an environmental factor, we chose a rather broad scope of mass customisation research to include as many studies on mass customisation as possible. Therefore, we analyse papers in which mass customisation was included in the title, addressed as a keyword, or mentioned in the abstract.
3.1 Procedure
First, we categorise papers into two groups, push production paradigm and pull production paradigm, based on a journal classification system such as Scimago (Scimago Journal & Country Rank Citation2019). Then, we count the number of publications over time for each group. We draw time series data representing push production paradigm papers and pull production paradigm papers. We apply a change-point detection algorithm to these two time series data.
Second, we train a topic model using all papers as data and we identify a fixed number, K, of topics made of probability distributions from all words in the papers. Here, each paper is represented as a vector of K probabilities. Each probability means the proportion of a topic in the paper. Then we identify each topic as either the push production paradigm or pull production paradigm. We aggregate the proportions of the topics for each group. This results in two time series data representing push production paradigm topics and pull production paradigm topics. We then apply the change-point detection algorithm to these two time series data.
Third, coders restate topics, bags of words, in certain subjects in production. The coders search hypertexts using salient words in a topic as search terms in a search engine like Google, interpret the subject that abstracts the hypertexts and then state the topic again in a new way. Finally, the coders categorise each topic in either the BMS or OM group.
Fourth, we perform a journal group trend analysis or topic group trend analysis using coefficients drawn from linear regressions on two journal groups or two topic groups. Subsequently, we analyse changes over time in the two production paradigm groups and induce mass customisation paradigm changes over time using two dimensions composed of the two production types and levels of management (or control). Figure shows a position map example with the two dimensions. We locate a circle representing each topic on this map according to its coverage on production (i.e. material flow control) types and control levels. The colour of a circle implies its scientific community. A red circle means BMS. A blue circle means OM. In the example, the market segmentation topic covers pull production type (i.e. material flow control depends on actual demand) and strategic level of management. On the other hand, the quality control topic covers push production type (i.e. material flow control depends on stocks and anticipated demand) and operational level of management.
Figure 2. An example of positioning map.
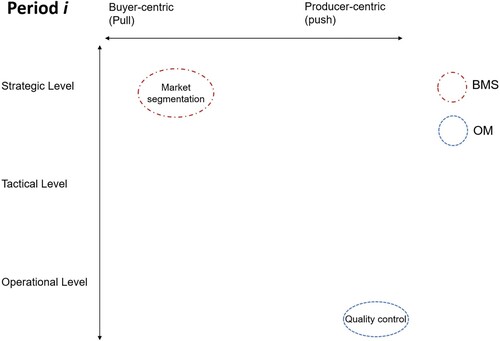
3.2 Change-point detection
Here, we apply the single change-point method to the entire time series of proportions of studies in BMS and OM iteratively using Python package ruptures (Truong, Oudre, and Vayatis Citation2020). It examines if they have a breakpoint where each time series before and after the breakpoint has different characteristics (Bai Citation1997). The method keeps comparing the cost of the total time series and the cost of having a split with a penalty. The method decides a split if the former cost exceeds the latter cost. This binary decision of partition is called a binary segmentation search method.
Change-point detection can be seen as an optimisation problem. It seeks the optimal segment points that minimise the total cost under certain constraints. And thus, it has three components: cost function, search method, and constraint (Truong, Oudre, and Vayatis Citation2020). In general, let be the value function that requires a set of c break points,
as input, and a cost function to compute the value generated from one time point to another (Equation (1)). Here, a change-point detection algorithm finds
that can minimise
. And the total time is T.
means y values from
to
(1)
(1)
Figure shows an example and assumes that we know the number of breakpoints, three, in advance. The total value can be computed as where
. The arguments that make the minimum value are
and
.
In particular, the binary segmentation search method looks for the change point that lowers the sum of costs the most in a given period(s). The binary segmentation search method seeks the change point that generates the minimum cost from partitioned periods iteratively. If we apply this binary segmentation search method to the example in Figure , six is found first. Subsequently, two partitions (0:6) and (6:30) are made. Then, the method is again applied to the two partitions. Thirteen is the next change point that lowers the sum of costs the most. Subsequently, three partitions (0:6, 6:13, and 13:30) are made. Next, the method is again applied to the three partitions, and 21 is the next change point. Consequently, four partitions (0:6, 6:13, 13:21, and 21:30) are found. Thus, the number of change points is three and the number of partitions is four, which meets the termination condition.
3.3. Topic modelling: Latent Dirichlet allocation with online variational Bayes algorithm
Latent Dirichlet allocation (LDA) is an archetypal statistical model for discovering topics in a collection of documents. LDA learns K latent variables made of the mixtures of words in D documents. The latent variables are called topics because they can be interpreted as subjects that comprise the collection of documents. K is fixed before applying LDA to the collection of documents, and it is difficult to define its optimality. One way to check if the fixed K is an optimal number is by using the concept of perplexity (Chuang, Manning, and Heer Citation2012). Perplexity, i.e. , is the degree of ambiguity of a prediction in information theory. Namely, if a probability distribution or model makes a good prediction, the loss of information to represent subjects is low and it means the prediction is certain. The lower the perplexity score, the better the representation of subjects. It can be computed as the inverse of the geometric mean per-word likelihood. It is an exponential function whose exponent is the log likelihood per word if xi is ith word as the following equation. We use the implementation of perplexity in Scikit-Learn (Pedregosa et al. Citation2011), where b is Euler’s number, e. In general, the optimal K is the minimum K among perplexity scores for a different number of topics.
LDA applies Bayesian learning to a hierarchical probability distribution, which is layered by probability distribution over topics and probability distribution of each topic over words (Blei Citation2012). LDA begins with random probability distributions but fits the distributions to given data. It observes words in each document and updates topic probability distributions over words conditioned on the observed words. Accordingly, it finds the probability distribution of each document over topics, the probability distribution of each topic over words, and topic assignment for each word when words in each document are fed. Namely, LDA generates , where
is a topic probability distribution of a document d and it is controlled by a parameter,
;
is a word probability distribution of a topic k generated by a parameter,
;
is a topic assigned to nth word in a document d; and
is an nth word observed in a document d. This is the posterior probability distribution, which is a multinomial distribution, and it can be computed by the following equation.
(2)
(2)
The numerator can be rewritten as the following equation.
(3)
(3)
It is a joint probability that is easy to compute. The first two terms stand for the prior probability. The latter is a model. The observable variable is w, which can be drawn from documents. LDA uses the Dirichlet–multinomial conjugate relationship. is a Dirichlet distribution conditioned on a parameter,
.
is a Dirichlet distribution conditioned on a parameter,
.
The denominator, , is difficult to compute because the marginal
is intractable. Possible computational solutions to approximately compute the denominator are Gibbs sampling and variational inference. Yet they are slow because they necessitate all the data to sample or infer a desirable probability distribution. To overcome this computational problem, we use LDA with online variational inference (Hoffman, Blei, and Bach Citation2010).
We also use LDAvis, a tool to help users to interpret topics using data visualisation (Sievert and Shirley Citation2014). It helps people to easily look at a ranked list of the most relevant terms in each topic. The authors provided a user-controlled variable called relevance. It measures the degree to which a word appears in a certain topic to the exclusion of others according to the user’s input, ranging from 0 to 1. The relevance of word w to topic k is determined by a weight parameter, λ. It is the rank of the word decided by its lift based on the number of the occurrences of the word when λ is 0. It is the rank of the word computed by its topic-specific probability when λ is 0. And it is the weighted sum of the previous two when λ is between 0 and 1. A topic-specific probability is explained by the concept of salience of a word (Chuang, Manning, and Heer Citation2012). Salience acts as an indicator to order the most important terms of each topic.
4. Result
We first collected data from research papers that mentioned mass customisation in the title or abstract or as a keyword, published between 1992 and 2019. Through Web of Science, data from 1947 papers in 160 journals were collected, including their title, source (journal), publication year, author names, abstract, and keywords. Based on the categories of ‘2018 SJR’ and ‘Journal quality list 2018,’ we reclassified all 160 journals into three categories: BMS, OM, and others. As a result of the LDA, 50 topics were drawn from the mass customisation papers (Table ). The topics were labelled based on the words representing each topic, and as we did to the journals, the topics were also classified into the three categories.
Table 1. Fifty topics from the mass customisation research area.
4.1. Overall analysis based on domain
First, we arranged the number of research papers and their journals in a year (Table ). In 1992, only one paper was published (Mueller-Heumann Citation1992) in a BMS journal. In the first few years, research papers on mass customisation had been published in BMS and other sources, yet none in OM journals. In 1996, three OM journals published four research papers related to mass customisation, 4 years after the first paper was published in a BMS journal. For BMS journals, the number of published papers increased to 15 until 2000 and remained stable. Meanwhile, the number of OM journal publications increased until 2010.
Table 2. Number of journals related to BMS, OM, and other topics and their paper publications.
Afterwards, we checked the overall trend of the number of BMS journal publications throughout the years (Figure ). The regression line showed a negative value of the slope (). According to the binary segmentation search method, five change points were found, resulting in six periods. At first, the proportion of BMS journal publications dropped in the first period (1992–1996), then rose to the peak point in the second period (1997–2001). During the last four periods (2002–2006, 2007–2011, 2012–2016, 2017–2019), the proportion of BMS journals to total publications decreased, with small fluctuations.
Figure 4. Linear regression and change-point detection using the publication ratio of (a) BMS journals and (b) OM journals.
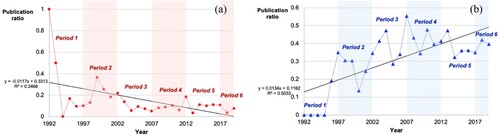
The OM journal publications ratio followed a different trend. The slope of the regression line had a positive value (). The publication ratio was 0 in the first four years, rose to 0.6 in 2007, then decreased to 0.4. For OM journals, the binary segmentation search yielded the same result as for BMS journals. Compared to BMS journals, the differences between each period were more distinct. The proportion of OM journal publications increased in the second (1997–2001) and third (2002–2006) periods, with a larger fluctuation. The peak point was found in the fourth period (2007–2011), and the proportion slightly decreased in the fifth period (2012–2016) and rebounded a little in the last period.
4.2. Topic analysis
4.2.1. Period classification based on topics
LDA gives the proportion and probability distribution of the topic values for each paper. Based on the topic classification, the sum of the probabilities of BMS and OM topics was calculated each year (Table ). For example, only one paper was published in 1992, and the paper consisted of BMS, OM, and other topics, with probabilities of 0.38, 0.38, and 0.22, respectively. Likewise, four papers were published in 1993, and the sum of their probabilities of BMS, OM, and other topics were 0.56, 2.61, and 0.81, respectively. Because we choose the top 50 topics, sometimes the total sum of the probabilities of the 50 topics in a year did not match the number of papers published in the same year. (For example, the sum of BMS, OM and other topics in the first year was 0.98, not 1.)
Table 3. Sum of BMS, OM, and other topic values and their proportions.
At first, the linear regression method was used to check the overall trend of the data (Figure ). With a negative value of the slope, the regression line shows a decreasing trend during the time horizon (), which can be observed in the graph as well (Figure (a)).
Figure 5. Linear regression and change-point detection using the proportion of (a) BMS topics and (b) OM topics.
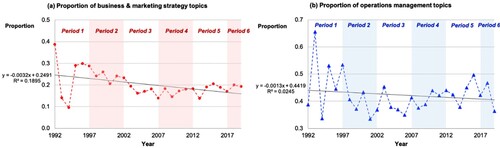
Figure 6. Proportion changes in BMS topics over six periods.
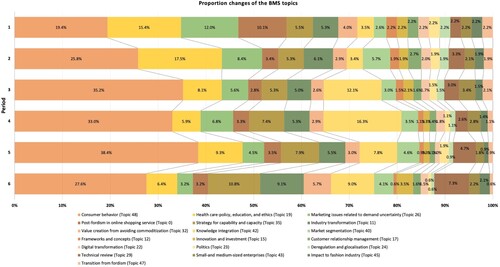
According to the binary segmentation search method, five change points were found, resulting in six periods. In the first period (1992–1996), the total proportion of BMS topics was low in the first 2 years, then rapidly rose to almost 30% in 1995. Afterward, the total proportion of BMS topics went down during the next two periods (1997–2001, 2002–2006) and stayed stable in the fourth period (2007–2011). In the last two periods (2012–2016, 2017–2019), the proportion slightly increased and remained stable.
For the topics in OM, the regression line also had a negative value in the slope () (Figure (b)). However, the data show high variation over time, and the R-square value is almost 0 (
). It is difficult to say the data show a decreasing trend from the linear regression. Rather, the distribution of the data shows different trends among periods, as seen in the result of the change-point detection.
In the first period (1992–1996), the proportion of OM topics changed dramatically, cycling between high and low values. In the second period (1997–2001), the proportion of operations research topics decreased rapidly, reaching the lowest point (25%) in the last year of the second period. In the third period (2002–2006), the proportion increased to 38% and then decreased again. The trend changed to incremental in the fourth period (2007–2011), and its value increased to 48% in the last year of the fifth period (2012–2016). However, its proportion decreased again in the last period (2017–2019).
4.2.2. Changes in BMS topics
We also present the proportion change of the BMS topics during the six periods to observe how research attention in the BMS area has evolved (Figure ). Among BMS topics, consumer behaviour (Topic 48) ranked highest in all periods. In the first period, consumer behaviour was followed by the health care-related topic (Topic 19), and their percentage difference was about 4%. However, the difference increased in the subsequent periods, and the largest gap was found in the fifth period (29%). In the last period, the health care-related topic fell to sixth.
The other two topics popular in the early years were marketing issues related to demand uncertainty (Topic 26) and post-Fordism in online shopping services (Topic 0), and they also showed a gradual decline over time. The proportion of demand uncertainty decreased from 12% to 3.25%, and that of post-Fordism in online shopping changed from 10.09% to 3.23%.
Meanwhile, some BMS topics that suggest more specific directions have gained more attention over time. For example, strategy for capability and capacity (Topic 35) was in fifth place in the first period (5.51%), but it rose to second place in the last period (10.83%). Likewise, knowledge integration (Topic 42), which is related to establishing management information systems (MIS), also increased from eighth (3.38%) to second place (12.1%). In the fifth period, however, its rank slightly decreased to fourth (7.85%). Technical review (Topic 29) started in 16th place at the beginning (2.23%) and was ranked fifth in the last period (7.3%).
At the bottom of the ranking, a few topics had no major changes in their proportions, such as small and medium-size enterprise (Topic 43) and customer relationship management (Topic 17). Other topics decreased in proportion over time, including transition from Fordism (Topic 47), deregulation and globalisation (Topic 24), and politics (Topic 23).
4.2.3. Changes in OM topics
Figure presents how the proportions of the OM topics changed over the six periods. Among all topics, optimisation with a multi-objective model (Topic 6) ranked at the top in all six periods, occupying almost half of the total proportion, between 39.65% and 56.53%. Keywords representing Topic 6 are rather widely used terms in OM (such as optimisation, algorithms, models, mathematical, model, multi, objective, computational, and methods), so this topic occupies a high proportion in most OM studies and shows the highest cumulative rate.
Figure 7. Proportion changes in OM topics over six periods.
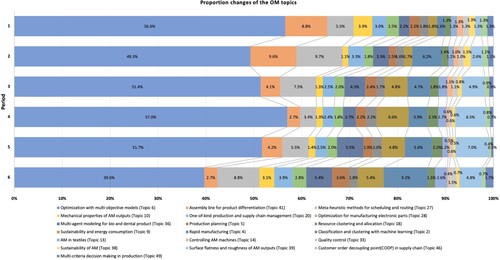
One traditional approach in OM, assembly line for product differentiation (Topic 41), was in the top three during the first two periods, but then fell to seventh and 11th in later terms. As an operational method for mass customisation, the topic got attention in the early years. However, after the second period, its cumulative proportion decreased to less than half its original size. For the other traditional topics in operations management, one-of-kind production and supply chain management (Topic 20) ranked above eighth, whereas topics including customer order decoupling point in supply chain (Topic 46), quality control (Topic 33), and multicriteria decision-making in production (Topic 49) got less attention. In contrast, the topic related to a problem-solving method, the meta-heuristic method (Topic 27), reached the higher ranks.
An interesting side finding was that additive manufacturing (AM)-related topics have gained more attention over time, whereas the proportion of attention to assembly line for product differentiation (Topic 41) has decreased. AM-related topics include rapid manufacturing (Topic 4) and surface flatness and roughness of AM outputs (Topic 39). Mechanical properties of AM outputs (Topic 10) ranked fourth in the first period (3.89%), and many scholars stressed the potential of AM as a key enabler of mass customisation. In the next period, however, it suddenly fell to 14th (1.13%), where it stayed for four periods. After surface flatness and roughness of AM outputs (Topic 39) was discussed (and its proportion decreased from 7.01% to 4.83%), attention moved to a more general perspective, the mechanical properties of AM outputs (Topic 10), with a proportion increasing to 3.07%.
In terms of sustainability, there are two contrasting results. On one hand, sustainability and energy consumption (Topic 9) started at 10th (1.8%) in the first period, rose to second (6.65%) in the fourth period, and fell slightly to sixth (4.8%) and fourth (5.42%) in the fifth and sixth periods, respectively. On the other hand, the sustainability of AM (Topic 38) received low attention in all periods (with a proportion between 0.45% and 1.27%).
Last, for topics related to specific applications, such as optimisation for manufacturing electronic parts (Topic 28), multi-agent modelling for bio and dental products (Topic 36), and AM in textiles (Topic 13), their positions were in the middle of the rankings.
5. Paradigm shift in mass customisation research
Since the concept of mass customisation was first introduced, research attention has shifted from topic to topic. As time passes, some topics have received more attention from researchers, whereas others have remained stable. Some topics have gained momentum from technological advances and been realised in operational or tactical methods, whereas others have been discussed at a strategic level, indicating potential and vision for the future.
In this section, we firstly re-organised the results according to the main findings, and from that, we furtherly discussed the future research directions of mass customisation focusing on the emerging technologies and their technological advances.
5.1. Topics that have gained more and less attention over time
In this study, from a comprehensive perspective, we analyse how popular topics have changed between the first (1992–1996) and last (2017–2019) periods, focusing on the correlation between OM topics with BMS and technology-related topics. First, the high-ranked topics are plotted according to the main perspective (horizontal axis) and managerial level (vertical axis; Figure ). Then, the paradigm shift among different topics is analysed using their position changes, including appearance and disappearance, and their relations with other topics, according to the representative studies of each topic.
Figure 8. Paradigm shift in the mass customisation research area between Period 1 (1992∼1996) and Period 6 (2017∼2019).
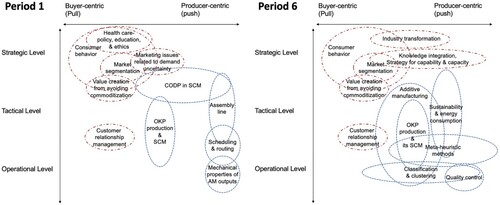
In the plots, the width of a topic represents the coverage area between a buyer-centric perspective (pull) and producer-centric perspective (push). For example, researchers from a buyer-centric perspective may focus on the increasingly diverse needs of the consumers and analyse how the markets and potential buyers would change in a new era. On the other hand, from a producer-centric perspective, subjects such as a firm’s capability to deal with fragmented, unexpected demands are considered more critical issues.
In Figure , most of the presented topics are in the higher ranks in each period, but topics that showed drastic changes in their ranks are also included. The positions, widths, and heights of the topics are based on their definitions and scopes of the methodologies used in the literature.
5.1.1. Marketing issues and consumer behaviour: research attention has moved from the perspective of companies to that of consumers
Initially, BMS studies focused on marketing issues related to demand uncertainty (Topic 26). Researchers sought to understand the new paradigm, mass customisation, and attempted to identify how to apply it and obtain profits from it from the perspective of companies. With the broader adoption of mass customisation, some research attention moved from companies to consumers, and topics such as consumer behaviour (Topic 48) have been explored more in recent periods.
After the concept of mass customisation was introduced, there was a gradual movement in marketing thought and practise such as mass marketing, market segmentation, niche marketing, micromarketing, and personalisation (Goldsmith Citation1999). Of note, studies that had a high level of marketing issues related to demand uncertainty (Topic 26) explored the existence of the mass customisation markets to ensure corporate profits. An early study that had a high proportion of Topic 26 showed that the most likely buyers of a personalised product tend to be younger, more well-educated consumers with higher-than-average incomes (Goldsmith and Freiden Citation2004).
Because the demand for mass customisation was regarded as a niche market, researchers have proposed rather general strategies to guarantee mass customisation firms’ profits, with a small number of consumers who have specific requirements for their products or services. For example, Goldsmith (Citation1999) emphasised that it was important for marketers to find potential buyers who would like a personalised version of the product. And only after selecting target segments successfully would marketers develop a marketing plan using methods such as the traditional 4Ps (product, price, promotion, and place) and additional 3Ps (personnel, physical assets, and procedure).
Over time, as mass customisation has become a popular strategy for business (following mass production), consumers have also become accustomed to customising their products in various ways (Pallant, Sands, and Karpen Citation2020). For example, customers of IKEA can co-produce their products by playing ‘assembler’ and being involved in the late stage of the development, with the design that IKEA developed. Customers of Nike By You co-configure the products by getting involved in the late stage of development but leading the product design. Meanwhile, the computer-aided LEGO Digital Designer allows customers to codesign LEGO models from the early stage of development.
Now most scholars seem to agree that mass customisation companies can guarantee profits by developing an appropriate strategy for the target market segment (Jost and Süsser Citation2020). However, along with the topic of consumer behaviour (Topic 48), there is less consensus regarding whether customer participation in the customisation process increases customer satisfaction (Khan and Haasis Citation2016; Zhao et al. Citation2019b; Turner, Merle, and Gotteland Citation2020). In an empirical study by Turner, Merle, and Gotteland (Citation2020), control and enjoyment of the codesign experience had a positive influence on customer satisfaction, whereas the complexity of the customisation process had a negative influence on customer satisfaction. In addition, factors such as design freedom, price, web user interface, visual presentation, and trust could also influence customer satisfaction and consumers’ purchase intention (Zhao et al. Citation2019b).
The recent line of customer behaviour research suggests that in mass customisation, not only can the product or service be the target of customisation, but also the customer participation process itself can be varied and even customised. As an example, a study on personalising the customisation experience showed that customer satisfaction with and likelihood of purchasing the product increased by matching the interface design to customers’ culture-specific processing style (de Bellis et al. Citation2019).
5.1.2. Advances in methodology: promoted by the increasing ability of computation
In the early periods of mass customisation, OM topics were discussed with a perspective of the mass customisation company (push) in a later stage of the production, due to the complexity of calculations to derive solutions for pure customised products. Most OM researchers tried to find ways to increase product variety and manufacturing flexibility while preserving production efficiency in the mass production system, through assembly lines (Topic 41). Consequently, the concepts of product platform and product family (Tseng and Jiao Citation1998; Jiao and Tseng Citation1999; Krishnan, Singh, and Tirupati Citation1999) and modularity (Duray et al. Citation2000; Joneja and Lee Citation1998; Salvador, Forza, and Rungtusanatham Citation2002) have drawn attention in mass customisation.
Although the results indicate that the cumulative proportion of the assembly line topic (Topic 41) was discussed more than other OM topics, it was difficult to find major studies about assembly lines in mass customisation. Even though studies mentioned assembly lines, from case studies (Suarez, Cusumano, and Fine Citation1996; MacDuffie, Sethuraman, and Fisher Citation1996) to theoretic development (Rubinovitz, Bukchin, and Lenz Citation1993; He and Kusiak Citation1997; Sawik Citation2000), they did not mention the term ‘mass customisation’ explicitly in their work, because they did not consider customised products. At that time, the focus was still on predefined products with limited variation, unable to consider custom requirements.
In the latter periods, however, various methods have appeared to deal with mass customisation, as the increasing amount of computing power available ensures better performance of problem-solving methods and algorithms (Amodei and Hernandez Citation2018). According to one analysis, computing power used for training AI systems approximately doubled every 2 years until 2012 (Amodei and Hernandez Citation2018), at which the doubling rate of computational power increased from 2 years to 3.4 months (Sastry et al. Citation2019).
This increased computational ability promoted the appearance of meta-heuristic methods (Topic 27) and classification and clustering (Topic 2), which cover a wider range from a producer-centric perspective (push) to a buyer-centric view (pull) with custom requirements (Figure ).
Meta-heuristic methods (Topic 27) were rarely exclusively discussed in a paper, but they occupied a certain proportion of scheduling and routing studies (Leng and Jiang Citation2019; Liu, Jin, and Price Citation2018). This problem-solving method also has been applied to other OM studies, such as supply chain configuration (Kumar and Chatterjee Citation2013; James and Mondal Citation2021), cost estimation of new product development (Relich and Pawlewski Citation2018), and evaluation of online toolkit design (H. Zhao et al. Citation2019a).
Methods related to classification and clustering (Topic 2) can be applied to both customers’ requirements and manufacturers’ properties. For example, in the study of a design framework for customising bicycle helmets, the target population was split into clusters based on shape similarity (Ellena et al. Citation2018). From the producer-centric view, the topic can be applied to group workers based on their learning profiles in production lines (Lohmann et al. Citation2019) or to develop modules in a product platform (Lei et al. Citation2016).
5.1.3. Industry transformation: mass customisation meets new practices
More than 3 decades have passed since the concept of mass customisation was introduced. Meanwhile, technological advances and economic changes have generated new themes and practices in mass customisation. In this respect, topics related to industry transformation were found to have gained more attention from researchers.
The topics related to industry transformation are mostly discussed at the strategic level, but they consist of a wide range of subjects from a producer-centric view (push) to a buyer-centric view (pull). First, regarding industry transformation (Topic 11, BMS), studies with a high presence of this topic suggested how the industry has been changing (or should change) in the new era. For example, Reynolds (Citation2017) introduced how advanced manufacturing technologies affect the location and spatial composition of production in mass customisation. Furthermore, the changing economics and emphasis on innovation is making manufacturing in cities and metropolitan areas a feasible option for firms with advanced manufacturing capabilities. Liu, Zhang, et al. (Citation2018) explored supply chain coordination issues arising from China’s strategy on boosting regional diplomacy, known as the One Belt and One Road initiative.
As another example, new online platforms for sharing and lending business models under the new era of digitalisation, such as Airbnb and Uber, have been facilitated by shifting consumer behaviour from private to shared ownership to match supply and demand (Richter et al. Citation2017). Because users can participate in the sharing economy as both a supplier and consumer, the number of variants in resources and services could be extremely increased through sharing, creating personalised experiences not only based on what the consumers get but also how they get it. As such, the service fulfils the characteristics of massification and adjusts to the individual needs of every customer at the same time, so it was argued that mass customisation can be a building block for the entrepreneurship of sharing in Szabó’s (Citation2017) work, wherein the probability of industry transformation (Topic 11) is 94%.
Despite the relation between mass customisation and the sharing economy, few attempts have been made to investigate the issues of the sharing economy from the perspective of OM. For example, based on a conceptual analysis of the definition and characteristics of crowd logistics initiatives (Carbone, Rouquet, and Roussat Citation2017), a recent study analysed 157 sharing economy firms and examined their supply chain networks (Atkins and Gianiodis Citation2021). From conceptual analyses, researchers may expand the discussion to operational issues and their solutions for sharing economy firms, such as cost–benefit analysis and operational planning and scheduling from the perspective of supply chain management.
Another topic related to industry transformation is health care (Topic 19). From the traditional notion of the doctor–patient relationship, customisation is at the core of the conception of health care (Minvielle et al. Citation2014). Therefore, in the first few periods, health care gained some research attention, with its potential to provide a personalised experience to customers in this service area. However, this attention has decreased over time; in the first period, health care ranked in the second position (15.4% of total topics), but its position dropped to sixth in the last period (6.4% of total topics).
Early studies that presented a high proportion of health care (Topic 19) only mentioned mass customisation as a possible suggestion for the future of healthcare (Lincoln and Builder Citation1998; Robinson Citation2001; Thompson and Nussbaum Citation2000). However, successful adoption of mass customisation in health care still faces the following barriers: (a) difficulty adjusting business models to the traditional cost-based approach of mass customisation, (b) lack of understanding of the beneficiaries’ perspectives (patients and their relatives), (c) lack of proper reengineering methods for the care process, and (d) absence of decision-support tools for multiple criteria and opposing objectives (Minvielle et al. Citation2014). That is, little has been understood about tactical and operational approaches for mass customisation in health care.
Recently, a new attempt has been made in the health care area: food production, which can be understood as a part of health care (Calegari and de Castro Fettermann Citation2018; Calegari, Avalone, and Fettermann Citation2020). However, according to Calegari and de Castro Fettermann’s (Citation2018) work, barriers still exist to implement mass customisation in the food industry, including food perishability, difficulty in processing, nutritional values quantification of the customised food, and perceived complexity of benefit by the customers. Future research on health care in mass customisation should further investigate ways to overcome those limitations.
5.1.4. Sustainability: is mass customisation a more sustainable option?
Other researchers emphasised the importance of sustainable development and suggested a future direction for lowering environmental impacts amid mass customisation. According to our results, the topics of sustainability and energy consumption (Topic 9) gained more attention in the latter periods (Figure ).
Sustainable development refers to ‘development that meets the needs of the present without compromising the ability of future generations to meet their needs’ (World Commission on Environment and Development Citation1987). After this broader macroeconomic definition, sustainability has been understood from a more microeconomic perspective, using the concept of the triple bottom line; organisational sustainability consists of the natural environment, society, and economic performance (Elkington Citation1998, Citation2004; Carter and Rogers Citation2008).
From the perspective of the product life cycle, different impact factors of sustainability are found in each lifecycle stage of mass customisation products (Badurdeen and Liyanage Citation2011; Pourabdollahian et al. Citation2014) and sustainable business model patterns are suggested (Hora et al. Citation2016). Although their importance remains, the conditions are different than those in mass production. For example, in mass customisation, things are produced only after detailed instructions are defined, such as what is necessary, when it is necessary, and what is required to achieve customer satisfaction (Boër et al. Citation2018). As such, the mass customisation strategy could prevent unwanted overproduction. On the other hand, it could also lead to lower efficiency of production and higher waste of resources, because the optimal production plan for a customised product may violate the optimal plan for all products. Therefore, a holistic approach should be used to investigate sustainability in a mass customisation environment.
Meanwhile, researchers also addressed sustainability at the micro level, focusing on AM, one of the frequently adopted manufacturing techniques in mass customisation; the comparison of environmental impacts of AM and the traditional CNC milling (Faludi et al. Citation2015) and part selection for AM (Lindemann et al. Citation2015). Some other researchers extended the discussion to a specific field such as architecture (Fisher Citation2015) and power generation (Tsigkas Citation2011).
Despite these recent advances, the following subjects have been less than satisfactory. First, in most cases, the focus has been given largely to the environmental aspect, neglecting the other pillar, social sustainability, with indicators such as working conditions and workforce, product responsibility, and relationship with the local community (Boër et al. Citation2013). For instance, the manager of a mass customisation company whose factory is near its markets could consider forming a supply chain with local suppliers as a charitable contribution to the local community.
Second, empirical and practical studies should be followed more. As an example, Hankammer, Kleer, and Piller (Citation2021) empirically revealed that mass customisation companies can nudge their customers toward more sustainable choices by designing sustainability-based starting solutions and providing sustainability information. In another example, Trentin, Forza, and Perin (Citation2015) traced a machinery manufacturing organisation as a longitudinal case study and found that synergies of pursuing a green mass customisation strategy may alleviate the difficulty of implementing that strategy.
Third, most sustainability studies of mass customisation focused on a part of the product life cycle, from a perspective of the producer, because it is difficult to trace the environmental impacts of the customised products in the usage phase. Although it is challenging, researchers could calculate these estimated values based on predefined scenarios (Betten et al. Citation2019).
5.2. Mass customisation-realising technologies and new possibilities
By analysing research attention changes regarding mass customisation topics, we noticed that one important factor that directly or indirectly stimulates research interests is the development of relevant technologies. Therefore, we further investigated technology-centred topics in the field of the mass customisation research area and analysed how they could influence the future trends and research topics in mass customisation.
5.2.1. Computer-aided design
As the level of mass customisation increases, product design elements become more customisable, from changing design parameters to tailoring with product specifications and drawings (Montreuil and Poulin Citation2005). In this manner, computer-aided design (CAD; Topic 44) has been considered one of the critical enabling technologies for a high level of mass customisation. For designers, CAD helps them adjust the values of design parameters easily, and many scholars in the apparel industry have applied CAD systems and the measurement data obtained from body-scanning systems to design personalised clothes (Istook and Hwang Citation2001; McKinney et al. Citation2017; Russel Citation2000; Su, Liu, and Xu Citation2015) and wearable products (Verwulgen et al. Citation2018).
Also, CAD offers the opportunity for customers to participate in the design process and create their own product design (Lu, Bateman, and Cheng Citation2006; Ulrich, Anderson-Connell, and Wu Citation2003). For example, a jewellery company, Jared, uses CAD software to provide 3D models to consumers to let them give their feedback about designs (Edelson Citation2021). However, due to the difficulty of learning CAD (Piegl Citation2005), little has been written about empirical analysis of participatory design using CAD.
Meanwhile, recent advances in AI technology have raised the possibility of lowering barriers to using CAD software. For example, the latest CAD programmes with AI-based algorithms support design optimisation using the parameters set by designers to suggest an array of design options that are lighter, stronger, and more economical (Langnau Citation2019). Although the marriage of AI and design software is in the early stages, it shows great potential to lower the customers’ burden in designing products for themselves. Based on this technology combination, detailed mechanisms and applications of customers’ participation in the design stage could be discussed in the near future.
5.2.2. Additive manufacturing
The coverage of AM in Figure is wider than that of other topics, given six of the 50 topics are related to AM (Topics 4, 10, 13, 14, 38, 39). Vertically, the subjects of studies that have a high proportion of AM-related topics range from tactical issues (Deradjat and Minshall Citation2018; Ituarte et al. Citation2018; Yap and Yeong Citation2014) to operational issues (Chandrasekhar et al. Citation2017; Ziegler et al. Citation2018). Horizontally, the subjects of studies that have a high proportion of AM-related topics tend to focus on production issues from the viewpoint of the manufacturers (Attaran Citation2017; Deradjat and Minshall Citation2018; Ituarte et al. Citation2018; Ziegler et al. Citation2018) or both producer- and buyer-centric perspectives (Crabtree et al. Citation2009; Pallari, Dalgarno, and Woodburn Citation2010).
From the perspective of producers, research subjects have changed with the introduction of AM’s new role in the production system. In the beginning, AM was popular as rapid prototyping to fabricate prototypes as communication and inspection tools (Kruth, Leu, and Nakagawa Citation1998; Santos et al. Citation2006). Afterward, the possible role of AM in a production system expanded to rapid tooling, the AM production of moulds and tools, which quantity requirements are insufficient to justify steel tooling (Bassoli et al. Citation2007; Levy, Schindel, and Kruth Citation2003; Ma et al. Citation2007). Until then, the research subjects were rather operational; for example, proper materials for rapid prototyping (Pilipović, Raos, and Šercer Citation2009) and rapid tooling (Ma et al. Citation2007).
After the concept of rapid manufacturing appeared, tactical issues were also addressed, such as product development (Prakash, Sridhar, and Annamalai Citation2014) and machine selection models (Roberson, Espalin, and Wicker Citation2013). Regarding factors outside the production system, after Ruffo, Tuck, and Hague (Citation2007) discussed the make-or-buy decision, researchers have studied tactical issues including the consumer-centric supply chain (Bogers, Hadar, and Bilberg Citation2016), and supply chain configuration with AM hub (Chekurov and Salmi Citation2017; Khajavi, Holmström, and Partanen Citation2018; Son, Kim, and Jeong Citation2020).
Since the functions of AM enlarged to rapid manufacturing machines, there has been a growing body of research on design for AM. This concept stems from design for assembly, and many relevant studies focused on the mass customisation companies’ interests, increasing production efficiency (Schmelzle et al. Citation2015; Chiu and Lin Citation2016) while preserving mechanical properties (Panesar et al. Citation2015). For example, in 2021, Adidas launched the 4DFWD running shoe model with a new midsole printed by AM machines. According to the company, the new midsole has a unique pattern that physically pushes runners forward and makes running feel easier (Griffiths Citation2021).
Although AM has been widely adopted in various studies as a key manufacturing technique of the production system in mass customisation, many unanswered questions remain about capability planning of mass customisation using AM (Kim and Jeong Citation2018). In Figure , strategy for capability and capacity (Topic 35) covers mostly the strategic level, whereas topics related to AM were positioned along the tactical and operational levels, which indicates that a linking area is still missing.
5.2.3. Machine learning and artificial intelligence
Recently, the technical advance of machine learning presents new possibilities for researchers and managers to overcome one of the most challenging processes for planning and scheduling in mass customisation – forecasting. In mass customisation, demand forecasting has been considered almost impossible because the manufacturer is unable to know the customers’ requirements until the actual demand occurs. Therefore, production planning and scheduling for mass customisation can be derived after the actual custom order arrives (Kim and Jeong Citation2018). However, some recent works and ongoing studies applied machine learning techniques to demand forecasting (Kim, Jeong, and Bae Citation2019; Fattahi, Dasu, and Ahmadi Citation2019) and marker estimation (Xu, Thomassey, and Zeng Citation2021). Based on recent advances, researchers may develop new methods for mass customisers to prepare and plan without knowing future customers’ requirements.
In the extension of forecasting, machine learning can also be a powerful tool to reduce the burden in the design process as well. For example, in an earlier study, Mavridou et al. (Citation2013) suggested a recommender system that reveals associations between user’s affective needs and the design parameters using data mining techniques. Recently, Wang, Zhao, and Wan (Citation2020), machine learning is used for transforming customer needs expressed in natural language to satisfactory product variants.
Outside of the research field of mass customisation, machine learning has been widely used to detect faults (Huang and Pan Citation2015) and predict outcomes at an early stage (Lieber et al. Citation2013; Weiss et al. Citation2016) to control the quality of the products (Weichert et al. Citation2019). Those methods were mostly developed for the mass production system, where plenty of learning data on the standard products can be gathered. However, in the mass customisation environment, the number of the customised product depends on the custom order, so it can be insufficient to learn the model. Therefore, traditional machine learning techniques for mass production must be modified before being applied to mass customisation production systems.
5.2.4. Identification technologies and internet-of-things
In an ideal environment for mass customisation, each type of product is identified with a unique ID. Such a production system requires advanced identification technology that can not only detect the location of each product but also share their status information. Thus, researchers of mass customisation have studied how to achieve better performance by applying identification technologies, such as radio frequency identification (RFID), to the production systems.
As the origin of the term ‘Internet of Things,’ RFID has been recognised as one of the most cost-effective technologies for object identification (Asthon Citation2010; Xu, He, and Li Citation2014). Using RFID technology, firms can integrate physical manufacturing and associated information flows (Lu, Bateman, and Cheng Citation2006; Zhong et al. Citation2013). Most studies that had a high proportion of RFID technology in mass customisation (Topic 8) are from the producer-centric view; they focus on a distributed control system (M. R. Liu et al. Citation2004), real-time manufacturing execution system (Dai et al. Citation2012), part and accessory management (Huang et al. Citation2012), and agent-based control framework (Tu et al. Citation2009).
In an article, the authors attempted to demonstrate a mass personalisation environment with RFID through the lab case (Wang et al. Citation2017). The lab demonstration represents an RFID-enabled smart factory where the personalised keychains are produced. At the beginning of the production, an RFID tag was collected together with other materials so that the RFID reader antenna at each workstation could read the tag.
However, unlike the lab case, the real-world mass customisation environment involves a larger number of product types. To handle the complicated system, sensors and RFID tags can be connected with other smarter objects (Pilloni Citation2018), or it may be required that the communication between the physical objects is expanded to include the digital counterparts residing in the cloud system (Seo et al. Citation2016; Li et al. Citation2018).
In addition, IoT-enabled production environments for mass customisation may entail the need for different management methods from those in mass production. For example, Wang et al. (Citation2017) raised a need to develop a systematic performance measurement model for an IoT-based production system. It would be interesting to examine the performance of IoT-enabled production systems for mass customisation, focusing on how the IoT level of the system can influence the system performance.
Researchers may consider methods for operational problems in mass customisation that can be solved with the IoT system. In a study of production scheduling, Lin et al. (Citation2017) presented the IoT-enabled synchronisation mechanisms for the batch production system. Likewise, synchronisation can be a powerful tool to deal with the various orders and complicated batch scheduling in mass customisation.
5.2.5. Virtual reality
Virtual reality (VR; Topic 37) enables customers to see how their products will appear and eliminates the guesswork for customers by offering features such as real-time 3D interactive views, allowing users to rotate, tilt, and magnify product images; easily obtained perception of size, layout, and match with multiple items; and compatible data format with other virtual prototyping display systems (Oh, Yoon, and Hawley Citation2004). Regarding the useful features of VR, studies have been conducted to develop a proper method for virtual prototyping and CAD and have demonstrated its effectiveness for customisation strategies (Bordegoni, Ferrise, and Lizaranzu Citation2011; Carulli, Bordegoni, and Cugini Citation2013; Füller and Matzler Citation2007; Kaiser, Vogt, and Tilebein Citation2017; Lin et al. Citation2017).
Recently, Arrighi and Mougenot (Citation2019) used augmented reality technology for precise positioning in the virtual world and combined it with intuitive physical interfaces for directed control – a mixed-reality system. Compared to traditional methods, the suggested tool helps users represent the future product accurately while offering simple, tangible user interfaces for the end user to interact and manipulate in the virtual environment intuitively. As an example, Burberry provides the online programme Burberry Bespoke, wherein customers can select detailed options for their products virtually (Sonne Citation2011). However, firms should also be aware that the customers’ act of co-configuring a product or service via VR may not be followed by their purchase (Pallant, Sands, and Karpen Citation2020), because the act of co-configuring a product or service via VR could reflect the notion of consumer play (Murray and Bellman Citation2011).
VR can be a powerful tool for designing smart factories in mass customisation as well. One example is offline programming, wherein robot movements and collision-free paths can be simulated in a virtual environment (Mitsi et al. Citation2005). Although online programming methods are needed for on-site adjustment, few studies have sought a common interface using VR (Bolano et al. Citation2020). D. Y. Kim et al. (Citation2020) developed rapid factory control prototyping to synchronise workstation design with a virtual model, but their work could be extended to connecting to VR systems.
Most scholars seem to agree that VR technology can be a powerful tool for the codesign process and smart factory design; however, relatively little is known about the economic results of such applications, including a detailed comparison of the cost and benefits for setting and utilising VR-related systems in mass customisation. Most recently, in the ongoing COVID-19 pandemic, VR has been considered an excellent tool for communication and collaboration (Javaid et al. Citation2020; Riva and Wiederhold Citation2020). It could promote the technological development of VR and foster its wider use, from unskilled individuals to professionals.
5.2.6. Human–robot collaboration
With collaborative robots (or cobots; Colgate, Peshkin, and Wannasuphoprasit Citation1996), the concept of human–robot collaboration (HRC; Topic 30) aims to integrate the flexibility of the human and the consistency of robots (Mateus et al. Citation2019). According to Müller, Vette, and Geenen’s (Citation2017) work, HRC can be classified into four states – coexistence, synchronisation, cooperation, and collaboration – and at the collaboration state, the tasks are executed by the human operator and co-bot together.
From the perspective of OM in mass customisation, HRC has been discussed mostly for the assembly line. Especially in assembly lines operated with smaller lot sizes of customised products, a robot assisted but guided by a human has both flexibility and adaptability to changing tasks, compared to fully automated lines (Bley et al. Citation2004; Krüger, Lien, and Verl Citation2009).
Thus, scholars in the latest period have conducted research on applying HRC to assembly lines in mass customisation, from proposing a conceptual planning process for automotive assembly lines with an HRC system (Scholer and Müller Citation2017) to presenting an intuitive learning framework for users (Akkaladevi, Plasch, and Pichler Citation2017). More recently, Mateus et al. (Citation2019) provided a generic methodology for the HRC assembly workspace. Their framework consists of four major stages: (a) part assembly information extraction from a CAD model, (b) work disaggregation, (c) capability assessment of human operators and machines, and based on this result, (c) generation and evaluation of collaborative product assembly sequences.
However, recent advances in the field of robotics and automated vehicles allow companies to construct production systems with greater design freedom; thanks to HRC, they are no longer confined to the assembly line. A British electric vehicle startup, Arrival, is currently building factories without conveyor belts, which have been the core of automobile factories (Boudette Citation2021). Instead, the factories have cell configurations with robots that manufacture a variety of cars to meet customer needs, with lower production cost and human labour. This trend of changing the manufacturing configuration of the automotive industry underscores the need for a transition strategy of traditional automotive factories with assembly lines. To develop transition strategies, researchers may consider how to utilise the existing capability of HRC in the current system.
Meanwhile, some studies argued that HRC could be more beneficial to small and medium-size firms with limited resources. Although HRC research has been largely driven by the electronics and automotive industries (Matheson et al. Citation2019), lower-cost cobots could be a viable option for other industries. Cencen, Verlinden, and Geraedts (Citation2018) introduced an HRC production design framework consisting of analysis, modelling, simulation, and evaluation stages. They also conducted a pilot study with the framework and showed how the HRC design methodology helped the stakeholders understand and evaluate HRC alternatives.
Moreover, researchers took on sustainability issues (Topic 9) in HRC studies. For example, Bi et al. (Citation2015) stressed the importance of sustainability when configuring an HRC environment for small and medium-size enterprises. The authors proposed a framework to support the reconfiguration of robotic systems while focusing on the 6Rs of sustainability (redesigning, reusing, remanufacturing, recovering, recycling, and reducing).
5.3. Production research strategy: a case study of the International Journal of Production Research
As an additional analysis, we chose an example journal, the International Journal of Production Research, and extended our work to investigate the research trends inside the journal to show the possibility of applying the same method in a narrower scope, such as journals.
The International Journal of Production Research (IJPR) is one of the leading journals in the areas of manufacturing, industrial engineering, operations research and management science (International Journal of Production Research Citation2022). The journal covers the production-related topics that were mostly from a perspective of a producer-centric (push), while vertically, it covers all the managerial levels from operational issues such as planning and scheduling to long-term decision-making issues such as strategies and policies, and engineering.
Like the primary analysis, we analysed the ranking changes of the 50 topics from the 73 papers related to mass customisation that was published in the IJPR. However, the publication was started from period 3 (2002–2006), so the first two periods were excluded from the analysis.
First, we compared the ratios of OM and BMS topics for the mass customisation-related papers from the IJPR journal (Table ). As a result, OM topics occupied 52.2% of 73 papers from the IJPR, which was higher than the average OM topic ratio, 41.3%, for 1,947 papers. Meanwhile, BMS topics accounted for only 7.7% of 73 papers, about 10.4% lower than the average BMS topic ratio (18.4%) of all 1,947 papers.
Table 4. Sum of BMS, OM, and other topic values and their proportions of the IJPR publications.
Second, we analysed the 73 papers from the IJPR focusing on the 50 topics of mass customisation, and the result indicated that the papers from the IJPR addressed the topics that are suited to the journal’s agenda. For example, optimisation with multi-objective models (Topic 6) ranked the top in all four periods (2002–2019), with the proportion of about three fourth of OM topics (72.01%). On average, the topic accounted for about half of the OM topics (49.94%) of all 1947 mass customisation papers. The higher proportion of topic 6 represents the research area that the journal has focused on.
In addition, in the case of IJPR publications, some topics received more research attention compared to the result analysed with all papers (Figure ). For example, one-of-kind production and supply chain management (Topic 20) and sustainability of AM (Topic 38) ranked third (5.96%) and fourth (2.53%) in the last period, respectively, much higher than the previous result. Also, assembly line for product differentiation (Topic 41) was positioned at the second rank (7.07% and 8.65%) in the fourth and fifth period (2007–2011 and 2012–2016), but it remained at the seventh (2.68% and 4.22%) in the previous result. Meanwhile, meta-heuristic methods for scheduling and routing (Topic 27) was one of the popular topics in the result of all 1947 papers, but it was one of the bottom-ranked topics in the case of IJPR (it only accounted for 0.14% on average).
Figure 9. Proportion changes in OM topics of the IJPR publications over the last four periods.
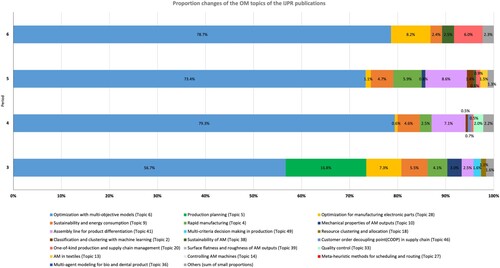
However, in terms of technologies, the papers from IJPR journals have put less weight on mass customisation-realising technologies that were addressed in Section 5.2, than the average mass customisation research papers did. Compared to the result of all 1947 papers related to mass customisation, the IJPR papers included fewer topics such as IT revolution: IoT, cloud computing, digital software, sensors (Topic 3) and virtual reality (Topic 37). The proportions of the two topics in the result of all 1,947 papers were 22.69% (Topic 3) and 8.72% (Topic 37) of other topics, but they were only 0.18% in the case of IJPR. In case of human–robot collaboration (Topic 30), it accounted for 9.29% of other topics in all mass customisation papers, but their proportion was only 6.71% for the IJPR. From the contrasting results about mass customisation-realising technologies, we suggest that future studies of mass customisation in the IJPR consider more about the environmental changes of the production systems due to emerging technologies.
6. Conclusion
Despite decades of research on mass customisation, the paradigm shift in mass customisation research has been rarely discussed. Some review articles provided valuable insights into specific research fields, but they relied primarily on the heuristic reviewing method with a limited number of selected papers. To overcome these limitations, this study used a quantitative approach to review the mass customisation literature, using text summarisation, topic modelling, and change-point detection.
From 1,947 research papers published between 1992 and 2019 that mentioned mass customisation in the title, abstract, or keywords, we drew 50 topics of the mass customisation research based on frequently used words. Afterwards, we classified them and the journals as BMS, OM, and others to prepare the analysis. According to the change-point detection method, the time horizon (from 1992 to 2019) was separated into six periods with a 5-year length. Then, we added the topic values of the papers for each topic in each period. The cumulative topic values were converted to percentages for comparison.
The result indicated that in the early days, most of the publications were from the BMS-related journals, but after, the number of publications from OM journals has drastically increased over time. The result also showed how research attention has been moved among topics in both the BMS and OM areas. Some topics, including strategy for capability and capacity (Topic 35), industry transformation (Topic 11), and rapid manufacturing (Topic 4), gained more attention over time, with increasing percentages. On the other hand, topics such as health care (Topic 19) and assembly line for product differentiation (Topic 41) lost their popularity between the first (1992–1996) and last (2017–2019) periods.
For further analysis, we plotted the popular topics in the first and last periods according to the main perspective (horizontal axis) and managerial level (vertical axis) and compared the two plots. We further discussed topics that gained or lost attention over time in the following four themes: marketing issues and consumer behaviour, advances in methodology, industry transformation, and sustainability.
In terms of the buyer-centric (pull) and producer-centric (push) perspectives, research attention has moved from the marketing strategy of the company to deal with the uncertain demands (push), to consumer behaviours and their participation in the design process (pull). The topics related to the operational methodology also have the same change due to the increased computational ability; it promoted to the appearance of meta-heuristic methods (Topic 27) and classification and clustering (Topic 2), which cover a wider range from a producer-centric perspective (push) to a buyer-centric view (pull) with custom requirements (Figure ). Recently, the topics related to industry transformation have gained more popularity. Although they are still discussed mostly at the strategic level, they consist of a wide range of subjects from a producer-centric view (push) to a buyer-centric view (pull). Meanwhile, sustainability also gets attention lately, but the related studies mostly focus on the sustainable production of the company (push).
By analysing changes in research attention regarding mass customisation topics, we noticed that one important factor that directly or indirectly stimulates research interests is the development of relevant technologies. Thus, we further investigated technology-centred topics in mass customisation research. By reviewing technological articles of computer-aided design, additive manufacturing, identification technologies and IoT, virtual reality, and human–robot interaction, we found new possibilities and future research agendas related to mass customisation.
Additionally, we chose an example journal, the IJPR, as an extension of our work to investigate the research trends of mass customisation inside the journal. The result indicates that the 73 papers from the IJPR mainly focused on optimisation with multi-objective model (Topic 6) in mass customisation, while they tended to put less weight on mass customisation-realising technologies that were addressed in Section 5.2, such as IT revolution, virtual reality, and human–-robot collaboration, than all mass customisation papers did.
This study sought to show how research attention has moved in the mass customisation area, and how emerging technologies offer a new way to shift it by realising mass customisation. By analysing topics that have gained more and less attention over time, we hope it helps to understand what prevented or promoted the growth of some research fields and find missing areas of production research. Also, we expect researchers to discover unprecedented problems and novel solution methods by understanding the influence of emerging technologies on mass customisation.
Despite this novel approach to reviewing the mass customisation literature, this study has certain limitations that indicate future research directions. First, part of the data preparation and processing required the authors’ judgment, such as topic labelling and classification. Second, in this study, a topic was assigned to only one class: BMS, OM, or others. However, a topic could belong in multiple classes using a representation tool such as fuzzy modelling. Third, the paper selection criterion overlooked the degree of importance or depth of mass customisation in the papers, so future studies should consider establishing secondary criteria to screen studies solely on mass customisation.
Disclosure statement
No potential conflict of interest was reported by the author(s).
Data availability statement
The data that support the findings of this study are available from the corresponding author upon reasonable request.
Additional information
Funding
Notes on contributors
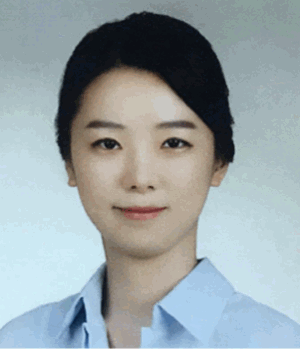
Songi Kim
Dr Songi Kim is a Visiting Professor of Makerspace Team at Institute of Convergence Science and Technology at Yonsei University, Republic of Korea. She received her Ph. D. degree in Industrial Engineering from Yonsei University, investigating production planning with additive manufacturing in the mass customisation environment. After, she has done a year of postdoctoral research in the same department and a year as a Research Professor at Yonsei University Graduate School of Communication & Art, focusing on mass customisation and optimisation for UX/UI design. Her research interests include analysis and modelling of manufacturing systems, mass customisation, and additive manufacturing
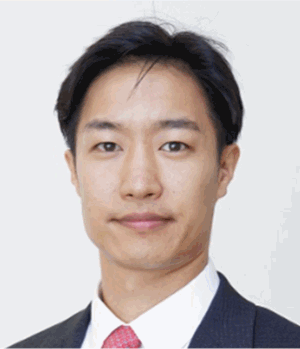
Keeheon Lee
Dr Keeheon Lee is currently an Associate Professor at Creative Technology Management, Underwood International College, Yonsei University, Korea. He is also affiliated at Graduate School of Information and Department of Innovation, Yonsei University. Additionally, he is the Director of Yonsei Digital Experience Centre, the Associate Director of Makerspace i7, and the Associate Director of Veritas Research Centre. He obtained a PhD in Industrial Engineering from Yonsei University, investigating technology analysis and forecasting using data mining and computer simulation. His teaching and research activities regard data science, computer simulation, science of science, forecasting, design-technological-social innovation, informetrics, new product development, process automation, HCI and makerspace.
References
- Agrawal, M., G. Mercer, and T. V. Kumaresh. 2001. “The False Promise of Mass Customization.” The McKinsey Quarterly 38: 62–71.
- Akkaladevi, Sharath Chandra, Matthias Plasch, and Andreas Pichler. 2017. “Skill-Based Learning of an Assembly Process.” Elektrotechnik Und Informationstechnik 134 (6): 312–315.
- Amodei, Dario, and Danny Hernandez. 2018. . “AI and Compute.” OpenAI. https://blog.openai.com/ai-and-compute/.
- Arrighi, Pierre Antoine, and Céline Mougenot. 2019. “Towards User Empowerment in Product Design: A Mixed Reality Tool for Interactive Virtual Prototyping.” Journal of Intelligent Manufacturing 30 (2): 743–754.
- Asthon, Kevin. 2010. “That ‘ Internet of Things ‘ Thing.” RFID Journal 4986. http://www.rfidjournal.com/article/print/4986.
- Atkins, Ryan, and Peter Gianiodis. 2021. “An Investigation at the Intersection of the Sharing Economy and Supply Chain Management: A Strategic Perspective.” International Journal of Logistics Research and Applications. 1–19. doi:10.1080/13675567.2021.1911970.
- Attaran, Mohsen. 2017. “The Rise of 3-D Printing: The Advantages of Additive Manufacturing Over Traditional Manufacturing.” Business Horizons 60 (5): 677–688.
- Badurdeen, Fazleena, and Jayantha P. Liyanage. 2011. “Sustainable Value Co-Creation Through Mass Customisation: A Framework.” International Journal of Sustainable Manufacturing 2 (2–3): 180–203.
- Bai, Jushan. 1997. “Estimating Multiple Breaks One at a Time.” Econometric Theory 13 (3): 315–352.
- Bassoli, Elena, Andrea Gatto, Luca Iuliano, and Maria Grazia Violante. 2007. “3D Printing Technique Applied to Rapid Casting.” Rapid Prototyping Journal 13 (3): 148–155.
- Betten, Thomas, Raed Bouslama, Daniel Wehner, and Ville Uusitalo. 2019. “Unlocking Sustainability Potentials in Product Development through Extended Knowledge and Predictions about the Product Use Phase.” In Proceedings of the Stuttgarter Symposium Für Produktentwicklung SSP, 223–232.
- Bi, Z. M., Yanfei Liu, Blane Baumgartner, Eric Culver, J. N. Sorokin, Amanda Peters, Blaine Cox, Jessica Hunnicutt, John Yurek, and Stephen O’Shaughnessey. 2015. “Reusing Industrial Robots to Achieve Sustainability in Small and Medium-Sized Enterprises (SMEs).” Industrial Robot 42 (3): 264–273.
- Blei, David M. 2012. “Probabilistic Topic Models.” In Communications of the ACM 55: 77–84.
- Bley, H., G. Reinhart, G. Seliger, M. Bernardi, and T. Korne. 2004. “Appropriate Human Involvement in Assembly and Disassembly.” CIRP Annals – Manufacturing Technology 53 (2): 487–509.
- Boër, Claudio R., Paolo Pedrazzoli, Andrea Bettoni, and Marzio Sorlini. 2013. Mass Customization and Sustainability: An Assessment Framework and Industrial Implementation. London: Springer.
- Boër, Claudio R., Claudia Redaelli, D. Boër, and M. T. Gatti. 2018. “Mass Customization and Personalization: A Way to Improve Sustainability Beyond a Common Paradox.” In Customization 4.0., edited by Stephan Hankammer, Kjeld Nielsen, Frank T. Piller, Günther Schuh, and Ning Wang, 237–250. Aachen, Germany: Springer Proceedings in Business and Economics.
- Bogers, Marcel, Ronen Hadar, and Arne Bilberg. 2016. “Additive Manufacturing for Consumer-Centric Business Models: Implications for Supply Chains in Consumer Goods Manufacturing.” Technological Forecasting and Social Change 102: 225–239.
- Bogue, Robert. 2013. “3D Printing: The Dawn of a New Era in Manufacturing?” Assembly Automation 33 (4): 307–311.
- Bolano, Gabriele, Arne Roennau, Ruediger Dillmann, and Albert Groz. 2020. “Virtual Reality for Offline Programming of Robotic Applications with Online Teaching Methods.” In 2020 17th International Conference on Ubiquitous Robots (UR), 625–630. Kyoto, Japan.
- Bordegoni, Monica, Francesco Ferrise, and Joseba Lizaranzu. 2011. “Use of Interactive Virtual Prototypes to Define Product Design Specifications: A Pilot Study on Consumer Products.” In IEEE International Symposium on Virtual Reality Innovations 2011, 11–18. Singapore: IEEE.
- Boudette, Neal E. 2021. “Arrival Developing Electric Vehicles Without Assembly Line.” The New York Times, April 21. https://www.nytimes.com/2021/04/21/business/arrival-electric-vehicles.html.
- Boyer, Ryan C., William T. Scherer, and Michael C. Smith. 2017. “Trends Over Two Decades of Transportation Research.” Transportation Research Record: Journal of the Transportation Research Board 2614 (1): 1–9.
- Calegari, Luiz Philipi, Marianne Costa Avalone, and Diego Castro Fettermann. 2020. “Barriers and Enablers to Food Mass Customization.” Journal of Agribusiness in Developing and Emerging Economies 10 (4): 403–428.
- Calegari, Luiz Philipi, and Diego de Castro Fettermann. 2018. “A Systematic Review to Identify Possible Applications and Barriers to the Adoption of Mass Customization in Food Production.” Brazilian Journal of Food Technology 21: 1–11.
- Carbone, Valentina, Aurélien Rouquet, and Christine Roussat. 2017. “The Rise of Crowd Logistics: A New Way to Co-Create Logistics Value.” Journal of Business Logistics 38 (4): 238–252. doi:10.1111/jbl.12164.
- Carter, Craig R., and Dale S. Rogers. 2008. “A Framework of Sustainable Supply Chain Management: Moving Toward New Theory.” International Journal of Physical Distribution and Logistics Management 38 (5): 360–387.
- Carulli, Marina, Monica Bordegoni, and Umberto Cugini. 2013. “An Approach for Capturing the Voice of the Customer Based on Virtual Prototyping.” Journal of Intelligent Manufacturing 24 (5): 887–903.
- Cencen, Argun, Jouke C. Verlinden, and J. M. P. Geraedts. 2018. “Design Methodology to Improve Human-Robot Coproduction in Small- and Medium-Sized Enterprises.” IEEE/ASME Transactions on Mechatronics 23 (3): 1092–1102.
- Chandrasekhar, Udayagiri, Lung Jieh Yang, Balasubramanian Esakki, Subramanian Suryanarayanan, and Sachin Salunkhe. 2017. “Rapid Prototyping of Flapping Mechanisms for Monoplane and Biplane Ornithopter Configurations.” International Journal of Modern Manufacturing Technologies 9 (2): 18–22.
- Chang, T., and H. Yang. 2017. “Latent Semantics Analysis of Research Papers on Smart Factory.” ICIC Express Letters 11 (4): 899–904.
- Chekurov, Sergei, and Mika Salmi. 2017. “Additive Manufacturing in Offsite Repair of Consumer Electronics.” Physics Procedia 89: 23–30.
- Chiu, Ming Chuan, and Yi Hsuan Lin. 2016. “Simulation Based Method Considering Design for Additive Manufacturing and Supply Chain An Empirical Study of Lamp Industry.” Industrial Management and Data Systems 116 (2): 322–348. doi:10.1108/IMDS-07-2015-0266.
- Chuang, Jason, Christopher D. Manning, and Jeffrey Heer. 2012. “Termite: Visualization Techniques for Assessing Textual Topic Models.” In Proceedings of the Workshop on Advanced Visual Interfaces AVI, 74–77. New York, NY, USA: ACM Press.
- Colgate, J. Edward, Michael A. Peshkin, and Witaya Wannasuphoprasit. 1996. “Cobots: Robots For Collaboration With Human Operators.” In 1996 ASME International Mechanical Engineering Congress and Exposition, 433–439. Atlanta, GA, USA. http://citeseerx.ist.psu.edu/viewdoc/summary?doi=10.1.1.37.7236.
- Crabtree, P., V. G. Dhokia, S. T. Newman, and M. P. Ansell. 2009. “Manufacturing Methodology for Personalised Symptom-Specific Sports Insoles.” Robotics and Computer-Integrated Manufacturing 25 (6): 972–979.
- Dai, Qingyun, Runyang Zhong, George Q. Huang, Ting Qu, T. Zhang, and T. Y. Luo. 2012. “Radio Frequency Identification-Enabled Real-Time Manufacturing Execution System: A Case Study in an Automotive Part Manufacturer.” International Journal of Computer Integrated Manufacturing 25 (1): 51–65.
- Das, Subasish, Karen Dixon, Xiaoduan Sun, Anandi Dutta, and Michelle Zupancich. 2017. “Trends in Transportation Research: Exploring Content Analysis in Topics.” Transportation Research Record 2614 (1): 27–38.
- Das, Subasish, Xiaoduan Sun, and Anandi Dutta. 2016. “Text Mining and Topic Modeling of Compendiums of Papers from Transportation Research Board Annual Meetings.” Transportation Research Record: Journal of the Transportation Research Board 2552 (1): 48–56.
- Da Silveira, Giovani, Denis Borenstein, and H. S. Fogliatto. 2001. “Mass Customization : Literature Review and Research Directions.” International Jounal of Production Economics 72 (49): 1–13.
- de Bellis, Emanuel, Christian Hildebrand, Kenichi Ito, Andreas Herrmann, and Bernd Schmitt. 2019. “Personalizing the Customization Experience: A Matching Theory of Mass Customization Interfaces and Cultural Information Processing.” Journal of Marketing Research 56 (6): 1050–1065.
- Deradjat, Dominik, and Tim Minshall. 2018. “Decision Trees for Implementing Rapid Manufacturing for Mass Customisation.” CIRP Journal of Manufacturing Science and Technology 23: 156–171.
- Duray, Rebecca, Peter T. Ward, Glenn W. Milligan, and William L. Berry. 2000. “Approaches to Mass Customization: Configurations and Empirical Validation.” Journal of Operations Management 18 (6): 605–625.
- Eastwood, Margaret A. 1996. “Implementing Mass Customization.” Computers in Industry 30 (3): 171–174.
- Edelson, Sharon. 2021. “Jared’s New Bright Spot: A Custom Jewelry Concept.” Forbes, March 9. https://www.forbes.com/sites/sharonedelson/2021/03/09/at-jareds-new-foundry-you-can-design-your-own-original-jewelry/?sh=379ead3f555b.
- Elkington, John. 1998. “Partnerships from Cannibals with Forks: The Triple Bottom Line of 21st-Century Business.” Environmental Quality Management 8 (1): 37–51.
- Elkington, John. 2004. “Enter the Triple Bottom Line.” In The Triple Bottom Line: Does It All Add Up, edited by Adrian Henriques and Julie Richardson, 1–16. London: Earthscan.
- Ellena, Thierry, Helmy Mustafa, Aleksandar Subic, and Toh Yen Pang. 2018. “A Design Framework for the Mass Customisation of Custom-Fit Bicycle Helmet Models.” International Journal of Industrial Ergonomics 64 (March): 122–133.
- Faludi, Jeremy, Cindy Bayley, Suraj Bhogal, and Myles Iribarne. 2015. “Comparing Environmental Impacts of Additive Manufacturing vs Traditional Machining via Life-Cycle Assessment.” Rapid Prototyping Journal 21 (1): 14–33.
- Fattahi, Ali, Sriram Dasu, and Reza Ahmadi. 2019. “Mass Customization and ‘Forecasting Options’ Penetration Rates Problem.” Operations Research 67 (4): 1120–1134.
- Ferguson, Scott M., Andrew T. Olewnik, and Phil Cormier. 2014. “A Review of Mass Customization Across Marketing, Engineering and Distribution Domains Toward Development of a Process Framework.” Research in Engineering Design 25 (1): 11–30.
- Fisher, Thomas. 2015. “Welcome to the Third Industrial Revolution: The Mass-Customisation of Architecture, Practice and Education.” Architectural Design 85 (4): 40–45.
- Fogliatto, Flavio S., Giovani J.C. Da Silveira, and Denis Borenstein. 2012. “The Mass Customization Decade: An Updated Review of the Literature.” International Journal of Production Economics 138 (1): 14–25.
- Fortunato, Santo, Carl T. Bergstrom, Katy Börner, James A. Evans, Dirk Helbing, Staša Milojević, Alexander M. Petersen, et al. 2018. Science of Science. American Association for the Advancement of Science.
- Füller, Johann, and Kurt Matzler. 2007. “Virtual Product Experience and Customer Participation – A Chance for Customer-Centred, Really New Products.” Technovation 27 (6–7): 378–387.
- Gilmore, James H., and B. Joseph Pine. 1997. “The Four Faces of Mass Customization.” Harvard Business Review, 1–23.
- Goldsmith, Ronald E. 1999. “The Personalised Marketplace: Beyond the 4Ps.” Marketing Intelligence & Planning 17 (4): 178–185.
- Goldsmith, Ronald E., and Jon B. Freiden. 2004. “Have It Your Way: Consumer Attitudes Toward Personalized Marketing.” Marketing Intelligence & Planning 22 (2): 228–239.
- Griffiths, Laura. 2021. “Interview: How Adidas Leveraged Carbon 3D Printing and Lattice Technology to Push Runners Forward.” TCT Magazine, May 7. https://www.tctmagazine.com/additive-manufacturing-3d-printing-news/interview-adidas-carbon-3d-printing-lattice-midsoles-push-forward/.
- Guo, Shu, Tsan Ming Choi, Bin Shen, and Sojin Jung. 2019. “Inventory Management in Mass Customization Operations: A Review.” IEEE Transactions on Engineering Management 66 (3): 412–428.
- Hankammer, Stephan, Robin Kleer, and Frank T. Piller. 2021. “Sustainability Nudges in the Context of Customer Co-Design for Consumer Electronics.” Journal of Business Economics 91: 897–933.
- Hart, Christopher W.L. 1995. “Mass Customization: Conceptual Underpinnings, Opportunities and Limits.” International Journal of Service Industry Management 6 (2): 36–45.
- Hayes, Robert H., and Steven C. Wheelwright. 1979. “Link Manufacturing Process and Product Life Cycles.” Harvard Business Review 57 (1): 133–140.
- He, David W., and Andrew Kusiak. 1997. “Design of Assembly Systems for Modular Products.” IEEE Transactions on Robotics and Automation 13 (5): 646–655.
- Hoffman, Matthew D, David M Blei, and Francis Bach. 2010. “Online Learning for Latent Dirichlet Allocation.” Advances in Neural Information Processing Systems 23: 1–9.
- Hora, Maike, Stephan Hankammer, Luca Canetta, Sultan Kaygin Sel, Shirin Gomez, and Stefan Gahrens. 2016. “Designing Business Models for Sustainable Mass Customization: A Framework Proposal.” International Journal of Industrial Engineering and Management 7 (4): 143–152.
- Hounshell, David. 1985. From the American System to Mass Production, 1850-1932. Baltimore and London: JHU Press.
- Huang, Szu Hao, and Ying Cheng Pan. 2015. “Automated Visual Inspection in the Semiconductor Industry: A Survey.” Computers in Industry 66 (January): 1–10.
- Huang, George Q., T. Qu, Yingfeng Zhang, and H. D. Yang. 2012. “RFID-Enabled Product-Service System for Automotive Part and Accessory Manufacturing Alliances.” International Journal of Production Research 50 (14): 3821–3840.
- International Journal of Production Research. 2022. “Aims and Scope.” https://www.tandfonline.com/action/journalInformation?show=aimsScope&journalCode=tprs20.
- Istook, C. L., and S. J. Hwang. 2001. “3D Body Scanning Systems with Application to the Apparel Industry.” Journal of Fashion Marketing and Management 5 (2): 120–132.
- Ituarte, Iñigo Flores, Sergei Chekurov, Jukka Tuomi, Julien Etienne Mascolo, Alessandro Zanella, Patrick Springer, and Jouni Partanen. 2018. “Digital Manufacturing Applicability of a Laser Sintered Component for Automotive Industry: A Case Study.” Rapid Prototyping Journal 24 (7): 1203–1211.
- James, C. D., and Sandeep Mondal. 2021. “Optimization of Decoupling Point Position Using Metaheuristic Evolutionary Algorithms for Smart Mass Customization Manufacturing.” Neural Computing and Applications 2021: 1–31.
- Javaid, Mohd, Abid Haleem, Raju Vaishya, Shashi Bahl, and Rajiv Suman. 2020. “Industry 4.0 Technologies and Their Applications in Fighting COVID-19 Pandemic.” Diabetes & Metabolic Syndrome: Clinical Research & Reviews 14 (4): 419–422.
- Jiao, Jianxin, and Mitchell M. Tseng. 1999. “A Methodology of Developing Product Family Architecture for Mass Customization.” Journal of Intelligent Manufacturing 10 (1): 3–20.
- Joneja, Ajay, and Neville Lee. 1998. “A Modular, Parametric Vibratory Feeder: A Case Study for Flexible Assembly Tools for Mass Customization.” IIE Transactions (Institute of Industrial Engineers) 30 (10): 923–931.
- Jost, Peter-J, and Theresa Süsser. 2020. “Company-Customer Interaction in Mass Customization.” International Journal of Production Economics 220: 107454.
- Kaiser, Christian, Simeon Vogt, and Meike Tilebein. 2017. “Virtual Development and Production Framework for Textile Orthotics.” International Journal of Computer Integrated Manufacturing 30 (7): 680–689.
- Khajavi, Siavash H., Jan Holmström, and Jouni Partanen. 2018. “Additive Manufacturing in the Spare Parts Supply Chain: Hub Configuration and Technology Maturity.” Rapid Prototyping Journal 24 (7): 1178–1192.
- Khan, Arshia, and Hans-Dietrich Haasis. 2016. “Producer–Buyer Interaction Under Mass Customization: Analysis Through Automotive Industry.” Logistics Research 9 (1): 1–7.
- Kim, Songi, and Bongju Jeong. 2018. “Mass Customization Capability Planning with Additive Manufacturing.” In Advances in Production Management Systems 2018, edited by Ilkyeong Moon, Gyu M. Lee, and Jinwoo Park, 184–192. Seoul: Springer Nature Switzerland AG.
- Kim, Myungsoo, Jongpil Jeong, and Sangmin Bae. 2019. “Demand Forecasting Based on Machine Learning for Mass Customization in Smart Manufacturing.” In ACM International Conference Proceeding Series, 6–11. Association for Computing Machinery.
- Kim, D. Y., J. W. Park, S. Baek, K. B. Park, H. R. Kim, J. I. Park, H. S. Kim, et al. 2020. “A Modular Factory Testbed for the Rapid Reconfiguration of Manufacturing Systems.” Journal of Intelligent Manufacturing 31 (3): 661–680.
- Kotha, Suresh. 1995. “Mass Customization – Implementing the Emerging Paradigm for Competitive Advantage.” Strategic Management Journal 16: 21–42.
- Krishnan, V., R. Singh, and D. Tirupati. 1999. “A Model-Based Approach for Planning and Developing a Family of Technology-Based Products.” Manufacturing & Service Operations Management 1 (2): 132–156.
- Krüger, J., T. K. Lien, and A. Verl. 2009. “Cooperation of Human and Machines in Assembly Lines.” CIRP Annals – Manufacturing Technology 58 (2): 628–646.
- Kruth, Jean-Pierre, Ming-Chuan Leu, and Terunaga Nakagawa. 1998. “Progress in Additive Manufacturing and Rapid Prototyping.” CIRP Annals – Manufacturing Technology 47 (2): 525–540.
- Kumar, Soumojit, and Ashis Kumar Chatterjee. 2013. “A Heuristic-Based Approach to Integrate the Product Line Selection Decision to the Supply Chain Configuration.” International Journal of Production Research 51 (8): 2399–2413.
- Lampel, Joseph, and Henry Mintzberg. 1996. “Customizing Customization.” Sloan Management Review 38 (1): 21–30.
- Langnau, Leslie. 2019. “CAD and AI: Making Design Better, Faster, and Easier.” Design World, September 3. https://www.designworldonline.com/cad-and-ai-making-design-better-faster-and-easier/.
- Lee, Keeheon, and Hosang Jung. 2019. “Dynamic Semantic Network Analysis for Identifying the Concept and Scope of Social Sustainability.” Journal of Cleaner Production 233 (October): 1510–1524.
- Lee, Keeheon, Hyojung Jung, and Min Song. 2016. “Subject–Method Topic Network Analysis in Communication Studies.” Scientometrics 109 (3): 1761–1787.
- Lee, Keeheon, and Chang Ouk Kim. 2014. “Topic Recipe-Based Social Simulation for Research Dynamics Analysis.” In Social Simulation Conference, 1–3. Bellaterra: Cerdanyola del Vallès.
- Lee, Keeheon, SuYeon Kim, Erin Hea-Jin Kim, and Min Song. 2017. “Comparative Evaluation of Bibliometric Content Networks by Tomographic Content Analysis: An Application to Parkinson’s Disease.” Journal of the Association for Information Science and Technology 68 (5): 1295–1307.
- Lei, Tiaoyu, Weiping Peng, Jin Lei, and Qing Xiao. 2016. “A Similarity Analysis Method of the Non-Isomorphism Generalized Module in Product Platform.” Proceedings of the Institution of Mechanical Engineers, Part B: Journal of Engineering Manufacture 232 (2): 358–370.
- Leng, Jiewu, and Pingyu Jiang. 2019. “Dynamic Scheduling in RFID-Driven Discrete Manufacturing System by Using Multi-Layer Network Metrics as Heuristic Information.” Journal of Intelligent Manufacturing 30 (3): 979–994.
- Levy, Gideon N., Ralf Schindel, and J. P. Kruth. 2003. “Rapid Manufacturing and Rapid Tooling with Layer Manufacturing (LM) Technologies, State of the Art and Future Perspectives.” CIRP Annals – Manufacturing Technology 52 (2): 589–609.
- Leydesdorff, Loet, and Adina Nerghes. 2017. “Co-Word Maps and Topic Modeling: A Comparison Using Small and Medium-Sized Corpora (N < 1,000).” Journal of the Association for Information Science and Technology 68 (4): 1024–1035.
- Li, Tianyang, Ting He, Zhongjie Wang, and Yufeng Zhang. 2018. “An Approach to Iot Service Optimal Composition for Mass Customization on Cloud Manufacturing.” IEEE Access 6: 50572–50586.
- Lieber, Daniel, Marco Stolpe, Benedikt Konrad, Jochen Deuse, and Katharina Morik. 2013. “Quality Prediction in Interlinked Manufacturing Processes Based on Supervised & Unsupervised Machine Learning.” Procedia CIRP 7 (January): 193–198.
- Lin, Yuan, Shiqiang Yu, Pai Zheng, Liming Qiu, Yuanbin Wang, and Xun Xu. 2017. “VR-Based Product Personalization Process for Smart Products.” Procedia Manufacturing 11 (June): 1568–1576.
- Lincoln, Thomas L., and Carl Builder. 1998. “Global Healthcare and the Flux of Technology.” Studies in Health Technology and Informatics 52: 1213–1217.
- Lindemann, Christian, Thomas Reiher, Ulrich Jahnke, and Rainer Koch. 2015. “Towards a Sustainable and Economic Selection of Part Candidates for Additive Manufacturing.” Rapid Prototyping Journal 21 (January): 216–227.
- Liu, Weibo, Yan Jin, and Mark Price. 2018. “New Meta-Heuristic for Dynamic Scheduling in Permutation Flowshop with New Order Arrival.” International Journal of Advanced Manufacturing Technology 98 (5–8): 1817–1830.
- Liu, Xiaotong, Kai Zhang, Bokui Chen, Jun Zhou, and Lixin Miao. 2018. “Analysis of Logistics Service Supply Chain for the One Belt and One Road Initiative of China.” Transportation Research Part E: Logistics and Transportation Review 117 (February): 23–39.
- Liu, Michael R., Q. L. Zhang, Lionel M. Ni, and Mitchell M. Tseng. 2004. “An RFID-Based Distributed Control System for Mass Customization Manufacturing.” In International Symposium on Parallel and Distributed Processing and Applications, Vol. 3358, 1039–1049. Berlin, Heidelberg: Springer.
- Lohmann, Matheus, Michel Jose Anzanello, Flavio Sanson Fogliatto, and Giovani Caetano da Silveira. 2019. “Grouping Workers with Similar Learning Profiles in Mass Customization Production Lines.” Computers and Industrial Engineering 131 (May): 542–551.
- Lu, B. H., R. J. Bateman, and K. Cheng. 2006. “RFID Enabled Manufacturing: Fundamentals, Methodology and Applications.” International Journal of Agile Systems and Management 1 (1): 73–92.
- Ma, S., I. Gibson, G. Balaji, and Q. J. Hu. 2007. “Development of Epoxy Matrix Composites for Rapid Tooling Applications.” Journal of Materials Processing Technology 192–193: 75–82.
- MacDuffie, John Paul, Kannan Sethuraman, and Marshall L Fisher. 1996. “Product Variety and Manufacturing Performance: Evidence from the International Automotive Assembly Plant Study.” Management Science 42 (3): 350–369.
- Mateus, João Costa, Dieter Claeys, Veronique Limère, Johannes Cottyn, and El Houssaine Aghezzaf. 2019. “A Structured Methodology for the Design of a Human-Robot Collaborative Assembly Workplace.” International Journal of Advanced Manufacturing Technology 102 (5–8): 2663–2681.
- Matheson, Eloise, Riccardo Minto, Emanuele G.G. Zampieri, Maurizio Faccio, and Giulio Rosati. 2019. “Human-Robot Collaboration in Manufacturing Applications: A Review.” Robotics 8 (4): 1–25.
- Mavridou, Efthimia, Dionisis D. Kehagias, Dimitrios Tzovaras, and George Hassapis. 2013. “Mining Affective Needs of Automotive Industry Customers for Building a Mass-Customization Recommender System.” Journal of Intelligent Manufacturing 24 (2): 251–265.
- McKinney, Ellen, Simeon Gill, Amy Dorie, and Shannon Roth. 2017. “Body-to-Pattern Relationships in Women’s Trouser Drafting Methods.” Clothing and Textiles Research Journal 35 (1): 16–32.
- Minvielle, Etienne, Mathias Waelli, Claude Sicotte, and John R. Kimberly. 2014. “Managing Customization in Health Care: A Framework Derived from the Services Sector Literature.” Health Policy 117 (2): 216–227.
- Mitsi, S., K. D. Bouzakis, G. Mansour, D. Sagris, and G. Maliaris. 2005. “Off-Line Programming of an Industrial Robot for Manufacturing.” International Journal of Advanced Manufacturing Technology 26 (3): 262–267.
- Montreuil, Benoit, and Marc Poulin. 2005. “Demand and Supply Network Design Scope for Personalized Manufacturing.” Production Planning & Control 16 (5): 454–469.
- Moore, J. T. 1950. “Standardization and Simplification in the Electrical Industry.” Proceedings of the IEE - Part I: General 97 (107): 223–230.
- Mueller-Heumann, Guenther. 1992. “Market and Technology Heumann Shifts in the 1990s : Market Professor of Marketing, Fragmentation and Mass.” Journal of Marketing 8 (4): 303–315.
- Müller, Rainer, Matthias Vette, and Aaron Geenen. 2017. “Skill-Based Dynamic Task Allocation in Human-Robot-Cooperation with the Example of Welding Application.” Procedia Manufacturing 11 (January): 13–21.
- Murray, Kyle B., and Steven Bellman. 2011. “Productive Play Time: The Effect of Practice on Consumer Demand for Hedonic Experiences.” Journal of the Academy of Marketing Science 39 (3): 376–391. doi:10.1007/s11747-010-0205-6.
- Oh, Hyunjoo, So-Yeon Yoon, and Jana Hawley. 2004. “What Virtual Reality Can Offer to the Furniture Industry.” Journal of Textile and Apparel, Technology and Management 4 (1): 1–7.
- Pallant, Jessica L., Sean Sands, and Ingo Oswald Karpen. 2020. “The 4Cs of Mass Customization in Service Industries: A Customer Lens.” Journal of Services Marketing 34 (4): 499–511.
- Pallari, Jari H.P., Kenneth W. Dalgarno, and James Woodburn. 2010. “Mass Customization of Foot Orthoses for Rheumatoid Arthritis Using Selective Laser Sintering.” IEEE Transactions on Biomedical Engineering 57 (7): 1750–1756.
- Panesar, Ajit, David J. Brackett, Ian A. Ashcroft, Ricky D. Wildman, and Richard J. Hague. 2015. “Design Framework for Multifunctional Additive Manufacturing: Placement and Routing of 3D Printed Circuit Volumes.” Journal of Mechanical Design 137 (November): 1–10. doi:10.1115/1.4030996.
- Partanen, Jari, and Harri Haapasalo. 2004. “Fast Production for Order Fulfillment: Implementing Mass Customization in Electronics Industry.” International Journal of Production Economics 90 (2): 213–222.
- Pedregosa, Fabian, Gaël Varoquaux, Alexandre Gramfort, Vincent Michel, Bertrand Thirion, Olivier Grisel, Mathieu Blondel, et al. 2011. “Scikit-Learn: Machine Learning in Python.” Journal of Machine Learning Research 12. http://scikit-learn.sourceforge.net.
- Piegl, Les A. 2005. “Ten Challenges in Computer-Aided Design.” CAD Computer Aided Design 37 (4): 461–470.
- Pilipović, Ana, Pero Raos, and Mladen Šercer. 2009. “Experimental Analysis of Properties of Materials for Rapid Prototyping.” International Journal of Advanced Manufacturing Technology 40 (1–2): 105–115.
- Pilloni, Virginia. 2018. “How Data Will Transform Industrial Processes: Crowdsensing, Crowdsourcing and Big Data as Pillars of Industry 4.0.” Future Internet 10 (4): 1–14.
- Pine B. Joseph. 1993. “Mass Customizing Products and Services.” Planning Review 21 (4): 6–55. doi:10.1108/eb054420.
- Pine, B. Joseph, Bart Pictor, and Andrew C. Boynton. 1993. “Making Mass Customization Work.” Harvard Business Review 71 (5): 108–119.
- Pourabdollahian, Golboo, Frank Steiner, Ole Horn Rasmussen, and Stephan Hankammer. 2014. “A Contribution Toward a Research Agenda: Identifying Impact Factors of Mass Customization on Environmental Sustainability.” International Journal of Industrial Engineering and Management 5 (4): 169–178.
- Prakash, Wankhade Nitesh, V. G. Sridhar, and K. Annamalai. 2014. “New Product Development by DFMA and Rapid Prototyping.” ARPN Journal of Engineering and Applied Sciences 9 (3): 274–279.
- Pyke, David F., and Morris A. Cohen. 1990. “Push and Pull in Manufacturing and Distribution Systems.” Journal of Operations Management 9 (1): 24–43.
- Relich, Marcin, and Pawel Pawlewski. 2018. “A Case-Based Reasoning Approach to Cost Estimation of New Product Development.” Neurocomputing 272: 40–45.
- Reynolds, Elisabeth B. 2017. “Innovation and Production: Advanced Manufacturing Technologies, Trends and Implications for US Cities and Regions.” Built Environment 43 (1): 25–43.
- Richter, Chris, Sascha Kraus, Alexander Brem, Susanne Durst, and Clemens Giselbrecht. 2017. “Digital Entrepreneurship: Innovative Business Models for the Sharing Economy.” Creativity and Innovation Management 26 (3): 300–310. doi:10.1111/caim.12227.
- Riva, Giuseppe, and Brenda K. Wiederhold. 2020. “How Cyberpsychology and Virtual Reality Can Help Us to Overcome the Psychological Burden of Coronavirus.” Cyberpsychology, Behavior, and Social Networking 23 (5): 277–279.
- Roberson, D., D. Espalin, and R. B. Wicker. 2013. “3D Printer Selection: A Decision-Making Evaluation and Ranking Model.” Virtual and Physical Prototyping 8 (3): 201–212.
- Robinson, James C. 2001. “The End of Managed Care.” Journal of the American Medical Association 285 (20): 2622–2628.
- Rubinovitz, Jacob, Joseph Bukchin, and Ehud Lenz. 1993. “RALB – A Heuristic Algorithm for Design and Balancing of Robotic Assembly Lines.” CIRP Annals – Manufacturing Technology 42 (1): 497–500.
- Ruffo, Massimiliano, Chris Tuck, and Richard Hague. 2007. “Make or Buy Analysis for Rapid Manufacturing.” Rapid Prototyping Journal 13 (1): 23–29.
- Russel, E. 2000. “Body Scanning: Beyond the Research.” World Clothing Manufacturer 81 (1): 13–15.
- Salvador, F., C. Forza, and M. Rungtusanatham. 2002. “Modularity, Product Variety, Production Volume, and Component Sourcing: Theorizing Beyond Generic Prescriptions.” Journal of Operations Management 20 (5): 549–575.
- Santos, Edson Costa, Masanari Shiomi, Kozo Osakada, and Tahar Laoui. 2006. “Rapid Manufacturing of Metal Components by Laser Forming.” International Journal of Machine Tools and Manufacture 46 (12–13): 1459–1468.
- Sastry, Girish, Jack Clark, Greg Brockmman, and Ilya Sutskever. 2019. “AI and Compute – Addendum.” OpenAI.
- Sawik, Tadeusz. 2000. “An LP-Based Approach for Loading and Routing in a Flexible Assembly Line.” International Journal of Production Economics 64 (1–3): 49–58.
- Schmelzle, John, Eric V. Kline, Corey J. Dickman, Edward W. Reutzel, Griffin Jones, and Timothy W. Simpson. 2015. “(Re)Designing for Part Consolidation: Understanding the Challenges of Metal Additive Manufacturing.” Journal of Mechanical Design, Transactions of the ASME 137 (11): 1–12. doi:10.1115/1.4031156.
- Scholer, Matthias, and Rainer Müller. 2017. “Modular Configuration and Control Concept for the Implementation of Human-Robot-Cooperation in the Automotive Assembly Line.” In IFAC(International Federation of Automatic Control) – PapersOnLine 50: 5694–5699.
- Scimago Journal & Country Rank. 2019. Accessed December 15. https://www.scimagojr.com/.
- Seo, Dong Bum, You Boo Jeon, Song Hee Lee, and Keun Ho Lee. 2016. “Cloud Computing for Ubiquitous Computing on M2M and IoT Environment Mobile Application.” Cluster Computing 19 (2): 1001–1013.
- Sievert, Carson, and Kenneth Shirley. 2014. “LDAvis: A Method for Visualizing and Interpreting Topics.” In Proceedings of the Workshop on Interactive Language Learning, Visualization, and Interfaces, 63–70. Baltimore, Maryland, USA.
- Son, Dongmin, Songi Kim, and Bongju Jeong. 2020. “Sustainable Part Consolidation Model for Customized Products in Closed-Loop Supply Chain with Additive Manufacturing Hub.” Additive Manufacturing 37: 101643.
- Song, Min, SuYeon Kim, and Keeheon Lee. 2017. “Ensemble Analysis of Topical Journal Ranking in Bioinformatics.” Journal of the Association for Information Science and Technology 68 (6): 1564–1583.
- Sonne, Paul. 2011. “Burberry Bespoke Tries to Sell Luxury Shoppers On Customized Trench Coats – WSJ.Com.” The Wall Street Journal, November 3. http://online.wsj.com/news/articles/SB10001424052970203804204577013842801187070.
- Su, Junqiang, Guolian Liu, and Bugao Xu. 2015. “Development of Individualized Pattern Prototype Based on Classification of Body Features.” International Journal of Clothing Science and Technology 27 (6): 895–907.
- Suarez, F. F., M. A. Cusumano, and C. H. Fine. 1996. “An Empirical Study of Manufacturing Flexibility in Printed Circuit Board Assembly.” Operations Research 44 (1): 223–240.
- Sun, Lijun, and Yafeng Yin. 2017. “Discovering Themes and Trends in Transportation Research Using Topic Modeling.” Transportation Research Part C: Emerging Technologies 77 (April): 49–66.
- Szabó, Katalin. 2017. “Institutional Entrepreneurship: Agents’ Ability and Activity for Building Up New Institutions by Combining Existing Elements.” Society and Economy 39 (3): 359–383.
- Thompson, Mark, and Rhoda Nussbaum. 2000. “An HMO Survey on Mass Customization of Healthcare Delivery for Women.” Women’s Health Issues 10 (1): 10–19.
- Trentin, Alessio, Cipriano Forza, and Elisa Perin. 2015. “Embeddedness and Path Dependence of Organizational Capabilities for Mass Customization and Green Management: A Longitudinal Case Study in the Machinery Industry.” International Journal of Production Economics 169: 253–276.
- Truong, Charles, Laurent Oudre, and Nicolas Vayatis. 2020. “Selective Review of Offline Change Point Detection Methods.” Signal Processing 167: 107299.
- Tseng, Mitchell M, and Jianxin Jiao. 1998. “Design for Mass Customization by Developing Product Family Architecture.” In Design Engineering Technical Conferences-Design Theory and Methodology, 1–19. Atlanta: American Society of Mechanical Engineers.
- Tsigkas, Alexander. 2011. “Open Lean Electricity Supply Communities: A Paradigm Shift for Mass Customizing Electricity Markets.” Energy Systems 2 (3–4): 407–422.
- Tu, Mengru Arthur, Jia Hong Lin, Ruey Shun Chen, Kai Ying Chen, and Jung Sing Jwo. 2009. “Agent-Based Control Framework for Mass Customization Manufacturing with UHF RFID Technology.” IEEE Systems Journal 3 (4): 551.
- Turner, Frances, Aurélie Merle, and David Gotteland. 2020. “Enhancing Consumer Value of the Co-Design Experience in Mass Customization.” Journal of Business Research 117 (May): 473–483.
- Ulrich, Pamela V., Lenda Jo Anderson-Connell, and Weifang Wu. 2003. “Consumer Co-Design of Apparel for Mass Customization.” Journal of Fashion Marketing and Management 7 (4): 398–412.
- Verwulgen, Stijn, Daniël Lacko, Jochen Vleugels, Kristof Vaes, Femke Danckaers, Guido De Bruyne, and Toon Huysmans. 2018. “A New Data Structure and Workflow for Using 3D Anthropometry in the Design of Wearable Products.” International Journal of Industrial Ergonomics 64: 108–117.
- Wang, Juite, and Chih Chi Hsu. 2021. “A Topic-Based Patent Analytics Approach for Exploring Technological Trends in Smart Manufacturing.” Journal of Manufacturing Technology Management 32 (1): 110–135.
- Wang, Yi, Hai Shu Ma, Jing Hui Yang, and Ke Sheng Wang. 2017. “Industry 4.0: A Way from Mass Customization to Mass Personalization Production.” Advances in Manufacturing 5 (4): 311–320.
- Wang, Yue, Wenlong Zhao, and Wayne Xinwei Wan. 2020. “Needs-Based Product Configurator Design for Mass Customization Using Hierarchical Attention Network.” IEEE Transactions on Automation Science and Engineering 18 (1): 195–204.
- Weichert, Dorina, Patrick Link, Anke Stoll, Stefan Rüping, Steffen Ihlenfeldt, and Stefan Wrobel. 2019. “A Review of Machine Learning for the Optimization of Production Processes.” The International Journal of Advanced Manufacturing Technology 104: 1889–1902.
- Weiss, Sholom M., Amit Dhurandhar, Robert J. Baseman, Brian F. White, Ronald Logan, Jonathan K. Winslow, and Daniel Poindexter. 2016. “Continuous Prediction of Manufacturing Performance Throughout the Production Lifecycle.” Journal of Intelligent Manufacturing 27 (4): 751–763.
- Woodward, Joan. 1965. Industrial Organization: Theory and Practice. 1st ed. Oxford University Press. https://books.google.co.kr/books/about/Industrial_Organization_Theory_and_Pract.html?id=Cx0uAAAAMAAJ&redir_esc=y.
- World Commission on Environment and Development. 1987. Our Common Future. New York: Oxford University Press.
- Xiong, Hui, Yi Cheng, Wenhao Zhao, and Jianhua Liu. 2019. “Analyzing Scientific Research Topics in Manufacturing Field Using a Topic Model.” Computers and Industrial Engineering 135 (September): 333–347.
- Xu, Li Da, Wu He, and Shancang Li. 2014. “Internet of Things in Industries: A Survey.” IEEE Transactions on Industrial Informatics 10 (4): 2233–2243.
- Xu, Yanni, Sébastien Thomassey, and Xianyi Zeng. 2021. “Machine Learning-Based Marker Length Estimation for Garment Mass Customization.” International Journal of Advanced Manufacturing Technology 113 (11–12): 3361–3376.
- Yang, Hyun-Lim, Tai-Woo Chang, and Yerim Choi. 2018. “Exploring the Research Trend of Smart Factory with Topic Modeling.” Sustainability 10 (8): 2779.
- Yap, Y. L., and W. Y. Yeong. 2014. “Additive Manufacture of Fashion and Jewellery Products: A Mini Review.” Virtual and Physical Prototyping 9 (3): 195–201.
- Zhao, Fangfang, Zhen He, and Du Wu. 2008. “Quality Assurance of Mass Customization: A State-of-the-Art Review.” In 2008 IEEE Symposium on Advanced Management of Information for Globalized Enterprises (AMIGE), 316–318. Tianjin.
- Zhao, Huiwen, Leigh McLoughlin, Valery Adzhiev, and Alexander Pasko. 2019a. “3D Mass Customization Toolkits Design, Part II: Heuristic Evaluation of Online Toolkits.” Computer-Aided Design and Applications 16 (2): 223–242.
- Zhao, Huiwen, Leigh McLoughlin, Valery Adzhiev, and Alexander Pasko. 2019b. “‘Why Do We Not Buy Mass Customised Products?’ – An Investigation of Consumer Purchase Intention of Mass Customised Products.” International Journal of Industrial Engineering and Management 10 (2): 181–190.
- Zhong, Ray Y., Q. Y. Dai, T. Qu, G. J. Hu, and George Q. Huang. 2013. “RFID-Enabled Real-Time Manufacturing Execution System for Mass-Customization Production.” Robotics and Computer-Integrated Manufacturing 29 (2): 283–292.
- Ziegler, Tobias, Jhina Hernandez Marquez, Raimund Jaeger, and Somphong Phommahavong. 2018. “Wear Mechanisms and Abrasion Rates in Selective Laser Sintering Materials.” Polymer Testing 67 (February): 545–550.
- Zipkin, Paul. 1997. “The Limits of Mass Customization.” Harvard Business Review 75 (2): 91–101.
- Zipkin, Paul. 2001. “The Limits of Mass Customization.” MIT Sloan Management Review 42 (3): 81–87.